14.1 Ways to prevent confounding
The previous chapter used confidence intervals and significance tests to address random error during data analysis. This chapter uses Mantel–Haenszel methods to address the problem of confounding.
Principles of confounding were introduced in Section 9.2. Recall that confounding derives from inherent differences in risk between the exposed and nonexposed groups that would exist even if the study exposure was absent from both groups. Thus, one way to understand confounding is to consider what might have occurred in the exposed group had the exposure been absent, that is, we ask: “What is the effect of the exposure in the group when it is isolated from all other causes?” This idea is counterfactual (“counter to fact”) since the group cannot simultaneously be exposed and nonexposed. Nevertheless, this type of thinking is helpful in providing clues about causation when experimentation is not possible.
Before presenting Mantel–Haenszel methods, let us consider various ways to prevent or control for confounding. These include:
- randomization
- restriction
- matching
- stratification
- regression modeling.
Randomization applies only to experimental studies. However, when ethical and feasible to do so, randomization prevents confounding by balancing extraneous factors (i.e., factors other than the exposure being investigated) in the groups being compared. Both measured and unmeasured extraneous factors tend to distribute equally in the exposed and nonexposed groups when the study exposure is randomly assigned. Confounding can, however, enter into a randomized study when, by chance, groups do not balance with respect to extraneous risk factors. For example, a small randomized study may, by chance, have a control group that is on average older or sicker than the treatment group. Any difference in results observed at the end of this small trial would then be confounded by age and severity of illness. However, because of the “law of large numbers,” large trials are unlikely to be imbalanced with respect to extraneous risk factors. Increasing the sample size of a randomized trial thus decreases the potential of confounding. In contrast, increasing the size of a nonrandomized study would have no effect on confounding.
Restriction is a technique that imposes uniformity through the use of admissibility criteria in the selection of the people studied. By imposing admissibility criteria, exposed and nonexposed groups are made homogenous with respect to restricted variables. When a study base is homogenous with respect to a potentially confounding factor, this factor can no longer confound results. For example, if a study of daily alcohol consumption and lung cancer was homogeneous with respect to smoking—either all nonsmokers or all smokers—then smoking could no longer confound results. Thus, restriction is a simple and effective way to prevent confounding in both nonexperimental and experimental studies.
Matching can be an effective means to control for confounding when applied judiciously. In cohort studies, matching refers to the selection of unexposed subjects who are identical (or similar) to the exposed subjects with respect to the distribution of one or more would-be confounding variables. In case–control studies, matching refers to the selection of controls who are identical (or similar) to the case series with respect to one or more variables. Matching on variables can be done on a one-to-one basis (individual matching) or can involve matching on factors that define strata (frequency matching). Note, however, that individual matching in case–control studies does not control for confounding unless a proper matched analysis is used.
Stratification is a common way to control for confounding in observational studies. This requires the epidemiologist to classify data into homogeneous subgroups and then use a statistical method to derive an adjusted summary measure of association. This chapter introduces one such method—the Mantel–Haenszel method—just for this purpose.
Regression models are used in epidemiologic studies to evaluate the causal role of one or more exposures while controlling for the confounding effects of other risk factors. Such models are particularly useful when many variables are to be investigated. Care is needed in using regression models, however, because regression models impose assumptions that are not transparent. Thus, even when regression modeling is used, they should be preceded with the type of stratified analysis technique about to be introduced (Vandenbroucke, 1987).
14.2 Simpson’s paradox
Simpson’s paradox (1951) is a strong form of confounding that results in the reversal of the direction of an association (Rothman, 1975).
Suppose a doctor is testing a treatment in two separate clinics. A statistician advises the doctor to allocate the treatment so that 91% of the patients in clinic 1 are randomly assigned the new treatment, leaving 9% to the old treatment. In clinic 2, 1% of the patients receive the new treatment, leaving 99% to the old treatment. (Treatments were assigned to provide the appropriate number of patients that could be handled at each location.) Upon completion of the study, the doctor gives the data to the statistician who cross-tabulates the data to form a single 2-by-2 table (Table 14.1A). Based on this analysis, the statistician criticizes the new treatment as a bad one. The doctor is baffled, however, as he perceives the treatment as a good one. The paradox is solved when data are stratified by clinic. Within each clinic, the new treatment is effective, approximately doubling recovery rates at each of the sites (Table 14.1B).
