- how we classify different types of variables;
- to recognise and define measures of central tendency, variability and range;
- four measures of disease frequency: prevalence, risk, incidence rate and odds;
- to identify exposure and outcome variables;
- to define and calculate absolute and relative measures of association between an exposure and outcome.
Epidemiology is a quantitative discipline. It involves the collection of data within a study sample and analyses using statistical methods to summarise, examine associations and test specific hypotheses from which it infers generalisable conclusions about aetiology (causes of disease) and health care evaluation in the target population. In order to be able to understand epidemiological research, one must have a basic understanding of the statistical tools that are used for data analysis both in epidemiological and basic science research.
Types of variables
A variable is a quantity that varies; for example, between people, occasions or different parts of the body. A variable can take any one of a specified set of values. Medical data may include the following types of variables.
Numerical variables
There are two types of numerical variables. Continuous variables are measurements made on a continuous scale; for example, height, haemoglobin or systolic blood pressure. Discrete variables are counts, such as the number of children in a family, or the number of asthma attacks in a week.
Categorical variables
There are two basic types of categorical variable, which are variables that take nonnumeric values and refer to categories of data. Firstly, unordered categorical variables are used to class observations into a number of named groups; for example, ethnic group, marital status (single, married, widowed, other), or disease categories. A special case of the unordered categorical variable is one which classes observations into two groups. Such variables are known as dichotomous or binary and generally indicate the presence or absence of a particular characteristic. Presence versus absence of chest pain, smoker versus nonsmoker, and vaccinated versus unvaccinated are examples of dichotomous or binary variables.
Secondly, ordered categorical variables are used to rank observations according to an ordered classification, such as social class, severity of disease (mild, moderate, severe), or stages in the development of a cancer. Often in epidemiological studies a variable may be measured as numerical and then subsequently categorised. For example height may be measured in feet and inches and then categorised as: <5ft, 5ft–5ft 5in, 5ft 5in–6ft, >6ft.
The type of variable will determine how that variable is displayed and what subsequent analyses are carried out. In general, continuous and discrete variables are treated in the same way.
Descriptive statistics for numerical variables
Most medical, biological, social, physical and natural phenomena display variability. Frequency distributions express this variability and are summarised by measures of central tendency (‘location’) and of variability (‘spread’). We will explore these measures using the following hypothetical data on the number of days spent in hospital by 19 patients following admission with a diagnosis of an acute exacerbation of chronic obstructive airways disease.
Measures of central tendency
There are three important measures of central tendency or location.
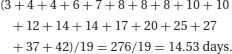
Table 2.1 Formulae for the mean and standard deviation.
Let us assume in the above example that the patient with the longest length of stay actually spent 120 days rather than 42 days in hospital because they could not be sent back home but required placement in a nursing home. This ‘unusual’ observation (outlier) would have a large effect on the mean value (now 18.6 days) whilst having no effect on the median and could make the performance of one hospital look worse than another depending on which summary statistic was being used for the comparison.
Measures of variability
The extent to which the values of a variable in a distribution are spread out a long way or a short way from the centre indicates their variability or spread. There are several useful measures of variability.

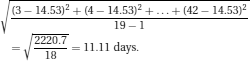
The Normal (or Gaussian) distribution (introduced in Chapter 1) is described entirely by its mean and standard deviation (SD). The mean, median and mode of the distribution are identical and define the location of the curve. The SD determines the shape of the curve, which is tall and narrow for small SDs and short and wide for large ones (see Figure 2.2).
