html xmlns=”http://www.w3.org/1999/xhtml” xmlns:mml=”http://www.w3.org/1998/Math/MathML” xmlns:epub=”http://www.idpf.org/2007/ops”>
In Sections 25.4 and 26.3.1 we described the use of multiple cohorts and multiple control groups, respectively. For cohort studies, cohort characteristics, except for the factor of interest, should be as similar as possible. For case-control studies, the cases and control groups should be as similar as possible, with the exception of the outcome. Even if the inclusion and exclusion criteria (Section 26.2) are the same except for specific items relating to the group being recruited, the groups may not be comparable on key prognostic variables and thus the comparisons may be biased. In this chapter, we describe how to make the groups more similar using matching. Matching means that participants in one or more groups are selected at least partially on their similarity on certain characteristics to participants in another group. Matching may be done either by matching individuals or by matching the distribution of the characteristics in the groups, called “frequency matching.” We focus our discussion on case-control studies, as matching is most commonly used in them and the selection of the control group is a critical decision for the validity of the study. Matching may also be appropriate in a comparative cohort study.
27.1 Why Match?
In interventional studies the investigators rely on randomization to make the study groups equivalent on prognostic factors, eliminating prognostic bias (Section 17.1.1). If the factors are very important, then stratified randomization can be used (Section 21.3.1). In observational studies, when groups are being compared, it is also important to ensure that the groups do not differ on important prognostic factors. Sometimes the investigators can rely on homogeneity within the populations or a very large sample size that should allow the prognostic factors to be distributed equally across all groups so that their effect can be assessed and incorporated in the analysis. However, if the groups studied differ on critical factors in addition to the characteristic used to identify the group (exposure in comparative cohort studies; outcome in case-control studies), then the exposure-outcome relationship may be affected by these other factors. This problem is called confounding (Section 16.2). Matching in an observational study is a way to reduce confounding.
You plan to do a case-control study in elderly individuals with a condition that becomes more common and tends to worsen as people get older. Cases would be recruited from a clinic, but controls would be recruited from the general population. Controls would tend to be younger then the cases, no matter what age range is used as the inclusion criterion, because older people are more likely to have medical and mobility issues, decreasing the chance of them volunteering as controls. Reducing the upper age limit on the cases would reduce the generalizability of the study, but the potential distribution of ages could still be different between the two groups and could still confound differences in the exposure(s) of interest between the two groups. Using matching to have a similar age distribution of cases and controls would reduce this concern.
27.2 Who Are You Matching?
Matching requires one group be identified as the index group and the other group(s) matched to this index group. In a case-control study the index group is the cases, while in the comparative cohort study the index group is the cohort which has the exposure of interest. In both designs, we use the terms “index case” for individuals in the index group and call the groups matched to the index group the “controls.” For simplicity, we assume there is only one control group. In practice there may be multiple control groups, especially in a case-control study, with each control group matched independently to the index group for different characteristics. Within each control group, there may be multiple participants matched to a single index case.
In a case-control study of vaccine side effects the investigators have a large database of members from an HMO. Given the large number of potential controls and small number of cases (individuals with side effects), the investigators designed the study to have up to five individually matched controls for each case. Potential cases and controls were then contacted and invited to participate in the study.
Using multiple controls is a way to gain statistical power (Section B.7) if the number of participants in the index group is relatively small. Usually the number of controls per index case is five or fewer, but larger numbers have been used. It is not necessary for each index case to have the same number of controls, since there are statistical methods to analyze data even with varying numbers of controls.
27.3 When Is Matching Done?
The basic definition of matching assumes that the process occurs during the recruitment phase of the study. The variables used for matching and the matching criteria are defined before the study begins. Participants are recruited into the index group as they become available, but participants in the control groups must match the index group to be included in the study. Frequently both the index group and the controls are recruited from a larger pool of available individuals in a cohort study, rather than specifically for the study.
In a case-control study of a particular birth defect, cases were infants born with this defect in a group of regional hospitals. The controls were infants born in the same hospitals during the same period. The mothers were matched on age, parity, and ethnicity. In addition, the infants had to match on sex and gestational age.
