Challenges
So what have we learned from this? The totality of evidence indicates that beta-carotene is a good marker of (and part of) a beneficial diet, but is insufficient on its own to provide any protection. We have also learned that dietary epidemiology is more challenging than was initially appreciated, and that due attention needs to be given to the challenges of measurement and confounding in observational studies; we want to minimise dilemmas such as those depicted in the cartoon for the public and for policy makers.
Compelling observational data such as those seen for smoking are, unfortunately, the exception. The multivariable and often hard-to-identify and remote causes of many chronic diseases are generally much weaker, leaving many studies under-powered with more room for results to vary by chance, quite apart from the real potential for weak effects to be due to residual confounding or bias. The resulting papers report weak or moderate effects (RRs less than two) which may well differ among studies investigating the same relationship. One result is a flow of sometimes contradictory newspaper headlines and journal articles depicting new panaceas or lifestyle risks. This highlights the importance of a sound systematic review to provide a balanced explanation and presentation of all relevant data (Chapter 11) and the need for authors, editors and medical journalists to show restraint and avoid the premature trumpeting of ‘interesting’ findings from the report of a single study.
So how can we address these challenges?
Synthesis and integration
The increasing focus on translational epidemiology (Khoury et al., 2010; Hiatt, 2010) offers a useful counter to those who have questioned the value of epidemiology, by promoting the value of data synthesis and collaborative engagements across research groups, as well as increased integration of epidemiology with other disciplines relevant to public health, especially social and laboratory sciences. The first two endeavours help minimise the play of chance and allow direct assessment of consistency of findings, while the third broadens causal perspectives and, through advances at the molecular and genetic level, permits sharper measures of both diseases and exposures.
The research outputs from epidemiology fit well with the growing desire for public health and medical research to be ‘translational’, i.e. directly applicable to a population or patient. Hiatt (2010) puts epidemiology at ‘the epicenter of translational science’.
The Cochrane Collaboration has set a superb example by encouraging investigators to conduct practically directed data syntheses in a standardised manner and, since its inception in 1993, Cochrane contributors have produced thousands of systematic reviews evaluating health care interventions (Ferrie, 2015). Collaboration among researchers is also becoming more common and an increasing number of groups of epidemiologists are sharing their data to permit direct pooling of their results with results from other studies. One of the first of these was established at Oxford University to help pin down the long-contentious relationship between use of the oral contraceptive pill and a woman’s risk of breast cancer (Collaborative Group on Hormonal Factors in Breast Cancer, 1996). Finally, recognising the need for integration across disciplines, funding bodies are increasingly demanding that research be ‘multidisciplinary’, bringing together scientists from a range of relevant fields. In addition to epidemiologists, these groups may include demographers, geographers, social scientists and health policy experts who provide perspective on the upstream ‘macro’ level drivers of health as well as geneticists and molecular biologists who add insights at the ‘micro’ level regarding individual susceptibility to disease and more sophisticated ways to classify disease (see, for example, serotyping of infectious agents in Chapter 13). The rapidly increasing integration with bench (laboratory) science, where new, sophisticated tools and the mapping of the human genome provide a welter of molecular, genetic and epigenetic data, complements the standard personal and health information of traditional epidemiology. However, although it is easy to focus on individual-level risk factors when searching for the causes of disease, we should not ignore the fact that that these exist within a much broader and more complex web of social and environmental factors (McMichael, 1999).
A qualitative sense of some of these multifactorial approaches is given in Figure 16.1, which shows the eco-social model which guided the design and research questions of the Thai Health-Risk Transition Study, a large-scale cohort study established in 2005 to evaluate forces behind changing disease patterns in Thailand (Sleigh et al., 2008).
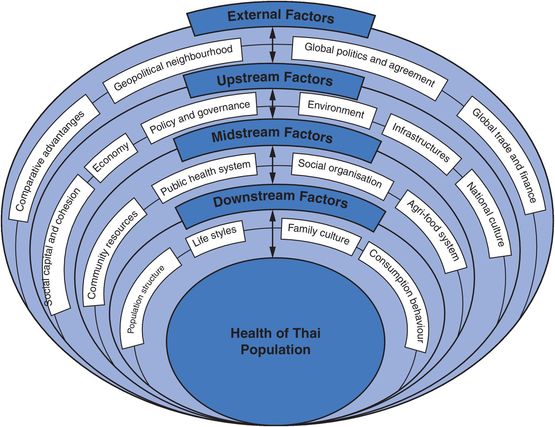
Multilevel model adopted for the Thai Health-Risk Transition Study.
