An individual’s state of health is often characterized by a number of numerical or categorical measures. In this context, an appropriate reference interval (Chapters 6 and 7) and/or diagnostic test may be used:
- by the clinician, together with a clinical examination, to diagnose or exclude a particular disorder in his or her patient;
- as a screening device to ascertain which individuals in an apparently healthy population are likely to have (or sometimes, not have) the disease of interest. Individuals flagged in this way will then usually be subjected to more rigorous investigations in order to have their diagnosis confirmed. It is only sensible to screen for a disease if there are adequate facilities for treating the disease at the pre-symptomatic stages, this treatment being less costly and/or more effective than when given at a later stage (or, occasionally, if it is believed that individuals who are diagnosed with the disease will modify their behaviour to prevent the disease spreading).
A diagnostic test may also be used:
- as one of an array of routine tests (e.g. blood tests) which may identify a disorder unrelated to the condition under investigation;
- as a staging test (e.g. for cancer);
- as a monitoring test to track a patient’s progress over time (e.g. blood pressure).
This chapter describes some of the methods that are used to develop these diagnostic tools for clinical use and explains how to interpret their results.
Reference Intervals
A reference interval (often referred to as a normal range) for a single numerical variable, calculated from a very large sample, provides a range of values that are typically seen in healthy individuals. If an individual’s value is above the upper limit, or below the lower limit, we consider it to be unusually high (or low) relative to healthy individuals.
Calculating Reference Intervals
Two approaches can be taken.
- We make the assumption that the data are Normally distributed. Approximately 95% of the data values lie within 1.96 standard deviations of the mean (Chapter 7). We use our data to calculate these two limits (mean ± 1.96 × standard deviation).
- An alternative approach, which does not make any assumptions about the distribution of the measurement, is to use a central range which encompasses 95% of the data values (Chapter 6). We put our values in order of magnitude and use the 2.5th and 97.5th percentiles as our limits.
The Effect of Other Factors on Reference Intervals
Sometimes the values of a numerical variable depend on other factors, such as age or sex. It is important to interpret a particular value only after considering these other factors. For example, we generate reference intervals for systolic blood pressure separately for men and women.
Diagnostic Tests
The gold standard test that provides a definitive diagnosis of a particular condition may sometimes be impractical or not routinely available. We would like a simple test, depending on the presence or absence of some marker, which provides a reasonable guide to whether or not the patient has the condition.
To evaluate a diagnostic test, we apply this test to a group of individuals whose true disease status is known from the gold standard test. We can draw up the 2 × 2 table of frequencies (Table 38.1):
Table 38.1 Table of frequencies.
Of the n individuals studied, a + c individuals have the disease. The prevalence (Chapter 12) of the disease in this sample is
Of the a + c individuals who have the disease, a have positive test results (true positives) and c have negative test results (false negatives). Of the b + d individuals who do not have the disease, d have negative test results (true negatives) and b have positive test results (false positives).
Assessing the Effectiveness of the Test: Sensitivity and Specificity
These are usually expressed as percentages. As with all estimates, we should calculate confidence intervals for these measures (Chapter 11).
We would like our test to have a sensitivity and specificity that are both as close to 1 (or 100%) as possible. However, in practice, we may gain sensitivity at the expense of specificity, and vice versa. Whether we aim for a high sensitivity or high specificity depends on the condition we are trying to detect, along with the implications for the patient and/or the population of either a false negative or false positive test result. For conditions that are easily treatable, we prefer a high sensitivity; for those that are serious and untreatable, we prefer a high specificity in order to avoid making a false positive diagnosis. It is important that, before screening is undertaken, subjects should understand the implications of a positive diagnosis, as well as having an appreciation of the false positive and false negative rates of the test.
Using the Test Result for Diagnosis: Predictive Values
We calculate confidence intervals for these predictive values, often expressed as percentages, using the methods described in Chapter 11.
The sensitivity and specificity quantify the diagnostic ability of the test but it is the predictive values that indicate how likely it is that the individual has or does not have the disease, given his or her test result. Predictive values are dependent on the prevalence of the disease in the population being studied. In populations where the disease is common, the positive predictive value of a given test will be much higher than in populations where the disease is rare. The converse is true for negative predictive values. Therefore, predictive values can rarely be generalized beyond the study.
The Use of a Cut-off Value
Sometimes we wish to make a diagnosis on the basis of a numerical or ordinal measurement. Often there is no threshold above (or below) which disease definitely occurs. In these situations, we need to define a cut-off value ourselves above (or below) which we believe an individual has a very high chance of having the disease.
A useful approach is to use the upper (or lower) limit of the reference interval. We can evaluate this cut-off value by calculating its associated sensitivity, specificity and predictive values. If we choose a different cut-off, these values may change as we become more or less stringent. We choose the cut-off to optimize these measures as desired.
The Receiver Operating Characteristic (ROC) Curve
This provides a way of assessing whether a particular type of test provides useful information, and can be used to compare two different tests, and to select an optimal cut-off value for a test.
To draw the receiver operating characteristic (ROC) curve for a given test, we consider all cut-off points that give a unique pair of values for sensitivity and specificity, and plot the sensitivity against one minus the specificity (thus comparing the probabilities of a positive test result in those with and without disease) and connect these points by lines (Fig. 38.1).
