21 Michael S. Bloom and John E. Vena Environmental epidemiology has become perhaps one of the most challenging specialty areas in human health research, with a complex theoretical paradigm and causal pathways involved that necessitate a multidisciplinary approach with toxicologists partnering with epidemiologists. Therefore, toxicologists and epidemiologists must learn each other’s discipline, become familiar with the special use of terms and concepts, understand these concepts, and have insights into the methodology used by each other’s discipline. We must learn to appreciate and understand different approaches and scientific cultures and perspectives. In this chapter, we highlight the principles of the epidemiologic approach as it applies to environmental and occupational exposures and health, with attention to toxicologic applications in the field and with specific examples. More specifically, this chapter will address: Associations between risk factors and human disease are exceedingly complex. Exposure scenarios are diverse. There are multifactorial etiologies and chronic low-dose risk factor exposures are usually involved. These exposures are concomitant with long latency periods to the development of many outcomes or study events of interest. It has become clear that there is a need to study intermediate causes and responses to disease. The environmental health paradigm involves mechanisms that affect the sequence of outcomes from release of an agent, or risk factor, involving transport, transformation, and fate processes, which lead to environmental concentrations and human exposure. There are also demographic, geographic, and lifestyle attributes that influence human exposure potential. There are toxicokinetic issues with regard to bioavailability, absorption, distribution, metabolism, and excretion that lead to internal doses and doses to the target tissues or sites. Finally, there are toxicodynamics such as compensation, damage, and repair eventually leading to the adverse outcome or effect. As noted by Saxon Graham in his seminal paper on the sociological approach to epidemiology, breaking the etiological chain anywhere along this continuum can prevent an adverse health outcome. Therefore, it is important for environmental epidemiology to explore all facets of this theoretical paradigm and most importantly to develop indicators or markers of exposure as well as disease that indicate preclinical damage. There are also susceptibility issues with much current interest in gene–environment interactions, gene–gene interactions, and interactions with epigenetic modifications of the genome. The importance of multidisciplinary efforts in environmental epidemiology was reinforced in the early 1990s. There was clear recognition of the need for cooperation among the disciplines including epidemiology and toxicology, as well as industrial hygiene and risk assessment to improve biologic monitoring of both exposures and outcomes. Several other issues that traversed many of the discussion topics were the importance of biologic mechanisms of toxicity, development of biological indicators, or biomarkers of exposure and outcomes and the value of cross-training environmental health professionals including epidemiologists and toxicologists. Madia, in an editorial in the journal Science, stated that environmental problems required the combined insight of a variety of scientific disciplines to understand the complexity of exposure pathways and related health effects. He went on to say that dealing with this level of complexity required not just more research, but a revolutionary new approach. Creativity and innovation in this new setting requires utilization of new technologies and the integration of various disciplines including epidemiology and toxicology. The number of potentially hazardous agents employed in modern industry and manufacturing increases is vast; there are more than 80,000 compounds in use in the United States. Approximately 3000–4000 of these compounds are produced in high volume, and hundreds of additional chemicals are introduced into the United States on an annual basis; myriad agents are detected in human specimens. Although a majority of these compounds are likely innocuous, an integrated approach incorporating epidemiology and toxicology is increasingly required to identify and characterize human risk as illustrated in Figure 21.1. Figure 21.1 Integration of toxicology and epidemiology for evaluating human health risks. Source: Adapted from Jaffery et al. (2002). Experimental studies are invaluable for the identification of agents posing a potential human hazard, but observational human studies are necessary to accurately quantify the concentrations of an agent that are hazardous to human health. Conversely, experimental studies are often necessary to characterize the mechanism of toxicity for an association observed in human populations, lending biologic plausibility to agent–outcome associations. Associations between potentially hazardous agents and human health outcomes are frequently identified first by occupational epidemiologists, who recognize and report on exposures in the workplace that are often higher than in the general populations and in which study populations are more well defined. Later, associations identified in the workplace by occupational epidemiologists may be considered among the general population by environmental epidemiologists. Initial concern for exposures in the workplace and/or in the environment is often prompted by the results of toxicologic investigation in the laboratory. For example, multidisciplinary evidences from toxicologic and epidemiologic studies, both occupational and environmental, provide the “weight of evidence” circumscribing the myriad hazards of human dioxin exposure. Environmental and occupational epidemiology by necessity involves approaches by interdisciplinary teams that involve experts from many disciplines including exposure assessors, biostatisticians, and especially toxicologists. A prime example is the New York State Angler Cohort Study, implemented in western and central New York by Vena et al. in the early 1990s. Toxicologists from the Toxicology Research Center, at the University at Buffalo, State University of New York, and the Wadsworth Center at the New York State Department of Health were an integral part of an interdisciplinary team and developed and implemented biomarkers of exposure and intermediate toxicologic outcomes. This collaboration entailed frequent team meetings to discuss and debate analytic methods, quality assurance and control, measurement error, and consistency of measures. Dialogue concerning disciplinary methods was essential to facilitate appreciation and understanding across disciplines, to foster learning and understanding of the approaches needed for innovative and cutting-edge research, and to generate knowledge generalizable to populations of concern and that could be used for risk assessment. Therefore, toxicologists and epidemiologists must learn each other’s discipline, become familiar with the special use of terms and concepts, understand these concepts, and have insights into the methodology used by each other’s discipline. A coupled role for epidemiology and toxicology is essential for discerning human health effects, and this is increasingly appreciated by experts in these respective fields. We must learn to appreciate and understand different approaches and scientific cultures and perspectives. Data collected from human studies is widely considered the “gold standard” for assessing human risk from potentially hazardous agents. However, with rare exceptions, it is impractical, infeasible, and unethical to conduct toxicologic experiments using human subjects; animal and in vitro models are thus invaluable tools for health risk assessment. While experimental animal and in vitro systems permit evaluation of an agent’s toxicity under rigorously controlled conditions, extrapolation of these data to the human experience is fraught with challenges. Interspecies differences in the bioavailability of an agent due to differences in absorption, distribution, metabolism, and