3.1 Measures of disease frequency
Background
Measures of disease frequency quantify how often a disease or condition occurs within a given population. Thus, measures of disease frequency are also called measures of occurrence.
The three main measures of disease frequency are:
- incidence proportion (risk)
- incidence rate (incidence density)
- prevalence.
All three of these measures of disease frequency are types of ratios consisting of a numerator and denominator. The numerator of each measure of disease frequency is some type of count of cases. The denominator is a measure of population size or “person-time.” As you learn each of these measures of disease frequency, pay careful attention to the similarities and differences in their numerators and denominators.
An important consideration when studying disease frequency is whether the population being studied is closed or opened.
Incidence proportion (risk)
The incidence proportion (cumulative incidence, average risk) of a disease is a longitudinal measure of disease occurrence in which the numerator consists of the number of disease onsets that occurred during the period of observation and the denominator consists of the number of individuals at risk in the closed population as of the beginning of follow-up:
3.1
Note that incidence proportions can only be measured in cohorts, and cannot be calculated in open populations. Also note that the denominator includes only individuals at risk of developing the condition being studied and therefore excludes individuals who are not capable of developing the condition under consideration. For example, in studying uterine cancer, the denominator excludes women who had already experienced uterine cancer, women with prior hysterectomies, and (of course) men, because these individuals are not capable of developing the condition being studied.
Interpretation: An incidence proportion is the average risk of developing the condition under consideration for the period of observation. Therefore, the terms incidence proportion and risk are used interchangeably in epidemiology. In addition, since the incidence proportion represents an accumulation of new cases over time, it is also referred to as cumulative incidence.
To interpret an incidence proportion properly, the length of the time at risk must be specified. In addition, characteristics of the population should be made clear. Consider, for example, the incidence proportion (risk) of breast cancer in American women. The lifetime risk for this outcome is 12% (1 in 8). In contrast, the risk in women between the ages of 60 and 69 is 3.5% (1 in 29). Finally, the risk between ages 50 and 59 is 2.4% (1 in 42). Our understanding of population characteristics and the length of follow-up should temper our interpretation of an incidence proportion.
Figure 3.4 represents the experience of a cohort consisting of five people followed for up to ten years. Each horizontal line in the schematic represents the experience of an individual. Two disease onsets occurred during the ten years of follow-up. Therefore, the ten-year incidence proportion = =0.40 or 40%.a
Let us consider a study that recruits 1000 women for a study of uterine cancer. Upon initial examination, the investigators discover that 100 of the potential study subjects had either already experienced uterine cancer or had a prior hysterectomy. This leaves 900 individuals at risk for uterine cancer. This cohort is followed for five years during which time 45 study subjects develop the disease. Thus, the five-year incidence proportion (risk) of uterine cancer = = 0.05 or 5%.
Incidence rate (incidence density)
The incidence rate (incidence density) of a disease is the number of disease onsets divided by the sum of person-time in the population:
3.2
A person-time unit is the amount of time a person is observed during the study. One person observed for one year contributes one person-year to the denominator. One person observed for two years accounts for two person-years. Two people observed for one year each also accounts for two person-years (and so on). Note that person-time is counted only when a person is at risk of being detected as a case. Person-time is no longer counted after: (a) the person develops the disease under investigation, (b) the person withdraws from the study, or (c) the study ends.
Interpretation: We may interpret incidence rates in several compatible ways. Firstly, incidence rates represent the speed, rapidity, density or intensity at which populations are expected to generate cases. For example, a rate of 5 per 100 person-years is expected, on the average, to generate 5 cases in 100 people followed for one year.
Secondly, incidence rates reflect the incidence proportion (risk) of the disease when the disease is “rare”b according to the formula: . For example, a rate of 1 per 100 person-years over a one-year period corresponds to a one-year risk
Thirdly, the incidence rate in a population is related to its survival experience. Figure 3.5, which is an adaptation of Figure 3.4, is intended to give the reader insight into this relationship. Note that the area under the curve in this diagram is equivalent to the person-time in the cohort. (See Chapter 17 for additional information about the relationship between the probability of survival and rates of occurrence.)