Table 14.1 Data for Illustrative Examples 14.1–14.3, “Simpson’s paradox.”.

How does one explain these paradoxical effects? “As with any paradox, there is nothing paradoxical once we see what has happened” (Blyth, 1972). Patients in clinic 1 were simply much less likely to recover than patients in clinic 2, and the new treatment was given mostly to clinic 1 patients. Therefore, the poor results of the treatment overall merely reflected its propensity for use in the clinic with more severe illness. This bias acted to make the new treatment appear worse than it actually was. If the tendency to use the new treatment was reversed so that patients in clinic 2 were preferentially exposed to it, the bias would have acted in the opposite direction.
14.3 Mantel–Haenszel methods for risk ratios
Mixing of effects
Confounding comes from the mixing of the effects of the confounding variable with the effects of the exposure variable. In the above example, the clinic was a surrogate variable for the seriousness of the illness being treated. Confounding came about because the comparison of the treatment was also a comparison of outcomes in seriously ill patients and less-ill patients. By stratifying the results into the separate clinics, the subgroups that were formed were relatively homogenous with respect to the confounding variable. In addition, the treatment was randomized within each clinic and the samples were large, suggesting that the results within clinics were not likely to be confounded.
The analysis of our hypothetical treatment could conceivably end here, with data reported separately for each clinic. However, it is often advantageous to summarize the relation being studied with a single, unconfounded measure of association. This can be accomplished by pooling the unconfounded measures of association within clinics to form a single summary measure of association.
Homogeneity assumption
A single unconfounded measure of association can be calculated by pooling measures of association calculated within homogenous strata. Various methods of pooling exist. In this chapter, we cover a flexible set of such techniques called Mantel–Haenszel methods. To apply Mantel–Haenszel methods judiciously, we must assume that the measures of association within strata are homogeneous. This homogeneity assumption allows us to pool strata-specific measures of association to form a single summary measure that has been adjusted for confounding.
Let us return to the data in Table 14.1B. In clinic 1, the incidence proportion difference = 10% – 5% = 5%. In clinic 2, the incidence proportion difference
= 95% – 50% = 45%. Thus, incidence proportion differences are not homogeneous across strata and pooling of the strata-specific incidence proportion differences should be avoided.
Let us now consider the potential to pool the strata-specific incidence proportion ratios for data in Table 14.1B. In clinic 1, the incidence proportion ratio = 2.0. In clinic 2, the incidence proportion ratio
= 1.9. These estimates are “close enough” to be described with a single incidence proportion ratio. It is therefore reasonable to pool these strata-specific estimates. One would predict that the summary incidence proportion ratio will fall somewhere between 1.9 and 2.0.
In considering the homogeneity condition, strata-specific measures of association need not be identical in order to be pooled. The pooling procedure allows for some statistical variation in measures of association among strata, and should be thought of as an averaging mechanism of strata-specific measures of association. Like all averages, pooling measures of association will fail to capture the variability of its component parts. However, when is it appropriate to suppress this non-uniformity, the pooled measure of association provides a statistical convenience whose purpose is to draw correct conclusions about the effect of the exposure.
Mantel–Haenszel summary risk ratio
The principle behind the Mantel–Haenszel technique is straightforward. Since the measures of association within homogeneous strata are unconfounded, we combine them in the form of an unconfounded summary measure of association. In Illustrative Example 14.3, it is reasonable to say that the unconfounded incidence proportion ratio is about 2, since patients at both clinics were about twice as likely to recover when given the new treatment compared with the old. The Mantel–Haenszel method merely provides a way to calculate a weighted average of strata-specific risk ratios (Cochran, 1954; Mantel and Haenszel, 1959).
Notation
Measures of association between an exposure and disease for all data combined (without stratification) will be called the crude measures of association and will be denoted without a subscript. For example, the crude incidence proportion ratio is represented with the symbol . For the data in Table 14.1A,
= 0.24. Subscripts will be used to denote measures of association within strata. For example,
will represent the incidence proportion ratio in stratum 1, and
will represent the incidence proportion ratio in stratus 2. For the illustrative data,
= 2.0 and
= 1.9. Additional notational conventions are shown in Table 14.2.
Table 14.2 Labels and notation for stratified 2-by-2 data, stratum ka.