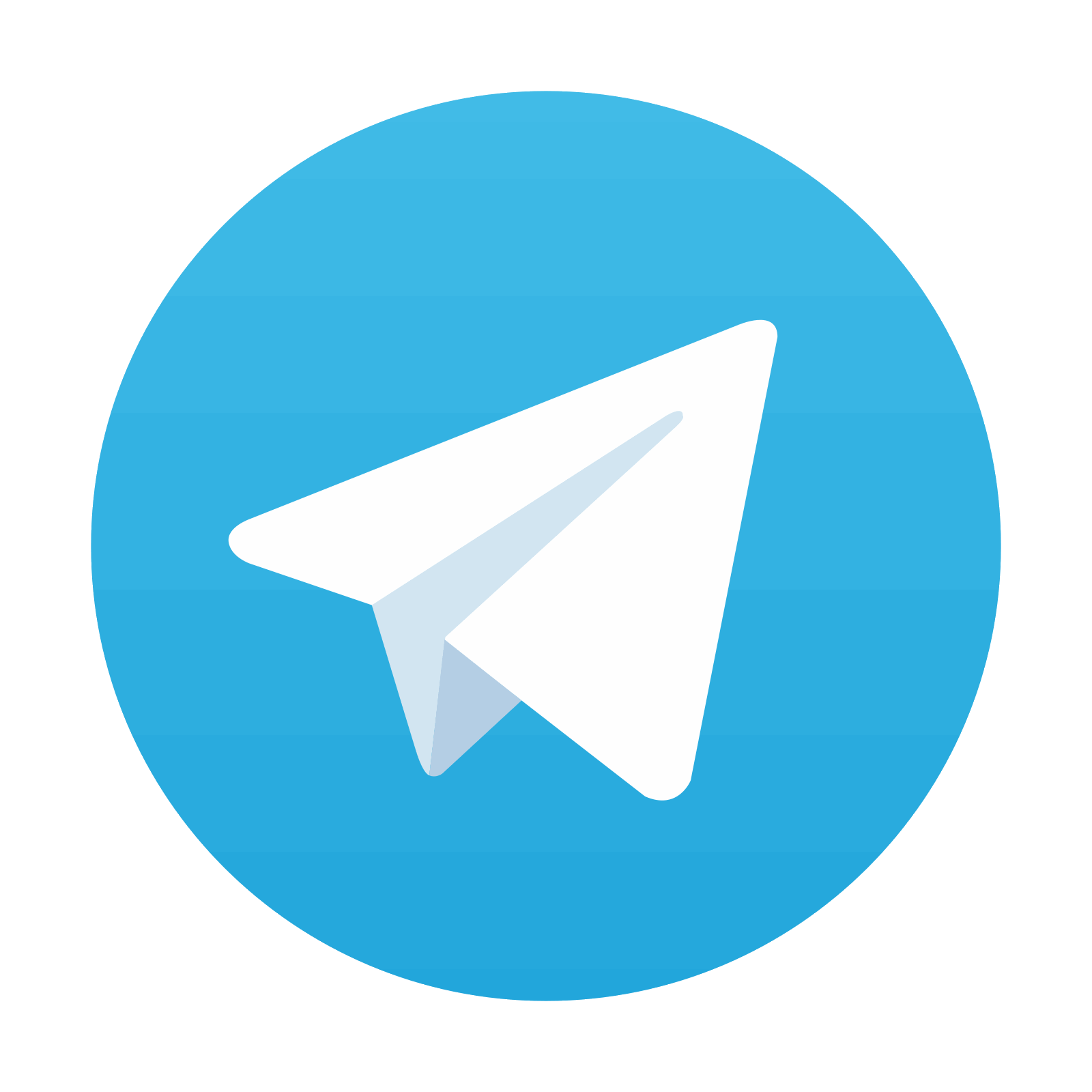
Stay updated, free articles. Join our Telegram channel

Full access? Get Clinical Tree
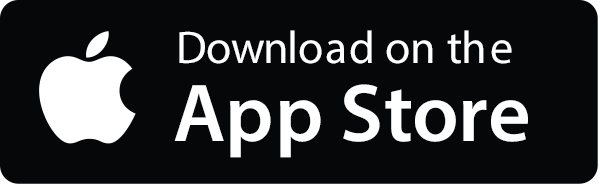
