Drug name
Drug indication(s)a
Effect allele(s) (Reference)
Method of allele identification
Abacavir
HIV-1 (U.S. Food and Drug Administration et~al. 2013)
Multiorgan clinical syndrome
HLA-B*5701 (Mallal et~al. 2002)
Candidate gene
ACE Inhibitors
Angiodema risk-MME
rs989692 d (Pare et~al. 2013)
GWAS,e Candidate gene
Allopurinol
Gout, hyperuricemia, nephrolithiasisb (Clinical Pharmacology Database 2011)
Serious dermatological reaction
HLA-B*5801 (Hung et~al. 2005)
Candidate gene
Atomoxetine
ADHD (U.S. Food and Drug Administration, Center for Drug Evaluation and Research 2014b)
Increased response (poor metabolizers)
CYP2D6 f (Ring et~al. 2002)
Response
NET/SLC6A2 g (Ramoz et~al. 2009)
NET/SLC6A2: candidate geneg
Carbamazepine
Epilepsy, trigeminal neuralgia
Serious dermatological reaction
HLA-B*1502 (Man et~al. 2007)
Candidate gene
HMG-CoA reductase Inhibitor
Hypercholesterolemia, hyperlipoproteinemia, hypertriglyceridemia, myocardial infarction prophylaxis and stroke prophylaxisb,h (Clinical Pharmacology Database 2013b)
Myalgia risk
GWAS
Irinotecan
Colorectal metastatic carcinoma (U.S. Food and Drug Administration, Center for Drug Evaluation and Research 2006)
Neutropenia risk- UGT1A1*28 (Ando et~al. 1998)
Candidate gene
Phenytoin
Seizures (U.S. Food and Drug Administration, Center for Drug Evaluation and Research 2014a)
Serious dermatological reaction
Candidate gene
Warfarin
Coagulation prevention
CYP2C9: PCR genotypingi
VKORC1: pos cloning, candidate gene, GWAS
7.2 GWAS Background: Overview of Gene Mapping
Our genes are located in a linear fashion along strands of deoxyribonucleic acid (DNA) that are divided into 23 pairs of chromosomes within the cell nucleus. This linear architecture provides the opportunity to map and identify the genes that contribute to traits such as drug response. To begin, we assess whether a trait such as drug response has a genetic component and we estimate the trait’s heritability. A significant heritability means that a fraction of the inter-individual variation in that trait is the result of variation due to genes (The 1000 Genomes Project Consortium 2010). These heritable traits are excellent candidates for gene mapping, which is designed to identify the specific genes contributing to the trait using information on gene marker locations along the chromosomes. Unfortunately, it is usually difficult to assess whether a particular response to a drug is heritable. This information would require the analysis of panels of related individuals, such as monozygotic and dizygotic twin pairs, who both receive the same pharmacologic agent. Concordance of response in those who share a greater proportion of their genes, the monozygotic twins, should be statistically greater than concordance in the dizygotic twin pairs. Such information is rarely available in an observational setting.
Linkage analysis is a well-established analytic tool that was used very extensively during the 1960s through the middle of the 2000s to map the genes for heritable traits to their chromosome locations. Linkage is based on a process that has been referred to as “reverse genetics”. That is, the approach works in the reverse order than the model describing how genes operate, biologically. While genes act in a forward fashion to produce and regulate the proteins that lead to a trait, reverse genetics starts with individuals having been measured for the trait, and uses linkage analysis, GWAS or other approaches to identify the predisposing genes. Here, the genes are identified last.