An investigator proposed to do a record review of variables associated with the occurrence of nosocomial infections in the surgical unit of a large hospital. Records of all surgeries are available from the hospital database. All patients who had evidence of post-surgical nosocomial infections will be selected. Once they are identified, up to 5 controls, matched on sex and surgery type, and similar in age and date of surgery will be selected from the remaining individuals in the file.
In some studies matched subgroups are created in the analysis phase of a study, after information on all the participants is available and the database is complete. This method is frequently referred to as post hoc matching. This is frequently done with data from a large cohort study which has information on the matching variables for all the participants. This should be part of the study design and the specific details of the matching procedure defined in the protocol.
The Framingham Heart Study, initiated in 1948, was designed as a longitudinal investigation of constitutional and environmental factors influencing the development of cardiovascular disease in men and women free of these conditions at the outset. The original study enrolled more than 5,000 participants for the first examination and at this writing is enrolling and testing a third generation of participants. In addition to the primary analyses, participants and data from this study were used for case-control, case-cohort, and matched cohort studies of other outcomes. For example, to study the causes of cognitive decline after stroke, investigators identified a group of 74 cases from the study cohort who had suffered a stroke during a 13-year period as cases and a control group of 74 participants, matched on age and sex, who had not had any cardiovascular events. The groups were compared on several variables thought to be associated with cognitive function, including pre-stroke measures.
If post hoc matching is not part of the basic design, it may introduce problems of credibility, since it may seem that the choice of variables and participants included in the control group was driven by the data rather than scientific theory.
Investigators completed a cohort study in children of the effects of an exposure on a specific outcome after 5 years. The results of the study were neither statistically significant nor clinically important. The investigators examined the data further and noticed that if they selected a subgroup that had the outcome and matched its members to specific controls, they would have a statistically significant difference. They were advised that if they published this analysis, it could be greeted with skepticism, since it was data driven. It could be followed up in a subsequent study designed to investigate the hypothesis, however.
27.4 Individual Matching
Individual matching is the most common method used in case-control studies and in comparative cohort studies when the cohorts are drawn from a larger population. The matching process must be the same for all index cases, and all controls must be matched and all index cases must have a match to be included in the analysis. You cannot mix matched and unmatched individuals in either group. If you believe that individual matching will be too difficult with the available population, then you might consider frequency matching (Section 27.5). If you begin a study planning on using individual matching, then determine that it is too difficult to find controls and would like to switch to a frequency matched design, then you should work with a statistician or other knowledgeable person to determine whether switching to frequency matching is a good choice in terms of practicality and validity.
27.4.1 Defining Matching Criteria
To do individual matching, you must first identify the variables on which to match and define the standards for matching on each variable that you have selected. The criteria for each variable can specify an exact match or an interval for the match, such as age plus or minus 5 years (this is sometimes referred to as “list matching”). Usually the width of the matching interval depends on the potential effect of the variable in the population to be studied, so you might allow a wider discrepancy in age when you are studying middle-aged adults than you would if you were studying young children or very old individuals. Sometimes you must specify standards for how values for the variable are defined, much as you did when defining inclusion and exclusion criteria for the study pool. Thus, if you wish to match on ethnicity, you must define your ethnic groups and specify how you will determine if a participant is a member of one of them.
