’s are dissolution values of individual units expressed as a % of label claim, are averages of n individual unit dissolution values tested up to that stage,
gives the number of dissolution values of individual units tested up to that stage that fall within range defined by A. Q, L and U are values having units of % label claim that must be justified by the product sponsor.
Table 23.1
Dissolution testing and acceptance criteria
Acceptance criteria | ||||||
---|---|---|---|---|---|---|
IR | DR | |||||
Stage/level | Number tested | Individual samples | Pooled sample | ER, MR, or TD | Acid medium | Buffer medium |
1 | 6 | ![]() | ![]() | ![]() | ![]() | ![]() |
2 | 6 | ![]() | ![]() | ![]() | ![]() | ![]() |
3 | 12 | ![]() | ![]() | ![]() | ![]() |
For IR products, a single dissolution time is also selected (generally less than 60 min). Similarly for DR products, dissolution periods in acid and buffer media respectively are chosen based on considerations given in USP chapter <711>. For ER, MR, or TD products, multiple time points are chosen and the requirements in Table 23.1 must be satisfied for all time points. Early time points are chosen as a check on dose-dumping and later times are chosen to assure complete dissolution/release. A different L and U will generally be justified for each time point. The acceptance criteria must be met for each time point tested.
USP standards are subject to change as technology and quality expectations evolve. Because of its potential relationship to safety and efficacy, dissolution specifications are the subject of debate among stake-holders. The current USP standards do not purport to control population mean (parametric) behavior of a product. Recent reports suggests that dissolution limits should be based on limiting the percentages of slow and fast dissolving units (Tsong et~al. 2004).
An important aspect of USP standards that is often overlooked is that they are not intended as batch release tests. Instead they represent a standard of quality that should be met by any product sample tested at any time during the product shelf life. The practice of employing a USP standard as a batch release test only assures that the standard will be met about 50 % of the time. In practice, many manufacturers use batch release tests more stringent than USP standards to ensure that the USP standard is met with high confidence.
The USP also provides informational guidance on dissolution. Chapter <1088>, In vitro and in vivo evaluation of dosage forms, discusses method development for IR and MR dosage forms, some basic aspects of IVIVC, and establishment of dissolution specification ranges. Chapter <1090>, Assessment of drug product performance—bioavailability, bioequivalence, and dissolution, discusses the relationship of in vitro dissolution to in vivo performance. It gives specific guidance on in vitro dissolution comparisons, statistical methods, and the justification of biowaivers. It provides a good overview of other regulatory guidance from FDA, ICH, and WHO. Finally, USP chapter <1092>, The dissolution procedure: development and validation, discusses factors that impact dissolution testing such as choice of medium, apparatus, equipment operation, sampling, choice of time points, validation and dissolution acceptance criteria.
23.2.2 Setting Specifications
The justification of the values of Q, L, and U as well as choice of dissolution testing times should be based on their relationship (or lack thereof) to in vivo performance. Often the choices for Q, L, and U are based on the observed mean dissolution profile of units from clinical study batches. A further objective is to ensure batch to batch consistency over the product life-cycle. These limits may also be useful in demonstrating equivalency during scale-up or in justification of certain post-approval changes. A suggested starting range for acceptable release is ±10 % LC from the mean dissolution profile. Wider ranges may be accepted if it can be demonstrated or argued that clinical lots with the same variability are bioequivalent. An IVIVC (see below) may sometimes be used to justify wider acceptance ranges.
USP <1092> recommends, with respect to IR products, a Q value in the range of 75–80 % LC. Acceptance criteria, including test times, are usually established on the basis of an evaluation of the dissolution profile and should be consistent with historical batches. There is an expectation that batches consistent with the in vivo performance, composition, manufacturing procedure of clinical batches will have results that generally fall within the acceptance criteria. However, some failure at the first stage of testing may be expected by regulators to assure an adequate consumer risk level. In negotiation of acceptance criteria with regulators, Hofer and Gray (2003) point out that there is a large discriminatory ability difference between stage 1 and subsequent testing stages and argue against rounding Q values to 5 % LC intervals (i.e., 70, 75, 80, 85,…). They suggest choosing a Q value based on computer simulations, or calculated from estimated variance components, that predict the future rate of stage 2 testing (e.g., such as 20 % probability of advancing to stage 2). Their approach should also be extended to ER, MR, and DR products.