excretion; unrealistic exposure scenarios, genetically homogeneous test populations; and nonphysiologic conditions are just a few of the limitations complicating the use of experimental data for predicting human risk and compelling investigators to collect data among human populations. Given the limitations inherent to the use of experimental models for human risk assessment, a nonexperimental or observational approach is required to ensure a systematic and repeatable strategy for the study of potential human health hazards. Epidemiology, translated literally from Greek roots as “…the study of that which is upon the people…” (epi, “upon”; demo, “people”; ology, “discourse”), is just such an approach. Using the epidemiologic approach, an investigator typically avails her- or himself of natural experiments to investigate variability in the occurrence of an outcome of interest (i.e., such as a disease) relative to a risk factor of interest (i.e., such as a chemical agent or element) and attempts to accommodate related but uninformative or nuisance sources of variability using statistical approaches. A Dictionary of Epidemiology defines the field as the “…study of the distribution and determinants of health-related states or outcomes in specified populations, and the application of this study to the control of health problems.” The overall aim of the epidemiologic approach is to generate knowledge that can inform policies and regulations to promote, protect, and restore human health. Studies of health-related outcomes among populations with involuntary exposure to hazardous agents fall within the purview of the subdiscipline environmental epidemiology. Populations are generally exposed to agents in their environment through inhalation of airborne contaminants, ingestion of contaminated food or water, or dermal absorption through direct contact with soil and dust, or with consumer products. Just a few examples of studies of exposure to environmental agents and human health outcomes are investigations of arsenic consumed in contaminated drinking water sources and spontaneous pregnancy loss, dietary exposure to polychlorinated biphenyls (PCBs) through contaminated animal fats and altered thyroid hormones, absorption of high-frequency nonionizing radiation through mobile phone use and brain tumors, or investigations demonstrating associations between secondhand exposure to environmental tobacco smoke and lung cancer. Environmental epidemiology studies are challenging to conduct as these often: Despite these challenges, the results of environmental epidemiology studies are often of substantial public health relevance, as the agents examined are frequently widespread and thus even small increases in risk translate to large numbers of attributed outcomes. Studies of health-related outcomes among populations with work-related exposure to potentially hazardous agents fall within the purview of the subdiscipline occupational epidemiology. For example, this might include investigations of associations between occupational diesel exhaust inhalation and lung cancer, exposure to toluene isocyanate during the manufacture of products from polyurethane foam and asthma, Percival Pott’s classic eighteenth-century study of scrotal cancer among young English chimney sweeps, or Irving Selikoff’s studies of mortality and asbestos exposure among insulation manufactures in the mid-twentieth century. Epidemiologic studies in occupational settings offer several advantages compared to environmental epidemiology studies, including: Despite some of the advantages offered by occupational health investigations, the results of such studies are also limited by several issues. The underlying distribution of risk for an outcome in a working population is likely to differ from that in the general population; employed persons are healthier on average than the unemployed component of the general population. This healthy worker effect may obscure associations between risk factors and outcomes of interest under certain conditions. Although exposure assessment is often more tenable in occupational epidemiology studies, as compared to environmental epidemiology studies, accurately assessing personal exposure to agents also presents with challenges in the occupational setting. Nonetheless, occupational epidemiologic studies often offer the opportunity to study high-dose exposures that can be extrapolated to the lower doses seen in environmental studies. Almost inevitably, the agents used in the occupational setting are discharged or released into the environment resulting in pathways to human exposure in far larger populations. To detect and quantify associations between risk factors and outcomes, or events of interest, epidemiologists employ a variety of metrics including rates and ratios, with the ultimate aim of assessing evidence for a causal relation. A selection of frequently used epidemiologic metrics can be found in Table 21.1. Epidemiologists count the number of outcomes of interest including deaths, diseases, injuries, or diagnoses, to name but a few, and describe these in terms of person (who is affected and who is not affected?), place (where are people affected or not affected?), and time (when are people affected or not affected?). These descriptive epidemiology data are useful for generating hypotheses, educated testable conjectures describing relations between environmental or occupational exposures to risk factors and study outcomes. Two types of study outcomes are counted, new or incident outcomes and existing or prevalent outcomes. To facilitate comparison between groups of different sizes and with different attributes, the frequency of an outcome is often expressed in terms of a risk or a rate—the number of outcomes experienced by a population unit or population–time unit across or during a time interval. Table 21.1 Common Epidemiologic Metrics and Measures of Association The incidence describes the probability for a new outcome in a specified population at risk—the number of new outcomes that accrue in a population at risk (Table 21.1). The incidence may be expressed in terms of numbers of people at risk for an outcome (i.e., cumulative incidence proportion) or in terms of the person-time at risk for an outcome (i.e., incidence density rate). The incidence is interpreted as the risk for an outcome. The instantaneous incidence rate, meaning the incidence rate over a time interval approaching zero, is referred to as the hazard rate and is a quantity conditioned on the probability that a participant did not experience an earlier outcome. These types of incident measures are essential for establishing causal associations between a risk factor and outcome of interest. For example, after accommodating difference in age distributions (i.e., age adjusted or age standardized), the U.S. incidence proportions for breast cancer were 121.0 cases per 100,000 white women and 117.0 cases per 100,000 black women in 2007. Thus, 121 new cases of breast cancer were diagnosed for each 100,000 White women, and 117 new cases of breast cancer were diagnosed for each 100,000 Black women in the U.S. population during 2007. Somewhat different from the incidence rate, the prevalence proportion describes the probability of an existing outcome in a population at a point in time (i.e., point prevalence) or during a time interval (i.e., period prevalence); prevalence includes past as well as new outcomes (Table 21.1). In a stationary population (i.e., no migration), the prevalence proportion is a function of the incidence rate and the duration of the study outcome such that prevalence ≈ incidence × duration. The prevalence proportion, while useful, cannot be interpreted as a risk as the duration of the outcome is presumed to vary by person, and thus, variability in prevalence will in part reflect time to recovery or death, contingent on the outcome. Somewhat rare outcomes with long duration, such as infertility (i.e., possibly lasting for many years) may have a high prevalence rate that belies a low incidence rate. In contrast, fairly common outcomes of short duration such as influenza (i.e., resolves or results in death in short