Key Concepts
Probability is an important concept in statistics. Both objective and subjective probabilities are used in the medical field.
Basic definitions include the concept of an event or outcome. A number of essential rules tell us how to combine the probabilities of events.
Bayes’ theorem relates to the concept of conditional probability—the probability of an outcome depending on an earlier outcome. Bayes’ theorem is part of the reasoning process when interpreting diagnostic procedures.
Populations are rarely studied; instead, researchers study samples.
Several methods of sampling are used in medical research; a key issue is that any method should be random.
When researchers select random samples and then make measurements, the result is a random variable. This process makes statistical tests and inferences possible.
The binomial distribution is used to determine the probability of yes/no events—the number of times a given outcome occurs in a given number of attempts.
The Poisson distribution is used to determine the probability of rare events.
The normal distribution is used to find the probability that an outcome occurs when the observations have a bell-shaped distribution. It is used in many statistical procedures.
If many random samples are drawn from a population, a statistic, such as the mean, follows a distribution called a sampling distribution.
The central limit theorem tells us that means of observations, regardless of how they are distributed, begin to follow a normal distribution as the sample size increases. This is one of the reasons the normal distribution is so important in statistics.
It is important to know the difference between the standard deviation, which describes the spread of individual observations, from the standard error of the mean, which describes the spread of the mean observations.
One of the purposes of statistics is to use a sample to estimate something about the population. Estimates form the basis of statistical tests.
Confidence intervals can be formed around an estimate to tell us how much the estimate would vary in repeated samples.
Presenting Problems
Neisseria meningitidis, a gram-negative diplococcus, has as its natural reservoir the human posterior nasopharynx where it can be cultured from 2–15% of healthy individuals during nonepidemic periods. The bacterial organism can be typed into at least 13 serogroups based on capsular antigens. These serogroups can be further subdivided by antibodies to specific subcapsular membrane proteins. In the United States, sero groups B and C have accounted for 90% of meningococcal meningitis cases in recent decades. The major manifestations of meningo coccal disease are acute septicemia and purulent meningitis. The age-specific attack rate is greatest for children under 5 years of age.
Epidemiologic surveillance data from the state of Oregon detected an increase in the overall incidence rate of meningococcal disease from 2 cases per 100,000 population during 1987–1992 to 4.5 cases per 100,000 population in 1994 (Diermayer et al, 1999). Epidemiologists from Oregon and the Centers for Disease Control wanted to know if the increased numbers of cases of meningococcal disease were indications of a transition from endemic to epidemic disease. The investigators found a significant rise in serogroup B disease; they also discovered that most of the isolates belonged to the ET-5 clonal strain of this serogroup. In addition, a shift toward disease in older age groups, especially 15- through 19-year-olds, was observed.
Information from the study is given in the section titled, “Basic Definitions and Rules of Probability.” We use these data to illustrate basic concepts of probability and to demonstrate the relationship between time period during the epidemic and site of infection.
A local blood bank was asked to provide information on the distribution of blood types among males and females. This information is useful in illustrating some basic principles in probability theory. The results are given in “Basic Definitions and Rules of Probability.”
In the United States, prostate cancer is the second leading cause of death among men who die of neoplasia, accounting for 12.3% of cancer deaths. Controversial management issues include when to treat a patient with radical prostatectomy and when to use definitive radiation therapy. Radical prostatectomy is associated with a high incidence of impotence and occasional urinary incontinence. Radiation therapy produces less impotence but can cause radiation cystitis, proctitis, and dermatitis. Prostate specific antigen (PSA) evaluation, available since 1988, leads to early detection of prostate cancer and of recurrence following treatment and may be a valuable prognostic indicator and measure of tumor control after treatment.
Although radical radiation therapy is used to treat prostate cancer in about 60,000 men each year, only a small number of these men from any single institution have had a follow-up of more than 5 years during the era when the PSA test has been available. Shipley and colleagues (1999) wanted to assess the cancer control rates for men treated with external beam radiation therapy alone by pooling data on 1765 men with clinically localized prostate cancer treated at six institutions. The PSA value, along with the Gleason score (a histologic scoring system in which a low score indicates well-differentiated tumor and a high score poorly differentiated tumor) and tumor palpation state, was used to assess pretreatment prognostic factors in the retrospective, nonrandomized, multiinstitutional pooled analysis. A primary treatment outcome was the measurement of survival free from biochemical recurrence. Biochemical recurrence was defined as three consecutive rises in PSA values or any rise great enough to trigger additional treatment with androgen suppression.