The ROC curve for a test that has some use will lie to the left of the diagonal (i.e. the 45 ° line) of the graph. Depending on the implications of false positive and false negative results, and the prevalence of the condition, we can choose the optimal cut-off for a test from this graph. The overall accuracy of two or more tests for the same condition can be compared by considering the area under each curve (sometimes referred to as AUROC); this area can be calculated manually or is given by the c statistic. c can be interpreted as the probability that a randomly chosen subject from the disease group has a higher predicted probability of having the disease than a randomly chosen subject from the disease-free group. The test with the greater area (i.e. the higher c statistic) is better at discriminating between disease outcomes. A test which is perfect at discriminating between the disease outcomes has c = 1 and a non-discriminating test which performs no better than chance has c = 0.5.
We also discuss the area under the ROC curve in Chapter 46 in the context of prognostic scores.
Is a Test Useful?
The likelihood ratio (LR) for a positive test result is the ratio of the chance of a positive result if the patient has the disease to the chance of a positive result if he or she does not have the disease (see also Chapter 32). For example, a LR of 2 for a positive result indicates that a positive result is twice as likely to occur in an individual with disease than in one without it.
It can be shown that
A likelihood ratio can also be generated for a negative test result and is most easily calculated as (1 − sensitivity)/specificity. A high likelihood ratio for a positive test result (e.g. >10) suggests that the test is useful and provides evidence to support the diagnosis. Similarly, a likelihood ratio close to zero (e.g. <0.01) for a negative result allows us to rule out the diagnosis. We discuss the LR in the context of diagnostic tests in a Bayesian framework in Chapter 45.
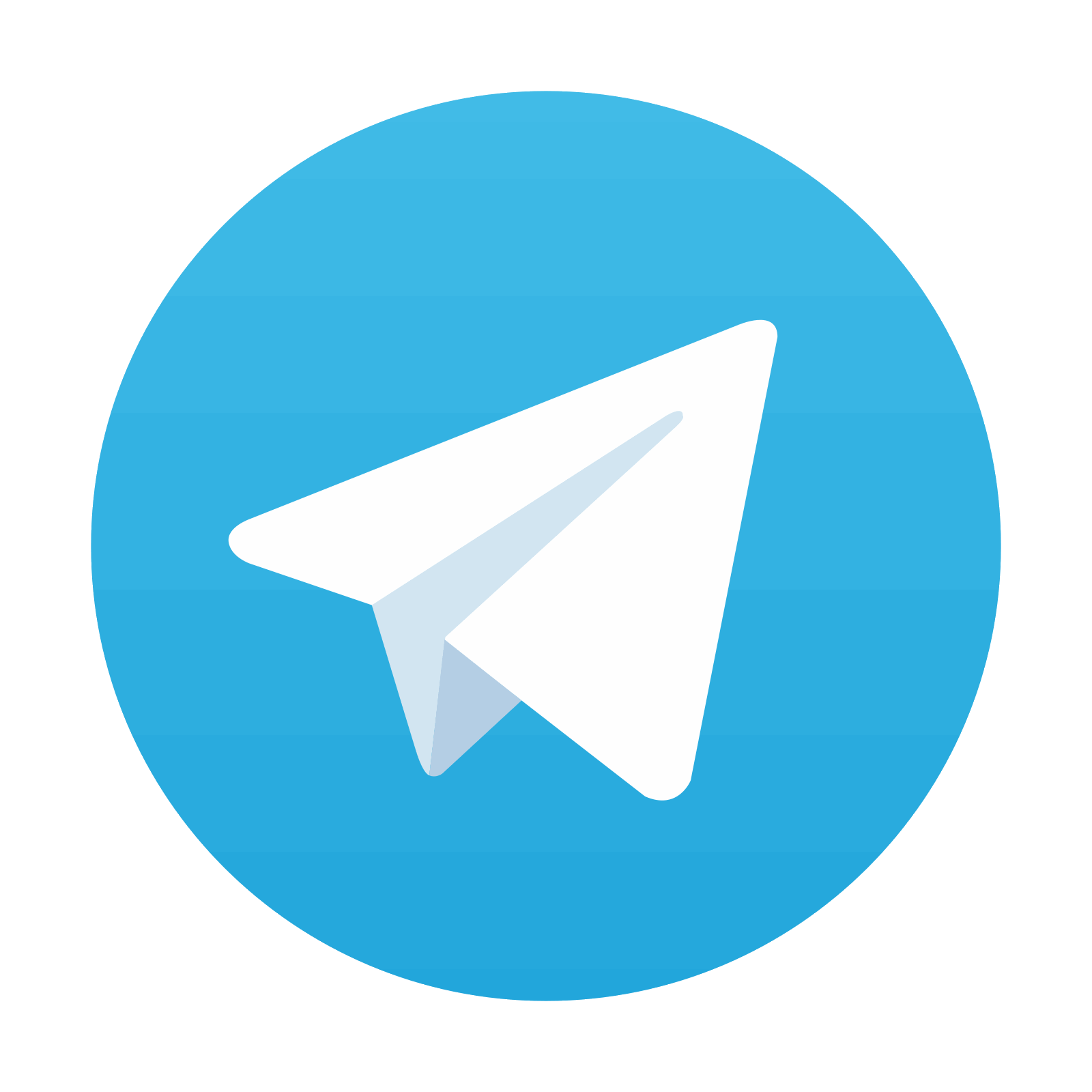
Stay updated, free articles. Join our Telegram channel

Full access? Get Clinical Tree
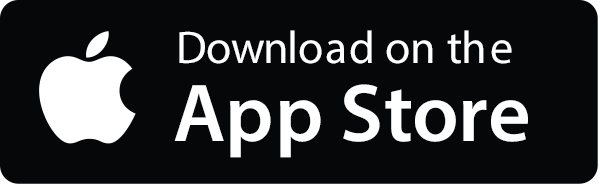
