9.1 Introduction
Random error and systematic error
Effective use of epidemiologic information requires more than knowing the facts. It requires understanding the reasoning behind the methods. A good place to enter into this understanding is to view epidemiologic studies as exercises in measurement with the objective of measuring either disease frequency (e.g., an incidence rate) or association (e.g., a rate ratio) as accurately as possible. With this said, we acknowledge that all measurements are affected by varying degrees of random error and systematic error.
Random error and systematic error represent distinct problems in epidemiology. This distinction can be illustrated with a metaphor. Imagine a marksman shooting at a target in which the bull’s eye represents the true value of the epidemiologic measure we want to learn about.
- The skilled sharpshooter with a properly calibrated sighting device consistently delivers shots to the bull’s eye center (Figure 9.1a). These shots are free from both random and systematic errors.
- If the sighting device of the gun is true, but the sharpshooter is forced to shoot from a randomly vibrating surface, the shots are scattered about the target (Figure 9.1b). These shots are free from systematic error but are affected by random error.
- If the sighting device of the gun is askew and the shooter is on stable, even ground, the shots will be consistently off-center (Figure 9.1c). These shots are free from random error but are affected by systematic error.
- If the instrument is improperly calibrated and the sharpshooter is forced to shoot from a vibrating surface, shots distribute themselves randomly and systematic error is present (Figure 9.1d).
With random error, each particular shot is unreliable but, on the average, the shots tend to center on the bull’s eye. With systematic error, shots are systematically off-center in a particular direction.
Parameters and estimates
Instead of considering sharpshooters, let us now consider epidemiologists “shooting” for the correct value of an epidemiologic measure. When calculating an incidence, for example, the epidemiologist is seeking the error-free value of the incidence in the population being studied. When calculating a risk ratio, (s)he is seeking the absolutely correct value of the risk ratio that describes the exposure–disease relation of interest.
We will refer to the error-free value of the epidemiologic measure as the parameter. For instance, when the epidemiologic measure of interest is a risk ratio, the risk ratio parameter quantifies the true effect of the exposure on the occurrence of the disease in relative terms.
Although parameters are objective characteristics of the population being studied, they are impossible to observe (read: calculate) directly. Instead, we calculate an imperfect estimate of the parameter based on the data from a study. This imperfect estimate is prone to both random and systematic errors. Thus, the parameter is analogous to the bull’s eyes of the targets in Figure 9.1, while a given estimate is analogous to a single shot.
Accordingly, we require different notation to preserve the distinction between the parameter we are trying to estimate and the estimate itself. In general, estimates will now carry an overhead hat (^) while parameters will remain hatless. For example, will represent a measure of association estimate from a particular study. In contrast, MA will represent the (error-free) measure of association parameter.
We can now think of independent studies labeled 1 through k deriving independent measures of association ,
, …,
. If these estimates were free of systematic error, they would scatter randomly around the “bull’s-eye” of the parameter. If they were free of random error, they would cluster tightly somewhere on the target. If they were free of both systematic error and random error, they would cluster tightly around the “bull’s-eye”—this is what the epidemiologist is “shooting for” (Figure 9.2).
Figure 9.2 Target metaphor applied to measures of association estimates () aiming at a parameter (MA).

A complementary way to conceptualize the problem of measurement error is to view each calculated estimate as the value of its underlying parameter plus “error terms” for random and systematic error:
The random and systematic errors inherent in an estimate bring the value of the estimate away from or toward the true value of the parameter. For example, an observed (calculated) risk ratio estimate of 3 might represent an overestimate of the risk ratio parameter by 1 with random error shifting the estimate up by 0.25 and systematic error shifting it up by an additional 0.75.
Although the amount of random error can be estimated from the data in the form of a standard error (or variance), the amount of systematic error cannot be easily quantified. Because random error and systematic error represent different types of problems with different types of solutions, they will be addressed separately.
9.2 Random error (imprecision)
Probability
It is often said that random error is governed by the laws of probability. However, probability is not easily defined when describing natural phenomena.
A fundamental question that arises about probability and natural phenomena is whether we are talking about probability as an objective construct of the world, or whether we are discussing probability as a way to quantify our limited understanding of a situation. The former posits chance as an inherent property of nature that affects natural phenomena from the genes we inherit to the environment into which we are born. The latter posits probability against a background of incomplete knowledge.