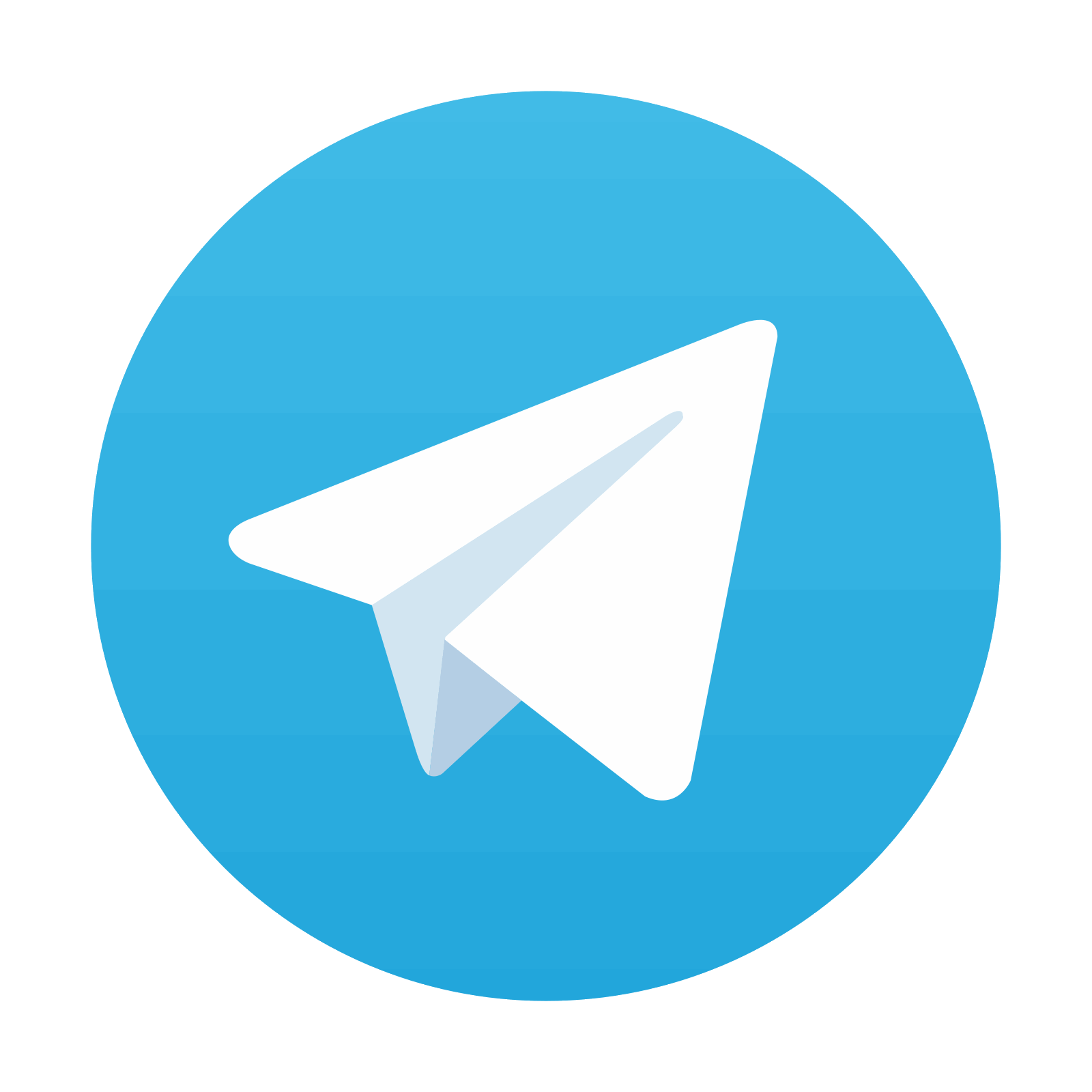
Stay updated, free articles. Join our Telegram channel

Full access? Get Clinical Tree
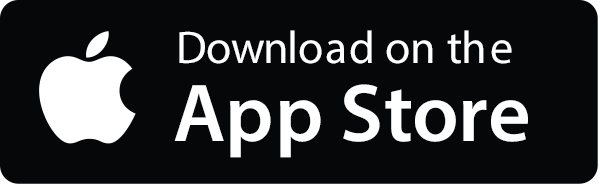

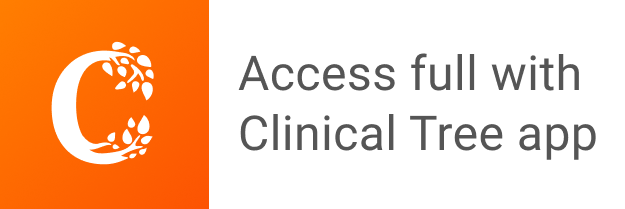