- that causal factors are probabilistic and may be neither necessary nor sufficient to ensure a specific outcome;
- what are the possible noncausal reasons for observing an association between an exposure and outcome;
- criteria used to guide for considering whether an exposure is causal;
- analytical methods to help determine causality;
- special observational designs and the use of genetic instruments to help in making causal inferences;
- that the population researcher’s and physician’s perspective of a potential causal exposure may differ.
Epidemiology, association and causality
The nature of causation (or causes) is the subject of extensive philosophical discussion. A pragmatic definition of causation is something that, of itself, influences the probability of an outcome. Possible causes are often called exposures. Exposures can be something that increases or decreases the incidence of disease but can also include interventions (drugs or lifestyle) that can alter the prognosis once disease has been established. Some causes are necessary, i.e. exposure to them is essential if a given outcome is to happen; causes may also be sufficient, i.e. exposure to them alone is enough to ensure that the relevant outcome happens. Most important causes in epidemiology are, however, neither necessary nor sufficient – for example around 90% of lung cancer in the population is attributable to exposure to tobacco smoke but exposure to tobacco smoke is not necessary, nor is it sufficient to cause lung cancer. We, therefore, often are dealing with a probabilistic definition of causes identified by studying groups of many people. Examples of the kinds of causal questions that we would like to answer in epidemiology are:
When we observe an association between an exposure (A) and an outcome (B), before assuming that this reflects causation we always consider four possible noncausal explanations.
Observational studies generally provide clues about causality. The ultimate test of a cause is experiment – if manipulation of a putative causal exposure under controlled experimental conditions leads to a predicted change in an outcome then this is the most convincing evidence of causality as one can control for bias and both known and unknown confounders (see Chapter 11).
Conditions for causality
In 1965 Austin Bradford Hill detailed ‘viewpoints’ or ‘conditions for causality’ (Phillips and Goodman,) for assessing evidence of causation in observational studies. These are listed in Box 7.1. Whilst these are often described as causal criteria (with the implication that they should be used as a checklist to rule causality in or out) it should be noted that Hill did not intend them to be used in this way stating in his paper that ‘None of my nine viewpoints can bring indisputable evidence for or against the cause-and-effect hypothesis and none can be required sine qua non’ (Hill, ).
Approaches to examining causality
A number of different analytical approaches have been suggested for testing causality in observational studies, including the use of Mendelian randomisation which will be considered later in the chapter. Two other analytical approaches are an examination of sensitivity and specificity of associations.
Sensitivity analyses
Once an association has been examined using conventional multivariable approaches with adjustment for all measured potential confounding factors, a sensitivity analysis can be undertaken to address the question – what magnitude of associations between a potential confounder and the risk factor and outcome of interest would be required to explain or reverse the observed (multivariably adjusted) association? Once this has been established the authors of a paper (and the readers) can consider whether it is likely that such a confounder exists. One problem with this approach is that it can fail to take account of the possibility that there may be many unmeasured confounders each with a small effect but jointly producing a very large (due to confounding) association. For example, it has been shown that the combined effects of 15 characteristics that were each independently associated with vitamin C and coronary heart disease, could in combination produce an odds ratio between vitamin C and coronary heart disease of 0.60 that was completely due to these confounding factors, despite the fact that individually each would only produce relatively modest vitamin C-heart disease associations (Lawlor et al., ).
Specificity of association
Bradford Hills suggested that the specificity of an association (that is, that the exposure is not associated with multiple outcomes) was a useful criteria for judging causation. Some exposures are likely to be causally related to more than one health outcome. For example, cigarette smoking causes increased risk of lung cancer and cardiovascular disease. However, in large cohort studies where one can look at associations with many outcomes, this is a potentially very powerful and cheap method for exploring causality. This approach involves thinking of an outcome that you would absolutely not expect to be associated with the outcome of interest other than through confounding and then comparing the association of the exposure you are interested in with this new outcome to the main association you think might be causal. This is an underused method in epidemiology. One of the best examples of this approach was a study that tested the hypothesis that hormone replacement therapy (HRT) causally reduced risk of coronary heart disease in women (long before any randomised controlled trials (RCT) had been done). The researchers argued that if the hormone replacement-cardiovascular disease association was not explained by socioeconomic confounding then one would not expect an association between hormone replacement and deaths due to accidents. Accidental deaths (as are cardiovascular deaths) are more common in individuals from poorer socioeconomic backgrounds but there is no biological reason to consider that HRT protects one from accidents. Instead they found that women who reported using HRT were half as likely to die of cardiovascular disease and accidental deaths (both odds ratios 0.5). They concluded that socioeconomic confounding was a strong candidate for explaining the association with cardiovascular disease (Petitti et al., ). This notion was supported by a subsequent large RCT that failed to find any cardiovascular benefit.
