© Springer Science+Business Media Singapore 2016
Yar Muhammad MughalA Parametric Framework for Modelling of Bioelectrical SignalsSeries in BioEngineering10.1007/978-981-287-969-1_11. Introduction and Motivation
(1)
Faculty of Information Technology, Thomas Johann Seebeck Department of Electronics, Tallinn University of Technology, Tallinn, Estonia
Healthcare is becoming an important challenge around the world. Many developed countries are facing socio-economic problems such as increasing healthcare costs. For example, in 2010, the USA alone spent about US $2.5 trillion (17 % of its GDP) on healthcare, and this number is expected to grow in the future due to emerging new requirements in healthcare. In response to these growing concerns, developed countries such as the USA, EU, and Japan are advancing a new healthcare model called “Personalized Healthcare” to control the healthcare costs while improving the medical quality by use of Information Communication Technology (ICT) (Wu 2011).
These concerns result from phenomena such as (a) increasing average life expectancy and the ageing of baby boomers, as well as (b) the growth of chronic diseases (e.g. cardiovascular diseases) related to, e.g. dietary and lifestyle factors.
Regarding (a), the increased size of the elderly population (65+) is becoming a worldwide demographic phenomenon. It is approximated that in 2050 people aged 65 or more will be found as follows: in Europe ~40 %, in Japan 36 %, in China the figure will increase from 10 % in 2006 to 28 % in 2040, and in the USA it will reach 19.6 % in 2030 (Wu 2011).
Regarding (b), cardiovascular diseases are very common. For example, in the USA cardiovascular diseases caused 788,000 deaths in 2010, which was 32 % of all deaths. The cardiovascular disease-related number of deaths increased considerably from 1900 to 1970 and remains high. Heart disease is the top cause of death; the primary case, coronary heart disease, caused 380,000 deaths (NIH 2010).
In Europe, cardiovascular diseases cause over 4 million deaths every year, including more than 1.9 million deaths in the European Union (EU). Cardiovascular diseases cause 47 % of all deaths in Europe and 40 % in the EU. Cardiovascular disease is the primary cause of death in women in all European countries and is the primary cause of death in men in all but 6 European countries (EHN 2012).
To deal with issues related to (a) and (b), there is an increasing need and requirements for monitoring and analysing human cardiovascular functions, especially for enhancing continuously for non-invasively and non-obtrusively underneath clinical and ambulatory conditions.
In particular, how to extract useful information from cardiac signals for the diagnosis of diseases and judgment of heart function is of special interest. Thus, the development of effective, robust, and efficient diagnostic tools for heart disease symptoms such as cardiac rhythm disorder and arrhythmia is required to help medical personnel to investigate and analyse the cardiac signals in detail (Kersulyte et al. 2009; Gargasas et al. 2004).
The electrocardiogram (ECG) analysis is mostly used in clinic practice, but usually only in a visual way (Gargasas et al. 2004; Kersulyte et al. 2009).
Generally speaking, the aim when developing new techniques and tools is to minimize the required cost, long hospitalizations and increase patient’s ease and safety (Solà et al. 2011). Thus, in this book, non-invasive electrical-based methods are of special interest; such non-invasive electrical signal-based procedures are now very common (further discussed in detail in Sect. 2.4.1 of Chap. 2). The main advantage of this type of procedures is that they do not need to break the skin and are not only used for making a diagnosis but are also usable for treating patients (e.g. electrotherapy, radiotherapy, as discussed in Sect. 2.4 of Chap. 2).
Nevertheless, non-invasive procedures are not without their own practical problems. In what follows, examples of such problems are discussed.
1.1 Problems and Statements of Data Acquisition
Non-invasive data acquisition suffers from various problems; some of them are discussed below.
(a)
The human body is inhomogeneous; this makes it difficult to measure accurate and valuable information from the body, such as when measuring cardiac and respiratory information.
(b)
The body evaluation model is a combination of three different sublevel models, i.e. electrical, mechanical (hydraulic and pneumatic), and geometrical models of the body (Malmivuo and Plonsey 1995).
(c)
It is important to place the measuring sensors according to the body’s model. The optimal positioning of the measuring sensors increases the measurement accuracy and influences the reliability of data, as well as repeatability and accuracy of the evaluated haemodynamic parameters.
(d)
The measured data is a combination of various signals (e.g. cardiac (SCardiac), respiratory (SRespiratory), motion artefacts (SArtefact), noise (SNoise), etc.). As an example, the measured data are useful only if one can separate cardiac and respiratory signals and simultaneously suppress the unwanted artefacts such as motion artefacts, noise, and stochastic disturbance.
(e)
The quality of instruments and sensors should assure the quality of the measurement.
1.1.1 Issues with Extraction of a Useful Signal: The Example of Separation of Cardiac and Respiratory Signals During Non-invasive Procedures
Separating cardiac and respiratory signals are useful to cardiologists (medical personnel) for diagnosing and monitoring purposes. By means of measurements, one can assess physiological activities and the structural configuration of a tissue, as well as offer the possibility to analyse dynamic processes in organs such as the heart and lungs. Thus, a method needs to be developed that is capable of separating the useful signals, mainly cardiac and respiratory ones, and to suppress the unwanted artefacts, such as noise and motion artefacts, from the measured signal. The method should work robustly and efficiently in a real-time environment.