Let us reconsider the data in Figure 3.4. In this schematic, person 1 contributes 2 person-years at risk, person 2 contributes 7 person-years at risk, and persons 3, 4, and 5 contribute 10 person-years at risk each. Thus, the sum of person-time in the cohort = 2 + 7 + 10 + 10 + 10 = 39 person-years. Two incidents of disease occur during the period of observation. Therefore, the incidence rate = per year or, equivalently, 5.13 per 100 person-years.c
When information is not available on individual follow-up time in a cohort, we can estimate the person-time in the cohort with this equation:
In Illustrative Example 3.2 we considered a cohort of 900 individuals at risk followed for 5 years each, during which time 45 incident cases emerged. Using Equation (3.3), Σ Person-time ≈ (no. of individuals at risk) × (duration of follow-up) = 900 persons × 5 years = 4500 person-years. Thus, the incidence rate ≈ = 0.010 0 per person-year or 1.00 per 100 person-years.
Actuarial adjustment: Note that the 45 individuals in this example that developed disease did so at some time during the 5 years of follow-up. Therefore, most did not contribute the full 5 person-years at risk. (After developing the condition they are no longer at risk.) We can adjust for this phenomenon by assuming that the average time of onset of disease was half-way through the follow-up period. Thus, we assume each case contributed half of the 5 years at risk, or 2.5 years each. This method is called the actuarial adjustment. Therefore, a slightly more accurate estimate for the incidence rate in this cohort is = 0.0103 per person-year or 1.03 per 100 person-years.d Notice that this adjustment did not have a large effect because the incidence of the outcome is relatively modest.
Up until this point we have considered rates only in closed populations (cohorts). Rates can also be estimated in open populations with this formula:
3.4
In open populations that are rapidly increasing or decreasing in size, it is common to use the population size mid-way through the period of observation as an estimate of the average population size.
In 2006, the United States had a mid-year population size of 299 398 000 residents. There were 2 426 264 deaths in that year. Therefore, the mortality rate = = 0.008 104 year−1 or 810.4 per 100 000 person-years.
In 2007, there were 2 423 712 deaths in the United States. The mid-year population size was estimated at 301 621 000. Thus, the mortality rate in 2007 was = 0.008 036 year−1 = 803.6 per 100 000 person-years.
We need not limit ourselves to one-year periods of observation. For example, the two years 2006 and 2007 had an average population of (299 398 000 + 301 621 000)/2 = 300 509 500. There were (2 426 264 + 2 423 712) = 4 849 976 deaths over these two years. Therefore, the mortality rate for 2006 and 2007 combined is = 0.008 070 = 807.0 per 100 000 person-years.
The concept of a rate can be flexibly applied to a variety of other “risk-units,” as demonstrated in Illustrative Example 3.6.
In 1994 there were 40 100 automobile-related fatalities and 2297 billion passenger-miles traveled in automobiles. Thus, the fatality rate associated with automobile travel in 1994 was = 17.5 fatalities per billion miles traveled.
Prevalence
Prevalence (prevalence proportion, point prevalence) refers to the proportion of individuals in a population that have a disease or condition at a specific point in time:
3.5
The numerator of a prevalence calculation includes all individuals with the condition under consideration regardless of when the disease commenced. The denominator is the total number of individuals under consideration. Prevalence can be calculated in both opened and closed populations.
Interpretation: Prevalence simply refers to the proportion of individuals in the population currently with a disease or condition. When based on a simple random sample from a population, the prevalence is an estimate of the probability that an individual currently has the condition in question.
A simple random sample of 1000 individuals from a population demonstrates 52 diabetics and 948 non-diabetics. Therefore, the prevalence of diabetes is = 0.052 or 5.2%.
Some epidemiologic sources consider a form of prevalence know as the period prevalence, which is
3.6
During the course of a semester, 23 of the 58 students in a class experienced at least one upper respiratory infection. Thus, the period prevalence of upper respiratory infections was or 39.7%.
Note: Period prevalences reflect some characteristics of incidence and some of prevalence. Therefore, some authorities recommend that we avoid use of the period prevalence and report separate incidence and point prevalence estimates instead (Elandt-Johnson and Johnson, 1980 p. 32).
