What Is Metabolomics?
Metabolomics is the newest addition to the “omics” science. An “omics” has been defined as a neologism referring to a holistic view on biologic macromolecules, such as in genomics or proteomics. Genomics aims to understand the structure and function of the genome by studying all nucleotide sequences, including the structural genes, regulatory sequences, and noncoding DNA sequences in the chromosomes of any organism. It also examines the molecular mechanisms that maintain genomic integrity and allow its transmission and the expression including any interplay of genetic and environmental factors in disease. Proteomics involves the identification and study of the complete set of proteins in a species and the determination of their role in physiologic and pathophysiologic functions. Together with these and other “omics” technologies, metabolomics contribute to the detailed understanding of the in vivo function of gene products, biochemical analysis, and regulatory networks. The metabolomics represents the collection of all low-molecular-weight molecules found in a given cell and can provide a snapshot of the physiology of a cell at a given time during development and differentiation including responses to food, drugs, and other challenges.
Biochemists view metabolomics as metabolites profiling or the quantitative measurement of the dynamic multiparametric metabolic response of living systems to pathophysiological stimuli or genetic modification. Biologists, particularly geneticists, on the other hand, view it as the science of a highly complex and organized biochemical network in which small molecules, such as metabolic substrates and products, lipids, small peptides, vitamins, amino acids, signaling molecules, and other protein cofactors, are interacting between them and with other biological macromolecules in the metabolome. These small molecules are acting usually at very low concentrations in tissue-signaling functions. Along with the understanding of the in vivo interaction of gene products, metabolomics also contributes a great deal to the mathematical description and simulation of the whole cell in the systems biology approach. Systems biology tries to integrate genomic, proteomic, transcriptomic, and metabolomic information to give a more complete picture of living organisms ( Fig. 14.1 ). Here, the biological events in organisms are systematically interpreted through the combination of complex measurements from various methods resulting in high-throughput data. In this chapter, we discuss addiction as a problem of systems biology with emphasis on metabolomics.

Substance Abuse and Its Effect on Health and Economy
Substance use, abuse, and addiction that include but are not limited to alcohol, nicotine, opioids, cocaine, cannabinoids, methamphetamine, and amphetamine, continue to be a significant public health concern and pose tremendous cost to our society. In the United States alone, in 1998, the economic cost associated with illicit drug use was estimated to be US$280 billion, for nicotine US$158 billion, and for alcohol abuse US$185 billion, with an average annual increase of 3.8% per year. This brings the combined total estimated economic impact of substance abuse in the United States to over half a trillion dollars. Recent data indicate that approximately 1.6 million people in the United States abuse or are dependent on prescription opioids. In the United Kingdom, the cost associated with alcohol abuse is approximately $39 billion each year. Drug and alcohol abuse is a major cause of morbidity and mortality both in the United States and worldwide. Alcohol use disorders including liver and heart diseases account for 4% of the global burden of disease and cause 1.8 million deaths. The excessive burden of drug abuse on our health and economy makes it necessary for us to better understand how these drugs affect the metabolomics of our cellular systems, mechanisms of action in different parts of the body, and factors that determine the variability of addictive responses. A better insight into these mechanisms will offer a better understanding of the problem as well as identify effective treatment and preventative approaches to addiction, which remains insidious in most societies.
Substance Abuse Leads to Addiction
At the center of the problem of substance abuse is addiction to these substances. It has been long hypothesized that the combination of genetic and environmental factors following drug use and abuse alters cellular physiology. This alteration is expected to be cell and organ specific. In due course, it may follow physiological adaptation, leading to an urge for the drug response and the development of addiction. Evidence for the involvement of genes in the drug addiction process comes from classical epidemiological and genetic studies. Data from both animal and humans support the relevance of genetic influences in substance abuse and dependence. Twin studies, for example, have shown robust genetic components for alcohol, opiate, cocaine, and tobacco addictions.
The major target of virtually all drugs in the human or animal body, either directly or indirectly, is the nervous system—specifically one or more pathways deep within the brain. Drug-related, especially alcohol-related, brain damage and associated neuropsychological changes have been well documented (see Oscar-Berman et al. ). There is increasing evidence that long-lasting changes in the brain result from the progression of casual user to addict. Acute drug intoxication is accompanied by highly localized and dynamic patterns of brain activation and deactivation, as well as complex cascades of transcriptional reprogramming.
