Fig. 11.1
Typical multi-tiered immunogenicity testing approach employed in clinical trials
Screening Assay
In the screening tier of immunogenicity testing, the samples responses are compared against the screening cut point. Samples with the response below the screening cut point are declared negative and excluded from further testing. Samples with responses at or above the screening cut point are defined as potentially positive and directed for additional testing in the confirmatory tier. Since samples below the screening cut point are never re-tested again, it is important to avoid false negative classifications. Therefore, selection of an appropriate screening cut point involves a tradeoff between sensitivity and specificity. High specificity (a low false positive rate) is likely to result in low sensitivity (a high number of false negatives). Since immunogenicity may have impact on patient safety, risk management dictates that false positives are preferred to false negatives (Koren et al. 2008). Regulatory agencies and white papers recommend setting the screening cut point to generate 5 % of false positive classifications with the aim of decreasing the number of false negatives.
While there is a relationship between false positive and false negative rates, the latter cannot be clearly defined or measured. This is due to the lack of a “gold standard” in immunogenicity assays. As explained above, ADA is not a single and well defined situation but rather a nebulous and ever changing entity that consists of multiple antibody clones binding to multiple epitopes of the therapeutic macromolecule. For obvious reasons, one cannot immunize human subjects with the drug for the sole purpose of obtaining ADAs that could serve as a positive control in an assay. Antibodies against the drug of interest are raised in animals and are used as surrogate positive controls with the caveat that they are a partial and imperfect representation of an ADA developed in patients. Animal-derived polyclonal (recognizing multiple epitopes) or monoclonal (recognizing a single epitope) anti-drug antibodies are used as positive controls to establish such assay performance parameters as intra- and inter-assay precision, sensitivity and drug tolerance during pre-study assay validation, and for in-study monitoring of assay performance. The problem of false negatives is especially difficult to address since it is unclear what ADA levels may lead to clinically meaningful outcomes. Regulatory agencies recommend that clinical immunogenicity assays be able to detect 250–500 ng/mL ADA. However, it is conceivable that some antibodies can have a large impact at significantly lower concentrations (e.g. IgE isotype); while other may have no observable consequences at higher concentrations. Therefore, a risk-based strategy is particularly important to immunogenicity assessment (Kloks et al. 2015; Koren et al. 2008; Rosenberg and Worobec 2004, 2005a, b). Section 11.3 describes in detail current statistical methods used for determination of the screening cut point.
Confirmatory Assay
The screening tier tries to eliminate all true negatives and results in a pool of samples that are greatly “enriched” in true positives in addition to some (approximately 5 %) false positive responses. The second tier of testing should eliminate the vast majority of these false positives and provide unambiguous positive/negative classification. The assay commonly used for this purpose involves competitive inhibition of signal by unlabeled drug to show that the signal is specific to the drug. Any signal generated by ADA should be inhibited by addition of the drug, whilst a signal resulting from non-specific binding should not be inhibited or inhibited to a lesser extent. It is unclear what level of signal inhibition corresponds to a cutoff between specific and non-specific binding. Neyer et al. (2006) proposed the use of a paired t-test to compare the sample signal in presence and absence of the drug. Arguably, with a sufficiently large number of observations (repeated measurements), very small differences caused by the addition of the drug may become statistically significant, regardless of biological variability and clinical relevance. Another approach applied by some laboratories is to spike samples with low, medium and high amounts of the positive ADA and to treat them with a known amount of drug. A confirmatory cut point is then derived by statistical analysis of the signal differences between spiked and non-spiked samples. This approach is strongly dependent on the nature of the positive control ADA, with a possibility that ADAs derived from animals are not representative of the immune response in patients. This complicates the interpretation of immunogenicity data (Smith et al. 2011). The most common bioanalytical approach is to obtain inhibition data by spiking excess of drug into 50–100 individual drug-naïve samples and statistically determining a cutoff response corresponding to 1 % or 0.1 % false positive rate; see Sect. 11.3 for the description of related statistical methods.