Consider the flip a coin. We say that the probability it will turn up heads is 50%. An objective view of probability says that if the coin is flipped many, many times, we expect to see half of the flips turn up heads. It is easy to imagine that estimates of probabilities based on this method will become increasingly reliable as the number of replications increases. For example, if a coin is flipped 10 times, there is no guarantee that exactly 5 heads will be observed—the proportion of heads can range from 0 to 1, although in most cases we would expect it to be closer to 0.50 than to either 0 or 1. However, if the coin is flipped 100 times, chances are better that the proportion of heads will be close to 0.50. With 1000 flips, the proportion of heads will be an even better reflection of the true probability.
Now consider the flip of a coin in the context of our limited ability to predict its outcome. In theory, given enough information about the height and velocity of the flip, its rate of rotation, and the initial starting position of the coin in relation to the ground, the probability of it turning up a head might be predicted with greater than 50% certainty. Thus, the probability has changed based on what we know. According to this second view of probability, probabilities are defined in terms of the variability in the data that cannot otherwise be explained.
This second view of probability has relevance when studying disease occurrence. With no background knowledge, it might be sensible to say a person has less than a 1% probability of developing lung cancer during a lifetime. But if we then discover the person is male and smokes cigarettes, a better estimate for this probability might be 17% (Villeneuve and Mao, 1994). Thus, the objective state of affairs has not changed but the revised probability has changed because our knowledge about underlying conditions is now different. This revised probability conveys the extent to which we now believe the event is likely to occur.
Fortunately, appreciations of these two views of probability are both founded on our experience of the relative frequency of phenomena.
When we say that the probability of a coin coming down heads on being tossed is one-half we have in mind, I think, that if it is tossed a large number of times it will come down heads in approximately half the cases. Even in extreme cases, say, when we attempt to assess the probability of a horse winning a given race, an event which cannot be repeated, we are, I think, picturing our estimation as one of a number of similar acts and assessing the relative frequency of the horse’s victory in that population.”
Kendall (1947, pp. 165–166)
Suppose a laboratory experiment wants to learn about the teratogenicitya of an agent. The investigator realizes that even genetically identical mice bred under identical laboratory conditions will express variable rates of congenital malformations when exposed to the teratogen. It is against this background of unexplained random noise upon which judgments will be made. The random noise in an experiment is given different names. It is called “experimental error,” “biological variation,” or “chance.” By any name, this is the variability in the outcome that cannot otherwise be explained.
In laboratory experiments we try to limit this random noise by controlling environmental conditions. But even here, unforeseen factors affect the outcome being studied. Ambient conditions vary, some mice eat more than others, batches of feed are not perfectly uniform, the pathologist diagnosing malformations may miss or misinterpret findings on necropsy, and so on. Randomness happens. Under an assumption of randomness, the effects from these unexplained factors are assumed to be independent of the treatment being studied and the random error associated with the estimate will follow a predictable probability distribution and can thus be dealt with mathematically.
Regardless of how one views probability and randomness, one thing is certain: as the size of a sample increases, the amount of random error associated with statistical estimation decreases. A brief consideration of sampling will illuminate this property.
Suppose an investigator wants to estimate the prevalence of smoking in a high school. The investigator is aware that any given random sample will not be an exact replica of the high school population. For example, a given sample of 10 may have three admitted smokers. However, the next sample of 10 may have five smokers. This is referred to as random sampling error.
The investigator is also aware that the amount of random sampling error in a sample will lessen if the sample size is increased. When based on a sample of, say, n = 10, sample-to-sample variability will be great. For example, a first sample may show 2/10 (20%), a second sample may show 5/10 (50%), and a third sample may show 3/10 (30%). However, larger samples of, say, n = 100, will derive more stable statistical results. For example, the first sample may show 29/100 (29%), the second sample 35/100 (35%), and the third sample 37/100 (37%). Estimates from large samples contain less random error than estimates from small samples.
On top of the problem of random sampling error, the investigator is concerned about systematic errors. Some of the teenagers in the survey may misrepresent their smoking habits (information bias). In addition, if given leeway, the interviewer may find it easier to select subjects from among teenagers who are easier or more pleasant to interview (selection bias). These systematic errors are quite different from the aforementioned random sampling error. Whereas the amount of random error will diminish with increasing sample size, the amount of systematic error is unaffected by sample size. The laws of probability are not suited for handling systematic errors.
