Acknowledgments
This work was made possible by grants from the National Center for Research Resources (P20 RR016479) and the National Institute of Mental Health (R15 MH077654). The author thanks Dr. Parthasarathi Nag for helpful comments on an earlier version of this chapter.
Introduction
Approaches to treating alcohol dependence are heterogeneous, ranging from group therapy in 12-step programs to pharmacotherapy. Such treatment heterogeneity is a reflection of client heterogeneity that results from the complex biopsychosocial architecture underlying multiple alcoholism types with different developmental trajectories.
Although each treatment approach is successful in reducing the number of drinking episodes and the amount of alcohol consumed per episode, no single treatment approach is superior to the others in all cases. One size does not fit all when it comes to treatment for alcohol dependence. If no single alcoholism treatment is equally effective for all individuals who seek treatment, is there some way to identify those who will respond best to a particular treatment? In other words, is there some way to personalize treatment for alcohol dependence? Although the relevant individual differences among treatment seekers have not been fully elucidated, it is likely that at least some of those relevant individual differences result from genetic variation in mechanisms crucial to etiology or to treatment response. In this sense, alcoholism treatment providers are in the same situation as much of the medical profession in the quest for personalized medicine. In addition, although there is much to anticipate about developments in the area of personalized medicine, progress has not kept pace with the clamor. As interest intensifies in personalized medicine, it seems prudent to consider the ways in which investigators will endeavor to make sense of often conflicting empirical results in an effort to understand complex biological systems across levels of analysis from gene to physiological systems to treatment outcome. In this chapter, an approach is presented that focuses on genetic variation in neurotransmitter systems and utilizes dynamic systems modeling to better understand the contribution of genetic variation to pharmacological treatment for alcohol dependence.
The goals of this chapter are to: (1) discuss personalized treatment and pharmacogenetics as it applies to alcoholism, (2) describe the Johnson Model of individual differences in response to pharmacological treatment for alcoholism, (3) discuss a dynamic control system model developed to examine the Johnson Model, and (4) discuss the potential for the use of control systems modeling to test hypotheses regarding the pharmacogenetics of alcoholism.
Personalized Alcoholism Treatment
Substantial efforts to identify personal traits that can inform choice of alcoholism treatment have not yet borne fruit. The most notable of such efforts is Project MATCH (Matching Alcoholism Treatment to Client Heterogeneity). Project MATCH was a large, multisite psychotherapy trial that ran for over a decade and enrolled over 1700 clients. It was designed to test 21 matching hypotheses by assigning clients randomly to three treatment groups—cognitive behavioral therapy, motivational enhancement therapy, or 12-step facilitation—and measuring the associations among matching characteristics and multiple alcohol outcomes across five follow-up periods. Project MATCH examined both primary (alcohol involvement, cognitive impairment, gender, meaning seeking, motivation, psychiatric severity sociopathy, support for drinking, and Babor’s Typology) and secondary (alcohol dependence, anger, antisocial personality disorder, interpersonal dependency, Axis I psychopathology, Alcoholics Anonymous history, readiness to change, religiosity, self-efficacy, and social functioning) matching hypotheses.
Clients in each of the Project MATCH treatment conditions showed rates of improvement, as measured by drinks per drinking day and abstinent days, similar to other treatment studies. Disappointingly, Project MATCH did not succeed at identifying personal traits associated with significant differences in treatment outcomes for the three alcoholism psychotherapies. Client anger, psychiatric comorbidity, and level of alcohol dependence were some of the client traits that provided limited prognostic utility.
Recently, a large trial to test alcoholism-treatment matching hypotheses was conducted in the United Kingdom. In the UK Alcohol Treatment Trial, investigators conducted 3- and 12-month follow-ups on clients ( n = 742 at baseline) who were assigned to either motivational enhancement therapy or social behavior and network therapy. The matching hypotheses in the UK study included (1) size of social network, (2) readiness to change/negative expectancies, (3) psychiatric severity, (4) anger, and (5) degree of alcohol dependence. The investigators found no evidence for matching effects on these client characteristics to the two types of treatment studied.