Neutralizing Antibody Assay
Neutralizing antibodies (NAbs) interfere with the binding of a drug to its target and prevent the drug from eliciting the desired physiological effect. Presence of NAbs can prove to be especially dangerous if they neutralize activity of non-redundant endogenous protein in humans. The neutralizing effect of NAbs can be best detected using cell lines which respond to the drug in question. The direct action of a drug on cells may result in cell proliferation or cell death. In the absence of NAbs, the drug should have its full impact on cells, whilst in the presence of NAbs, the effect of a drug should diminish. Drugs may have indirect effects on cells when they enhance or prevent binding of soluble ligands to cell surfaces. When NAbs are absent, a drug can fully engage with the ligand and prevent it from exercising its physiological function. On the other hand, presence of NAbs leads to apparent enhancement of the ligand activity. Cell-based assays can prove extremely challenging due to lack of appropriate cell lines and difficulties associated with their growth and maintenance. Cell-based assays often suffer from low sensitivity, narrow dynamic range, and poor tolerance to circulating drug and serum proteins. Alternatively, ligand-binding assays can be used to analyze NAbs and typically are not subject to the disadvantages of cell-based assays. When a drug target is present on cell surface, cell-based assays are arguably best suited for detection of NAbs and evaluation of in vivo interactions between ADA, drug and target. At the same time, a legitimate argument can be made that ligand-binding assays with their higher sensitivity and robustness are ultimately more informative and better suited to the analysis of clinical samples.
11.1.3 Assay Platforms
An analytical platform utilizes certain unique physical-chemical properties of the analyte to detect and quantify it in a matrix of interest. A thorough understanding of these properties is indispensable in order to properly interpret bioanalytical data. Detailed discussion of the plethora of analytical techniques available to biopharmaceutical researcher is beyond the scope of this chapter and here we will focus only on ligand-binding assays, which are widely used for PK and PD measurements as well as for ADA detection.
11.1.3.1 Ligand-Binding Assays
Ligand-binding assays (LBAs) depend on interactions between a receptor and its ligand. In broader terms, the receptor-ligand pair can include combinations of various proteins (peptides, antibodies or their fragments, enzymes, receptors, etc.), nucleic acids (RNA, DNA), and relatively small molecules (e.g. steroids). These interactions are characterized by high affinity and specificity. Consequently, ligand-receptor complexes are formed in the presence of other similar species. These properties make ligand-binding assays ideal for detection and quantification of biological molecules in complex matrices. The large number of possible interactions allow for a variety of possible assay formats. The vast majority of ligand-binding assays require some sort of solid support to capture the analyte of interest from the sample and remove the excess of matrix. This solid support can be in the form of appropriately activated plastic surfaces (plates, tubes, biosensor chips etc.) or in form of beads or nanoparticles which allow application of fluidics. A general description of assay formats is shown in the Fig. 11.2.
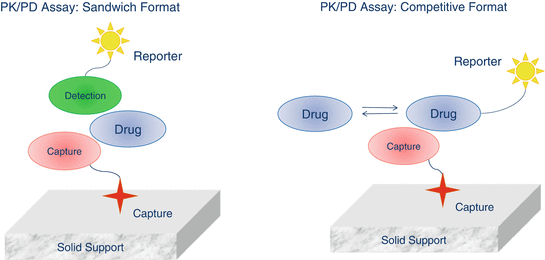
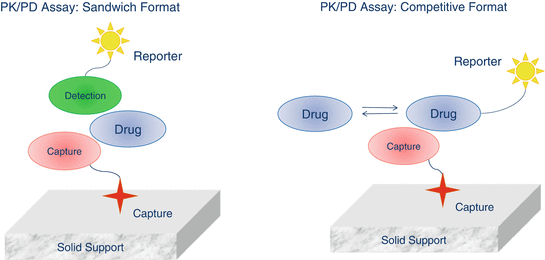
Fig. 11.2
General schematic description of ligand-binding assay platforms
Sandwich Formats
In sandwich formats, the drug is “sandwiched” between the capture and detection species. The capture species (e.g. anti-drug antibody, drug receptor) is immobilized on the solid support surface, which is incubated for a certain time with the sample to bind to the drug. The solid support is then washed to remove excess matrix components and subsequently incubated with the detection species (e.g. anti-drug antibody, drug receptor etc.). The detector is typically conjugated with a reporter capable of generating a quantifiable signal. In the earliest ligand-binding assays, the detection species carried a radioactive label such as 3H, 35S or 125I. However, radioactive reagents are now used less often due to problems with their disposal and personnel safety issues. One of the most common LBA formats is an enzyme-linked immunosorbent assay (ELISA), where the detector is conjugated with an enzyme which turns over a substrate to generate signal (e.g. optical density or light emission). The amount of the bound reporter and the intensity of generated signal are proportional to the concentration of the drug in the sample. Ligand-binding assays often utilize the extremely high affinity of streptavidin and avidin for biotin. Strept(avidin)-biotin interactions can be used to bind the capture species (labeled with biotin) to the streptavidin-coated surface. Similarly, the detection species can be labeled with biotin to capture streptavidin conjugated with the reporter.