23.2.3 Stability
An important aspect of USP standards is that they are expected to be met for any sample taken from any batch of the product at any point during its shelf life. When dosage form characteristics such as enteric coating integrity, moisture content, or other excipient properties drift over time, dissolution can change on product storage. Thus it is critical that dissolution stability be taken into account in the design and justification of dissolution testing procedures and acceptance limits.
When dissolution testing is conducted over multiple dissolution time points (e.g., for MR, DR, TD, or ER products) the stability indicating response should be treated as multivariate due to correlations among results at different dissolution time points. Various statistical procedures such as principle component analysis or a dissolution profile model are available to reduce the dimensionality, but a multivariate stability model may still be required.
23.3 Dissolution Profile Comparisons
23.3.1 Regulatory Guidance
The FDA guidance (1997b) suggests that for certain drugs, in vitro dissolution results might be sufficient to gain regulatory approval for post-marketing changes and waiver of bioequivalence requirements for lower strength dosage forms (Moore and Flanner 1996a, b). A formal similarity evaluation is a regulatory (FDA 1995, 1997a, b, c, 2000a, b, 2003; EMA 2008) for this purpose. Thus the question of demonstrating similarity or equivalence between the reference and test drug dissolution curves is of both scientific and regulatory importance.
Various methods comparing drug dissolution profiles have been proposed. In general, they can be classified into three categories: (1) model-independent approaches based on a similarity factor, (2) model-independent methods using multivariate statistical distance (MSD) test, and (3) model-dependent methods using parametric curves to describe dissolution profiles.
The profile comparison methods recommended in regulatory guidance lacks detail. Perhaps this is intentional since the scope of products regulated is wide and regulatory bodies often expect sponsors to justify approaches scientifically appropriate for a given product. Below are some details that sponsors should take into consideration when justifying a profile comparison method.
Define what is meant by “similar”: Dissolution profiles can differ in many ways. When possible, it is good to identify those aspects of the reference profile that, for safety and/or efficacy reasons, be maintained in the test profile. Depending on the product, dose dumping, incomplete dissolution, slow dissolution, unusual deviations at critical time points, dissolution stability, or unit to unit variability may be most critical. Popular similarity approaches such as f 2 and MSD may not always be the best choices as they are aggregate summary measures and do not consider deviations at specific time points. Availability of an IVIVC may place limits on how much the test profile can deviate from the reference profile.
Similarity can be expressed either empirically or parametrically. Empirical similarity is expressed by placing limits on observed data or statistics calculated from observed data. The f 2 statistic is an example of empirical similarity. When defined empirically, similarity depends on aspects of the study design. For instance, changing the number and location of time points introduces bias into the f 2 statistic.
Parametrical similarity requires specification of a statistical model of the dissolution profile (ideally based on mechanistic understanding) and is expressed by placing limits on underlying model parameters. Parametric similarity is not described in dissolution regulatory guidance, but is a central aspect of bio-equivalence regulatory guidance. When defined parametrically, similarity is not dependent on study design.
Clarification of inference space: The inference space is the population to which the inference (i.e. decision about profile equivalence) applies. Depending on the data available and the statistical model used for the comparison, the inference space can be a) a pair of batches (i.e., 1 test batch and 1 reference batch), b) 2 groups of batches (i.e., multiple batches each of test and reference), or c) to the populations (test and reference) of batches from which tested batches are drawn. Ideally the last of these is the only one that provides any assurance about the behavior of future batches made by the new process. Clarifying the inference space will help determine some aspects of the study design—for instance how many batches of each type should be tested.