The prevalence of a disease in a population depends on the rate of inflow of cases into the population and outflow of cases from the population. Inflow is determined by the incidence rate of the disease in the population and the immigration into the population of people who already have the disease. Outflow is determined by the rate of resolution either through recovery or death, and also by the emigration of cases from the population.
To understand the dynamics of prevalence, imagine the fluid level in a basin in which water flows in through incidence and drains out through death (Figure 3.6). The level of water in the basin represents the prevalence of the condition. Note that the prevalence of a condition can increase from either an elevation in incidence or decreases in the death rate. For example, improved survival of HIV/AIDS patients through effective treatment will increase the prevalence of the condition in the population if the incidence of HIV/AIDS remains constant.
Thus, the prevalence of disease is related to the duration of the disease according to this formula: prevalence ≈ (incidence rate) × (average duration of disease).e For example, a disease with an incidence rate of 0.01 year−1 and average duration of ½ year under steady-state conditions has prevalence ≈ 0.01 year−1 × 0.5 year = 0.005.
Comparison of incidence and prevalence: Incidence and prevalence represent distinct measures of disease frequency. Incidence addresses the transition from the disease-free state to the diseased state. In contrast, prevalence addresses current health. Thus, because it is linked to the duration of illness, prevalence is not as well suited as incidence for studying causation. Other differences between incidence and prevalence are summarized in Table 3.1.
Table 3.1 Comparison of incidence and prevalence.
Incidence | Prevalence |
Counts onsets of events only | Counts both “new” and “old” cases |
Independent of mean duration of disease | Depends on mean duration of disease |
Can be measured as a rate or proportion | Always measured as a proportion |
Reflects likelihood of developing disease over time | Reflects likelihood of having disease at point in time |
Preferred measure when studying disease etiology | Preferred measure when studying health services utilization |
3.2 Measures of association
Background
Measures of association in epidemiology are used to quantify the effect of an exposure on an outcome. Therefore, measures of association are also called measures of effect.
In measuring association, we will use the term exposure to denote any explanatory factor thought to increase or decrease the likelihood of the health outcome under consideration. We will also use the term disease to denote any dependent variable or health outcome. For example, we may speak of (a) smoking as an exposure that causes lung cancer, (b) advanced maternal age at pregnancy as an exposure that causes Downs syndrome, (c) high dietary fat as an exposure that causes coronary artery disease, and (d) improved fitness as an exposure that reduces overall mortality. “Exposure” and “disease” are jargon for “explanatory variable” and “response variable,” respectively.
Absolute versus relative comparisons
Measures of association are made by comparing the rate or risk of disease in an exposed group to that of a nonexposed group. Before addressing epidemiologic comparisons, let us review relevant arithmetic principles by comparing the weight of a man who weights 100 kg with the weight of a woman who weighs 50 kg.

Absolute measures of effect
As noted, absolute comparisons are made by subtraction. Thus, the rate or risk difference (RD) quantifies the effect of an exposure in absolute terms according to this formula:
3.7
where R1 represents the risk or rate of disease in the exposed group and R0 represents the risk or rate in the nonexposed group. This formula may also be applied to prevalence “rates,”g in which case it describes a prevalence difference.
Positive RDs indicate the excess rate associated with exposure in absolute terms. Negative RDs indicate the deficit in the rate or risk.
An important historical study found an age-adjusted lung cancer mortality of 104 per 100 000 person-years in doctors who smoked (Doll and Peto, 1976). Doctors who had never smoked had an age-adjusted lung cancer mortality rate of 10 per 100 000 person-years. Therefore, the RD = R1 – R0 = (104 per 100 000 person-years) – (10 per 100 000 person-years) = 94 per 100 000 person-years. Thus, the effect of smoking was to increase lung cancer mortality by producing an additional 94 lung cancer deaths per 100 000 person-years.
Illustrative Example 3.9 demonstrated a positive association between the exposure and disease. Here is an example of a negative association.