Competitive Formats
In competitive formats, the drug present in the sample competes for the capture species with the drug labeled with reporter. The nature of interactions is the same as that in sandwich formats but the signal intensity is inversely proportional to the drug concentration with maximum signal generated by blank matrix. In competitive assays only one species is required to bind to the drug. Consequently, these assay formats are not as specific as the sandwich assays where a drug is recognized by two different binding partners.
11.1.3.2 Immunogenicity Assay Formats
The heterogeneity of immune responses poses enormous problems for the design of assay formats. Ideally, a screening assay should be capable of detecting all immunoglobulin classes (IgG, IgM, IgA, IgD and IgE). An assay should also be able to demonstrate sufficient sensitivity and have a low level of false positive classifications. To detect ADA, the drug used in an assay must be as close as possible to its native form, since addition of molecules that facilitate capture or detection of ADA (labeling with radioactive isotopes, biotinylation, ruthenylation or passive absorption on solid support) may block or distort the existing epitopes and conceivably introduce others resulting in non-specific signals. The necessity of using a drug as a reagent to detect presence of ADA means that immunogenicity assays are susceptible to interference by the circulating drug. Preferably, immunogenicity samples should be collected when the drug is washed out from circulation but this is not always feasible. A solid understanding of the advantages and limitations of an assay format can aid the design of immunogenicity assessment programs as well as interpretation of resulting data. The most common formats for immunogenicity assays are briefly described below (Fig. 11.3).
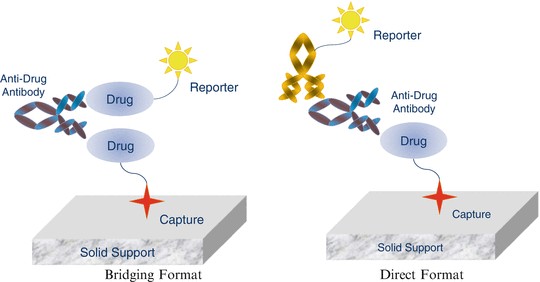
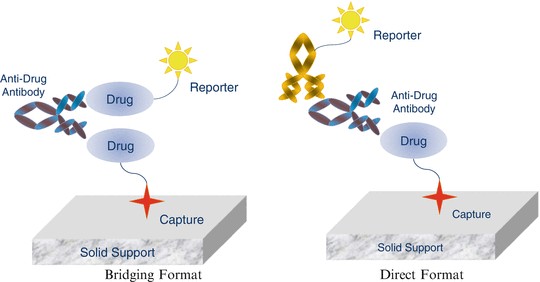
Fig. 11.3
Different ADA assay formats
Bridging Formats
Bridging assays are arguably the most common immunogenicity assay format. This format depends on the ability of ADA to bind to more than one molecule of the drug at the same time. Two different forms of the drug are needed for the assay: one that allows capture onto a solid surface and one that can serve as a reporter for generation of a signal. Upon binding of ADA with the two different forms of drug, the resulting antibody-drug complex can be captured and detected. Capture of the drug is accomplished by either passive absorption on solid support, or by labeling the drug with biotin and capturing it on streptavidin or avidin-coated surfaces. The most commonly used platforms for this type of assay are ELISA and electrochemiluminescent immunoassay (ECLIA), where the signal is measured either as optical density (ELISA) or as light emitted by a ruthenium chelate reporter (ECLIA).
Popularity of the bridging assays is due to their high sensitivity and capability to detect all immunoglobulin classes and most isotypes. These assays can be used for detection of ADA in different species which allows use of the same format for non-clinical and clinical studies. The major disadvantage of this format is that it does not detect antibodies directly; rather it detects multivalent species that are capable of binding to more than one molecule of the drug at the same time. For this reason, bridging formats can be considered as excellent screening assays with an inherent potential for generation of false positive responses.
