(1)
where <.> indicates window averaging. The estimated MSC for a given frequency f ranges between 0 (no coupling) and 1 (maximum linear interdependence).
2.3 Phase Locking Value
One of the mostly used phase synchronization measures is the PLV approach. It assumes that two dynamic systems may have their phases synchronized even if their amplitudes are zero correlated [5]. The PLV is defined as the locking of the phases associated to each signal, such as


(2)
In order to estimate the instantaneous phase of our signal, we transform it using the Hilbert transform (HT), whereby the analytical signal H(t) is computed as

where is the HT of x(t), defined as

where PV denotes the Gauchy principal value. The analytical signal phase is defined as


(3)

(4)

(5)
Therefore for the two signals x(t) and y(t) of equal time length with instantaneous phases ϕ X (t), ϕ Y (t), respectively, the PLV bivariate metric is defined given by

where Δt is the sampling period and N is the sample number of each signal. PLV takes values within the [0, 1] interval, where 1 indicates perfect phase synchronization and 0 indicates lack of synchronization.

(6)
2.4 Nonlinear Synchronization (State-Space Approach)
However, a physiological time series such as the EEG appears to have more than the single degree of freedom represented just by plotting the voltage as a function of time. To free up some of these unknown parameters, a standard technique is to map the scalar time series to a vector-valued one in a higher dimensional space
, thereby giving it an extension in space as well as time, forming dynamical evolving trajectories, known as attractors [9]. Hence, one may measure how neighborhoods (i.e., recurrences) in state space located in one attractor maps into the other. This idea turned out to be the most robust and reliable way of assessing the extent of GS [6]. First, we reconstruct delay vectors out of our time series:

where n = 1 … N, and m and τ are the embedding dimension and time lag, respectively. Let r n,j and s n,j , j = 1, …, k denote the time indices of the k nearest neighbors of x n and y n , respectively. For each x n the squared mean Euclidean distance to its k neighbors is defined as



(7)

(8)
And the Y-conditioned squared mean Euclidean distance R n (k)(X|Y) is defined by replacing the nearest neighbors by the equal time partners of the closest neighbors of y n .
If the set of reconstructed vectors (point cloud x n ) has an average squared radius R(X) = (1/N)∑ n = 1 N R n (N − 1)(X), then R n (k)(X|Y) ≈ R n (k)(X) ≪ R(X) if the systems are strongly correlated, while if they are independent. Hence, an interdependence measure is defined as


(9)
Since by construction, it is clear that S ranges between 0 (indicating independence) and 1 (indicating maximum synchronization).
2.5 Network Construction (Graph Topology)
For every subject, run, band, and synchronization measure the interdependence for each channel pair (there are 61(61–1)/2 =1,830 channel pairs since the number of active EEG channels is 61) is calculated. The results were stored to 61 × 61 interdependence matrices (IM) with elements ranging from 0 to 1. In order to obtain a graph from an IM we need to convert it into an N × N adjacency matrix, A. The easiest way of achieving that is to define a threshold variable T, such that 0 ≤ T ≤1. The value A(i, j) is either 1 or 0, indicating the presence or the absence of an edge between nodes i and j, respectively. Namely, A(i, j)=1 if IM(i, j) ≥ T; otherwise, A(i, j) = 0. An adjacency matrix defines a graph. Thus given an IM we may define a graph for each value of T (i.e., if the threshold takes the values T = 0.001, 0.002, …, 0.999, 1 then 1,000 such graphs may be defined; one for every thousandth of T ) [10]. For each edge of a graph we may define its value as W(i, j) = IM(i, j) when IM(i, j) ≥ T.
After constructing A, we visualize the network edges as straight line segments (Fig. 2). Additionally we can visualize the edge values using the heat map color scheme and width of edge segments; for high edge values that correspond to strong interdependence we draw red-shaded thick line, while for low edge values we draw blue-shaded thin line (Fig. 3). Next, we compute various properties of the resulting graph. These include the average degree K, the assortativity coefficient r [11], the clustering coefficient C, the average shortest path length L, and the efficiency E f [14].
Before we describe the above network statistics we should define a graph and a few graph-theoretic concepts. A graph G = (V, E) consists of a set of n nodes V = {v 1, v 2, …, v n } and a set of m edges E, where e ij denotes an edge between nodes v i and v j . The neighborhood N i of a node v i is defined as the set of vertices that have an edge to v i , namely, N i = {v j | (v i ,v j ) ∈ E}.
2.5.1 Average Vertex Degree
The degree k i of a node is the number of vertices in its neighborhood, i.e., |N i |. The average degree of a graph is the average of the degrees of all nodes, as
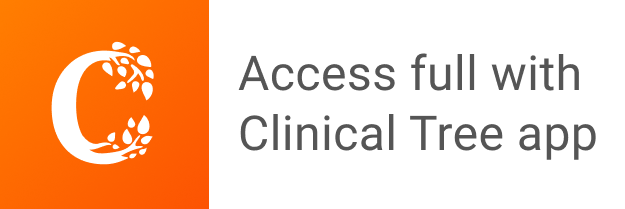