Reverse genetics became fully feasible in the 1990s, when a very extensive panel of multi-allelic markers or genetic variations spanning the human genome was identified. Linkage analysis is a statistical method that follows genotyped marker alleles and measured trait values within family pedigrees to identify chromosome regions where the marker alleles and trait values are aligned. Alignment is assessed statistically and helps us infer that alignment is seen in a certain chromosome region more than one would expect by chance alone. That is, we infer that the gene leading to some aspect of the trait value is ‘linked’ to a marker with a known location along the chromosomes. If the whole genome is analyzed for linkage with the trait, the approach is referred to as a full genome linkage scan. If specific genes are tested, they are referred to as “candidate genes”. In summary, in the regions exhibiting significant linkage, we infer that the gene affecting the trait is close to the linked marker, and reverse genetics is achieved. With linkage, the resolution at the locus is usually quite poor, as many genes can reside within a linked region. Nevertheless, a statistically significant linkage result limits the search for the predisposing gene to those in the linked region. Using the advances made by the Human Genome Project, reverse genetics has been very effective in identifying genes causing rare single gene traits that only result from mutations in a single or a few different genes.
A major change in gene mapping occurred during the last 10 years, with the development of the essential tools for successful GWAS. “Reverse genetics” is also used to conduct GWAS, however, the genotyped markers are bi-allelic, having only two versions, and referred to as single nucleotide polymorphisms (SNPs). These markers occur more frequently and are spaced much more closely than linkage markers. The collection of SNPs on our chromosomes developed over evolution. SNPs are random changes to the DNA that are passed down among humans over time. Most SNPs have no detectable effects on those who inherit them, but their proximity on the chromosomes to the changes that do predispose to traits of interest makes them valuable. This chapter is devoted to presenting the current study designs and methods for testing the GWAS SNPs to identify predisposing genes through association, which can ultimately inform pharmacogenetics.
7.3 Concepts, Designs and Statistical Methods for GWAS
We begin with a brief overview of the GWAS approach, assuming the trait under analysis is binary. For example, an individual either exhibits a particular side effect when given the drug, or they do not. Individuals that exhibit this trait form the sample of cases, and a group matched for age, sex, ethnicity, and other relevant factors, including ethnicity, form the control group. Both samples are genotyped using very dense SNP marker panels that have become available on commercial arrays. These genotyping arrays have evolved over time to contain an increasing number of SNPs, and most often we see studies with one million SNPs, each having a known location along the chromosomes. Genes that contribute to the trait are identified by statistically testing the individual SNPs for an association with the trait, in this case comparing the cases and controls. It should be emphasized that the SNPs themselves are just landmarks along the chromosomes and are not likely to predispose to developing the trait. They just mark alleles that contribute to the trait by their proximity. That is, identifying that a SNP is associated with the trait indicates there is likely to be an allele of a gene that contributes to the risk for developing the trait within a small chromosomal region close to that SNP. This is because the commercially available GWAS SNP panels have been designed to capitalize on an important genetic feature referred to as linkage disequilibrium (LD).
7.3.1 Linkage Disequilibrium Among SNPs that Are in Close Proximity
LD is a genetic factor that allows us to conduct GWAS arrays that provide the genotypes of one million SNPs when there are an estimated 30 million over the whole population. It is ubiquitous along the chromosomes and reflects the genetic history of a population. Thus, we expect LD to be found between the trait allele and a nearby SNP allele if the trait and SNP alleles have traveled together in close proximity on the same chromosome throughout time and there have been only a few crossovers between them. Crossovers occur when gametes are formed and the probability of a crossover between two SNPs is correlated with the distance between the SNPs. We can use the genotype of one allele to predict the genotype of the other if LD exists between the two. The mechanism by which fewer genotyped SNPs can capture the variation of other SNPs in close proximity is referred to as “tagging.” Tagging is illustrated Fig. 7.1, where the SNP genotype sequence for 16 SNPs is given for 6 people, with each person’s DNA sequence represented by a row. It is clear that the SNPs are not distributed independently within individuals. There are patterns that reflect LD. For example, the first and second SNPs are in LD and they ‘tag’ each other. Therefore, only one of them has to be genotyped. If a person has an “A” at the first SNP, they will have a “G” at the second. Likewise, a “T” at the first indicates there is a “T” at the second. Similarly, SNPs 4, 5 and 6 tag each other.
