Chapter Contents
Data Collection: Forwards and Backwards 40
Advantages of Cohort Studies 41
Disadvantages of Cohort Studies 42
What to Look for in Cohort Studies 43
Who is at Risk? 43
Who is Exposed? 43
Who is an Appropriate Control? 43
Have Outcomes Been Assessed Equally? 44
Tracking Participants Over Time 44
Have Losses Been Minimised? 44
Reporting Cohort Studies 44
Variations on the Cohort Theme 47
Conclusion 48
A cohort study tracks two or more groups forward from exposure to outcome. This type of study can be done by going ahead in time from the present (prospective cohort study) or, alternatively, by going back in time to comprise the cohorts and following them up to the present (retrospective cohort study). A cohort study is the best way to identify incidence and natural history of a disease and can be used to examine multiple outcomes after a single exposure. However, this type of study is less useful for examination of rare events or those that take a long time to develop. A cohort study should provide clear, specific, and measurable definitions of exposures and outcomes; determination of both should be as objective as possible. The control group (unexposed) should be similar in all important respects to the exposed, with the exception of not having the exposure. Observational studies, however, rarely achieve such a degree of similarity, so investigators need to measure and control for confounding factors. Avoiding loss to follow-up over time is a challenge, because differential losses introduce bias. Variations on the cohort theme include the before-after study and nested case-control study (within a cohort study). Strengths of a cohort study include the ability to calculate incidence rates, relative risks, and 95% confidence intervals (CIs). This format is the preferred way of presenting study results, rather than solely with p values.
The term ‘cohort’ has military, not medical, roots. The term was first used in research by Frost in a 1935 study examining mortality from tuberculosis. A cohort was a 300- to 600-man unit in the Roman army; 10 cohorts formed a legion ( Fig. 4.1 ). The etymology of the term provides a useful mnemonic: a cohort study consists of bands or groups of persons marching forward in time from an exposure to one or more outcomes.

This analogy might be helpful, because cohort studies have a bevy of confusing synonyms: incidence, longitudinal, panel, forward-looking, follow-up, concurrent, and prospective studies. Although the terminology can seem daunting, the cohort study is easy for clinicians to understand, because it flows in a logical direction (unlike the case-control study). Here, we explain the terminology, describe the strengths and weaknesses of cohort studies, consider several logistical concerns, mention two permutations of cohort studies, and summarise their analysis.
Data Collection: Forwards and Backwards
A cohort study follows two or more groups from exposure (or varying amounts of exposure) to outcome. In its simplest form, a cohort study compares the experience of a group exposed to some factor with another group not exposed to the factor. If the former group has a higher or lower frequency of an outcome than the unexposed, then an association between exposure and outcome is evident.
The defining characteristic of all cohort studies is direction: they track people forward in time from exposure to outcome. Researchers doing this kind of study must, therefore, go forward in time from the present or go back in time to choose their cohorts ( Fig. 4.2 ). Either way, a cohort study moves in the same direction, although gathering data might not. For example, an investigator who wants to study the epidemic of multiple births stemming from assisted reproductive technologies could begin a cohort study now. Women exposed to these technologies and a similar group who conceived naturally could be tracked forward through their pregnancies to monitor the frequency of multiple births (a concurrent cohort study). Alternatively, the investigator might use existing medical records and go back in time several years to identify women exposed and not exposed to these technologies. The researcher would then track these women forward through records to determine the birth outcomes. Again, the study moves from exposure to outcome, though the data collection occurred after the fact (a retrospective cohort study). Other names for this approach include historical cohort study, historical prospective study, nonconcurrent prospective study, and (paradoxically) prospective study in retrospect.

Yet a third variation exists: ambidirectional . As the name implies, data collection goes in both directions. This approach can be useful for exposures that have both short-term and long-term outcomes. In this hypothetical example, assisted reproductive technologies might be associated with multiple births and with breast cancer later in life. The investigator might, therefore, look back through records for multiple births and also start to follow up these women into the future for breast cancer occurrence. Ambidirectional cohort studies include those that start after exposure has occurred but before the outcome has developed (e.g., smoking or asbestos exposure and later lung cancer).