A study of physical fitness and overall mortality found that men who improved their physical fitness from the unfit level to the fit level had an age-adjusted death rate of 67.7 per 10 000 person-years (Blair et al., 1995). Men who remained unfit had an age-adjusted death rate of 122.0 per 10 000 person-years. Thus, improved physical fitness was associated with an RD = R1 − R0 = (67.7 per 10 000 person-years) – (122.0 per 10 000 person-years) = − 54.3 per 10 000 person-years. This indicates 54.3 fewer deaths per 10 000 person-years associated with improved fitness.
Relative measures of effect
The rate or risk ratio (RR) quantifies the effect of an exposure in relative terms:
3.8
where R1 once again represents the risk or rate in the exposed group and R0 represents the risk or rate in the nonexposed group. Formally, the ratio of two incidence rates is a rate ratio and the ratio of two incidence proportions is a risk ratio. When this formula is applied to the ratio of two proportions, it results in a prevalence ratio. All of these ratio measures of effect are referred to as relative risks.
Interpretation: The RR quantifies the excess (RRs greater than 1) or deficit (RRs less than 1) in the rate or risk of disease associated with exposure in relative terms. It is literally the risk multiplier associated with exposure. For example, an RR of 2 indicates that the exposure doubles the rate or risk of disease, while an RR of ½ indicates that the exposure cuts the rate or risk in half.
Thus, the RR indicates both the direction and strength of an observed association. RRs greater than 1 indicate a positive association; those less than 1 indicate a negative association. Just as importantly, the further the RR gets from 1, the stronger the association. For example, an RR of 3 indicates a stronger positive association than an RR of 2. Analogously, an RR of 1/3 indicates a stronger negative association than an RR of ½.
A seroprevalence survey performed in the New York State female prison population revealed that 61 of 136 (44.85%) intravenous drug users were HIV positive. In contrast, 27 of 339 (7.96%) of non-users were HIV positive (Smith et al., 1991). Therefore, = 5.63. This indicates that the prevalence of HIV in the exposed (intravenous drug user) group was 5.6 times that of the nonexposed group.
Note: Prevalence ratios will be equivalent to risk ratio when the disease outcome is rare (risk less than or equal 5%), the mean duration of disease among the exposed and nonexposed cases is the same, and developing the disease does not change the exposure status of study subjects.
Here is an example of a negative association expressed as a rate ratio.
Recall the physical fitness and mortality data used in Illustrative example 3.10. The adjusted mortality rate in men who improved their fitness was 67.7 per 10 000 person-years. The mortality rate in those who did not improve their fitness was 122.0 per 10 000 person-years. Therefore, the rate ratio = This negative association indicates that improved fitness was associated with cutting mortality almost in half.
Relative risk difference: The relative risk difference (RRD) is an alternative expression of the RR that is derived by subtracting 1 from the RR: RRD = RR – 1. This statistic expresses the risk difference relative to the baseline risk established by the nonexposed group,h and offers an effective way to explain relative risks to the public. For example, the rate ratio of 0.55 in Illustrative Example 3.12 can now be expressed as RRD = (RR – 1) = 0.45 – 1 = −0.45, indicating a 45% reduction in mortality with improved fitness. The prevalence ratio in Illustrative Example 3.13 can be expressed as RRD = 5.63 – 1 = 4.63, indicating 463% greater prevalence in the intravenous drug user group. This expression is more palatable than the alternative “a prevalence that is 5.63 times that of the non-IV drug users.”
Odds ratios
The odds ratio (OR) provides an alternative measure of relative effect. However, instead of being based on proportions, it is based on odds.
The odds of an event is simply its ratio of “successes” to “failures.” For example, if 1 in 5 people experience an adverse event, the risk of the event is 1 in 5 (20%) but its odds are 1 to 4 (0.25). Odds may be used in place of incidence proportions (risk) and prevalences, but cannot be applied to person-time data where the number of “failures” (non-cases) is not available.
Let us use the notation in Table 3.2 to contemplate ORs. Using this notation, A represents “case” and B represents “non-case,” while the subscript “1” represents “exposed” and subscript “0” represents “nonexposed.” For example, A1 represents the number of exposed cases and A0 represents the number of nonexposed cases.
Table 3.2 Notation for cross-tabulated counts.