Direct Formats
The unique characteristic of this assay format is its direct or specific detection of ADA. In contrast to the bridging assays that utilize the multivalent nature of ADA binding, the direct format explicitly detects ADA. In its simplest variant, a drug is captured (e.g. passively absorbed) onto a solid surface and allowed to bind with ADA present in the sample. In turn, ADA is detected by using an anti-IgG antibody labeled with a reporter molecule. Alternatively, the drug can be anchored to a surface via a specific monoclonal antibody or by biotin (for streptavidin-coated surfaces). As before, ADA is detected by an anti-IgG antibody conjugated with a suitable reporter. Direct formats suffer from two major draw-backs. First, they are not well suited for biotherapeutics that are monoclonal antibodies since they cannot differentiate between ADA and the drug itself. Second, due to heterogeneity of ADAs, it is difficult to detect all possible subclasses (e.g. IgG, IgM, IgE).
11.1.4 Assay Development and Validation
During assay development, appropriate assay platforms suitable for requirements of the program under development need to be determined along with various assay conditions such as: reagents, minimal required dilution (MRD), incubation times, etc. Design of experiments (DOE) is particularly useful to find the optimal combination of various assay conditions. At the completion of assay development, Standard Operating Procedure (SOP) is established with detailed description of the analytical procedure. A validation protocol specifies the assay parameters to be evaluated and sets a priori acceptance criteria that need to be met in order to demonstrate that the analytical method is fit for its intended purpose. The exact assay parameters that need to be validated together with their acceptance criteria depend on the type of assay (e.g. quantitative or semi-quantitative). Analytical challenges include, but are not limited to, analyte stability during sample handling, potential interfering factors, and cross-reactive species. At completion of the validation step, the SOP is finalized for in-study use. A detailed description of the validation experiments with results is presented in a validation report. For compliance purposes, the level of details included in the validation report should be sufficient to independently reconstruct the study at a later date.
For quantitative assays such as a PK assay, the main validation parameters are sensitivity, specificity/selectivity, linearity, dynamic range, accuracy, precision, limit of detection (LOD), limit of quantification (LOQ), stability and robustness. Some of these parameters are defined below. Readers are referred to the regulatory documents and industry white papers for more details (EMA 2011; FDA 2014b; Booth et al. 2015; DeSilva et al. 2003; Gupta et al. 2011; Kelley and DeSilva 2007; Lee et al. 2006; Miller et al. 2001; Shankar et al. 2008; USP chapter <1106> 2014).
Accuracy
Describes the closeness of the analyte concentration as determined by the analytical method to the nominal concentration. Accuracy can be determined within an analytical run (intra-assay) and across analytical runs (inter-assay).
Precision
Describes the closeness of the repeated individual measurements of the analyte (intra- and inter-assay) and is typically expressed as coefficient of variation.
Sensitivity
In bioanalytical sciences, sensitivity typically refers to the lowest analyte concentration that can be detected (limit of detection LOD) or quantified (lower limit of quantification LOQ) by a given analytical method.
Dynamic Range
Typically refers to the range of the concentrations between the lower and upper limits of quantification.
Selectivity/Specificity
Selectivity describes the ability of the analytical method to accurately detect the analyte of interest in the presence of other components of a biological matrix. Specificity can be considered as a subset of selectivity as it describes the method’s ability to detect only the analyte of interest in the presence of other similar analytes. In ligand-binding assays, the term specificity is often used interchangeably with cross-reactivity.
Stability
Describes analyte stability when exposed to different situations that may be encountered during sample handling and analytical procedures. Stability evaluation typically includes testing of multiple freeze-thaw cycles, prolonged storage at room temperature, and stability during refrigeration and freezing.
Due to the broad definition of biomarkers, PD measurements can face more challenges than PK measurements. When biomarkers are well defined (e.g. steroids) and a suitable reference standard is available, results from the PD assay are definitive and can be validated in the same way as PK assays. When the reference standard is not available in purified form, or is not representative of the endogenous forms of the biomarker, a PD assay generates continuous responses which are semi-quantitative and expressed as the intensity of the generated signal. Purely qualitative assays generate categorical data which lack proportionality to the concentration of the analyte in the sample (Lee et al. 2006).