Clarification of desired confidence level and power for inference: For clinical studies, it is generally expected that a study plan will include a specification for type I error (=1 − confidence level) and type II error (=1 − power). Similarly it is good practice to prospectively state the operating characteristics of a dissolution similarity comparison study. By specifying the confidence level and power in advance, it is possible to determine the required number of units that must be tested.
Clarify whether a difference or equivalence test is appropriate: A difference test would provide a statistical test of the null hypothesis of similarity. In other words, similarity would be concluded unless contradicted by the data. A difference test would be appropriate in cases where the risk that a change in the process produced a change in dissolution profile was a priori low. The burden of proof is on demonstrating a lack of similarity.
An equivalence test on the other hand is a test of the null hypothesis of non-similarity. This reverses the usual roles of type I and type II errors. The burden of proof is on showing acceptable degree of similarity. In an equivalence test, a “region” of similarity is defined (ideally parametrically). If the estimated similarity metric is well within the similarity region, similarity is concluded. An equivalence test would be called for if there is a substantial risk a priori that a change to a process would produce a change to the dissolution profile. Equivalence tests may require larger sample sizes.
23.3.2 Reasons for Comparisons
There are a number of situations in which a comparison of in vitro dissolution profiles may be required.
Development of an IVIVC (see below).
Development and/or validation of a dissolution test procedure.
Demonstration of equivalency during manufacturing process scale-up.
Demonstration of equivalency after manufacturing process, site, or excipient changes.
23.3.3 In Vitro–In Vivo (IVIVC) Correlations
In vitro drug release profiles are known to be affected by formulation factors such as API particle size, excipients, presence of surfactants or polymers. Besides these formulation factors, the in vivo absorption is also complicated by interactions with physiologic parameters such as pH, transit times, presence or absence of food, and gastrointestinal physiology (Kesisoglou and Wu 2008). Characterizing the link between in vitro release and clinical outcome has been a challenging task for immediate release formulations and remains an area of intensive scientific interest. Recent papers have reported the use of dissolution media simulating physiologic conditions as an improved way to develop an IVIVC exploiting mechanistic models (Jamei et~al. 2009; Sugano 2009; Sjögren et~al. 2013) relating physical and chemical properties of the API with oral absorption and dose.
23.3.3.1 Bio-Pharmaceutical Classification System (BCS)
The BCS system (Amidon et~al. 1995) categorizes drug substances into four groups depending on aqueous solubility and intestinal permeability as shown in Table 23.2.
Table 23.2
BCS classification system
Aqueous solubility | |||
---|---|---|---|
High | Low | ||
Intestinal permeability | High | I | II |
Low | III | IV |
The BCS system also takes into account the in vitro dissolution characteristics of a drug product. Together, the aqueous solubility, intestinal permeability and in vitro dissolution rate are often considered key processes that govern the rate and extent of oral absorption for an IR solid oral dosage form. When in vitro dissolution is conducted under conditions that mimic those in the gastro-intestinal tract, it may be supposed that the observed dissolution behavior reflects that which occurs in vivo. If this observed in vitro dissolution is rapid relative to other pharmacokinetic processes such as membrane absorption, transport to the site of action, metabolism, and elimination, moderate differences in dissolution rate between different dosage forms may not impact bioavailability characteristics, and thus efficacy and safety, of the product. In fact, it may be difficult or impossible to demonstrate an IVIVC for drug substances in IR dosage forms classified as BCS I or III. For drug substances classified as BCS I, depending on stability in the gastro-intestinal tract, effect of excipients, therapeutic index, site of absorption, a biowaiver for bioavailability and bioequivalence studies, and wider dissolution testing limits may be justified.
23.3.4 Dissolution Profile Models
Much interest has focused on models describing in vitro release profiles over a time scale. A good review of mechanistic profile models is given by Costa and Lobo (2001). These and some empirical release models are considered by Costa et~al. (2003). Some of these models are given in Table 23.3.