time) may have a high incidence rate that belies the comparatively low prevalence rate. As a reflection of existing cases of a disease, injury, or condition, prevalence data are useful for formulating public policy and budgeting resources, as well as for generating hypotheses. For example, the prevalence of major congenital malformations has been reported to be 3% in U.S. live births; this value indicates that 3 of every 100 live infants have a major congenital malformation and reflects both the incidence of the malformation during gestation and the survival of a malformed fetus to delivery. Given that collection of incidence data is often costly and time-consuming as a population must be followed over time for the occurrence of new outcomes, prevalence data are often more readily available or readily collected for epidemiologic study. Although incidence and prevalence measures are of great utility in characterizing outcomes in terms of person, place, and time, these alone provide little insight into the causes of those outcomes. However, ratios of these values for different groups can provide valuable information in terms of the relative difference in incidence or prevalence. The ratio of incidence proportions or rates for an outcome among persons exposed to a risk factor of interest, such as a specific chemical agent, relative to the outcome among persons unexposed to that risk factor (i.e., referent group) is referred to as the relative risk (RR) or rate ratio (Table 21.1). Various ratios, including ratios of risks, rates, hazards, and prevalence proportions, are used by epidemiologists to characterize associations between risk factors and outcomes of interest. Ratios are unitless quantities with values exceeding one suggesting a higher probability for the outcome among persons exposed to a risk factor (i.e., adverse effect), values less than one suggesting a reduced probability for an outcome among persons with a risk factor (i.e., protective effect), and a value equal to one suggesting no association between a risk factor and an outcome. For example, in a hypothetical study of the association between female blood Cd level and infertility among couples trying to conceive (i.e., no pregnancy), an RR equal to 1.82 would indicate an 82% increase in the probability for infertility among women with high blood Cd concentrations, compared to women with low blood Cd concentrations. The attributable or excess risk describes the additional number of outcomes, or events of interest, experienced in association with exposure to a risk factor. It is defined as the absolute difference in incidence measures for the outcome of interest between those with exposure and those without exposure to the risk factor of interest (Table 21.1). One common interpretation of this value is that it represents the number of outcomes that would be eliminated in exposed members of the study population should the risk factor of interest be removed. For the aforementioned hypothetical example, a cumulative incidence rate of 182 cases of infertility (i.e., no pregnancy) per 1000 women with high blood Cd and 100 cases of infertility per 1000 women with low blood Cd suggests that 82 women did not achieve pregnancy due at least in part to high blood Cd. By extension, the attributable fraction describes the proportion of cases experienced by the exposed group, in association with the risk factor of interest. Although there are several approaches to operationalize this quantity, one common definition entails division of the attributable risk by the incidence measure for an outcome among those exposed to the risk factor of interest, and then the quotient is multiplied by a constant (Table 21.1). A frequent interpretation of this value is that it represents the proportion of outcomes, among the exposed, that would be eliminated from the study population should the risk factor of interest be removed. For the earlier hypothetical example, the attributable fraction for Cd and pregnancies suggests that decreasing Cd exposure would decrease the number of infertile women with high Cd by 45%. Under some circumstances, such as the methodologic constraints introduced by the case–control design (to be described later in this chapter) or to simplify a statistical analysis, the odds of a study outcome may be estimated. Odds are not a proportion or a rate, but rather represents the ratio for the probability that an outcome occurred to the probability that the outcome did not occur (Table 21.1). In the earlier hypothetical example the odds for infertility among women with high blood Cd is 0.22, whereas the analogous odds for women with low blood Cd is 0.11. The odds ratio characterizes the odds for an outcome among the group exposed to a risk factor of interest relative to the odds for the outcome among a group unexposed. Odds ratios are interpreted in a fashion analogous to rate ratios, with their meaning contingent on the design of the epidemiologic study from which the data emerge (i.e., there are prevalence odds ratios, incidence odds ratios, and exposure odds ratios). Under a limited set of circumstances, principally that the outcome under study is rare in the study population from which the study sample was recruited (i.e., <10%), the odds ratio for an outcome reflects the RR for that outcome. However, with increasing prevalence of the outcome in the study population, the odds ratio increasingly overestimates the RR. For example, in the hypothetical study described earlier, the odds ratio for infertility among women with high blood Cd is 2.0, which modestly overestimates the aforementioned RR equal to 1.82. However, the prevalence of infertility is 14.1 cases per 100 women in the hypothetical study population (i.e., 14%). Given that complete ascertainment of a study population or census is frequently infeasible and impractical, epidemiologists employ sampling in which a smaller group from the population is studied, and the results are then generalized to the greater whole. Ideally, an investigator aspires to recruit a study sample that reflects the distribution of risk factors, study outcomes, and other important factors in the source study population; however, this can be quite challenging. Failure to recruit a sample representative of the study population limits the generalizability of study results; the investigator is unable to extrapolate the results to the study population leading to limited external validity. For example, in Figure 21.2, three study samples of n = 200 are taken from a hypothetical study population of 1000 people. Study sample “a” reflects the population in terms of appearance and age distribution; there is no sampling error. However, study samples “b” and “c” appear different from the study population as reflected in their disparate age structures; there is substantial sampling error. When a sampling error occurs in a nondifferential fashion, meaning it does not vary by the risk factor or outcome of interest or by any other important study variable, it is defined as random. Should sampling error occurs in a differential fashion, meaning it varies by the risk factor of interest, outcome of interest, or another important study factor, it is referred to as biased; this type of systematic error undermines the internal validity of a study (i.e., the results generated in the study sample). Internal validity is of primary concern as this is a prerequisite to external validity; vis-à-vis, the results in the study sample must first be valid to extrapolate these to the study population. While preventable through appropriate study techniques, bias is insidious and difficult to assess and quantify, and once introduced, it is difficult if not impossible to eliminate. Epidemiologic bias will be discussed in greater detail later in this chapter. Figure 21.2 Selection of three study samples from a hypothetical population of 1000 persons. All study results are associated with error, including incidence rates, rate ratios, and other epidemiologic metrics. Epidemiologists will frequently assess study results for statistical significance in one fashion or the other; inherently, this quantifies the role that random error (i.e., chance) plays in a study result. Under limited conditions, hypothesis testing offers the investigator guidance as to the role played by random sampling error in the observed study results. The type 1 error, or “α,” describes the probability for detecting an association in the study sample that does not exist in the study population (i.e., a false-positive result due to sampling error). By convention, type 1 error is often set at a threshold of 5%, meaning that a result likely to occur randomly in less than 1 of 20 studies is considered valid. However, many other approaches to quantifying random error are also employed. Often, epidemiologists quantify the random error associated with a study result using P-values (i.e., using thresholds such as p < 0.05) or confidence intervals (i.e., such as 95% confidence intervals). A study sample must also be of sufficient size, given the hypothesis, to find an association if one does indeed exist in the study population. This potential is referred to as statistical power, assessed as 1 − β. The quantity “β” is referred to as the type 2 error, and it describes the probability for not detecting an association in the study sample, which does exist in the study population (i.e., a false-negative result due to sampling error). Type 1 and type 2 errors are inversely related in that increases in the type 1 error (i.e., corresponding to decreasing specificity) are associated with decreases in the type 2 error (i.e., corresponding to greater sensitivity), and vice versa. By convention, at least 80% power is required for a study to be considered sufficiently precise, as power is inversely associated with random error (i.e., greater statistical noise inhibits an investigator’s ability to detect a true signal). Thus, it behooves an investigator to reduce random error whenever possible. However, studies are frequently conducted with power less than 80%, substantially so in many cases. For a thorough treatment of these issues, the reader is referred to the additional sources listed at the end of this chapter. The design of observational studies accounts for much of the discipline of epidemiology, with specific adaptations particular to various subdisciplines including environmental and occupational epidemiology. An epidemiologic study generally proceeds in three stages: (i) the design stage, (ii) the implementation stage, and (iii) the analysis and reporting stage. It is important for toxicologists to be involved in every stage of an epidemiologic study to provide the insight and expertise from that discipline. In the design stage, a study hypothesis is refined and developed as the specificity and nature of the question should govern the remainder of the design process; the hypothesis serves as the anchor for an epidemiologic study. In the design stage, a study population is identified and a strategy to recruit a representative and unbiased study sample is prepared; this step will facilitate the success or failure of an investigation. In the implementation stage of an epidemiologic study, participants are recruited and data and possibly biologic specimens are collected and analyzed; this phase may be of limited duration such as under a cross-sectional study design, or it may take many years such as under a prospective cohort study design. Randomized controlled trials, though epidemiologic in nature, are rarely encountered in the investigation of exposure to environmental and occupational risk factors. This quasiexperimental study design is frequently used to investigate pharmaceutical treatments, medical procedures, medical devices, or other health interventions and so is not addressed in this chapter. The features, strengths, and limitations of the most commonly employed study designs in environmental and occupational epidemiology are summarized in Table 21.2. Table 21.2 Common Epidemiologic Study Designs Many of the obstacles to successful study implementation can be prevented by meticulous planning during the design stage. The decisions made in the design stage of a study are frequently indelible once a study moves into the implementation stage. Biases introduced deliberately or inadvertently may be impossible to eliminate during the analysis phase of the study, if not prevented or if appropriate and sufficient data are not collected. There are of course some issues to which the investigators may adapt during the course of implementation. However, these may present additional challenges, as the introduction of differential participant recruitment strategies or data collection methodologies during the implementation stage may invalidate previously recruited participants or previously collected data. Very careful attention must be paid by the investigators to the myriad nuances of subject recruitment and data collection a priori. In the analysis and reporting stage, data are cleaned, statistical analysis is conducted, and the results are reported in oral and written modalities as conference presentations, peer-reviewed publications, and technical reports. During the analysis and reporting stage, the investigator will have the opportunity to adjust or control for certain biases due to confounding contingent on collection of the requisite data (discussed in detail later in this chapter), to evaluate and on occasion adjust or control other sources of bias, and even to conduct additional hypothesis testing in the so-called secondary studies. Here, we briefly summarize the most salient components, limitations, and strengths of the most widely used epidemiologic study designs. The reader is referred to the sources listed at the end of this chapter for a more comprehensive treatment. Epidemiologic studies are traditionally dichotomized as descriptive or analytic/inferential. In descriptive studies, investigators seek to characterize an outcome of interest in terms of factors related to person, place, and time as described earlier in this chapter. These studies generally provide data concerning the frequency and rates of outcomes and exposure to risk factors of interest; these data are invaluable for hypothesis generation as well as for surveillance, policy development, and program planning and evaluation. Case studies, case series, and surveillance studies are types of descriptive studies. Analytic/inferential studies focus on statistical tests of etiologic hypotheses with the ultimate goal of inferring causal associations. Analytic/inferential epidemiologic study designs are defined in terms of the strategy employed to recruit study participants and the unit of analysis used. Most analytic studies include a descriptive component. Two general analytic/inferential strategies are implemented as defined by the analysis at the group level or the individual level. In group-level or ecological study designs, data are averaged over groups of individuals that serve as the unit of analysis. Groups are often fixed at a single point in time and vary geographically, or alternately groups may be fixed geographically and vary temporally. Under a traditional spatial ecologic design, the incidence or prevalence of an outcome is compared across different geographic units that have different distributions for a risk factor of interest; attempts are frequently made to ensure that groups are otherwise similar but this may prove challenging. For example, studies report higher rates of lung cancer in regions with very high concentrations of inorganic As contaminating ground drinking water sources than in regions without such contamination. Ecologic studies are often feasible using existing data sources, so these may be more convenient and less expensive than study designs requiring individual-level data, which is typically more resource intensive. However, the results generated by ecologic studies are highly limited and are considered useful primarily for hypothesis generation but not for causal inference. Foremost among the limitations of the ecologic approach is the ecologic fallacy; associations between groups may differ substantially from associations between individuals due to differential heterogeneity of within-group study factors. Furthermore, under the spatial