Using this notation, the odds of disease in the exposed group is A1/B1 and the odds in the nonexposed group is A0/B0. The ratio of these odds is:
3.9
or equivalently,
3.10
Interpretation: The OR is most often interpreted as if it were an RR. This is because, when the disease outcome is rare, OR ≈ RR. However, the OR is also an effective measure of association in its own right, expressing the relative odds of the outcome in the exposed and nonexposed groups.
Neural tube defects (e.g., spina bifida) are a common type of birth defect affecting approximately 4000 pregnancies annually in the United States (CDC, 1992). Milunsky et al. (1989) examined the relationship between the use of folic acid-containing vitamins around the time of conception and neural tube defects in an HMO population. All the study subjects were undergoing maternal screening. Ten of the 10 713 women who used multivitamins that contained folic acid during the first 6 weeks of pregnancy were reported to have had a baby with a neural tube defect. In comparison, 11 of 3157 pregnancies in women who had not used multivitamins before or after conception had a baby with a neural tube defect. Data are shown in Table 3.3. The OR = indicating a 73% reduction in neural tube defects associated with folic acid containing multivitamins.
Table 3.3 Data for Illustrative Example 3.13, folic acid and neural tube defects (NTD).

Relation between the RR and RD
The risk ratio (RR) and risk difference (RD) describe different aspects of the association between an exposure and disease. As noted earlier, RRs provide relative measures of effect, while RDs provide absolute measures of effect.
Mathematically we note: RD = R1 – R0. Dividing both sides of this equation by R0 derives = RR – 1. Since
, then RD = (RR − 1)R0. Scrutiny of this last expression reveals that the RD is the product of the segment of RR above 1 and the rate in the nonexposed group (R0). Thus, even a large RR can have a modest RD when the disease is rare. In contrast, a small RR can have a large RD when the disease is common.
To see how this plays out in an epidemiologic context, consider the data in Table 3.4. Although the RR for smoking and lung cancer is much greater than the RR for smoking and heart disease (10.4 versus 1.4), the RD for smoking and lung cancer is far smaller than the RD for smoking and heart disease (94 per 100 000 versus 152 per 100 000). This is because heart disease is much more common than lung cancer. Therefore, the modest RR of 1.4 for heart disease greatly increases the number of cases in a population. In contrast, because lung cancer is relatively rare, the large RR associated with smoking translates to fewer additional cases.
Table 3.4 Adjusted mortality rates for lung cancer and ischemic heart disease in smokers and nonsmokers.

3.3 Measures of potential impact
Attributable fraction in the population
The attributable fraction in the population (AFp) is the difference between the current population rate and the rate associated with absence of the risk factor expressed as a fraction of the current population rate. Thus,
3.11
where R (no subscript) represents the rate in the population as a whole and R0 represents the rate in the absence of exposure. This statistic answers the question: “What fraction of the disease burden in the population would potentially be averted with blanket removal of the exposure from the population?”
For example, the rate of lung cancer in the population as a whole (R) is approximately 15.9 per 100 000 person-years, while the rate in nonsmokers (R0) is 3.5 per 100 000 person-years. Therefore, = 0.78, indicating that up to 78% of the lung cancer cases in this population are potentially preventable through the elimination of smoking.
An alternative (equivalent) formula for the AFp is
where RR is the risk ratio associated with the exposure and pe is prevalence of exposure in the population. For example, if 40% of the population smoked (pe) and the RR of lung cancer associated with smoking in this population was 10, then
Formula (3.12) demonstrates that the AFp is a function of the strength of the association expressed as an RR and prevalence of the exposure, pe.
Yet another alternative formula for the population attributable fraction is
3.13
where pc represents the proportion of cases in the population that are classified as exposed and RR represents the risk ratio. This formula is useful when working with case–control data (Chapter 8) in which the OR can substitute for the RR and pc can be determined directly from the case series. Suppose, for example, that 87% of lung cancer cases in a case–control study smoked (pc) and the odds ratio for smoking and lung cancer in this study is 10. Thus, =
= 0.78.