The research community has developed many algorithms to solve the separation problem (Krivoshei et al. 2008; Krivoshei 2006; Krivoshei et al. 2006), including our previous studies (Mughal et al. 2013; Mughal 2014), where algorithms are developed to solve the separation problem of the cardiac and respiratory signals from measured data. However, none of these methods provides any mechanism to evaluate the performance of the developed algorithms.
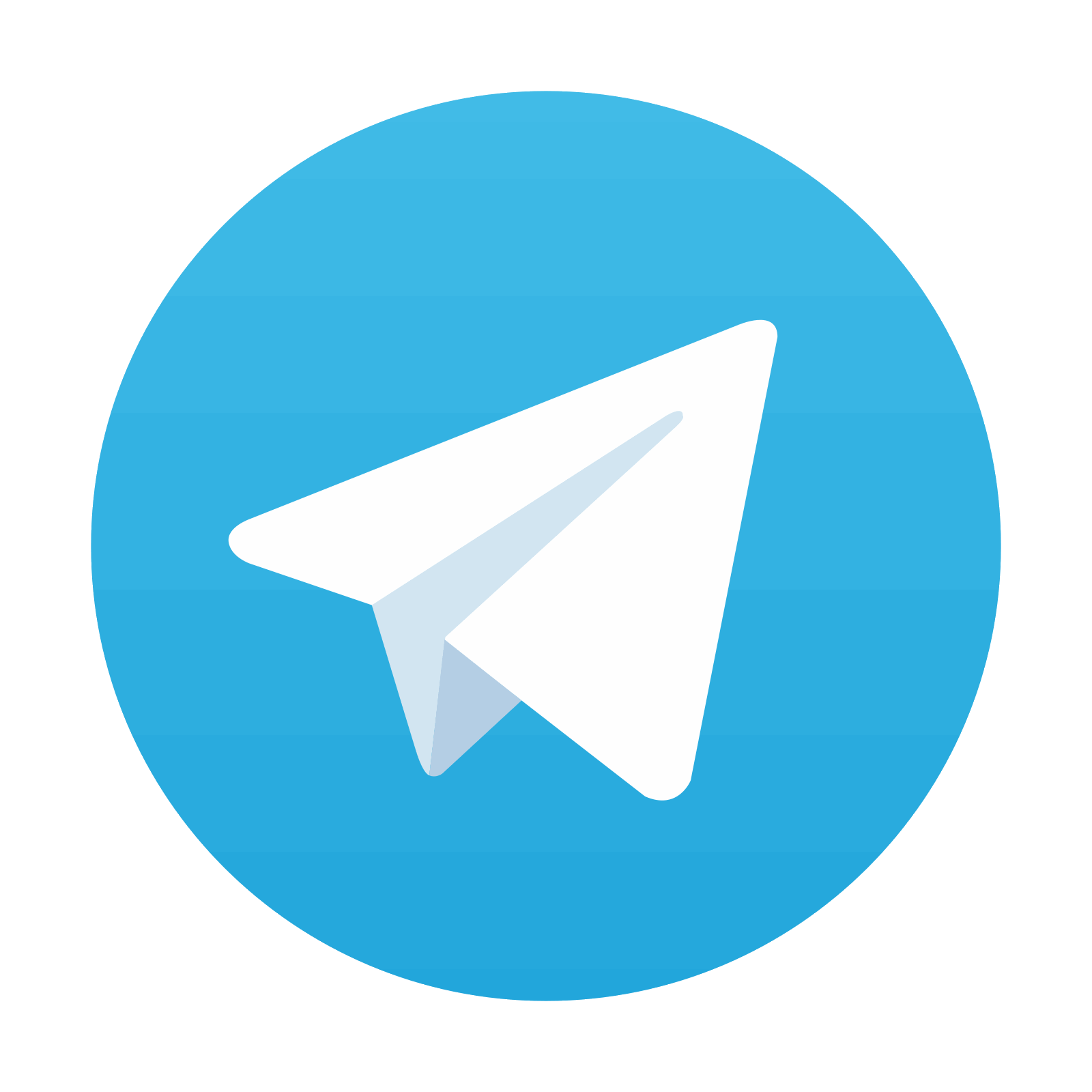
Stay updated, free articles. Join our Telegram channel

Full access? Get Clinical Tree
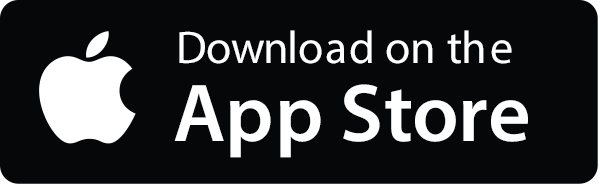