Alternative observational methods for determining causality
It is generally accepted that an RCT provides the strongest evidence for a causal association (see Chapter 11). However, there are a number of important causal epidemiological research questions for which it is not feasible or ethical to conduct an RCT. There are a variety of alternative observational approaches that can be used (see Chapter 17 for assessing public health interventions) that go some way to consider residual confounding. We will describe four different methods: parental–offspring comparisons; within and between sibling (including twin) comparisons; cross-cohort comparisons; Mendelian randomisation.
Parental–offspring comparisons
Do maternal exposures during pregnancy have a direct biological effect on offspring outcomes, through influencing the intrauterine environment in which the foetal development of the offspring occurs? Comparing maternal–offspring to paternal–offspring associations is useful for examining whether maternal pregnancy exposures acting via intrauterine mechanisms are causally related to offspring outcomes. If there were a direct biological effect of intrauterine exposure on offspring health status, then the link with offspring health should be much stronger for exposure among mothers than for exposure among fathers. For example, maternal smoking during pregnancy is associated with lower offspring birth-weight, whereas smoking by the father during pregnancy is only weakly associated (and entirely abolished after adjustment for maternal smoking).
Within and between sibling (including twin) comparisons
Examining associations within sibling-pairs is a useful method for controlling for any potential confounding factors that are identical or very similar in siblings (such as socioeconomic position), whether these confounding factors are actually measured in the study or not. For example, in a large record linkage study of 386,485 singleton-born Swedish men from 331,089 families, the association between birthweight and gestational age with blood pressure at age 18 was examined (Lawlor et al.,). Lower birth-weight and earlier gestational age were associated with higher systolic blood pressure (measured at age 17–19) both within siblings and between nonsiblings. The similarity in associations within siblings and between unrelated people suggested that this association in general cohorts/populations was equally unlikely to be explained by confounding background socioeconomic position. Twin studies also can control for potential confounding by genetic factors (by comparing within twin pair associations between monozygotic (identical for genetic variation in nucleic DNA) and dizygotic twins) (see Chapter 6). For example, the Swedish Twin Registry (Bergvall et al.,) found that in monozygotic (genetically identical) pairs, a 500g lower birth-weight was associated with an odds ratio of hypertension of 1.74 (95% CI 1.13, 2.70), and within dizygotic pairs the equivalent odds ratio was 1.34 (95% CI 1.07, 1.69). The similarity of associations within both types of twins provides evidence that the inverse association of birth-weight with hypertension is independent of genetic and environmental factors shared by twins including maternal and fetal genotype (except foetal mitochondrial DNA), socioeconomic factors and gestation.
Cross-cohort comparisons
This approach is based in part on Bradford-Hill’s viewpoint that a consistent association ‘observed by different persons, in different places, circumstances and time’ increases the likelihood that the association is causal. For example, a large number of cohort studies have suggested that individuals who are breast fed (as opposed to formulae fed) have lower BMI, blood pressure and other cardiovascular risk factors and higher IQ in later life, but these associations could be explained by confounding. In a cross-cohort comparison (one from the UK and one from Brazil), the authors found no association between family income and breastfeeding in the Brazilian cohort, but a strong positive association in the UK cohort, with higher rates of breastfeeding observed in the higher income groups (Brion et al.,). Children who were breastfed had higher IQ scores in both studies, with the magnitudes of these associations being similar. By contrast in the UK cohort children who were breastfed had lower BMI and blood pressure even after adjustment for income and other potential confounders, whereas there were no such associations in the Brazilian cohort. These findings suggest that the association of breastfeeding with IQ is causal but those of breastfeeding with BMI and blood pressure are likely to be confounded.
Mendelian randomisation studies
Mendelian randomisation studies have been likened to a ‘natural’ RCT. Mendelian randomisation is the term that has been given to studies that use genetic variants in observational epidemiology to make causal inferences about modifiable (nongenetic) risk factors for disease and health-related outcomes. Such studies exploit the facts that (a) genetic variants are rarely associated with the wide range of socioeconomic and lifestyle characteristics that confound many nongenetic observational association studies and (b) because genes are allocated at conception their associations with later outcomes cannot be explained by reverse causality.