Advantages of Cohort Studies
Cohort studies have many appealing features. They are the best way to ascertain both the incidence and natural history of a disorder. The temporal sequence between putative cause and outcome is usually clear; the exposed and unexposed participants can often be confirmed free of the outcome at the outset. By contrast, this chicken–egg question often frustrates cross-sectional and case-control studies. For example, cross-sectional studies indicate that chronic pain is associated with mental disorders, including depression. Do mood and anxiety disorders increase perceived pain, or do patients with chronic pain develop mood and anxiety disorders because of their pain?
Cohort studies are useful in investigation of multiple outcomes that might arise after a single exposure. Stated alternatively, a cohort study of one exposure could examine many outcomes. A prototype would be cigarette smoking (exposure) and stroke, emphysema, oral cancer, and heart disease (outcomes). Although assessment of many outcomes is often cited as a positive attribute of cohort studies, this feature can be abused.
P hacking, also known as data mining, snooping, fishing, and significance chasing, is defined as ‘trying multiple things until you get the desired results’. One review of observational study reports identified 10 articles that tested over 100 associations between exposure and outcome, the maximum being 264! Researchers commonly test associations between exposure and many outcomes but only report the significant ones, raising the likelihood of false-positive findings (alpha error). Investigators should have prespecified primary and secondary associations to examine (sometimes called hypothesis confirmation). Although investigators can look at other outcomes (hypothesis generation), they should report the findings of all tested associations, not just significant ones, so that readers can correctly interpret the results.
The cohort design is also useful in the study of rare exposures; a researcher can often recruit people with uncommon exposures (e.g., to ionising radiation or chemicals) in the workplace. A hospital or factory might provide a large number of individuals with the exposure of interest, which would be rare in the general population. Also, with rare exposures, cohort studies facilitate sampling a high fraction of the exposed while sampling a small fraction of the unexposed. That leads to study efficiency. Unlike case-control studies ( Chapter 5 ), which are useful for studying rare outcomes, cohort studies are adept at studying rare exposures.
Cohort studies also reduce the risk of survivor or prevalence–incidence bias, first described by Neyman. Diseases that are rapidly fatal are difficult to study with a case-control design because of this. For example, a hospital-based case-control study of the link between snow shovelling and myocardial infarction would miss all cases who died in the driveway, shovel in hand. The most severe cases would be missed, and enrolled cases would not be representative of all infarct patients. A cohort study would be a less biased (but admittedly more cumbersome) approach: compare rates of myocardial infarction among those who shovel and those who do not shovel. Finally, cohort studies allow calculation of incidence rates, relative risks, and confidence intervals, the preferred measures for dichotomous outcomes. Other outcome measures in cohort studies include life-table rates, survival curves, and hazard ratios ( Panel 4.1 ). In contrast, case-control studies cannot provide incidence rates; at best, odds ratios approximate relative risks only when the outcome is uncommon.
Survival Analysis
Survival analysis is useful when lengths of follow-up vary substantially or when participants enter a study at different times. The Kaplan-Meier method provides a more sophisticated expression of the risk of the outcome over time than does a simple dichotomous outcome (e.g., alive or dead). It can determine the probability ( p ) of the outcome at any point in time; this result is graphed as a step function (which jumps at every event). A complementary, mirror-image graph portrays the likelihood of avoiding the outcome (1 – p ) as a function of time (Kaplan-Meier survival curve). The log-rank test compares survival curves of different groups.
Proportional Hazards Model
Another approach to different lengths of follow-up is the Cox proportional hazards model. It is a multivariable technique that has time-to-event (such as illness) as the dependent variable. By contrast, multiple logistic regression has ‘yes–no’ as the dependent variable. Coefficients from this model can be used to calculate the hazard ratio of the outcome, after controlling for other covariates in the equation. The hazard ratio (with 95% CIs) is interpreted in the same way as a relative risk for dichotomous outcomes.
Disadvantages of Cohort Studies
Cohort studies have important limitations, too. Selection bias is built into cohort studies. For example, in a cohort study investigating effects of jogging on cardiovascular disease, those who choose to jog probably differ in other important ways (such as diet and smoking) from those who do not exercise. In theory, both groups should be the same in all important respects, except for the exposure of interest (jogging), but this seldom occurs. The cohort design is challenging for rare diseases (e.g., scleroderma). Cohort studies of diseases that take a long time to develop (e.g., cancer) can be prohibitively expensive. However, several large long-term cohort studies have made landmark contributions to our knowledge of many diseases, both common and uncommon. Examples include the Royal College of General Practitioners’ Oral Contraceptive Study, the Framingham Heart Study, the Nurses’ Health Study, and the British Physicians’ Study.