All compounds with abuse potential have the ability to disrupt information processing in the brain by subverting or affecting the expression of gene(s) involved in one or more of the common neurotransmitter systems (i.e., gamma-aminobutyric acid, glutamate, acetylcholine, dopamine, serotonin, and opioid peptides). However, an early increase in dopamine signaling has been one of the most consistent observations across studies of the reinforcing effects of drugs of abuse. Although studies with various knockout mice have emphasized the role of specific gene products working in the brain (see Mayfield et al., such as Homer 2, opioid receptors, and alpha 4 nicotinic receptors in conferring either protection from or increased risks of addiction). In addition it is apparent that the contribution of any single gene in the development of addition for any drug is only a small part of the picture. Like most familial behavioral phenotypes, drug and alcohol use disorders result from the complex interaction of multiple genes. This complexity may account for ongoing challenges associated with the development of addiction and solutions to deal with them. Needless to say, multiple genes exert their effects in the context of genetic networks, which are typically under the influence of environmental factors. These early effects initiated by the gene product induced by drugs or alcohol most likely cascade through the signaling pathways and generate a domino effect. To understand the complete molecular or gene expression changes that may occur in the brain due to drug and alcohol use it is important to capture those changes as a whole and perform a systematic analysis. One novel and effective approach that has been used in recent years to decipher and unravel this complex mystery is metabolomics.
Metabolomics: The Beginning
The completion of the human genome project has made it possible to investigate the whole genome using high-throughput technologies and analyze data via a systems approach (see Fig. 14.1 ). Derivation of molecular-based strategies, development of new computer application and technologies, and the application of bioinformatics are accelerating the elucidation of molecular underpinnings of human diseases as well as helping to effectively prevent, diagnose, and treat these diseases. These strategies can also be successfully applied in addiction-related disorders.
Since molecular biology’s early years, biological questions have been successfully approached mainly by studying individual gene function(s) and gene products, one or a few at a time. Despite understanding the cause of many biological problems, however, many fundamental biological questions remain to be answered. This is mainly because the majority of gene products function together, interacting with other gene products and influencing multiple pathways. Therefore, biological processes should be considered as complex networks of interconnected components. In addition to studying the components individually, it is important to study the combined nature of these gene products in the metabolomic networks and pathways.
Metabolomics in Addiction Research: Current Approach
Selection of Technology to Capture Metabolomic Changes
Recent advances in the latest technologies allow profiling of all metabolic components in a biological system at any given time, investigating of dynamic changes in component quantity or quality in a system under external stimuli or perturbation, and finally, analyzing the changes of one component in relation to another. The goal here would be to generate protein-protein, protein-DNA, or other component-component mapping of the networking pathways involved. Gene expression microarray is one such high-throughput technology that allows detection of cellular changes at the transcript level and has been used extensively in research on alcohol and other drugs of abuse. Gene expression profiling using microarray chips has proved to be the most successful genome-wide technology to capture the temporal-spatial expression pattern of a cell. Because the expression microarrays are an RNA-based method, they are highly effective in the simultaneous identification and measurement of virtually all transcripts that are differentially expressed between any two samples representing treatment (e.g., ethanol) and control. Transcript profiling using microarrays is the most widespread functional genomics technique because of its relative technical simplicity, low cost, and short turnaround time. In recent years, the development of high-density microarray chips has allowed us to present the entire transcriptome of more complex organisms such as human and mouse on a single chip. The availability of improved algorithms and easy to use software has made it possible to interpret and analyze the microarray data without much computer knowledge. Of all the addictions, such studies have been extensively used in alcoholism.
Over the last 5 years, studies on humans and animal models using microarray have contributed to our knowledge of the molecular effects of alcohol and identified a number of potential candidate genes of interest in the context of alcohol response alone. In addition, microarray experiments generate a large number of ethanol-responsive genes, some of which are repeatedly identified as such in multiple reports regardless of experimental paradigm. However, it would be extremely difficult and time consuming to investigate this large number of genes using a single or candidate gene approach. Because these genes belong to multiple biochemical pathways including stress response, gene regulation, apoptosis, cell growth, and cell signaling, we need to analyze them altogether in the context of cell and tissue system.