Although this brief review of personalized alcoholism treatment is not exhaustive, it does provide powerful evidence that easily observable client characteristics do not appear to be useful for matching individual clients to particular psychosocial treatments. Much of the excitement regarding personalized medicine, however, has to do with a better understanding of the biological underpinnings of disease and disorder, as well as the promise of applying this understanding to improving pharmacotherapy by informed dosage practices, increased response rates, and a reduction in the number and severity of side effects. This area, known as pharmacogenetics, is the major thrust of personalized medicine and is reviewed in the next section.
Pharmacogenetics
Pharmacokinetics is an area of focus within pharmacogenetics that studies how genetic differences influence the bioavailability of an agent. Individual differences in the rate of an agent’s metabolism produce variability in treatment response and may be crucial in serious or fatal adverse reactions to the medication. The cytochrome P450 (CYP) enzymes are located on organelles in liver cells and are one of the most studied components in agents’ metabolism. Approximately 7% of Caucasians can be classified as “poor metabolizers” on the basis of their genotype at the CYP2D6 polymorphism, which is known to be involved with the breakdown of many psychotropic medications. The end result of metabolizing psychotropic agents at a slower than average rate is that higher levels of the agents remain in the bloodstream, effectively raising the dose to levels that could potentially be harmful.
Pharmacodynamics is an area of focus within pharmacogenetics that studies the ways in which genetic differences in the proteins at which medications act produce individual differences in treatment response. An example of pharmacodynamic research that is important to psychiatry is the examination of the association between genetic variation in the gene that codes for the serotonin transporter and the response to depression treatment with selective serotonin reuptake inhibitors. Because reuptake of serotonin from the synapse via the serotonin transporter is the primary mode for inactivating serotonin’s actions and because the serotonin transporter is the primary target of selective serotonin reuptake inhibitors, it is logical to assume that genetic variation that influences serotonin transporter function would also affect efficacy of selective serotonin reuptake inhibitor treatment.
For more than a decade, the most studied genetic polymorphism in psychiatric or behavior genetics has been the so-called 5-HTTLPR insertion/deletion in the regulatory region located upstream of the structural gene that codes for the serotonin transporter. A recent search on PubMed for the term “HTTLPR” resulted in over 500 hits. The polymorphism is both functional and common, which makes it an excellent candidate for association studies. The L allele is a high-functioning allele that results in 2–3 times higher transcriptional efficiency than the S allele. Recently, investigators have been using functional magnetic resonance imaging (fMRI) to study associations between the 5-HTTLPR genotype and the activity of different brain regions. Specifically, amygdala activity in response to fearful or threatening facial expressions is greater in individuals who carry at least one S allele compared with those with the LL genotype. In addition, the functional connectivity between the amygdala and the prefrontal cortex is weaker in those who carry an S allele, which suggests that those individuals may be less able to dampen amygdala activity following an assessment of the potential threat.
Because the serotonin transporter is the primary target of selective serotonin reuptake inhibitors, it is of interest whether the 5-HTTLPR polymorphism is associated with a different response to selective serotonin reuptake inhibitor treatment. In a recent meta-analysis of 15 studies of selective serotonin reuptake inhibitor treatment for depression including a total of 1435 subjects, the L allele was associated with better treatment outcomes.
In addition to response to selective serotonin reuptake inhibitor treatment for depression, the 5-HTTLPR polymorphism is also associated with differences in the personality trait of neuroticism, suicide, impulse control disorders, and eating disorders. It is important to note that in the context of the present chapter, a meta-analysis of 17 published studies examining the association between 5-HTTLPR polymorphisms and alcohol dependence showed that the S allele was associated with an increased risk for alcohol dependence and that this association was strongest in the presence of psychiatric comorbidity.