Because the data already exist, retrospective cohort studies are popular. This feature is a double-edged sword: the quality of extant data is usually inferior—or incomplete—compared with information collected prospectively and targeted to the question at hand. Moreover, privacy regulations make contacting patients for supplementary information difficult or impossible. Hence, data quality is usually better with prospective than retrospective cohort studies.
Loss to follow-up can be a difficulty, particularly so with longitudinal studies that continue for decades. However, with multiple-stage follow-up procedures, high follow-up rates are achievable in large, contemporary cohort studies. Differential losses to follow-up between those exposed and unexposed can bias results. Over time, the exposure status of study participants can change. For example, a proportion of women who use oral contraceptives will switch to an intrauterine device, and vice versa . Partitioning by duration of exposure to a method can avoid a blurring of exposure, sometimes termed ‘contamination’.
What to Look for in Cohort Studies
Who is at risk?
All participants (both exposed and unexposed) in a cohort study must be at risk of developing the outcome. For example, because women who have had a tubal sterilisation operation have almost no risk of salpingitis, they should not be included in cohort studies of this disease. Similarly, women after hysterectomy have no risk of cervical cancer and should be excluded from studies of this cancer.
Who is exposed?
Cohort studies need a clear, unambiguous definition of the exposure at the outset. This definition sometimes involves quantifying the exposure by degree, rather than just ‘yes’ or ‘no’. For example, a large cohort study of smoking and nasopharyngeal cancer in China defined a daily smoker as ‘one who smoked at least one cigarette per day for at least 6 months’. Another large cohort study of the relationship between super-obesity and perinatal outcomes in Australia and New Zealand defined the exposure explicitly: any pregnant woman at ≥20 weeks’ gestation with a body mass index >50 or a weight >140 kg.
Who is an appropriate control?
The key notion is that controls (the unexposed) should be similar to the exposed in all important respects, except for the lack of exposure. If so, the unexposed group will reveal the background rate of the outcome expected in the community. The unexposed group can come from either internal (persons from the same time and place, such as a hospital ward) or external sources. Internal comparisons are preferable. In a particular population, individuals segregate themselves (or through genetics or medical interventions) into exposure status (e.g., cigarette smoking, occupation, contraception). In a Scottish study of the potential effect of statins on prolonging breast cancer survival, the exposed were those who received a statin after breast cancer diagnosis, while the unexposed lacked this exposure; no association was found.
If satisfactory internal controls are not available, researchers must look elsewhere. In a trial of an occupational exposure, finding an adequate number of employees in the factory without the exposure might be difficult. Hence, one might choose workers in a similar factory in the same community. This choice assumes that workers in the other factory have the same baseline risk of the outcome in question, which might not be the case. Even less desirable is use of population norms; disease-specific mortality rates are an example. A researcher might compare lung-cancer death rates among workers in the factory with rates of persons of the same age and sex in the population. Bias inevitably creeps into such comparisons because of the healthy-worker effect: those who work are healthier, in general, than those who do not (or cannot) work. Additionally, work has obvious economic benefits, which might further bias comparisons.
Have outcomes been assessed equally?
Outcomes must be defined in advance; they should be clear, specific, and measurable. Identification of outcomes should be comparable in every way for the exposed and unexposed to avoid information bias. Failure to define objective outcomes leads to uninterpretable results. This challenge relates not only to subjective outcomes such as Gulf War and chronic fatigue syndromes, but also to more mundane diseases such as endometritis. Just how tender must a uterus be to merit this diagnosis? Because endometritis cannot be objectively defined, it cannot be studied; febrile morbidity may be a proxy. Similarly, the voluminous literature on the metabolic syndrome is currently unintelligible because of many different definitions.
Keeping those who judge outcomes unaware of the exposure status of participants (blinding) in a cohort study is important for subjective outcomes, such as cellulitis or stiffness. By contrast, with objective outcome measures, such as fever or death, blinding the exposure status is less important.
Outcome information can come from many sources. For mortality studies, the death certificate is often used. Although convenient, the validity of the clinical information is highly variable. For nonfatal outcomes, sources include hospital charts, electronic medical records, insurance records, laboratory records, disease registries, hospital discharge logs, and physical examination and measurement of participants. Optimally, the person who judges outcomes should be unaware of the exposure. When diagnoses vary in their confidence, assignment of levels of assurance might be helpful, such as definite, probable, and suspect. A better approach is to have blinded adjudication of all possible important outcome measures reported by participants in a study.