ecologic approach, temporality, in which exposure to a risk factor chronologically precedes the outcome of interest, may not be established, thereby introducing possible reverse causality, in which the outcome of interest alters the level of exposure to the risk factor. In addition, adjustment for confounding and consideration of effect measure modification (issues discussed in detail later in this chapter) may be more challenging than when using individual-level data study designs; group-level data for these factors is also subject to the aforementioned ecologic fallacy. Thus, interpretation of study results made at the group level may have no meaning or may have an entirely different meaning at the individual level. However, circumstances in which factors exclusive to groups, such as implementation of a new public policy in a specific legislative district, or in which extrapolation to groups are of primary interest may be uniquely apropos to the spatial ecologic study design. A design related to yet distinct from the spatial ecologic design is the time-series design. This framework is essentially an ecologic design in which risk factors and outcomes are grouped by time interval and are often fixed in space. This approach is very useful to investigate the effects of environmental or occupational exposures that change over time in a single geographic area. Like the traditional ecologic design, time-series studies are vulnerable to ecologic bias and confounding bias. In addition, time-series designs present with several unique challenges including autocorrelation, in which observations across time are associated with one another (i.e., most statistical analyses presume independent study outcomes); latency, in which an outcome associated with exposure to a risk factor during an earlier time interval may manifest during a later time interval; and bias due to time-varying confounders; fairly complex statistical approaches may be required to accommodate these issues. Time-series study designs have been used to great effect for investigating the effects of air pollution on human health outcomes. For example, a substantial increase in mortality rates in London, England, primarily associated with respiratory disease, was documented shortly after the infamous London Fog pollution “Pea Soup” episode of 1952. In that scenario, widespread use of coal for household heating coincided with a temperature inversion trapping airborne pollutants close to the earth’s surface and resulting in widespread respiratory exposure to sulfur oxides and particulates over a limited duration. At the individual level, data are collected from each study participant, and thus, study designs are not vulnerable to the aforementioned ecologic fallacy. There are several individual-level study designs commonly implemented by epidemiologists, and there are many variations on each theme. The simplest, quickest, and usually least resource-intensive individual-level approach is the cross-sectional study design. The cross-sectional design essentially comprises a “snapshot” in time as described by Figure 21.3a. Participants are recruited to the study sample from a study population at a single instance or over a single time interval. Exposure to one or more risk factors of interest and one or more outcomes of interest are assessed simultaneously. Thus, participants are selected without regard to exposure or outcome, and thus, the study sample provides an estimate of prevalence for both in the study population. This study design is among the most frequently found in the environmental and occupational epidemiology literature. Figure 21.3 Common epidemiologic study designs: (a) cross-sectional design, (b) cohort design, and (c) case–control design. It is important to note that the cross-sectional approach captures only prevalent cases; it is also referred to as a prevalence study. As a consequence, associations between exposure to risk factors and outcomes may be a consequence of association with the duration of the outcome rather than with the incidence of the outcome, as discussed earlier in this chapter. Moreover, this approach precludes assessment of temporality, and thus, the cross-sectional design is vulnerable to the earlier discussed reverse causality. Cross-sectional studies provide prevalence rate ratios or odds ratios to characterize associations between one or more risk factors and one or more study outcomes. For example, an investigation that simultaneously measures PCBs, dioxins, and thyroid hormones in the blood of study participants, at a single point in time, and then evaluates these data for statistical associations between the PCBs and dioxins with thyroid hormones is cross-sectional in nature. Not infrequently, an investigator may target recruitment of subjects likely to have higher or lower exposures or subjects more likely or less likely to have experienced an outcome of interest in order to increase the available statistical power (i.e., greater variability in exposure to risk factors generally increases statistical power). In the aforementioned cross-sectional investigation of PCBs and dioxins and thyroid function, an investigator might recruit individuals likely to have high and low rates of Great Lakes sport fish consumption, such as licensed anglers living in close proximity to the Lake Ontario, to ensure a wide distribution of exposure to the risk factors (lipophilic compounds including PCBs and dioxins are known to concentrate in the fat of Great Lakes sport fish). Table 21.3 describes a generic cross-sectional study of a single risk factor and study outcome of interest using a 2 × 2 table, or contingency table. Study participants are classified according to exposure status and then distributed across columns according to whether or not the outcome of interest occurred. These data can be employed to calculate prevalence proportions or odds and ratios described in Table 21.1. Table 21.3 Distribution of Participants in Epidemiologic Studies: Cross-Sectional (Prevalence Data) or Cohort (Incidence Data) Study Designs Outcome proportion or risk in the exposed, A/(A + B); outcome proportion or risk in the unexposed, C/(C + D); prevalence proportion ratio or relative risk, A/(A + B)/C/(C + D) Individual-level epidemiologic studies more complex than the cross-sectional design incorporate temporality, although these usually require a greater investment in time and resources than the cross-sectional approach. In a cohort study, a sample of persons not having experienced the outcome of interest (i.e., a “cohort”) is followed over time for occurrence of that outcome as described in Figure 21.3b. A group of participants exposed to the risk factor of interest is followed as is a comparable group that is unexposed to the risk factor of interest, a referent group. At the end of a defined study period, or period of follow-up, incidence measures for outcomes in the exposed group are compared to the unexposed group. A cohort comprised of participants not having experienced the study outcome and exposed to a risk factor, as well as participants not having experienced the study outcome and unexposed to a risk factor, can be identified at baseline and then followed prospectively through time until occurrence of the study outcome or until the end of the follow-up period. This approach can often be enormously time-consuming contingent on the latency for the outcome of interest, such as a solid tumor that may take 20 years or longer to develop, and thus can be prohibitively expensive. Alternately, persons may be identified as exposed or unexposed to a risk factor of interest retrospectively, using preexisting records or archived biologic specimens collected in the past, and figuratively followed up for the study outcome of interest or the end of the study period, having occurred in the past or occurring in the present. Occupational or medical insurance records can often facilitate this process assuming the availability of accurate and unbiased medical records, and this approach is frequently used for occupational epidemiologic investigations. Unlike the aforementioned cross-sectional design, the cohort design can provide