Tissue and Organ of Interest
In order to investigate the effect on gene expression following drug or alcohol exposure, cells or tissues from participants and their matched controls are needed. In most cases brain and brain regions are viewed as most appropriate. However, matched samples of brains are not available for such studies. The next best option is to make use of brain bank samples. This too is not always practical. More importantly, matching participant and control brains is not an easy task, as they differ in a large number of parameters that may affect gene expression. We will not discuss this issue any further except to state that in the lack of human brain matched samples, and due to the problem of manipulative experiments on humans, most studies have relied on a number of animal models. Of course this approach has its own drawbacks. With this realization, we focus this discussion on one of the favored animal model (e.g., mouse). This has a number of advantages. Established genetic strains of mice are available and widely used that differ in responses to various drugs, including preference to voluntary alcohol consumption. For example, strain C57BL/6 J consistently demonstrates high ethanol preference, which is about 60% in comparison to strains A/J (26%), BALB/CJ (30.4%), and DBA/2 J (11.8%), given the free choice of water and 10% ethanol over a 14-day period. Once a suitable genetic animal model is selected and the drug preference phenotype is reconfirmed with an in-house study, the animal model can be used in necessary experimental treatment with the drug under study following appropriate methods. This allows collection of the desired organ following appropriate treatment along with strain/genotype-, age-, and sex-matched controls. The drug treatment may involve various modes and durations as appropriate. It is also possible to include various environmental manipulations including stresses before or after the exposure to the addictive drug being investigated. The tissue of interest (e.g., brain) is collected at the appropriate time and processed for studies on DNA, RNA, and protein including structural and developmental alterations that may be affected in any drug response including addiction. Although such studies in the past have concentrated on RNA and protein including cellular changes, it is important to point out that future studies may include DNA and protein changes, reflecting epigenetic modifications. In fact it is likely that the addiction to a drug that is acquired following repeated exposures may involve epigenetic changes (DNA methylation, histone modification including chromatin alterations), which has remained on the side line of such a research. We will not go into any detail except to point out that such changes will explain a variety of RNA and protein changes that have been reported in response to drugs and drug addictions. For mainstream research in the new genomic era, the tissues of interest are harvested from an experimental animal model and used for RNA isolation. The purified RNA is hybridized to a suitable expression array, which allows identification of genes that are affected as a result of drug exposures.
Generating a Gene List
The microarray is hybridized, scanned, and analyzed following the manufacturer’s recommended method and software. Microarray experiments generate a large number of genes, which are analyzed further using bioinformatics tools and software. It is beneficial to develop a list of genes that show significant difference in expression between treatment, gender, and age, or any other selected parameters. This list can be further refined by primarily excluding genes for which no information is available in the public databases such as the National Center for Biotechnology Information (NCBI; http://www-ncbi-nlm-nih-gov.easyaccess1.lib.cuhk.edu.hk /), Ensembl ( http://www.ensembl.org/index.html ), GO ( http://www.geneontology.org /), UCSC ( http://genome.ucsc.edu /), and so on (see Verstreken et al. ). These genes are used in the subsequent analysis as explained in Fig. 14.2 .

Bioinformatics Tools and Analysis
It is apparent that in most cases a single gene may carry out several functions in the cell by interacting with other genes in the network. In this way, the action of one gene product dynamically affects the action of others in a cascading fashion in the cellular pathways, generating a complex global network. In order to identify how a particular gene interacts in a subsystem, how all the subsystems coordinate into a particular pathway, and how the collective actions of multiple implicated pathways emerge into a global network in the metabolome, causing multifaceted alcohol or drug effect, the genes are analyzed using specialized software to predict biological association network. Currently many biological pathway analysis and visualization software programs are available. Some of the commercial and free software are listed in Table 14.1 .