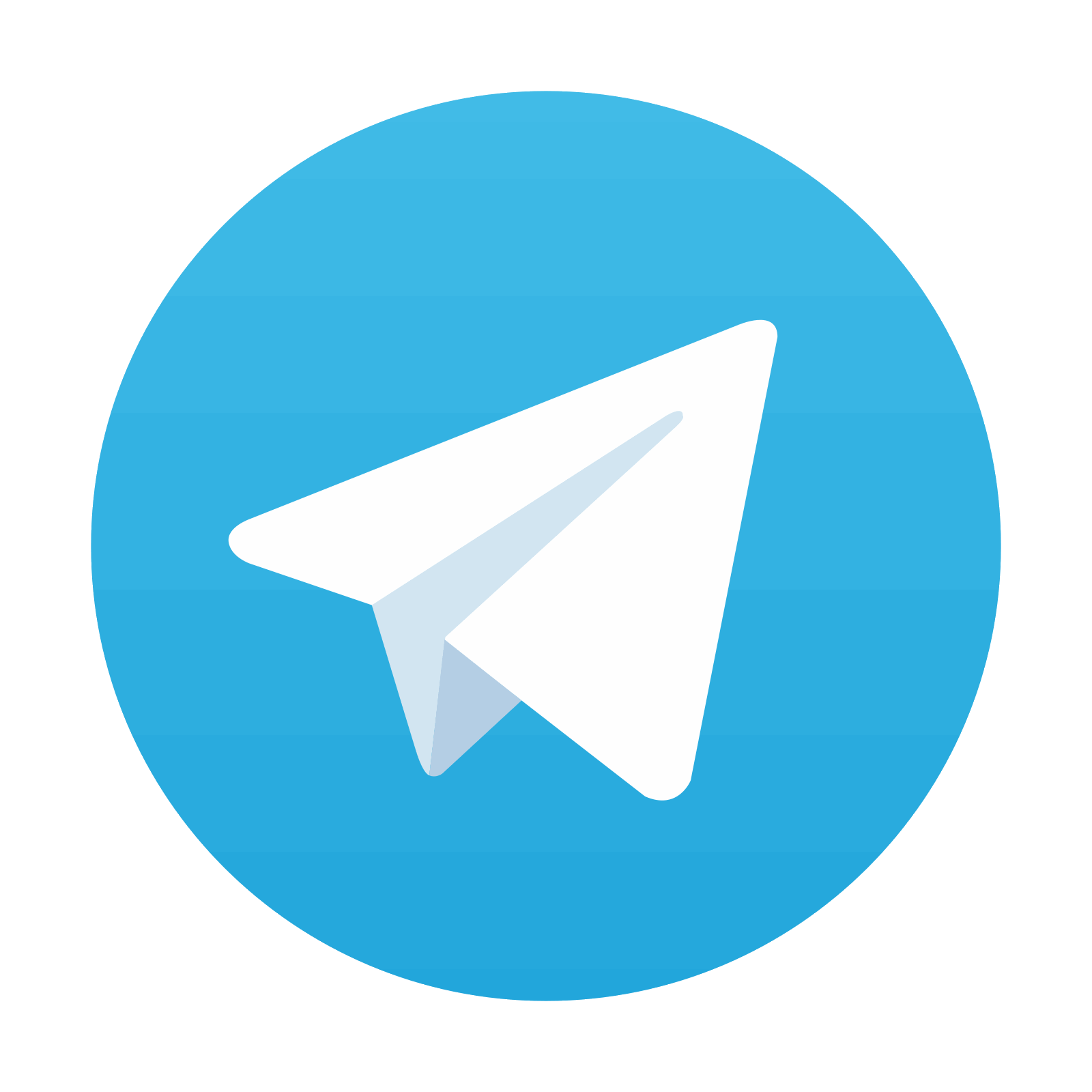
Stay updated, free articles. Join our Telegram channel

Full access? Get Clinical Tree
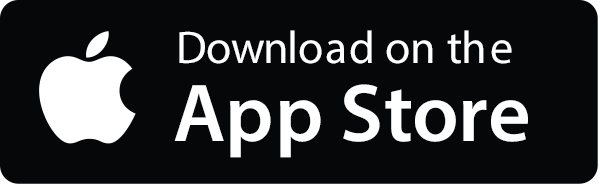
