Clusters of gene expression can then be tested to determine if any correlate with particular characteristics of the samples of interest. For example, profiling might indicate that a cluster of genes with a correlated expression profile is found more frequently in samples from tumor A than from tumor B, whereas another cluster of genes with a correlated expression profile is more frequent in samples derived from tumor B than from tumor A. Clusters of genes whose expression correlates with each other and with a particular set of samples constitute a so-called expression signature characteristic of those samples. In the hypothetical profiles in Figure 15-12, certain genes have a correlated expression that serves as a signature for tumor A; tumor B has a signature derived from the correlated expression of a different subset of these 100 genes.
Application of Gene Signatures
The application of gene expression profiles to characterize tumors is useful in a number of ways.
• First, it increases our ability to discriminate between different tumors in ways that complement the standard criteria applied by pathologists to characterize tumors, such as histological appearance, cytogenetic markers, and expression of specific marker proteins. Once distinguishing signatures for different tumor types (e.g., tumor A versus tumor B) are defined using known samples, the expression pattern of unknown tumor samples can then be compared with the expression signatures for tumor A and tumor B and classified as A-like, B-like, or neither, depending on how well their expression profiles match the signatures of A and B. Pathologists have used expression profiling to make difficult distinctions between tumors that require very different management approaches. These include distinguishing large B-cell lymphoma from Burkitt lymphoma, differentiating primary lung cancers from squamous cell carcinomas of the head and neck metastatic to lung, and identifying the tissue of origin of a cryptic primary tumor whose metastasis gives too little information to allow its classification.
• Second, different signatures may be found to correlate with known clinical outcomes, such as prognosis, response to therapy, or any other outcome of interest. If validated, such signatures can be applied prospectively to help guide therapy in newly diagnosed patients.
• Finally, for basic research, clustering may reveal previously unsuspected connections of functional importance among genes involved in a disease process.
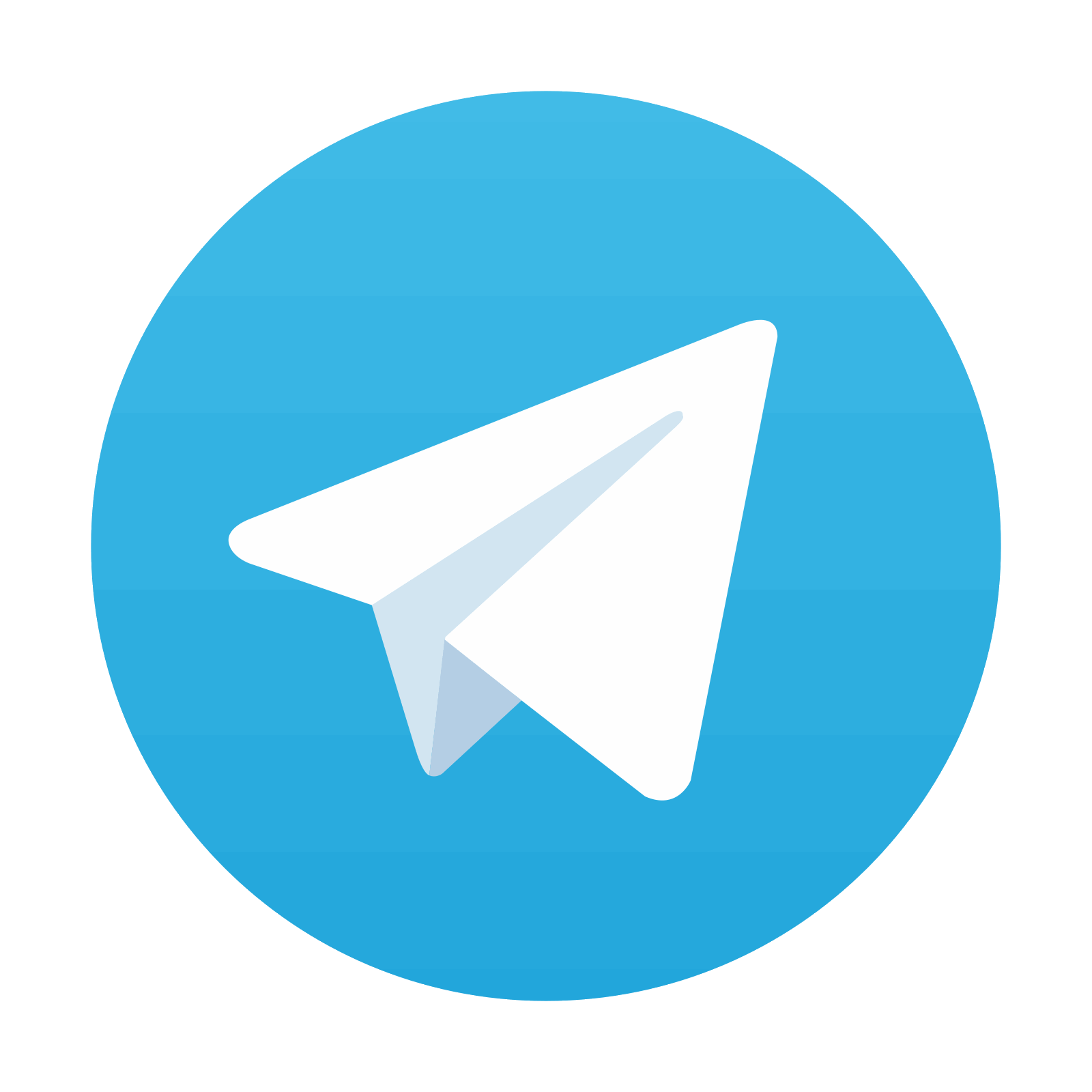
Stay updated, free articles. Join our Telegram channel

Full access? Get Clinical Tree
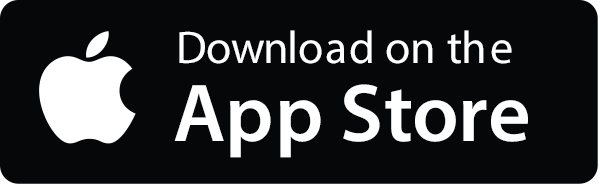