The pharmacogenetics of alcoholism presents a complicated picture with a plethora of molecular targets for both ethanol and the medications used in alcoholism treatment. An examination of the pharmacokinetics of alcohol reveals genetic variants in ethanol-metabolizing enzymes that are associated with different levels of risk for alcohol dependence and that vary across ethnic groups. The well-studied enzymes in ethanol metabolism—alcohol dehydrogenase and aldehyde dehydrogenase—clearly affect the bioavailability of ethanol and a toxic by-product, acetaldehyde. Alcohol dehydrogenase converts ethanol to acetaldehyde, which is then quickly converted by aldehyde dehydrogenase to acetate. If these two conversions take place at similar rates, levels of the toxic acetaldehyde stay rather low in the blood. However, if the alcohol dehydrogenase enzyme is fast or if the aldehyde dehydrogenase enzyme is slow, toxic levels of acetaldehyde result. The medication disulfiram (Antabuse) inhibits aldehyde dehydrogenase, and when an individual drinks alcohol while taking disulfiram, severe nausea and other aversive symptoms result. This reaction is similar to the flushing response that occurs when individuals with one or two copies of a mutant version of aldehyde dehydrogenase-2 that produces a nonfunctioning version of the enzyme drink alcohol.
The pharmacodynamics of alcohol is complicated by its many targets. Ethanol facilitates the activity of γ-aminobutyric acid A (GABA A ) receptors, inhibits the activity of N -methyl- d -aspartate (NMDA) glutamate receptors, and, at high doses, inhibits many voltage-sensitive calcium channels. In addition, ethanol has direct influences on neurotransmission via serotonin, dopamine, and norepinephrine neurons, and modulates opioid neuropeptides. The endogenous opioid system is implicated in the rewarding effects of alcohol consumption and in alcohol craving. Genetic variation at these many sites of action is likely to be responsible for variation in responses to ethanol exposure.
Both Project MATCH and the UK Alcohol Treatment Trial focused solely on psychosocial therapies and excluded pharmacotherapy to simplify the designs of such large complicated studies. Clearly, there are biologically based individual differences that are likely to influence treatment outcome. Of interest, the Project MATCH investigators have recently begun to explore how variation in specific genes may prove to be predictive of drinking behavior and of the outcome of psychosocial treatment for alcoholism. A high-risk genotype of the GABRA2 gene (A/A) was associated with less variability in treatment outcome and higher risks for drinking and heavy drinking.
The pharmacodynamics of agents used in alcoholism pharmacotherapy is also of interest. Examples of medications used for the treatment of alcoholism include opiate antagonists (e.g., naltrexone); medications that interact with glutamate (e.g., acamprosate); modulators of both glutamate and GABA systems (e.g., topiramate); agents that influence serotonin uptake (e.g., selective serotonin reuptake inhibitors); and those that modulate serotonergic function and, as a consequence, dopaminergic neurotransmission (e.g., ondansetron). There are known genetic variants for neurotransmitter system components (e.g., receptors and enzymes) in all of these systems in which medications to treat alcoholism might act.
In this section, I provide a general description of pharmacogenetics along with a more specific discussion of the pharmacogenetics of alcohol and of medications used to treat alcoholism. It is clear that there are sufficient pharmacogenetics targets to begin examining the best candidates empirically. In the next section, a pharmacogenetics theoretical model is described that takes important steps toward the goal of better understanding how genotypes can be used to identify the most effective pharmacotherapy for alcoholism treatment.