At another level, we have also briefly discussed lifecourse epidemiology (Kuh and Ben-Shlomo, 2004) which brings temporal integration. It aims to identify and integrate exposures and other influences across a person’s life (as far back as conception, and possibly beyond) and relate them to their health at different ages. Capturing details of habits, diets and other aspects of early life is mostly too much to expect from retrospective questioning in adulthood, but historical records from early surveys, schools and hospitals have proved invaluable. For example, a group of Bristol epidemiologists identified good dietary records from the 1930s for over 4000 children, more than 85% of whom have been followed up to the present for mortality, and a proportion resurveyed six decades later (the Boyd Orr study; Frankel et al., 1998). A number of pregnancy and birth cohorts have also been established to collect detailed early-life data prospectively, with the intention of life-long follow-up of the study participants (for example the ALSPAC Study, see Box 4.3). Improvements in data linkage will help these studies ensure high follow-up rates, something that will be critical to avoid selection bias in the long term.
Limiting error
We have given a lot of attention to the challenges of error and confounding throughout the book so will not revisit these in detail here, but dealing with these biases effectively is crucial for epidemiology to be a consistently useful translational discipline. New analytic methods for identifying, quantifying and (to a degree) dealing with bias and confounding provide some tools to help us achieve this. By making the relationships between the variables we are studying explicit, directed acyclic graphs (DAGs) can sharpen our causal thinking and improve our ability to identify and control for confounders appropriately. Some of the newer methods we have alluded to like instrumental variables and Mendelian randomisation, itself a spinoff of our new genetic knowledge, offer the potential to remove, or at least reduce, the bias in our analyses. And perhaps most importantly, keeping an eye on the exchangeability of the groups we are comparing can help us design better studies as well as in assessing whether selection bias or confounding might affect our results. In addition to this we must continually strive to sharpen our measurement – not just of exposures and the outcome we are interested in, but also of confounders so that we can adjust for these more fully. In particular, anything we can do to decrease random misclassification will increase the precision of our estimates and so help clarify weaker associations that lie close to the null.
Improving measurement
As epidemiology is, at its core, a measurement science we will expand a little further on this here. New technologies now allow more sophisticated measurement both of exposure (e.g. serum biomarkers, DNA damage in cells) and of outcome (e.g. early cellular changes, molecular subtypes of disease based on genetic profiles). Increasing the precision and accuracy of our measurement is paramount, but we also need to be highly specific about what we mean by exposure and outcome. A single infectious agent may have different strains, cigarettes have varying levels of tar, menopausal hormone therapy comes in different formulations and doses, and so on. Duration, intensity, pattern and timing of exposure may be critical. Is someone who drinks a glass or two of wine each night getting the same ‘causal dose’ of alcohol as their neighbour who drinks only on Friday nights but then drinks a bottle or two? For liver cirrhosis the answer might be ‘yes’, but for risk of injury it would clearly be ‘no’. Similarly, we need maximum precision in defining outcomes. Uterine cancer was recorded as one disease until the mid-twentieth century when cancers of the body of the uterus were separated from those of the cervix (neck of the uterus), as they are histologically quite distinct. They were then found to have completely different risk factors: obesity and oestrogen exposure for endometrial cancer and human papillomavirus (HPV) infection for cervical cancer, requiring very different preventive strategies. We now know that risk factors also differ for different histological types of cancer at the same site – for example, cigarette smoking is associated only with the rare mucinous type of ovarian cancer (Collaborative Group on Epidemiological Studies of Ovarian Cancer, 2012) while a history of endometriosis is associated only with two other histological types (endometrioid and clear cell) (Pearce et al., 2012). With our increasing genetic and molecular capability we can now subdivide the histological types of cancer further based on their molecular characteristics. In many cases these ‘molecular subtypes’ have a very different prognosis and, in the case of breast cancer, they are now used to determine treatment as different drugs target different molecular characteristics of the cancers. For example, Herecptin® is given only to women with breast cancer if their cancer tests positive for human epidermal growth factor receptor 2 (HER2). This sophisticated molecular information also allows us to refine our case definitions and thus has the potential to increase our ability to identify causal relationships by allowing us to study more homogeneous groups that are more likely to share a common aetiology.
A dramatic example of the effects of clearly defining both exposure and outcome comes from studies of the relation between HPV infection and cervical cancer (see also Box 16.3). In early studies HPV was detected in 30%–60% of cases and the observed associations suggested a 2- to 5–fold increase in risk. Improved detection methods mean that HPV is now identified in 99% of cases and, as a result, we now see odds ratios of 100–900 for the association between the presence of HPV DNA and risk of invasive cervical cancer (Franco and Tota, 2010). The massive effects of this improvement in measurement on the magnitude of the observed association are shown in Figure 16.2. This increasing precision has in turn helped identify the best targets for the recently introduced vaccines against HPV intended to prevent cancer of the cervix. Good translation thanks to improved measurement.