For example, it is well established that heavy alcohol use by pregnant women has adverse effects on health outcomes in their children, including effects on cognitive development, though such exposure and effects (such as foetal alcohol syndrome) are rare. Many women, however, do drink some alcohol during pregnancy, generally at moderate levels. Current public health advice is conflicting with some official bodies suggesting moderate alcohol use during pregnancy is ‘safe’ and others advising abstention. In part this is because of inconsistencies in the evidence base: with some studies suggesting that moderate alcohol use during pregnancy was associated with better cognitive outcomes in children compared to abstention from alcohol, but such an association may be confounded.
The metabolism of alcohol in the body is influenced by several genes. A particular rare variant of the alcohol dehydrogenase gene (which makes it difficult to break down alcohols that are otherwise toxic) is consistently associated with abstinence from alcohol (compared to moderate use). This genetic variant is not associated with mothers’ education or other socioeconomic characteristics and nor is it associated with other lifestyle characteristics such as smoking. This genetic instrument was used to investigate effects of maternal alcohol use on children’s cognitive outcome in a large UK based birth cohort study (Fitz-Simon et al., 2010). In this study, in keeping with results from previous studies, the children of mother’s reporting moderate alcohol use during pregnancy had higher IQ scores and did better in standard school tests than the children of mothers reporting abstinence from alcohol. Moderate alcohol using mothers however were also more affluent, more educated, less likely to smoke, more likely to take exercise and to have a healthy diet, in other words were confounded by a range of possible influences on their children’s cognition.
Adjusting for these factors in statistical models lessened but did not abolish the apparently beneficial effects of alcohol on the cognitive outcomes studied – illustrating the limitations of statistical adjustment and problem of ‘residual confounding’. When the children of women whose genotype would predispose them to abstinence from alcohol were compared to children of women without this genotype a completely opposite picture emerged to that seen using the classical epidemiological approach. Children of women with the ‘abstinence gene’ did better in school tests and had higher IQ scores than women with ‘moderate use’ genes. This is convincing evidence that maternal alcohol use actually exerts a damaging effect on children’s cognitive development, an effect that only became apparent with use of a study design – Mendelian randomisation – able to adequately address the issue of residual confounding. Other examples include studies that have examined the causal role of c-reactive protein, BMI, triglyceride levels and lipoprotein(a) with cardiovascular risk factors and events; the association of fat mass with bone density and of folate acid/homocystein levels with mental health outcomes.
Causality at the level of the population – implications for the doctor-patient relationship
The overall impact of an exposure on disease in the population depends on the size of the risk and the prevalence of the exposure. From a public health/population perspective a rare exposure with a high risk ratio may be less important than a common exposure with a modest or low risk ratio. The population attributable risk (also known as aetiological fraction which is related to the risk difference as explained in Chapter 2) is one measure of the impact of an exposure on disease at the population level.
Epidemiology, however, cannot fully address the question of the cause of an individual’s disease. This applies equally for RCTs as for observational epidemiological studies. For clinicians this has important implications. When informing patients about whether a particular treatment or lifestyle change will be beneficial to them we need to be honest and acknowledge that (currently) there is no scientific means of being sure about this. What can be said is, for example, amongst all adults with high blood pressure (hypertension) there will be a greater proportion who have a stroke in the future than amongst all adults without hypertension and that if all those with hypertension are treated with antihypertensive medication the proportion of strokes in that group will be reduced. We can also attach approximate probabilities to these outcomes given different scenarios based on epidemiological evidence.
Patients are not unfamiliar with notions of probability and chance. For example, in our clinical experience it is actually unusual for patients to invoke the apocryphal ‘Uncle Norman’ who smoked 60 cigarettes a day till he was run over by a bus at the age of 90 as evidence that smoking is harmless. Patients appear to accept, as we regularly remind them, that 50% of lifelong smokers die from a smoking related cause but they also seem to realise that this means 50% of smokers will not die from a smoking related cause and that the difference between the two groups may be substantially a product of chance. Uncle Norman was clearly lucky in relation to the consequences of his smoking, if unlucky with regard to the fatal bus.
Clinicians have often struggled with the question of how best to use epidemiological evidence to inform conversations with patients. For example, in relation to treatment discussions an approach popularised by the Evidence Base Medicine movement involves use of the ‘number needed to treat’ (see Chapter 11) the reciprocal of the absolute risk difference. This metric, within certain assumptions, tells a patient how many people have to take a particular treatment – typically for a year – for one of them to avoid the adverse outcome the treatment is intended to avert. How helpful patients find this information in relation to their own treatment decisions is unclear. In our experience, one of the most frequent questions asked of doctors by patients is still, ‘What would you do if you were me?’