Table 23.3
Some dissolution profile models
Model | Interpretation | Expression for fraction dissolved |
---|---|---|
Higuchi | Fickian diffusion | ![]() |
Korsmeyer-Peppas | Diffusion based | ![]() |
Quadratic | Empirical, parabolic curve | ![]() |
Hixson-Crowell | Erosion release | ![]() |
Exponential | First order kinetics | ![]() |
Probit | Normal cumulative distribution function | ![]() |
Logistic | Empirical sigmoid shape | ![]() |
Weibull | Life-time distribution function | ![]() |
Gompertz | Growth/distribution function curve | ![]() |
The models do not consider a lag time or background. They assume that the drug release at t = 0 is zero and that drug release commences immediately. However, many of the models in the table above can be modified to accommodate lag times and/or background levels of dissolved drug. Additional parameters such as mechanistic constants can be accommodated in some models. The maximum dissolution can also be accommodated in the exponential, probit, logistic, Weibull, and Gompertz models. Of the models presented in the table, the Weibull model, which is has a mechanistic interpretation, often provides the most satisfactory fits to dissolution data.
Each parameter in these models can be treated as fixed and estimated using nonlinear least squares methods. In some cases the models can be linearized by various transformations and the parameters estimated by linear least squares. Random variation can also be incorporated into the models either as measurement variance or as variance associated with one or more of the model parameters (i.e. as a nonlinear mixed model).
Hierarchical models (see Gelman and Hill 2007) can be considered which account for random variation at various levels, such as batch to batch, run to run, or vessel to vessel. The appropriate model can be identified using available data by the usual modeling approaches (e.g., minimum AIC). However, model selection should not be based on statistics, but should consider known underlying mechanistic processes.
23.3.5 Challenges in Defining Similarity
In order to make a judgment about the similarity of two dissolution profiles, a clear, prospective definition of similarity must be established. It is convenient to view similarity geometrically as a region in some space. If the projection of two profiles onto this space falls within a defined region, similarity can be concluded. Similarity regions can either be defined within the parameter (population) space or the observed data space. This concept is illustrated in Fig. 23.1.
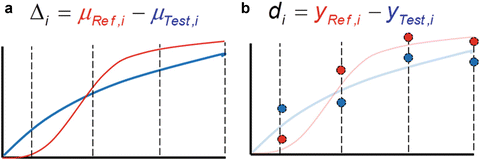
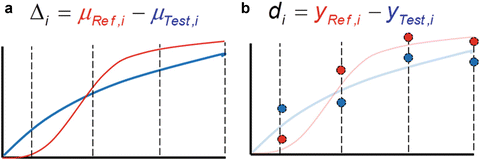
Fig. 23.1
(a) Parametric similarity region. (b) Data space similarity region
Consider a comparison of a Test and Reference profile. The true (population) profiles are shown in Fig. 23.1a in blue and red, respectively. These true profiles are unknown, not based on observed data, and are therefore conceptual. A region of similarity might be defined in the p-space of the true (population) differences (
’s where i = 1, 2,…,p and p = number of time points) between the profiles. Restrictions may be placed on the magnitudes of the
, or some function of them, based on bioavailability and bioequivalence considerations, to define a similarity region. The resulting region of similarity is thus defined in the space of population parameters. This population based similarity definition is useful because a decision rule for similarity can then be based on statistical equivalence testing of the true population parameters (in this case the
) once observed data are available. This is the approach that was taken in defining bioequivalence (FDA 2001). This was also the approach also advocated by Eaton et~al. (2003) for similarity comparisons. Statistical modeling presumes some understanding of the underlying dissolution process and thus represents a basis for risk based decision making and continuous knowledge building. It is important to recognize that safety and efficacy risk is associated with an underlying true population state and that similarity must be defined parametrically. Parametric definitions often permit us to leverage known theory and understanding of underlying mechanisms within a statistical model.