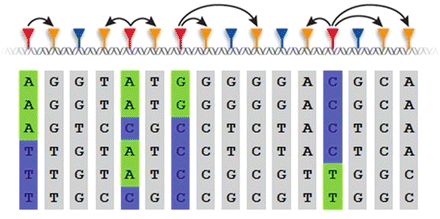
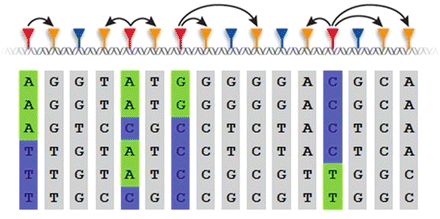
Fig. 7.1
Illustration of tagging SNPs
In the next sections we describe the biological concepts applied in conducting GWAS and explain the appropriate statistical methods to identify important associations leading to enhancement of our pharmacogenetics knowledge.
7.3.2 Alleles and Minor Allele Frequencies
Alleles are the key elements in genetic variation. They differ from each other in a small genetic element and result in alternate versions of the same gene. Some alleles will change the protein product of a gene, some will affect the amount of protein product produced, and some will be neutral and have no obvious effect. Population geneticists study the frequency of alleles and make inferences about population history from them. Those interested in personalized medicine use the frequencies to identify the genes contributing to traits. One way to describe an allele in a population is to estimate its minor allele frequency (MAF). The MAF is estimated by drawing a sample of individuals and counting the number of copies of that allele in the sample and divide by the number of alleles at that locus, which is two times the number of individuals in the sample, because, for each individual, a genotype is composed of two alleles, one from the mother and one from the father.
7.3.3 GWAS Study Designs and Statistical Analyses
Unlike other methods of searching for genes associated with a trait, GWAS are noteworthy for being genome-wide, producing an unbiased search that does not rely on candidate genes. To begin, the study sample is collected. Then, a microarray of SNPs is genotyped for each individual. The microarray is purchased from a company that specializes in genotyping chips and the genotyping is usually done at a facility that specializes in that work. Most genetic centers have core facilities with the technology and expertise to generate genotypes. The current commercial genotyping chips have about one million SNPs on them. Sometimes there are specialized chips that have been designed for specific classes of disorders. Regardless of the microarray used, the methodology to clean and process the data will be the same. This is described in Sect. 7.5. Currently, there is preliminary work generating SNP genotypes using exome or whole genome sequencing, but the high cost and other considerations associated with data processing have not yet made it fully feasible.
7.3.3.1 Case and Control Designs for GWAS
A case and control study design is used when the outcome of interest is dichotomous. For example, a dichotomous trait in pharmacogenetics might be whether an individual shows response to a drug. In the study sample of those who take the drug, those who have a positive response might be categorized as “cases”, and those who do not might be categorized as “controls”. All individuals are genotyped using the same microarray platform. There will be one million genotypes per individual, so special software to accommodate this volume of data is required. The PLINK software accommodates extensive genotype data (Plink 2009). The files are compressed into a binary format, and data cleaning and statistical analyses programmed into PLINK are conducted on the files that have been compressed. The case and control samples are tested for differences in MAF over the one million SNPs, each undergoing a separate statistical test. Multiple testing is a major concern, and the accepted GWAS approach is discussed in Sect. 7.4.1. The cases and controls should all be from the same ethnic group, as different ethnicities have different allele frequencies, which could lead to a significant number of type 1 statistical errors.