valid risk estimates as only incident outcomes are captured; the incidence in the referent group (unexposed) characterizes the background risk for an outcome in the study population. These data are then used to generate RR, risk or rate ratios, or attributable risks to characterize the association between a risk factor and one or more study outcomes. The cohort study design is very useful when investigating exposure to rare or infrequently encountered risk factors as exposed individuals are targeted for recruitment. However, this approach also limits the number of risk factors that can be studied, as these must be decided a priori and a group recruited for each risk factor of interest, or combination thereof. In contrast, the cohort study affords an investigator the opportunity to assess multiple study outcomes contingent on the size of the study sample; rare outcomes may not manifest in sufficient number to facilitate statistical analysis unless the cohort is unusually large. The latter caveat makes the cohort design less useful for the study of rare outcomes. Under some circumstances, one or more participants may leave or drop out of a cohort study prior to experiencing a study outcome and before the end of the study, and so follow-up must be terminated or censored at that point; this is referred to as right censored. Conversely, follow-up for one or more participants may be initiated after a study has begun, and thus, follow-up prior to enrollment in the cohort must be censored for a subject at that point; this is referred to as left censored. When censoring of follow-up takes place, there will be heterogeneity in the time individuals in the study sample are at risk for an outcome. In this scenario, epidemiologists often employ survival analysis approaches to analyze data, as these utilize conditional probabilities to accommodate the variability in time at risk for individuals in the study. The reader is referred to the sources listed at the end of this chapter for additional information. If censoring is related to the study outcome of interest, then a selection bias may be introduced into a cohort study (discussed later in this chapter). As with all epidemiologic study designs, cohort studies are susceptible to confounding bias (discussed later in this chapter). Cohort studies are used to generate various ratios, which as discussed are used to evaluate the effect of a risk factor on an outcome of interest. For example, the Harvard Six Cities Study, a prospective cohort investigation of air pollution and mortality, detected increased risks for respiratory and cardiovascular-related mortality in association with long-term exposure to higher ambient concentrations of fine airborne particulates. Table 21.3 describes a generic cohort study of a single risk factor and study outcome of interest using a contingency table. Study participants are classified according to exposure status, with row margins fixed by the investigator (i.e., the number of participants exposed to the risk factor and the number of participants unexposed to the risk factor, or referents), and then distributed across columns according to whether or not the outcome of interest occurred. These data can be employed to calculate outcome risks and subsequently the RR as described in Table 21.1. In contrast to the epidemiologic cohort study design, which may be implemented in a prospective or retrospective fashion, the case–control study design is strictly retrospective (it is not infrequently referred to as a retrospective study design). The apparent simplicity of the case–control design belies its true complexity, a comprehensive treatment of which is beyond the scope of the current chapter but which is available in the sources listed at the end of this chapter. The case–control study design is quintessentially epidemiologic; it essentially entails strategies for sampling from an underlying cohort so that associations between risk factors and outcomes can be assessed in a less resource and time-intensive manner than that offered by cohort designs. As described by Figure 21.3c, subjects experiencing the outcome of interest in the study population are recruited as cases (all cases in a study population or a random sample thereof), and a sample of individuals not experiencing the outcome at the time of the study are recruited as controls. It is important to note that controls are not necessarily healthy individuals, but are those who have not experienced the outcome or the study event of interest. This study design is very useful for rare outcomes, such as cancers or congenital malformations, which would require a very large cohort to capture a sufficient number of incident outcomes to facilitate statistical analysis. Under the case–control design, the investigator creates in essence a sample with a high proportion of cases irrespective of presence in the study population; thus, the prevalence in the study population cannot be estimated from the study sample. The investigator will choose the number of cases to include as well as one or more controls per case for purposes of comparison. To be valid, recruitment of case and control participants must be independent of their exposure to the risk factors of interest, and controls must represent the exposure experience in the study population (i.e., if the controls had indeed been cases, they would have been captured as such by the study). Otherwise, a selection bias may be introduced into the study. Contingent on the outcome and risk factor of interest, case–control studies are also vulnerable to recall bias and exposure misclassification bias (described later in this chapter). As described by Figure 21.3c, case and control participants are each retrospectively assessed for exposure to a risk factor or risk factors of interest, often through interviews, archived biologic specimens, or previously collected records. Study participants may be recruited using a cumulative incidence sampling strategy in which cases and controls are identified at the end of a defined study period. Under this scenario, a single subject can participate in the study only as a case or as a control. Alternately, participants may be recruited using an incidence density sampling strategy, in which a cohort is followed and incident cases are recruited to the study sample as these occur; controls are then selected from among those individuals in the study population without the outcome at the time of each case. Perhaps counterintuitively, an individual may participate in such a case–control study as both a control and a case. However, statistical analysis of data arising from this type of study requires a specialized approach. Variations on the case–control approach include the nested case–control study design and the case-crossover study design. In a nested case–control study, cases and controls are sampled from the participants in a completed or partially completed cohort study, providing the advantage of complete enumeration (i.e., outcomes are known for all members of the cohort). In some scenarios, costs may be prohibitive, so that only a sample of the cohort can be assessed for an expensive laboratory analysis or for a secondary hypothesis. Nested case–control study participants are “sampled” from the cohort study, which then serves as the study population of interest. In the case-crossover approach, participants with outcomes are identified, and then various reference intervals are sampled for each participant, so that a participant serves as her or his own control. This design presents the advantage of eliminating confounding bias by non-time-varying factors, such as genetic polymorphisms and sex. The case-crossover study design is usually employed to assess the acute effects of transient risk factor exposures; it is frequently employed to evaluate acute human health effects associated with exposure to air pollutants. Case–control studies are used to generate odds ratios, which as discussed earlier provide an unbiased estimate of the underlying study population RR if the outcome is rare. For example, a series of case–control studies conducted by the U.S. National Institute of Occupational