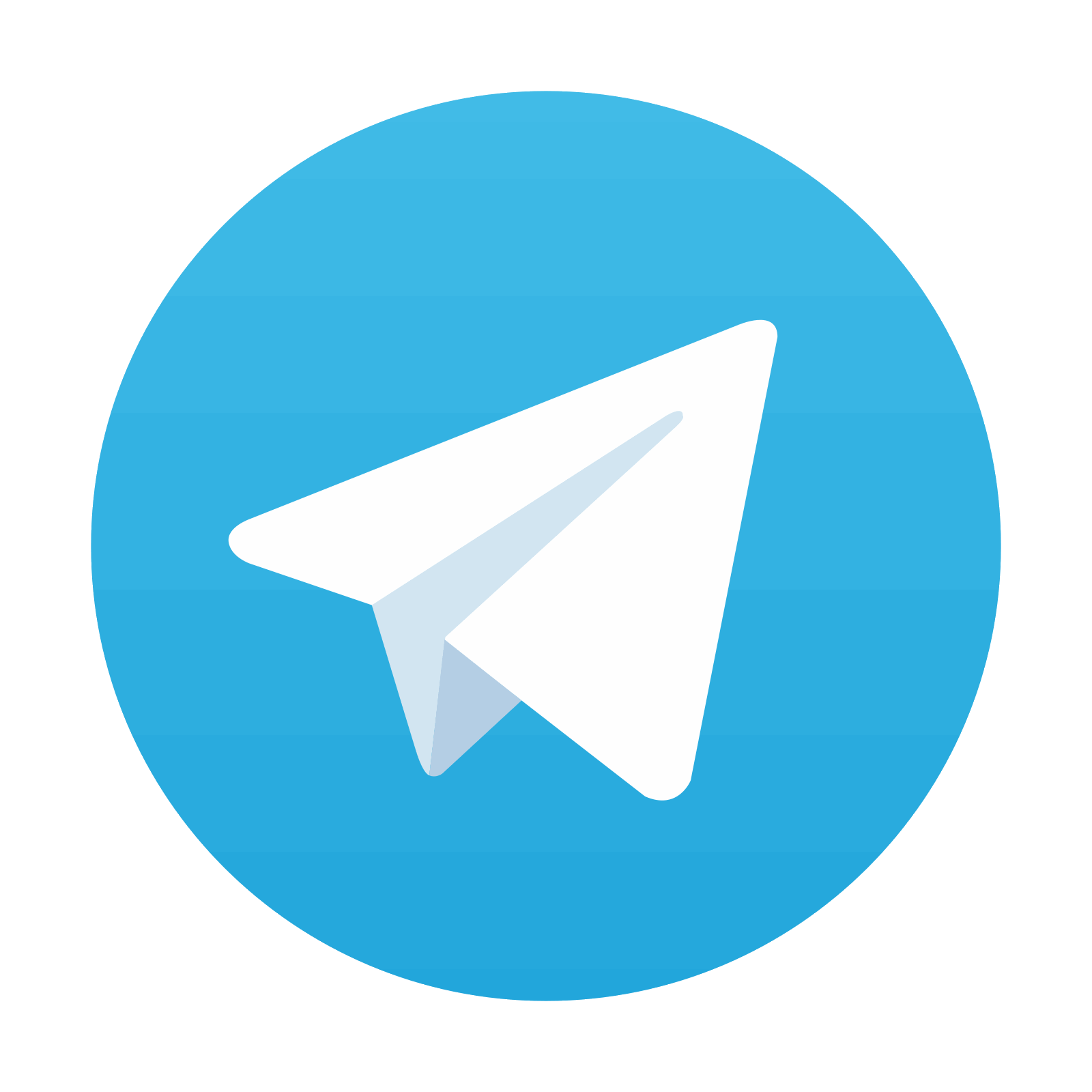
Stay updated, free articles. Join our Telegram channel

Full access? Get Clinical Tree
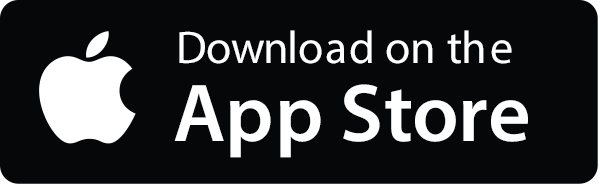