Immunogenicity assays are a good example of semi-quantitative assays. Due to the lack of a reference standard, the results from ADA assays cannot be presented as concentrations and are typically expressed as titer or minimum dilutions that render the sample negative (below the assay cut point). Consequently, the assay cut points, and especially the screening cut point is a critical assay parameter. Although many assay parameters have the same meaning as those for quantitative assays, some are different. For example, the assay sensitivity is defined as the lowest concentration at which the control antibody preparation consistently produces a positive result, which is closely related to the cut point. Accuracy is typically defined as the portion of true classifications (positive and negative) from all classifications of quality control samples.
The rest of this chapter is organized as follows. Section 11.2 introduces the statistical aspects of PK assay development and validation with the focus on calibration curve, accuracy and precision as well as incurred sample reanalysis. Section 11.3 describes the various statistical methods for determination of a cut point, including recent developments.
11.2 PK/PD Assay Development and Validation
In this section, we focus on PK/PD assay development and validation. These aspects are guided scientifically by regulatory guidance such as EMA (2011) and FDA (2014b) as well as industry white papers by DeSilva et al. (2003) and Booth et al. (2015). Lee et al. (2006) covers PD/biomarker assays. Booth et al. (2015), DeSilva et al. (2003), Kelley and Desilva (2007), DeSilva and Bowsher (2010) cover many aspects of LBA assays with technical details including assay reagent selection, reference material, stability, specificity, selectivity, calibration curve, accuracy, precision and many others. These are recommended sources for practitioners.
For quantitative PK or PD assays, a typical analytical run consists of calibration standards, matrix blank (processed matrix sample without the analyte), a set of appropriate quality controls, and finally, the study samples to be analyzed. Calibration standards are prepared by spiking a known amount of the analyte into an appropriate matrix to generate a concentration-response relationship from which the concentrations of the study samples can be interpolated. The blank matrix sample shows the response of the processed matrix which ideally should be below that of the lowest calibration standard. In cases where an endogenous analyte is present in the sample, its response may be subtracted from the responses of the calibration standards. The quality control samples consist of an analyte spiked into the assay matrix at the low, medium and high levels of the quantitative range of the assay. Additional quality controls may be prepared at the LLOQ and ULOQ (Lower and Upper Limits of Quantification, respectively). The concentrations of the quality control samples are interpolated from the calibration curve and compared with their respective nominal concentrations. Preferably, the quality control samples should be treated in the same way as the study samples and mimic them in every respect (storage, processing and treatment in the bioanalytical assay). In order for an analytical run to be accepted and results from the unknown samples reported, the calibration standards and quality controls must meet a set of pre-defined acceptance criteria. During analytical method validation, quality control samples are used to determine accuracy and precision.
11.2.1 Calibration Curve
A calibration curve is the description of the relationship between signal from the analyte of interest and its concentration, and is used to measure unknown concentrations of study samples given their signal readings. The curve is prepared by utilizing reference material where known concentrations of analyte are spiked into an assay matrix. The concentration of individual calibration points are established during method development, confirmed during the validation stage, and used for in-study sample testing. The calibration curves are needed for all PK and PD (biomarker) measurements, when well characterized reference standards are available. The concentration-response relationship in ligand binding assays (LBA) is well known to have a nonlinear mean response and non-constant variance. DeSilva et al. (2003), DeSilva and Bowsher (2010) and Boulanger et al. (2010) have described how to establish calibration curves in general. They recommend that during method development, the number of standard points and replicates should be large enough to allow the selection of the most appropriate calibration model; see Table 3 of DeSilva et al. (2003) for the design of calibration experiments for the different stages of method validation. A more statistically rigorous method is to apply design of experiment (DOE) method.
