CHAPTER 10 Hypothesis Testing
1. Is there a significant difference in the proportion of a variable in one of the groups?
2. Is the treatment or intervention more beneficial than another pathway, such as the current standard of care?
4. Does one variable have an effect on another variable (such as the outcome)? What is the strength of effect?
These are very representative of the types of questions posed in research studies. The questions look at different problems but the overall approach to getting an answer is the same. The type of statistical test that is used is different, as is shown by the flow diagrams in Appendices A and B. The specific test depends on the type of variable and the question being asked. However, the logic behind the tests uses the same standard approach. We will look at each of these situations in more detail by focusing on the type of statistical test. Before we delve into the individual situations, however, we first need to understand the format that is used when performing statistical tests.
• State the research hypothesis. This is a pertinent question that can be answered.
• Define the population and variables to be studied.
• Identify outcome variable, if applicable, and statistical test to be used.
• Estimate the number of subjects needed to get a reliable answer.
• Obtain appropriate committee approval and financing.
• Obtain the test statistic using the statistical method based on type of variables and type of question being asked.
• Apply the test statistic to a sampling distribution.
• Make a decision to accept or reject the null hypothesis.
THE NULL HYPOTHESIS AND ALTERNATIVE HYPOTHESIS
All statistical tests start out with the premise that the data are a result of chance variation. This hypothesis is called the null hypothesis, or H0. This is different from the research hypothesis, which is the question that the experiment was designed to answer. The null hypothesis is the first step in the actual process that is used in the statistical analysis.
• The null hypothesis, symbolized as H0, states that there is no difference or no relationship among groups.
It is customary to state an alternative hypothesis, which would be accepted if the null hypothesis were shown to be highly unlikely. In this case, we would reject the null hypothesis of no difference (or relationship) and adopt the alternative hypothesis that a treatment difference (or relationship among variables) does indeed exist. The typical symbol for the alternative hypothesis is Ha. Occasionally the symbol H1 will be used. The specific alternative hypothesis depends on the question being asked. We will see examples of this in the following chapters.
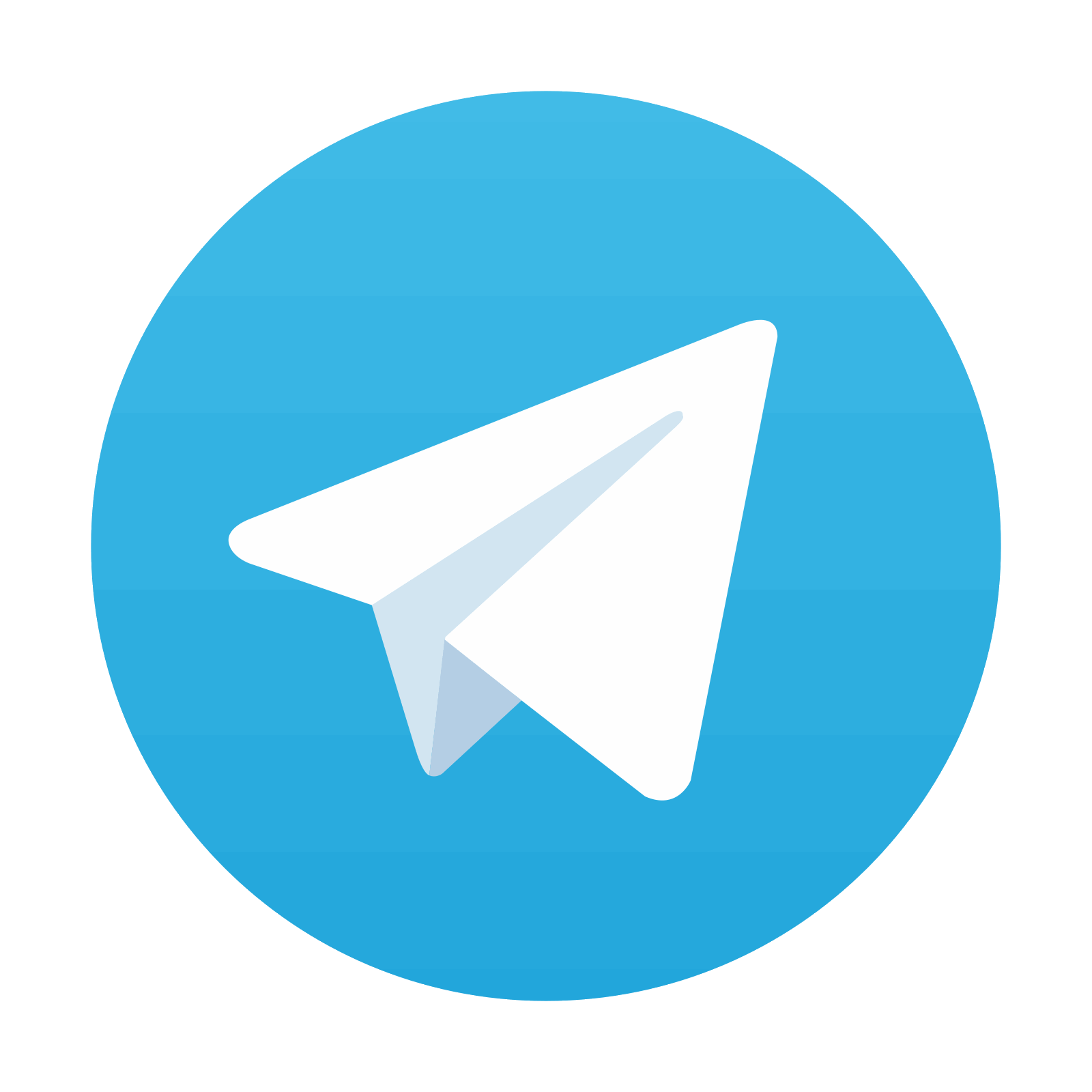
Stay updated, free articles. Join our Telegram channel

Full access? Get Clinical Tree
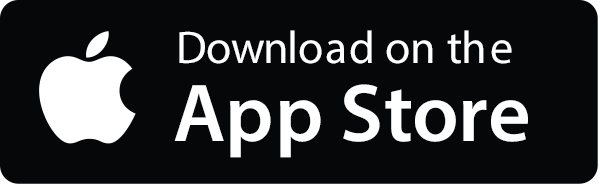