Introduction to statistical inference
Statistical inferenceb is the process by which we address random error in data. The landmark statistical paper written by Gossett written under the pseudonym Student (1908) made the point in reference to the random error in experiments as follows:
Any experiment may be regarded as forming an individual of a “population” of experiments which might be performed under the same conditions. A series of experiments is a sample drawn from this population.
Now any series of experiments is only of value in so far as it enables us to form a judgment as to the statistical constants of the population to which the experiments belong. (note emphasis added)
The same manner of thinking is applied to observational (nonexperimental) studies. To paraphrase “Any series of observational studies is only of value in so far as it enables us to form a judgment as to the statistical constants of the population to which the observational studies belong.” Note that the statistical constants to which we refer are the parameters we have so far been discussing.
The two standard methods used to infer parameters in statistical analyses are estimation and hypothesis testing. The objective of estimation is to “locate” the value of the parameter. The objective of hypothesis testing is to test a claim about the parameter. Let us start by considering estimation.
Estimation (confidence intervals)
Estimation comes in two forms: point estimation and interval estimation. Point estimation provides the most likely value of the parameter. Interval estimation provides a range of values for the parameter in the form of a confidence interval.
Consider a rate difference of . This is the point estimate for the true but unknown value of the underlying rate difference parameter. To construct a confidence interval for this parameter, we surround the point estimate with a calculated margin of error. Suppose the margin of error associated with the aforementioned risk difference estimate is
with 95% confidence. Then, the 95% confidence interval for the rate difference parameter is:
In this example, is the lower confidence limit (LCL) and
is the upper confidence limit (UCL) (Figure 9.3). We can now say with 95% confidence that the rate difference parameter after accounting for random error (but not for systematic error) lies between these limits.
The confidence level of a confidence interval quantifies our confidence in the procedure used to create the interval. Confidence intervals can be calculated at almost any confidence level. However, the most common level of confidence are 95, 90, and 99%. A 95% confidence interval, for example, is designed to capture the parameter 95% of the time. This means that 5% of such intervals will miss the parameter. In addition, keep in mind that this technique addresses random error only and provides no protection against systematic errors.
The length of confidence interval reflects the estimate’s precision. Long confidence intervals indicate that the estimate is imprecise; narrow confidence intervals indicate that the estimate is precise. All other things being equal, studies based on a large number of cases produce narrow (precise) confidence intervals; studies based on small numbers produce wide (imprecise) confidence intervals.
A common misapplication of confidence intervals is to view them as “significant” or “not significant” by comparing their limits with a fixed value. This reduces estimation to a fixed-level hypothesis test—a process that is be discouraged when studying natural relationships. An example of this misapplication is illustrated by considering a relative risk of 1.7 with a 95% confidence interval of from 0.9 to 3.1. While some readers may interpret this confidence interval as insignificant because it does not rule out a relative risk of 1 with 95% confidence, it ignores the fact that data are as compatible with a relative risk of 3.1 as they are with a relative risk of 0.9. In addition, it is quite possible that, say, the 94% confidence for the same data would exclude a relative risk of 1 from its midst. ‘Surely, God loves 94% confidence nearly as much as 95% confidence’ (adaptation of a quote in Rosnow and Rosenthal, 1989).
Figure 9.4 displays 95% confidence intervals for relative risks from 10 prospective cohort studies on total fat consumption and breast cancer. Study 2 and study 7 present the most precise estimates. Study 4 offers the least precise estimate. Some studies demonstrate relative risk point estimates that are slightly greater than 1 (e.g., studies 3, 4, 5, 6, and 9), and some demonstrate relative risks that are slightly less than 1 (e.g., studies 1, 2, and 7). None of the studies by themselves are precise enough to rule out chance as an explanation for their observed direction of the association. Thus, taken as a whole, these data suggest that there is no association between total fat intake and breast cancer risk.
Figure 9.4 Confidence intervals for Illustrative Example 9.3. Relative risks of breast cancer and total fat intake, prospective cohort studies. Graph based on the data in Table 3 of Hunter and Willet (1994).