The wider issue here is perhaps the fact that there are many things patients would like us to be certain about that we cannot know and we have to be honest that we cannot know whether they will fall into the group who do not die because of their smoking or not. We also cannot know if they will be the one (amongst the number needed to treat) who will avoid the adverse outcome if they all took a particular treatment. Acknowledging and discussing uncertainty is a fundamental part of both clinical medicine and public health. For more discussion of this idea visit the understanding uncertainly website http://understandinguncertainty.org/.
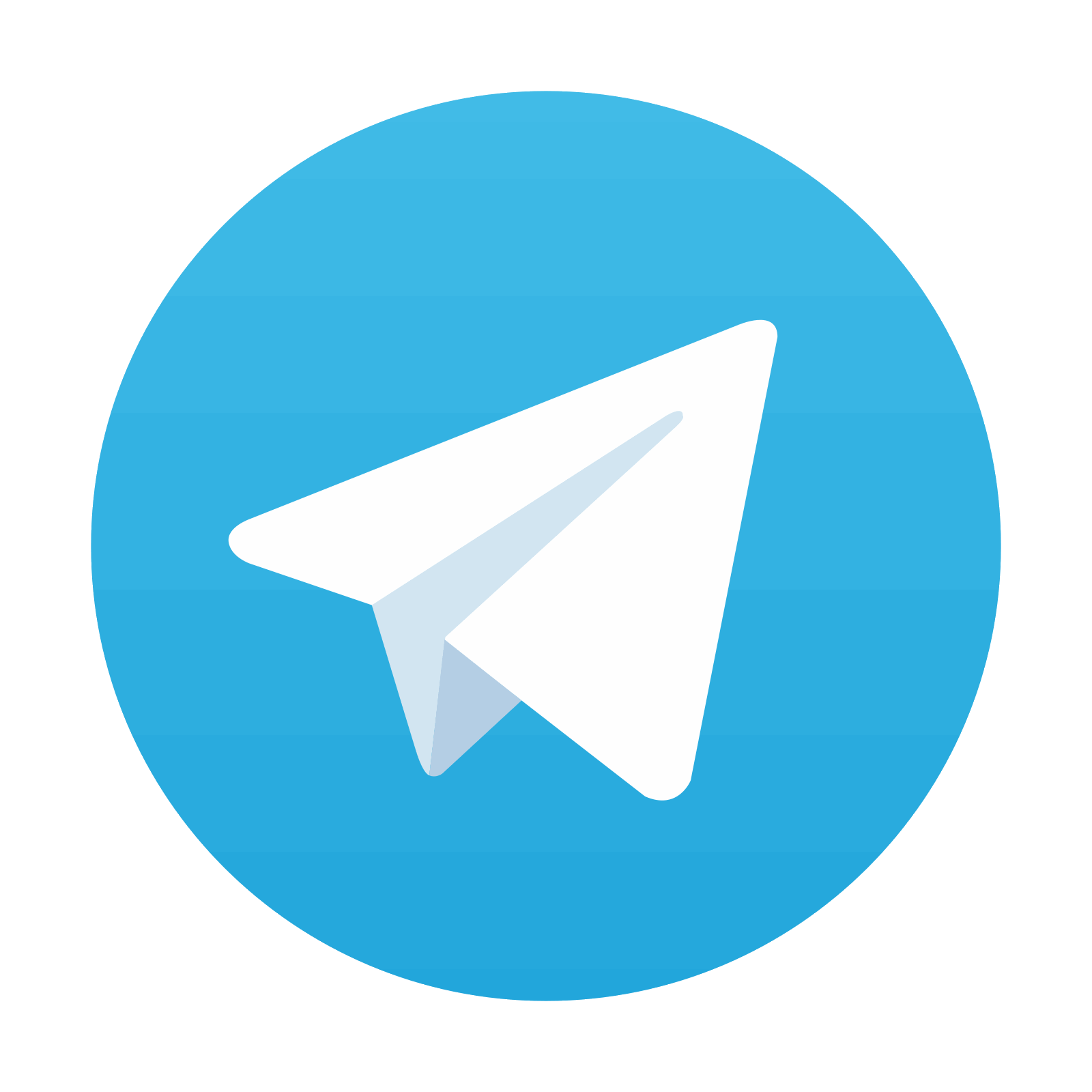
Stay updated, free articles. Join our Telegram channel

Full access? Get Clinical Tree
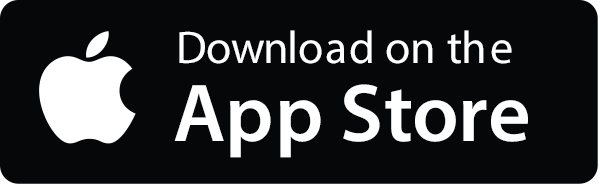
