Introduction
Correlation analysis is concerned with measuring the degree of association between two variables, x and y. Initially, we assume that both x and y are numerical, e.g. height and weight.
Suppose we have a pair of values, (x, y), measured on each of the n individuals in our sample. We can mark the point corresponding to each individual’s pair of values on a two-dimensional scatter diagram (Chapter 4). Conventionally, we put the x variable on the horizontal axis, and the y variable on the vertical axis in this diagram. By plotting the points for all n individuals, we obtain a scatter of points that may suggest a relationship between the two variables.
Pearson Correlation Coefficient
We say that we have a linear relationship between x and y if a straight line drawn through the midst of the points provides the most appropriate approximation to the observed relationship. We measure how close the observations are to the straight line that best describes their linear relationship by calculating the Pearson product moment correlation coefficient, usually simply called the correlation coefficient. Its true value in the population, ρ (the Greek letter rho), is estimated in the sample by r, where
which is usually obtained from computer output.
Properties
- r ranges from −1 to +1.
- Its sign indicates whether, in general, one variable increases as the other variable increases (positive r) or whether one variable decreases as the other increases (negative r) (see Fig. 26.1).
- Its magnitude indicates how close the points are to the straight line. In particular if r = +1 or −1, then there is perfect correlation with all the points lying on the line (this is most unusual, in practice); if r = 0, then there is no linear correlation (although there may be a non-linear relationship). The closer r is to the extremes, the greater the degree of linear association (Fig. 26.1).
- It is dimensionless, i.e. it has no units of measurement.
- Its value is valid only within the range of values of x and y in the sample. Its absolute value (ignoring sign) tends to increase as the range of values of x and/or y increases. Therefore, restricting the sample by imposing an upper or lower limit on the range of values of x or y or adding individuals to the sample who have values of x or y that are more extreme than those in the original sample will affect the magnitude of the correlation coefficient; furthermore, correlation coefficients should not be compared in populations which have a different range of values of x or of y.
- x and y can be interchanged without affecting the value of r.
- A correlation between x and y does not necessarily imply a ‘cause and effect’ relationship.
- r2 represents the proportion of the variability of y that can be attributed to its linear relationship with x (Chapter 28).
When not to Calculate r
It may be misleading to calculate r when:
- there is a non-linear relationship between the two variables (Fig. 26.2a), e.g. a quadratic relationship (Chapter 33);
- the data include more than one observation on each individual;
- one or more outliers are present (Fig. 26.2b);
- the data comprise subgroups of individuals for which the mean levels of the observations on at least one of the variables are different (Fig. 26.2c).
Figure 26.2 Diagrams showing when it is inappropriate to calculate the correlation coefficient. (a) Relationship not linear, r = 0. (b) In the presence of outlier(s). (c) Data comprise subgroups.

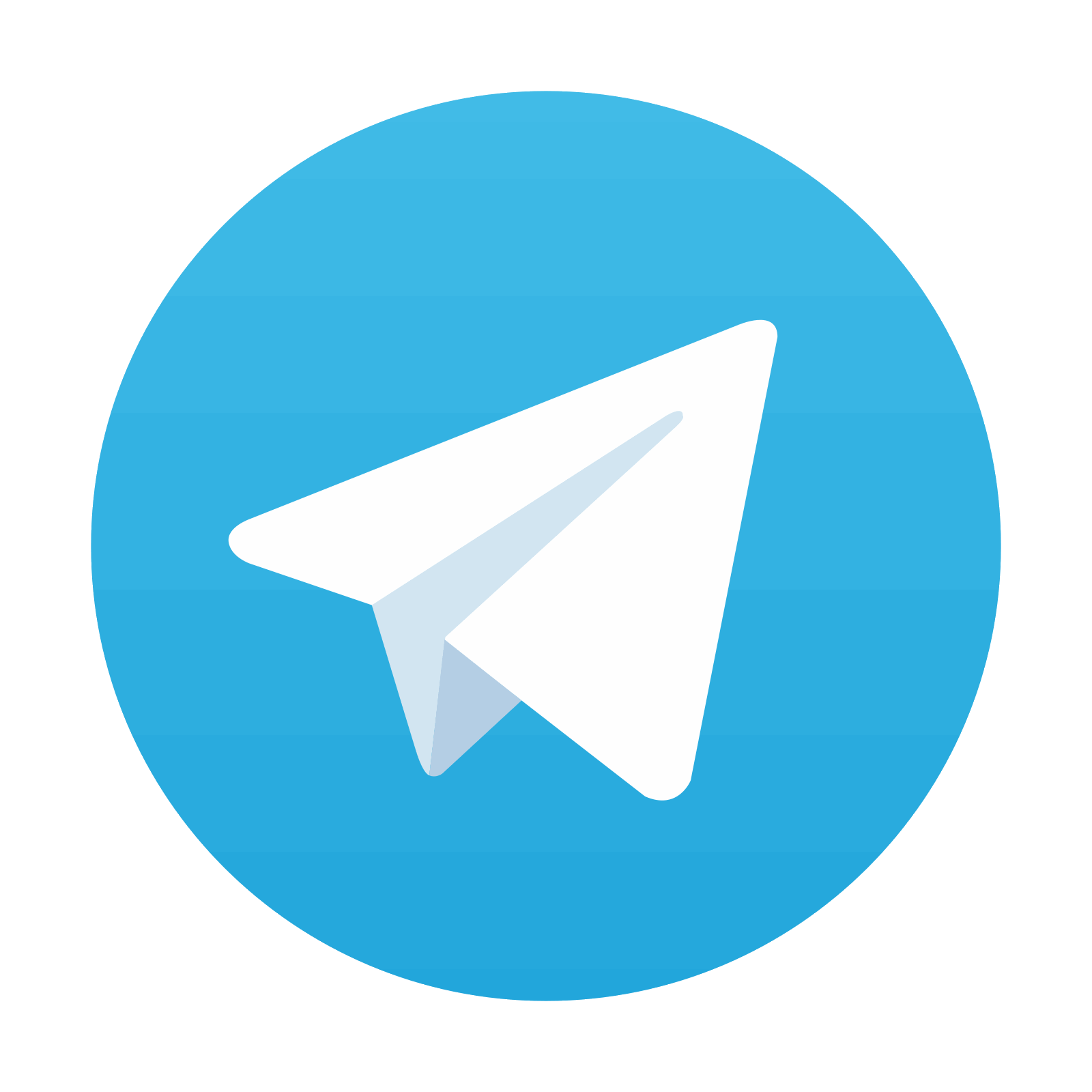
Stay updated, free articles. Join our Telegram channel

Full access? Get Clinical Tree
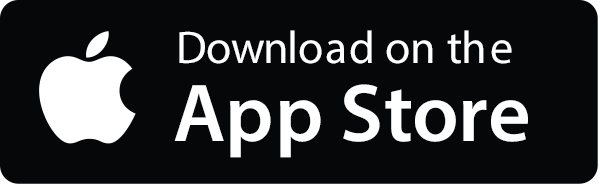