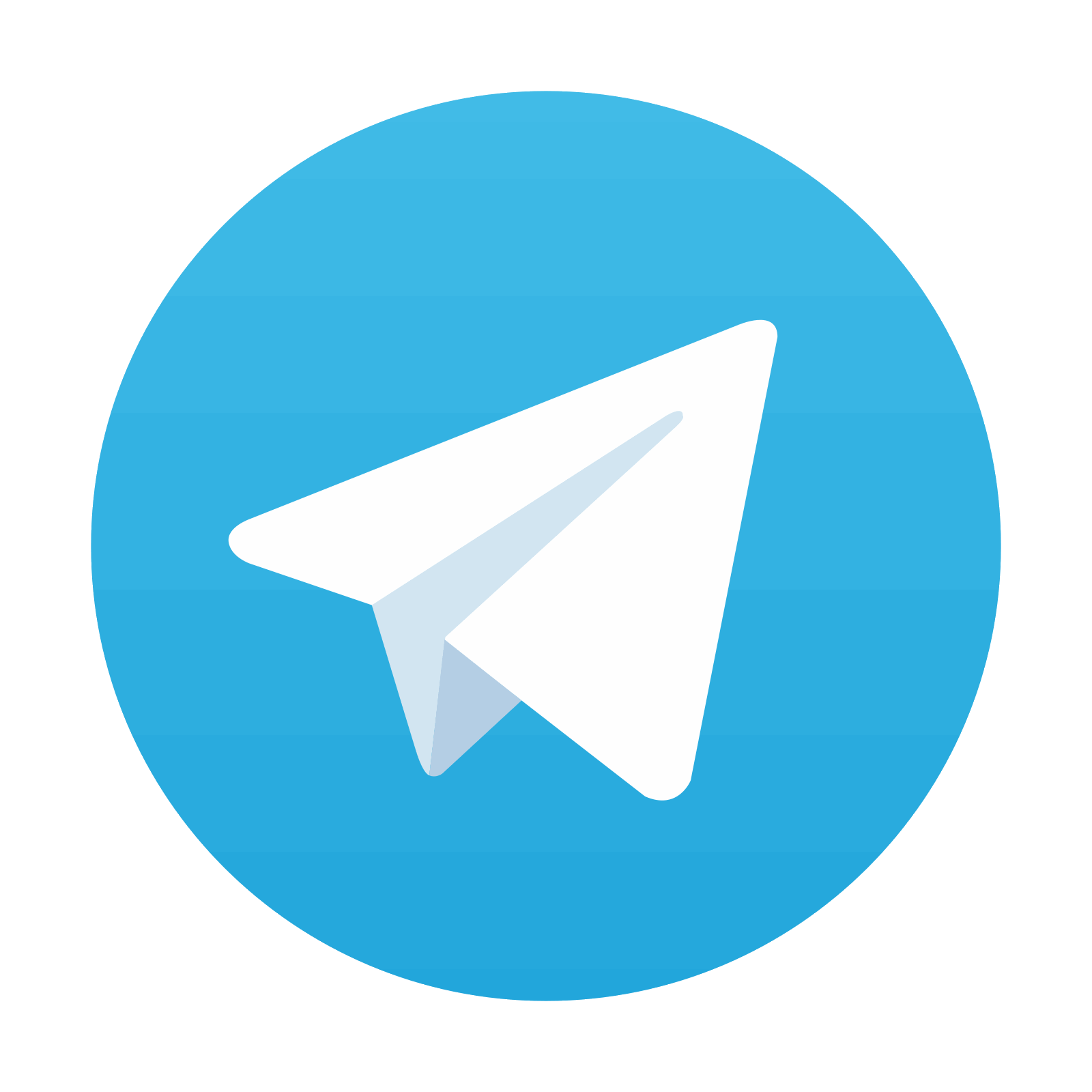
Stay updated, free articles. Join our Telegram channel

Full access? Get Clinical Tree
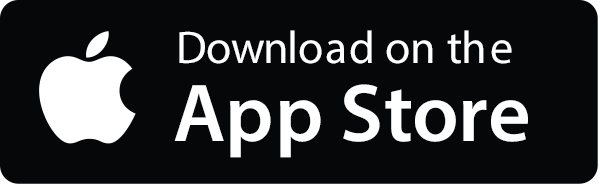