The Johnson Model
Because both the serotonin and dopamine neurotransmitter systems are intimately involved with alcoholism in ways that are complex and not yet fully understood, theoretical models focusing on the impact of genetic variation at the level of the synapse may provide a productive approach to better understanding of the individual differences in response to alcoholism treatment. Such work combines empirical data from several different areas of study, such as human alcoholism treatment trials, candidate gene association studies, and pharmacological studies with both human and nonhuman animals. Alcoholism treatment trials can provide information regarding characteristics of subjects that are predictive of treatment response or of etiological significance. Candidate gene association studies can identify allelic variants associated with different alcohol phenotypes. The best of these candidates also will produce functional differences in the physiological systems of interest and are often targets of pharmacological agents. Pharmacological studies provide evidence regarding the effects of agonists and antagonists on system function and on alcohol phenotypes. The development of theoretical models that attempt to elucidate the impact of variation at functional candidate genes on the response to pharmacological treatment for alcoholism is an important stage in a systematic approach to personalized alcoholism treatment. Johnson and Ait-Daoud presented a theoretical model that was elaborated upon by Johnson and that aimed at better understanding the role of genetic variation in the serotonin transporter promoter region (5-HTTLPR) and differential response to two pharmacological treatments for alcoholism (hereafter called the Johnson Model). In this section, I provide details of this model on which a subsequent control system model was based.
The development of the Johnson Model was motivated by the desire to better understand the contribution of allelic variation at components of neurotransmitter systems to individual differences in pharmacological alcoholism treatment response. As such, these efforts should constitute a pharmacodynamic approach because the genetic differences examined are hypothesized to influence the proteins at which the medications act.
Fig. 65.1 presents the Johnson Model schematically. The main premise of the model is that relative serotonergic hypofunction, a result of efficient reuptake for individuals with the 5-HTTLPR LL genotype, produces an upregulation of serotonin-3 receptors and an enhanced urge to drink. This heightened urge to drink is the result of dopaminergic activation due to the action of serotonin at postsynaptic serotonin-3 receptors on dopaminergic neurons.

Several lines of empirical evidence led to the Johnson Model (see references in Johnson and Johnson and Ait-Daoud ):
- 1.
The S allele of the 5-HTTLPR polymorphism is a common variant that is dominant to the L allele and reduces serotonin reuptake by approximately one-third via a reduction in the number of serotonin transporter proteins produced (i.e., it significantly influences serotonin neurotransmission).
- 2.
Selective serotonin reuptake inhibitors bind to the serotonin transporter and block serotonin reuptake, thereby enhancing serotonin neurotransmission.
- 3.
Response to selective serotonin reuptake inhibitor treatment for alcoholism is better for those with late-onset alcoholism than for those with early onset alcoholism.
- 4.
Serotonin-3 receptors are located on mesocorticolimbic dopamine-containing neurons and are involved in the rewarding effects of alcohol.
- 5.
Ethanol potentiates the activity of serotonin-3 receptors.
- 6.
Response to alcoholism treatment with the serotonin-3 receptor antagonist ondansetron is better for those with early onset alcoholism than for those with late-onset alcoholism.
- 7.
Acute alcohol exposure increases serotonin function.
- 8.
Chronic alcohol exposure decreases serotonin function.
The primary focus of the Johnson Model was to explain the observation that for individuals with early onset alcoholism (assumed to be predominantly of the LL genotype), alcoholism pharmacotherapy with ondansetron was more effective than treatment with selective serotonin reuptake inhibitors. The efficacy of ondansetron was hypothesized to be due to its presumed effectiveness at reducing the rewarding effects of alcohol (via a reduction in dopaminergic activation due to serotonin-3 antagonism). The relative effectiveness of selective serotonin reuptake inhibitor pharmacotherapy for individuals with late-onset alcoholism (assumed to be predominantly carrying one or more S alleles) was hypothesized not to be due to serotonin-3 mechanisms. Rather, individuals with late-onset alcoholism and chronic selective serotonin reuptake inhibitor treatment were hypothesized to experience an anti-rewarding effect as a result of drinking alcohol because of a long-term inhibition of dopaminergic activity.
Because of the large number of components involved in the Johnson Model and the rather complex nature of the interactions among them, a systems-based model provides a platform for systematic testing of the model’s hypotheses. In the next section, I use a dynamic systems-based model to examine the Johnson Model.