Safety and Health (NIOSH) established a link between occupational exposure to vinyl chloride gas and hepatic angiosarcoma, a rare form of liver cancer. Table 21.4 describes a generic case–control study of a single study outcome and risk factor of interest using a contingency table. Participants are classified according to case or control status, with row margins fixed, and then distributed across columns according to the presence (i.e., exposed) or absence (i.e., unexposed) of the risk factor of interest. These data can be employed to calculate exposure odds and subsequently the odds ratio described in Table 21.1. Table 21.4 Distribution of Participants in Epidemiologic Studies: Case–Control Study Design Exposure odds in cases, A/B; exposure odds in controls, C/D; odds ratio, AD/BC. Study bias is defined as a directional deviation of study sample results from the true underlying study population value; vis-à-vis, effect estimates systematically shifted too high or too low. Bias in epidemiologic studies often results from unintentional trends in the collection, analysis, interpretation, publication, or review of data. Bias undermines the aforementioned validity of a study and contingent on manifestation may compromise the earlier described internal and/or external study validities. There are essentially three sources of bias in epidemiologic studies, the manifestations of which are often insidious and frequently specific to a particular study design, including (i) information bias, (ii) selection bias, and (iii) confounding bias. Contingent on study design, information bias and selection bias are often preventable. However, once introduced, an investigator is usually unable to exclude these biases and is limited to characterizing the impact on study results, sometimes using sensitivity analysis or using external information or data to compensate for a known bias. Sensitivity analysis comprises evaluation of changes in study results when subjects, variables, or approaches are excluded or changed to assess quantitatively the effects of those components. In contrast, it is often feasible to correct for confounding bias by statistical adjustment or exclusion. This is subject to the collection of data describing anticipated confounders during the design and implementation phases of the study. Dialogue on these issues between the epidemiologist and toxicologist will determine the quality of the investigation. Information bias results from faulty assessment of exposure or outcome in a fashion that is differential between groups compared; information accuracy differs between exposed and unexposed persons, between cases and noncases, or between both. Types of information bias commonly encountered by epidemiologists include recall bias, interviewer bias, and misclassification bias. Selection bias is produced when study recruitment or participation occur contingent on exposure to the risk factor under the case–control study design, contingent on the outcome under the cohort study design, or in which selection is linked to both under the cross-sectional design. Types of selection bias commonly encountered by epidemiologists include self-selection bias/loss to follow-up bias, nonresponse bias, detection bias, Berkson’s bias, healthy control bias, and collider-stratification bias. Two types of selection bias unique to occupational epidemiology are the healthy worker effect and the unhealthy reproducer effect, which may manifest when occupational groups are compared to the general population. For more detailed explanations and examples, the reader is referred to the sources listed at the end of this chapter. Confounding bias results from a mixing of the association in which the investigator has interest, with one or more associations in which the investigator does not have interest. This scenario is a consequence of the differential distribution of risk factors among people with and without the study outcome, according to factors other than the outcome under consideration. Confounding is a property inherent to a study population, and factors that confound associations of interest can be identified as those that are common causes of both the risk factor and the outcome of interest. Though not sufficient to cause confounding, a confounding variable necessarily (i) has a causal association with the risk factor of interest in the study population, (ii) has a causal association with the outcome of interest independent of the risk factor of interest, and (iii) is not itself affected by the risk factor or the outcome of interest. Confounding bias is a nuisance and investigators aspire to eliminate confounding wherever possible. In contrast to information bias and selection bias, statistical adjustment can frequently reduce or eliminate confounding if the relevant factors are identified during study design, and data is accurately captured during study implementation. Approaches to eliminate confounding include exclusion, stratification, standardization, matching, and multivariable analysis, among others. The reader is referred to the sources listed at the end of this chapter for a detailed discussion of these approaches. When confounding is incompletely addressed, residual confounding will continue to bias study results. Age, sex, race and ethnicity, body mass index, socioeconomic status, cigarette smoking, and alcohol consumption frequently merit attention as confounders in environmental and occupational epidemiologic studies. For the purposes of illustration, Figure 21.4 demonstrates a hypothetical example of cigarette smoking as a confounder of an association between carrying a lighter and lung cancer. In Figure 21.4a, an RR of 10 suggests a 10-fold increase in risk for lung cancer among persons who carry a lighter in their pocket relative to those who do not. However, after adjustment for “cigarette smoking,” which is a common cause of “carry lighter” and “lung cancer” in Figure 21.4b, the aforementioned risk disappears as indicated by RR = 1 for the association between “cigarette smoking” and “carry lighter.” In this scenario, the association between “cigarette smoking” and “lung cancer” was mixed with the association for “cigarette smoking” and “carry lighter,” resulting in confounding. Figure 21.4 Example of confounding bias: (a) causal pathway spuriously suggesting that “carry lighter” is associated with a 10-fold increase in “lung cancer” and (b) causal pathway demonstrating confounding of “a” by “cigarette smoking.” In contrast to bias, which is a nuisance to be prevented and eliminated if possible, effect modification is of interest and cannot be eliminated from a study. Effect modification reflects the biology or other critical component of a risk factor–outcome association. In fact, ignorance of effect modification will counterproductively introduce bias into study results. Effect modification occurs when the association between two or more factors varies by one or more additional factors. An investigator will generally suspect effect modification a priori based on scientific literature, presumed causal pathways between the risk factor and study outcome, or on biology. As a hypothetical example, exposure to an environmental chemical agent might interfere with reproductive success in Asian women and men, yet not in non-Asian women and men; so race is an effect modifier of the agent–reproduction association in this scenario. Sex, age, genetic polymorphisms or markers thereof such as race and ethnicity, and socioeconomic status are frequently encountered as effect modifiers in environmental and occupational epidemiologic studies. The reader is referred to the sources listed at the end of this chapter for further detail. Exposure entails contact between a person and a risk factor, which is a multifactorial and dynamic process governed by fate–transport processes and toxicokinetics. The goal of an epidemiologic exposure assessment is to delineate groups with various degrees of contact with a risk factor of interest. When the risk factor under consideration in an epidemiologic study is a hazardous or potentially hazardous agent, the exposure analysis represents a critical link for the