Several publications such as DeSilva et al. (2003) and DeSilva and Bowsher (2010) provide details relating to finding and fitting appropriate calibration curves without too many statistical details. The basic calibration curve is a four-parameter logistic model which has the following form:
where yj is the j-th observed response at concentration xj and b is the slope parameter, a is the response at zero concentration and d is the response at an infinite concentration with b > 0, c is the inflection point around which the mean calibration curve is symmetric; it is the concentration corresponding to the mean response halfway between a and d. If d > a, then the concentration-response relationship is increasing; when a > d, the relationship is decreasing. Sometimes, the parameters a and d have alternative interpretations. In this case, a is the maximal response and d is a minimal response; when b>0, the concentration-response relationship is decreasing and vise-versa when b < 0. The four-parameter logistic model is believed to be valid for competitive assays while a more general five-parameter logistic model better characterizes the concentration-response relationship of non-competitive assays. The five-parameter logistic model has the following form:
This model allows for an asymmetric concentration-response curve by adding an additional parameter g, which effectively allows the mean response function to approach the minimal or maximal response at a different rate (Findlay and Dillard 2007). In both of these models, εj is the intra-assay error term.
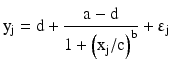
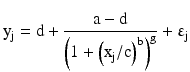
For the above calibration models, the variance of the error term is often not constant but changes across the concentration range. This phenomenon is known as heteroscedasticity. Taking into account this variance heteroscedasticity generally can improve the overall quality of model fitting as well as the accuracy and precision of the resulting calibration, as emphasized by Findlay and Dillard (2007). For a regression model with constant variance, a least-squares method is often adopted. To account for the variance heteroscedasticity, a weighted least-squares method can be used for parameter estimation. The idea is to place less weight on responses with higher variability. Mathematically, consider the following general regression problem:
The weighted least-squares method is to find the estimation of β by minimizing:
Instead of using the standard deviation at each concentration as weights, which is discouraged especially when the number of replicates is small, various weighting function forms are proposed. O’Connell et al. (1993), for example, considers a specific type of weighting function which works well empirically for immunoassay calibration curves. Specifically, they consider the following functional form:
with
. The variance at concentration xj is a power function of the mean response. Essentially, the task of model fitting during the development stage is to find the best appropriate calibration curve with an appropriate weighting function. Computationally, however, the model fitting procedure is much more complex than that of linear models. In the remainder of this section, we will discuss the statistical details of model building for non-linear calibration models.
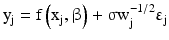
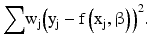
![$$ {\mathrm{y}}_{\mathrm{j}}=\mathrm{f}\left({\mathrm{x}}_{\mathrm{j}},\upbeta \right)+\upsigma \mathrm{g}\left[\mathrm{f}\left({\mathrm{x}}_{\mathrm{j}},\upbeta \right)\right]{\upvarepsilon}_{\mathrm{j}} $$](/wp-content/uploads/2016/07/A330233_1_En_11_Chapter_Eque.gif)
![$$ \mathrm{g}\left[\mathrm{f}\left({\mathrm{x}}_{\mathrm{j}},\upbeta \right)\right]={\left(\mathrm{f}\left({\mathrm{x}}_{\mathrm{j}},\upbeta \right)\right)}^{\uptheta} $$](/wp-content/uploads/2016/07/A330233_1_En_11_Chapter_IEq1.gif)
11.2.1.1 Calibration from a Single Run
The parameter estimation of a nonlinear calibration curve with non-constant variance is not trivial. Unlike the linear regression model, there is no closed-form solution and iteration is often needed. Here, we introduce the approach proposed in O’Connell et al. (1993) and Davidian and Giltinan (1995). A more systematic exposition of the non-linear regression model in general can be found in Seber and Wild (1989) and Bates and Watts (1988).
A Generalized Least Squares (GLS) with variance function estimation is preferred since it does not assume distribution of the error term and is relatively robust to distribution misspecification. When the error term is assumed to be normally distributed, the GLS estimation is the same as the maximum likelihood estimation. Since there is no closed-form solution for the parameter estimations, numerical iteration is warranted. The GLS estimation starts with an initial estimator of β, usually the un-weighted least squares estimator. The variance parameters are estimated by utilizing the relationship between the residuals from the least squares regression and the concentration, using the likelihood method. The resultant weights are then used to obtain an updated weighted least-squares estimate of β. This procedure repeats until a convergence criterion is met. Mathematical details can be found in Appendix.