Table 3.5 lists estimates for population attributable fractions for various risk factors and cancer (all forms combined). Although these are only rough estimates, they are useful for indicating where preventive efforts should be focused to achieve the greatest potential reductions in cancer-related incidence and death. Note that interventions directed toward tobacco and diet have the greatest potential impact.
Table 3.5 Population attributable fractions for various types of modifiable risk factors and cancer incidence and mortality.
Risk factor type | Attributable fractionpopulation (%) |
Tobacco | 29–30 |
Dietary | 20–35 |
Occupational | 4–9 |
Reproductive and sexual | 7 |
Sunlight and background radiation | 3–10 |
Pollution | 2 |
Drugs and medical radiation | 1–2 |
Industrial and consumer products | <1 |
Infective processes | 5–10 |
Based on estimates published in Doll and Peto (1981), Miller (1992), Farrow and Thomas (1998), and Brownson et al. (1993).
Table 3.6 lists AFps for lung cancer and selected modifiable risk factors. Notice that the sum of the attributable fractions exceeds 100%. This should come as no surprise since removal of one component cause in a sufficient causal mechanism will prevent disease occurrence (see Section 2.3). Thus, any given case can be prevented in multiple ways.
Table 3.6 Range of population attributable fractions associated with modifiable risk factors for lung cancer, United States.
Risk factor | Attributable fractionpopulation |
Cigarette smoking | 80–90 |
Occupational exposures | 10–20 |
Residential radon | 7–25 |
Low vegetable diet | 0–5 |
Environmental tobacco | 0–2 |
Based on information in Farrow and Thomas (1998), Brownson et al. (1993), Reynolds et al. (1991), and Alberg et al. (2007).
Attributable fraction in exposed cases
The attributable fraction in exposed cases (AFe) is:
where R1 is the rate in the exposed population and R0 is the rate in the nonexposed population. This statistic answers the question: “What fraction of the exposed cases would have been averted if they had not been exposed to the risk factor in question?”
Algebraic manipulation of Formula (3.14) derives this equivalent formula:
3.15
For example, the RR of lung cancer associated with moderate smoking in the United States has been estimated to be 10. Therefore, = 0.90, suggesting that 90% of the lung cancer cases among moderate smokers could have been averted had they not smoked.
Relation between the AFp and AFe: The AFe is the proportion of exposed cases attributable to the risk factor in question. Since no case can be attributed to exposure unless they are exposed, the proportion of cases in the population attributable to the exposure (AFp) is equal to the product of AFe and the proportion of population cases that are exposed to the risk factor in question (pc):
3.16
Suppose for example that a risk factor with an AFe of 0.5 is present in 40% of the cases. Therefore, AFp = AFe × pc = 0.5 × 40% = 20%.
Preventable fraction
The formulas for AFe and AFp do not allow for the calculation of attributable fractions associated with factors that decrease risk. One way to address this limitation is to interchange the definition of “exposure” in the study so that the group denied the beneficial factor is now denoted as “exposed.” This will result in an RR greater than 1, permitting application of the prior formulas.
Alternatively, we may directly calculate the preventable fractions. There are two types of preventable fractions. The preventable fraction in the unexposed is analogous to the attributable fraction in the exposed, and the preventable fraction in the population is analogous to the attributable fraction in the population
The preventable fraction in the unexposed (PFu) is defined as and is easily calculated with this algebraically equivalent formula:
This statistic answers the question: “What proportion of unexposed cases could conceivably be prevented if exposed to the beneficial factor in question?” This is synonymous with the efficacy of the intervention.
The preventable fraction in the population (PFp) is defined as , where R represents the rate in the population as a whole and R1 represents the rate if everyone had been exposed to the beneficial factor. This statistic answers the question: “What proportion of the disease in the population would be averted if the entire population were exposed to the beneficial factor?” An equivalent formula for the preventable fraction in the population is:
3.18
where PFu = 1 −RR (Formula (3.17)) and pcu represents the proportion of cases in the population that are unexposed to the beneficial factor in question.