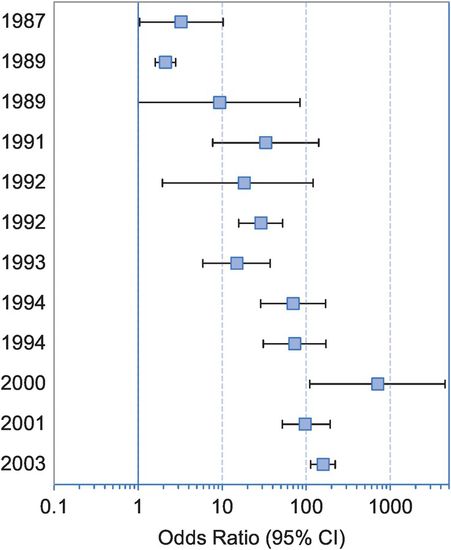
Odds ratios and 95% confidence intervals (CI) for the association between human papillomavirus (HPV) infection (via HPV DNA detection) and invasive cervical cancer risk in successive molecular epidemiologic studies.
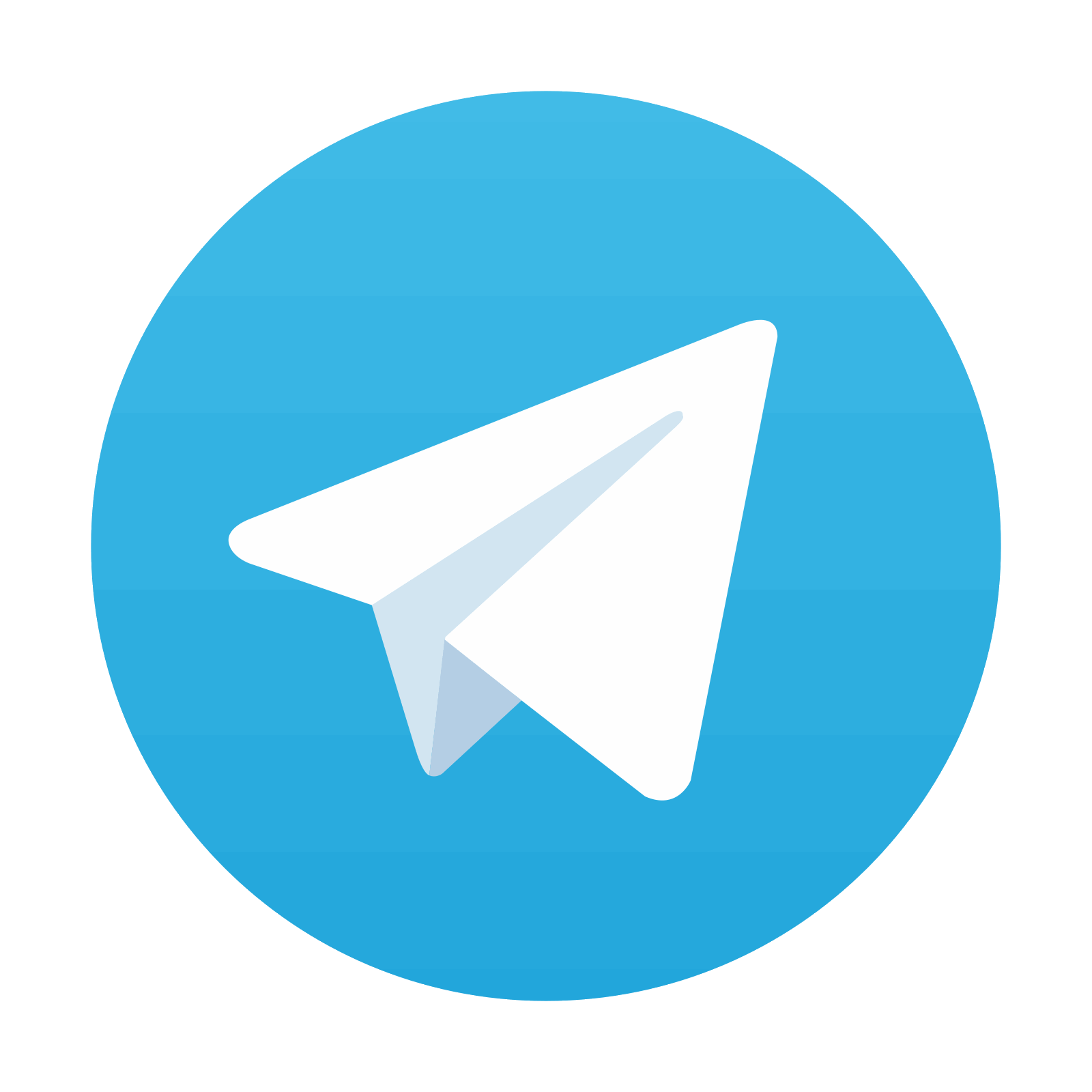
Stay updated, free articles. Join our Telegram channel

Full access? Get Clinical Tree
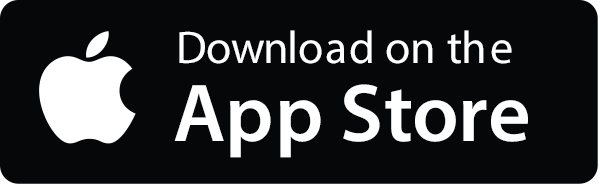