A Dynamic Systems-Based Model
Although control system modeling has a relatively long history, with Wiener’s influential book on cybernetics first published in 1948, it has not been widely used in behavior genetic analysis. However, with an increasing focus on the mechanisms of heredity-behavior relations made possible by advances in molecular genetics and neuroscience, it seems that control system modeling is poised to catalyze significant contributions to our understanding of how genes influence behavior.
The Johnson Model is well suited for implementation as a control system model because it considers the system as a whole that can be described by the levels or rates of specified parameters. Levels are sometimes referred to as “stocks” or “states” and rates as “flows.” For example, in the Johnson Model, the level of serotonin in the synapse can be considered a stock and the rate of serotonin reuptake determined by 5-HTTLPR genotype can be considered a flow. Control system models are dynamic models, in that they utilize difference or differential equations to account for change over time, deriving subsequent states of the system from the current state. Control system models are particularly useful for modeling the influence of genetic differences or of pharmacological intervention on neurotransmitter system function where feedback loops are known to occur and where epistatic interaction plays a role. It is important to keep in mind that these kinds of control system models are not intended to be exact replicas of the system being simulated. These models describe qualitative behavior of the actual system under varied parameter states that can simulate genetic variation or pharmacological manipulation. An important goal of the control system model is to help to identify leverage points—that is, components of the system that are key in controlling system function. Therefore, a complete catalog of every component of the system is not necessary. Similarly, in control system modeling there is little focus on scaling every parameter so that biological realism is obtained. On the contrary, one often works with standardized parameters so that matters of scale are de-emphasized. One is more concerned with the functional relations among the parameters than with the relative lack of biological realism.
One of the most favorable features of control system modeling using systems of differential equations is that when these equations are solvable and produce unique solutions, the toolkit of the mathematician can be brought to bear, such as fast, general-purpose differential equation solvers such as Berkeley Madonna. Most importantly in the context of pharmacogenetics, one can examine the controllability of the system under study. That is, it may be possible to adjust the parameters of a system to move its functioning from an undesirable state to a desirable one. In the context of the serotonin system, there is substantial empirical evidence that it is possible to manipulate system parameters either by the use of medications or by genetic techniques (e.g., constructing “knockout” lines of mice) to alter serotonin system function. Although a complete understanding of the dynamics of the serotonin system has not yet been achieved, the use of control system modeling may enable the systematic variation of parameters in silico to achieve empirically based controllability of the system. In other words, dynamic systems models such as this one may provide us with the platform on which we can build an empirically derived understanding of how to adjust system parameters with medication to move an individual’s serotonin function into a desired state.
The model to test the Johnson Model arose out of efforts to model presynaptic regulation of the serotonin system. In that base model, presynaptic regulation of serotonin function was hypothesized to be controlled by three components, each of which varied genetically ( Fig. 65.2 ). These components included the serotonin transporter that removes serotonin from the extracellular space via reuptake; the somatodendritic autoreceptor (serotonin-1A) that inhibits neural firing and, therefore, serotonin release; and the terminal autoreceptor (serotonin-1B) that influences the amount of serotonin released. Each of these components was hypothesized to have a high and a low functioning variant. The main outcome variable for this model of presynaptic regulation is the level of extracellular serotonin. Another outcome that can be considered is the firing rates of serotonin neurons.
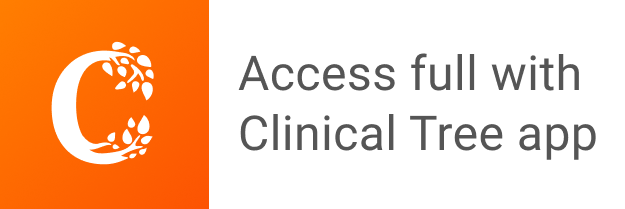