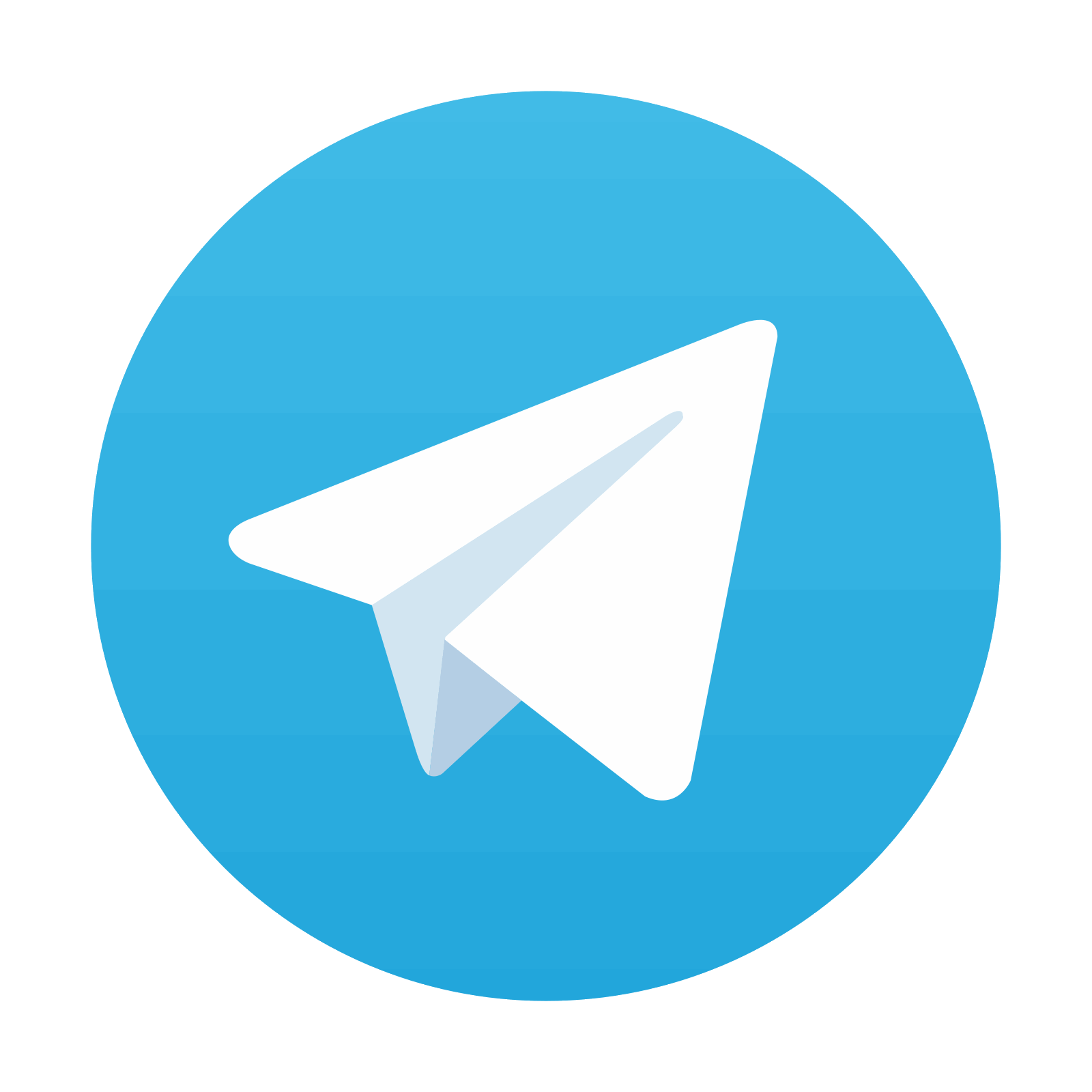
Stay updated, free articles. Join our Telegram channel

Full access? Get Clinical Tree
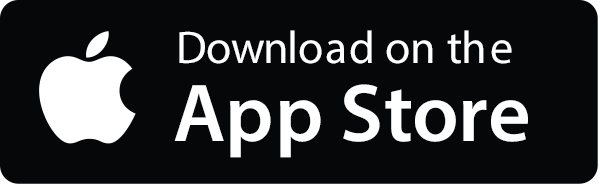