< div class='tao-gold-member'>
Only gold members can continue reading. Log In or Register a > to continue
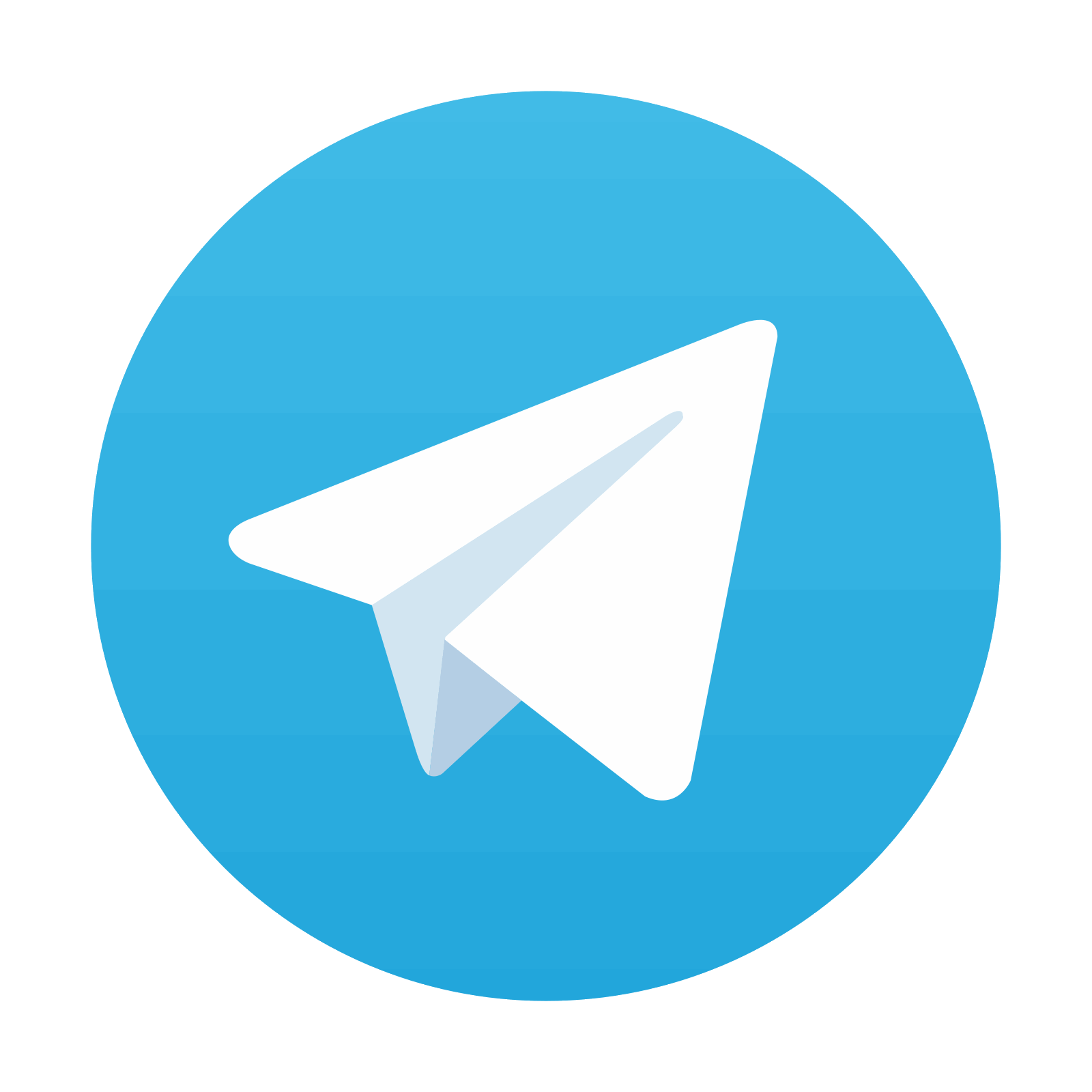
Stay updated, free articles. Join our Telegram channel

Full access? Get Clinical Tree
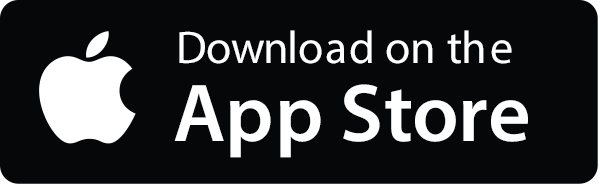