evaluation of associations with an outcome of interest. Invalid or imprecise exposure assessment introduces information bias and random error into epidemiologic studies. When such error is differential between individuals with a study outcome and those without, internal study validity is compromised. Nondifferential exposure assessment error undermines study power. When exposure in the study sample is not representative of the study population, external validity is compromised. In addition, humans are exposed simultaneously to numerous risk factors in the environment and in the workplace, the effects of which may be additive, synergistic, or antagonistic, and so consideration of exposures to mixtures of risk factors is of increasing concern. The complete mix of all risk factors to which an individual is exposed has been recently defined as the exposome. So challenging is the issue of exposure assessment that it is often referred to as the “Achilles heel” of environmental and occupational epidemiology. As demonstrated by Figure 21.5, there are many factors to consider when assessing exposure, which modify the association between an environmental or occupational risk factor and a study outcome from the point of agent release until an incident outcome. Once released from the source, a risk factor or chemical agent in the environment or the workplace is subjected to processes that will affect redistribution in the environment including transport by air, water, soil, climatic factors, and geographic factors, among others, the so-called fate and transport. Agents are deposited in the environment or in the workplace, and contingent on their proximity to persons and their source of deposition, these may come into contact with humans, comprising an exposure. Exposure is a function of (i) the magnitude or the concentration of an agent with which an individual comes into contact (this is often defined in terms of the average exposure over time, the cumulative or total amount of agent over time, or the peak or highest concentration exposure received); (ii) the frequency of contact with an agent; (iii) the duration of contact with an agent, which may be acute (brief), subchronic (over days or weeks), or chronic (over months or years); and (iv) the route of exposure (inhalation, ingestion, dermal absorption, or injection). Figure 21.5 Mechanistic basis for the sequence of events leading to environmental-related illness or injury. Source: Adapted from Sexton et al. (1992). © 1992 Taylor & Francis.
EPIDEMIOLOGICAL ISSUES IN OCCUPATIONAL AND ENVIRONMENTAL HEALTH
21.1 ENVIRONMENTAL AND OCCUPATIONAL EPIDEMIOLOGIC STUDIES
21.2 WHAT EPIDEMIOLOGY IS
Environmental Epidemiology
Occupational Epidemiology
21.3 EPIDEMIOLOGIC METRICS AND MEASURES OF ASSOCIATION
Metric
Use
Formula
Cumulative incidence proportion
Describe the risk for an outcome across a time interval
Incidence density rate
Describe the rate for an outcome during a time interval
Point prevalence proportion
Describe the number of existing outcomes (old and new) at a single time
Period prevalence proportion
Describe the number of existing outcomes (old and new) across a time interval
Relative risk
Describe the relative cumulative risk for an outcome across a time interval between exposed and unexposed groups
Rate ratio
Describe the ratio of outcome rates during a time interval between exposed and unexposed groups
Hazard ratio
Describe the relative instantaneous risk for an outcome between exposed and unexposed groups
Prevalence proportion ratio
Describe the relative prevalence proportion for an outcome between exposed and unexposed groups
Attributable risk
Describe the additional number of outcomes associated with exposure to a risk factor
Incidence rateExposed to a risk factor − incidence rateUnxposed to a risk factor
Attributable fraction
Describes the proportion of outcomes in the exposed group, associated with exposure to a risk factor
Odds
Estimate the odds for an outcome relative to no outcome
Odds ratio
Estimate the underlying population relative risk using the ratio of odds for an outcome between exposed and unexposed groups
Incidence
Prevalence
Ratios
Attributable Risks
Odds
Random Error in Epidemiologic Studies
21.4 EPIDEMIOLOGIC STUDY DESIGNS
Design
Basic Features
Strengths
Limitations
Descriptive studies
Count outcomes in terms of person, place, and time
Provide data for public health policy development, program planning, and budget allocation; useful for generating hypotheses for future study
Unable to assess associations between risk factors and outcomes or to provide information to infer causality
Ecologic studies
Compare rates for outcomes at the group level of measurement; a group exposed to a risk factor is compared to a group unexposed to a risk factor; groups are defined in terms of geographic space or time
Usually less resource intensive than other designs, often can make use of readily available data collected as part of descriptive studies, useful to infer causality at the group level, useful for hypothesis generation at the individual level
Results valid only at the group level of measurement (vulnerable to the ecologic fallacy), difficult to address confounding at the individual level of measurement, unable to assess temporality in some approaches and thus vulnerable to reverse causation
Cross-sectional
A “snapshot” in time at the individual level of measurement; exposure to a risk factor is assessed simultaneously with outcomes of interest
Usually less resource intensive than other study designs, uses individual level data, and permits simultaneous assessment of multiple risk factors and outcomes; useful for hypothesis generation
Captures prevalent outcomes and so cannot directly estimate risk, unable to assess temporality and thus vulnerable to reverse causation, vulnerable to recall bias
Case–control
Compares individuals with a study outcome (cases) to those without (controls) and retrospectively assesses exposure to risk factors
Very useful for rare study outcomes; permits simultaneous assessment of multiple risk factors, uses individual-level data, and incorporates temporality between risk factor and study outcome; often less resource intensive than cohort studies
Usually limited to a single study outcome; control participants can be difficult to identify and recruit; vulnerable to selection bias and recall bias; retrospective exposure assessment is vulnerable to exposure measurement misclassification bias; estimates population relative risk only when the study outcome is rare or using a more complex incidence density sampling strategy
Cohort
Among individuals free from a study outcome, compares a group exposed to a risk factor of interest to a group unexposed to a risk factor of interest and follows through time for occurrence of study outcomes
Provides risk estimates and unbiased estimates for population ratios, permits simultaneous assessment of multiple study outcomes, and incorporates temporality between a risk factor and study outcome; prospective exposure assessment
Usually limited to one or a few risk factors of interest; study dropouts (loss to follow-up) may introduce selection bias; rare study outcomes may require very large study samples to facilitate statistical analysis; study outcomes with long latency periods may require long periods of follow-up; often highly resource intensive and time-consuming
Group-Level Epidemiologic Study Designs: Ecologic Studies
Individual-Level Epidemiologic Study Designs: Cross-Sectional Studies
Outcome (Cases)
No Outcome
Total
Exposed to risk factor
A
B
A + B
Unexposed to risk factor (referents)
C
D
C + D
A + C
B + D
A + B + C + D
Individual-Level Epidemiologic Study Designs: Cohort Studies
Individual-Level Epidemiologic Study Designs: Case–Control Studies
Exposed to Risk Factor
Unexposed to Risk Factor
Total
Outcome (cases)
A
B
A + B
No outcome (controls)
C
D
C + D
A + C
B + D
A + B + C + D
Bias in Epidemiologic Studies
Effect Modification in Epidemiologic Studies
21.5 EPIDEMIOLOGIC EXPOSURE ASSESSMENT
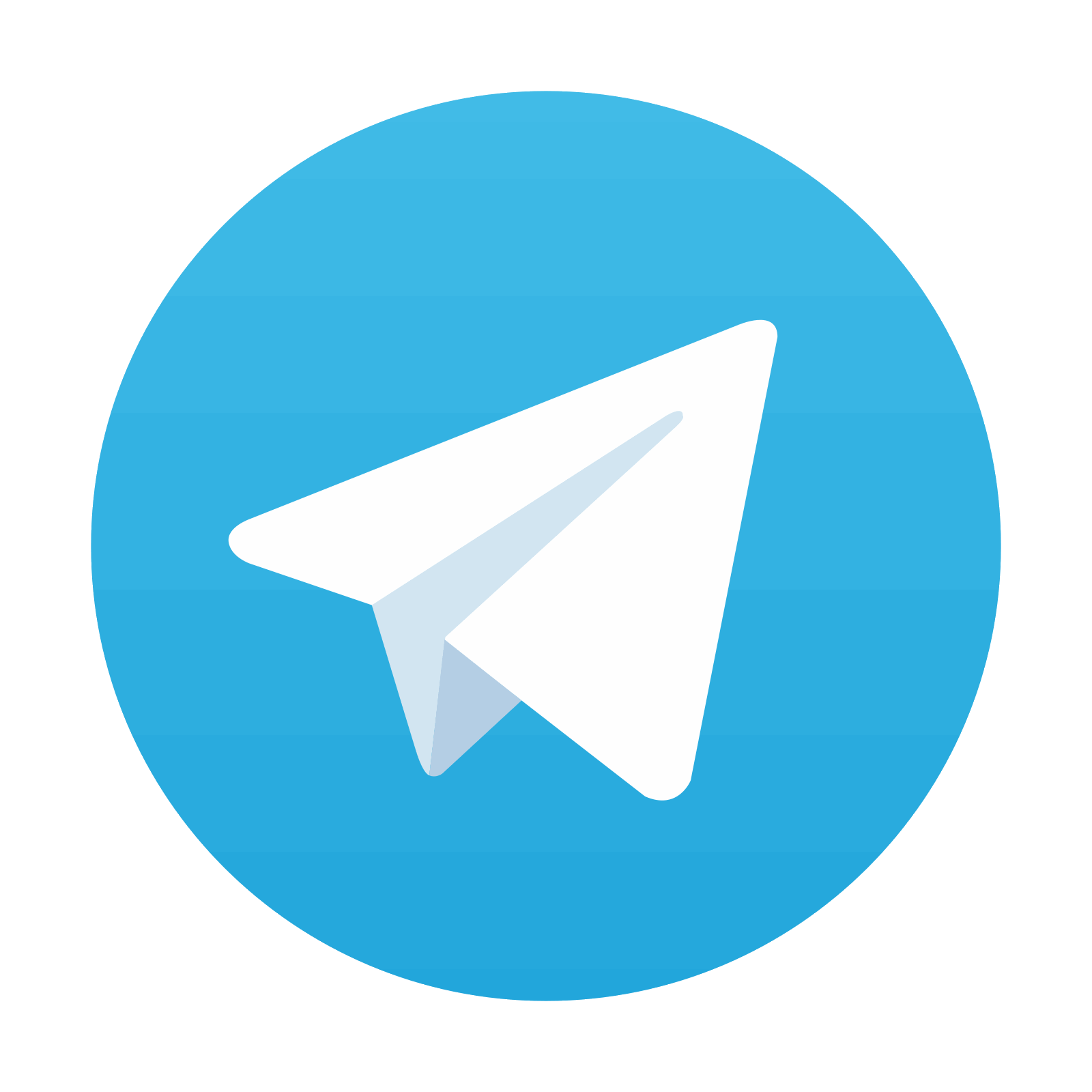
Stay updated, free articles. Join our Telegram channel

Full access? Get Clinical Tree
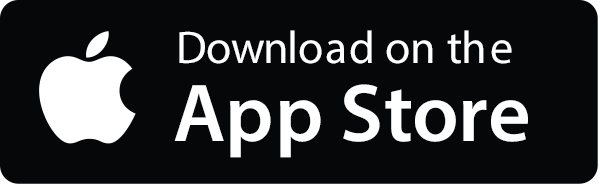