< div class='tao-gold-member'>



Only gold members can continue reading. Log In or Register a > to continue
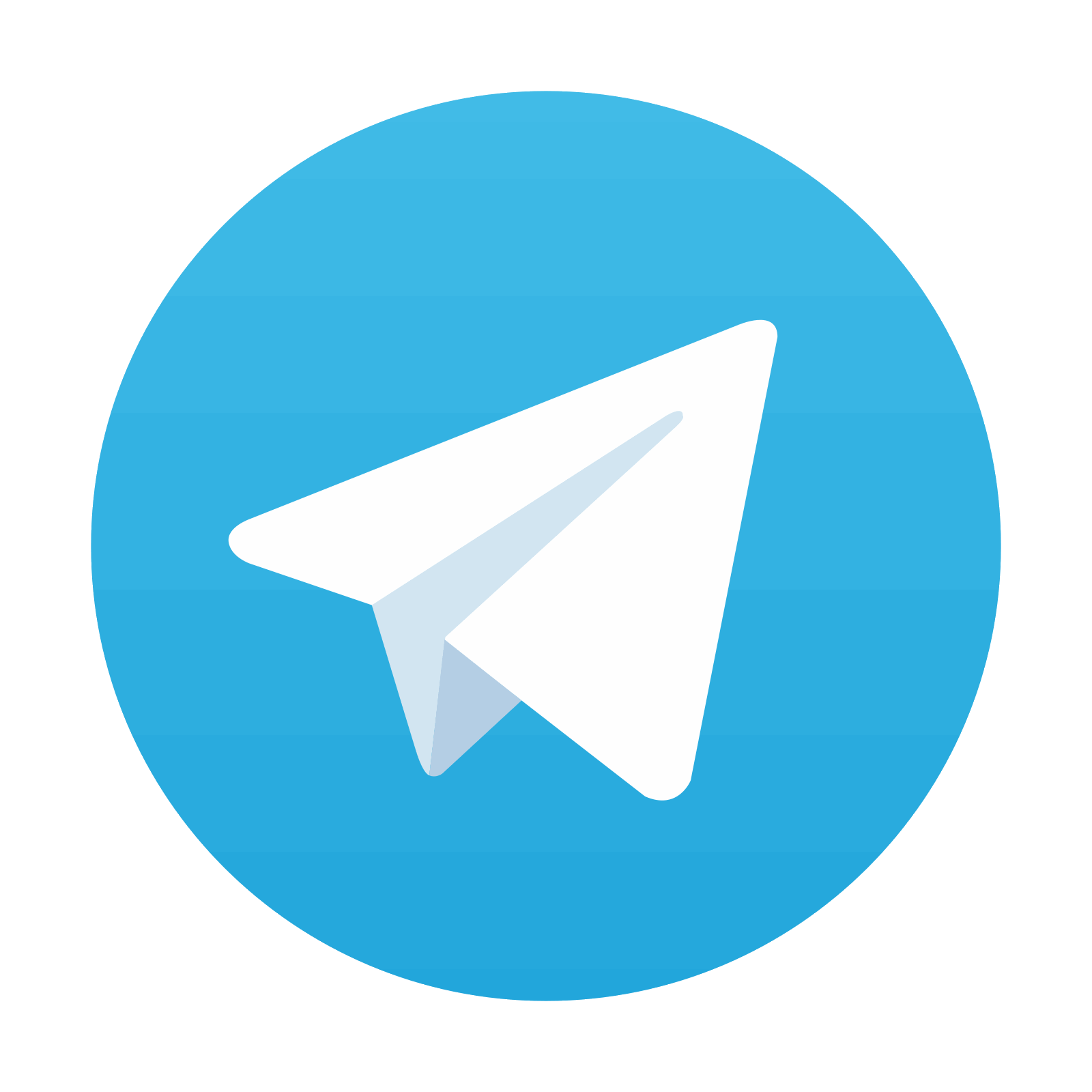
Stay updated, free articles. Join our Telegram channel

Full access? Get Clinical Tree
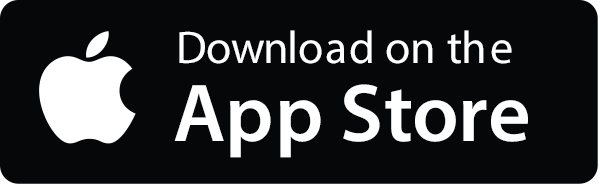