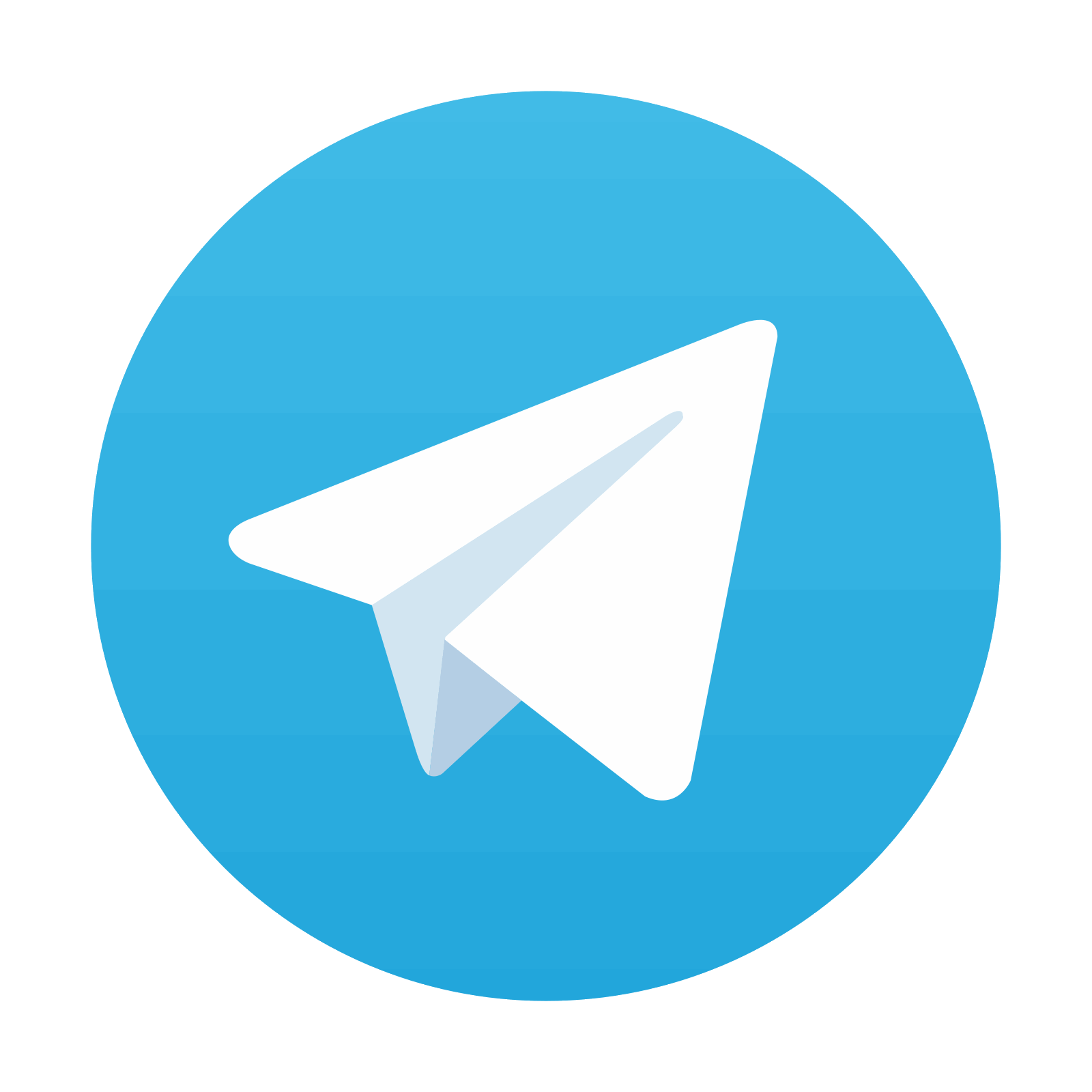
Stay updated, free articles. Join our Telegram channel

Full access? Get Clinical Tree
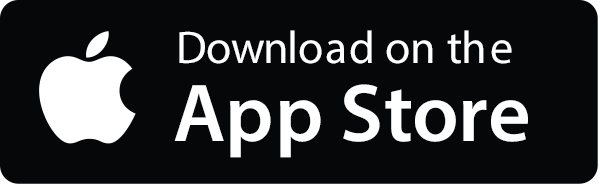

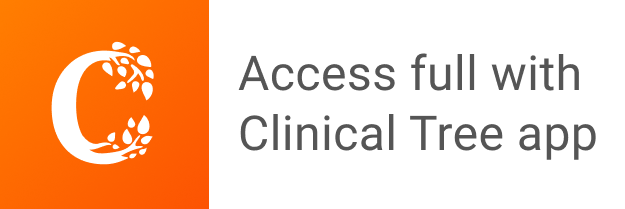