< div class='tao-gold-member'>
Only gold members can continue reading. Log In or Register a > to continue
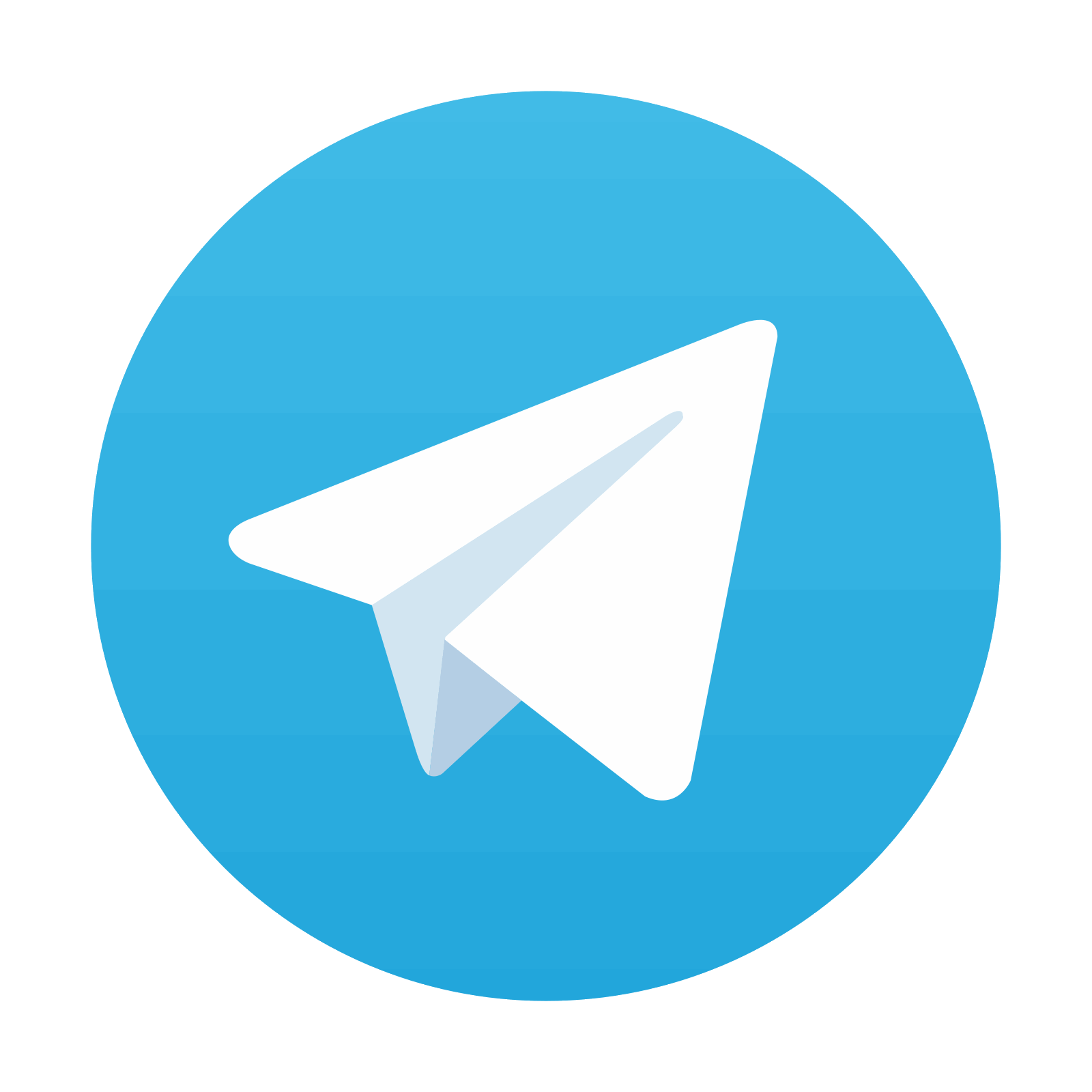
Stay updated, free articles. Join our Telegram channel

Full access? Get Clinical Tree
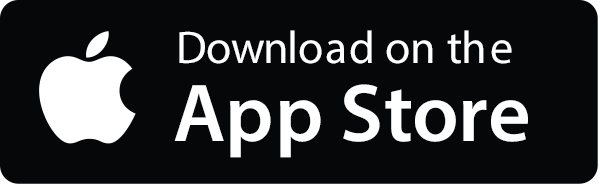
