Clustered data conform to a hierarchical or nested structure in which, in its simplest form (the univariable two-level structure), the value of a single response variable is measured on a number of level 1 units contained in different groups or clusters (level 2 units). For example, the level 1 and level 2 units, respectively, may be teeth in a mouth, knees in a patient, patients in a hospital, clinics in a region, children in a class, successive visit times for a patient (i.e. longitudinal data, Fig. 41.1), etc. The statistical analysis of such repeated measures data should take into account the fact that the observations in a cluster tend to be correlated, i.e. they are not independent. Failure to acknowledge this usually results in underestimation of the standard errors of the estimates of interest and, consequently, confidence intervals that are too narrow and P-values that are too small, leading to increased Type I error rates.
Figure 41.1 Diagrammatic representation of a two-level hierarchical structure for longitudinal data.

For the purposes of illustration, we shall assume, in this chapter, that we have longitudinal data and our repeated measures data comprise each patient’s values of the variable at different time points, i.e. the patient is the cluster. We summarize the data by describing the patterns in individual patients, and, if relevant, assess whether these patterns differ between two or more groups of patients.
Displaying the Data
A plot of the measurement against time for each patient in the study provides a visual impression of the pattern over time. When we are studying only a small group of patients, it may be possible to show all the individual plots in one diagram. However, when we are studying large groups this becomes difficult, and we may illustrate just a selection of ‘representative’ individual plots (Fig. 41.3), perhaps in a grid for each treatment group. Note that the average pattern generated by plotting the means over all patients at each time point may be very different from the patterns seen in individual patients.
Comparing Groups: Inappropriate Analyses
It is inappropriate to use all the values in a group to fit a single linear regression line (Chapters 27 and 28) or to perform a one-way analysis of variance (ANOVA, Chapter 22) to compare groups because these methods do not take account of the repeated measurements on the same patient. Furthermore, it is also incorrect to compare the means in the groups at each time point separately using unpaired t-tests (Chapter 21) or one-way ANOVA for a number of reasons:
- The measurements in a patient from one time point to the next are not independent, so interpretation of the results is difficult. For example, if a comparison is significant at one time point, then it is likely to be significant at other time points, irrespective of any changes in the values in the interim period.
- The large number of tests carried out implies that we are likely to obtain significant results purely by chance (Chapter 18).
- We lose information about within-patient changes.
Comparing Groups: Appropriate Analyses
Using Summary Measures
We can base our analysis on a summary measure that captures the important aspects of the data, and calculate this summary measure for each patient. Typical summary measures are:
- change from baseline at a pre-determined time point;
- maximum (peak) or minimum (nadir) value reached;
- time to reach the maximum (or minimum) value;
- time to reach some other pre-specified value;
- average value (e.g. mean);
- area under the curve (AUC, Fig. 41.2);
- slope or intercept of the patient’s regression line (describing the relationship between the measurement and time).
Figure 41.2 Calculation of the AUC for a single student. The total area under the line can be divided into a number of rectangles and triangles (marked a to j). The area of each can easily be calculated. Total AUC = Area (a) + Area (b) + … + Area (j).

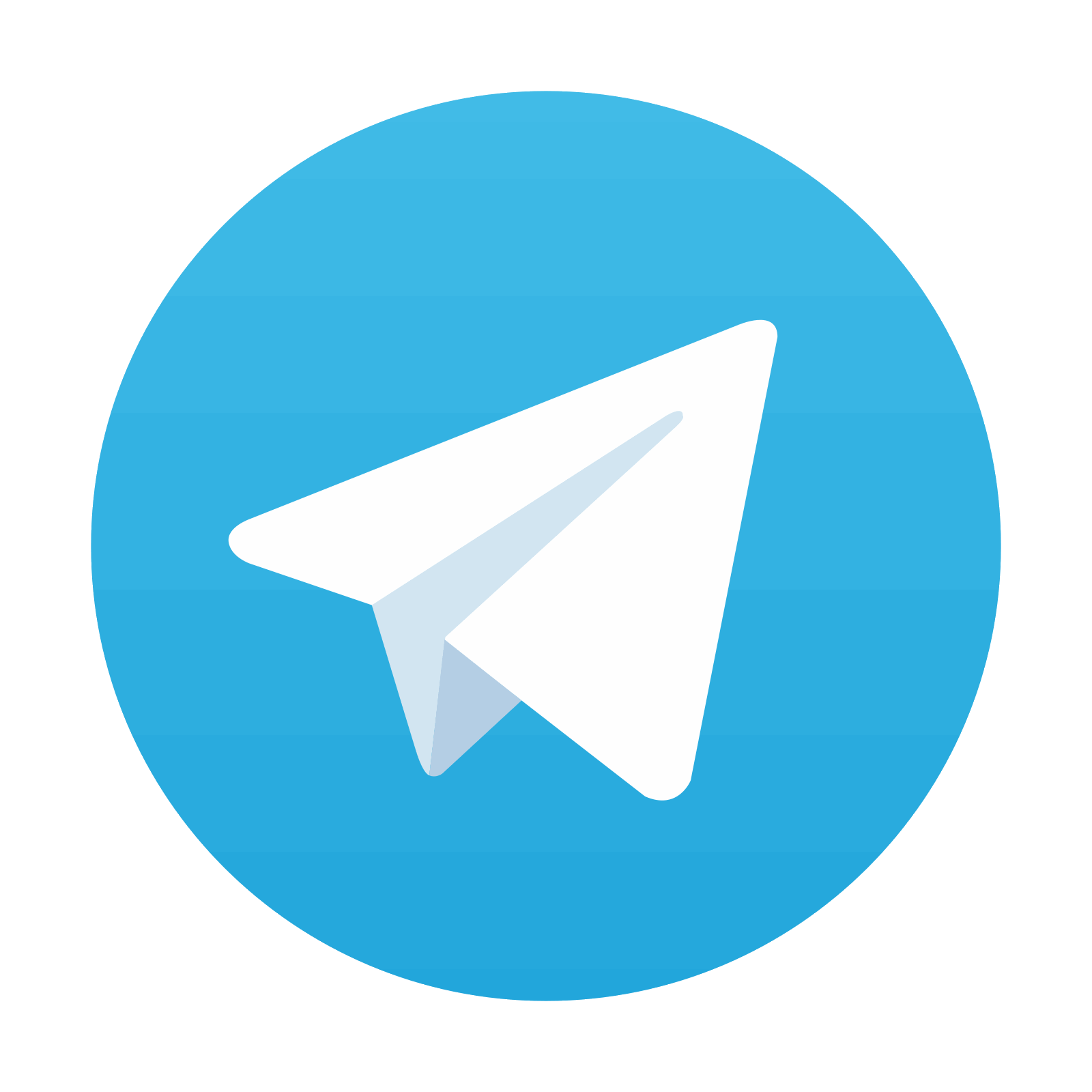
Stay updated, free articles. Join our Telegram channel

Full access? Get Clinical Tree
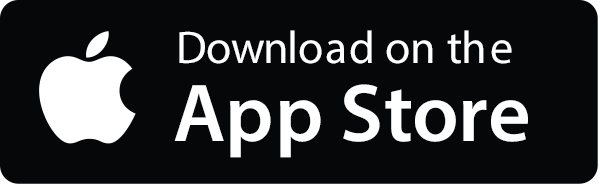