Prognostic indicators including pretreatment PSA values indicate the probability of success of treatment with external beam radiation therapy for subsets of patients with prostate cancer. The probabilities of 5-year survival in men with given levels of pretreatment PSA are given in the section titled, “Sampling Distributions.” We use these rates to illustrate the binomial probability distribution.
The Coronary Artery Surgery Study was a classic study in 1983; it was a prospective, randomized, multicenter collaborative trial of medical and surgical therapy in subsets of patients with stable ischemic heart disease. This classic study established that the 10-year survival rate in this group of patients was equally good in the medically treated and surgically (coronary revascularization) treated groups (Alderman et al, 1990). A second part of the study compared the effects of medical and surgical treatment on the quality of life.
Over a 5-year period, 780 patients with stable ischemic heart disease were subdivided into three clinical subsets (groups A, B, and C). Patients within each subset were randomly assigned to either medical or surgical treatment. All patients enrolled had 50% or greater stenosis of the left main coronary artery or 70% or greater stenosis of the other operable vessels. In addition, group A had mild angina and an ejection fraction of at least 50%; group B had mild angina and an ejection fraction less than 50%; group C had no angina after myocardial infarction. History, examination, and treadmill testing were done at 6, 18, and 60 months; a follow-up questionnaire was completed at 6-month intervals. Quality of life was evaluated by assessing chest pain status; heart failure; activity limitation; employment status; recreational status; drug therapy; number of hospitalizations; and risk factor alteration, such as smoking status, BP control, and cholesterol level. Data on number of hospitalizations after mean follow-up of 11 years will be used to illustrate the Poisson probability distribution (Rogers et al, 1990).
An individual’s BP has important health implications; hypertension is among the most commonly treated chronic medical problems. To examine variation in BP, Marczak and Paprocki (2001) found the mean and standard deviation in a group of healthy persons. For men and women between the ages of 14 and 70, mean 24-h systolic pressure was 119.7 mm Hg, and the standard deviation was 10.9. We use this information to calculate probabilities of any patient having a given BP.
Purpose of the Chapter
The previous chapter presented methods for summarizing information from studies: graphs, plots, and summary statistics. A major reason for performing clinical research, however, is to generalize the findings from the set of observations on one group of subjects to others who are similar to those subjects. Shipley and colleagues (1999) concluded that the initial level of PSA can be used to estimate freedom from biochemical recurrence of tumor. This conclusion was based on their study and follow-up for at least 5 years of 448 men from six institutions. Studying all patients in the world with T1b, T1c, or T2 tumors (but unknown nodal status) is neither possible nor desirable; therefore, the investigators made inferences to a larger population of patients on the basis of their study of a sample of patients. They cannot be sure that men with a specific level of pretreatment PSA will respond to treatment as the average man did in this study, but they can use the data to find the probability of a positive response.
The concepts in this chapter will enable you to understand what investigators mean when they make statements like the following:
The difference between treatment and control groups was tested by using a t test and found to be significantly greater than zero.
An α value of 0.01 was used for all statistical tests.
The sample sizes were determined to give 90% power of detecting a difference of 30% between treatment and control groups.
Our experience indicates that the concepts underlying statistical inference are not easily absorbed in a first reading. We suggest that you read this chapter and become acquainted with the basic concepts and then, after completing Chapters 5, 6, 7, 8, and 9, read it again. It should be easier to understand the basic ideas of inference using this approach.
The Meaning of the Term “Probability”
Assume that an experiment can be repeated many times, with each replication (repetition) called a trial and assume that one or more outcomes can result from each trial. Then, the probability of a given outcome is the number of times that outcome occurs divided by the total number of trials. If the outcome is sure to occur, it has a probability of 1; if an outcome cannot occur, its probability is 0.
An estimate of probability may be determined empirically, or it may be based on a theoretical model. We know that the probability of flipping a fair coin and getting tails is 0.50, or 50%. If a coin is flipped ten times, there is no guarantee, of course, that exactly five tails will be observed; the proportion of tails can range from 0 to 1, although in most cases we expect it to be closer to 0.50 than to 0 or 1. If the coin is flipped 100 times, the chances are even better that the proportion of tails will be close to 0.50, and with 1000 flips, the chances are better still. As the number of flips becomes larger, the proportion of coin flips that result in tails approaches 0.50; therefore, the probability of tails on any one flip is 0.50.
This definition of probability is sometimes called objective probability, as opposed to subjective probability, which reflects a person’s opinion, hunch, or best guess about whether an outcome will occur. Subjective probabilities are important in medicine because they form the basis of a physician’s opinion about whether a patient has a specific disease. In Chapter 12 we discuss how this estimate, based on information gained in the history and physical examination, changes as the result of diagnostic procedures. Steiner (1999) discusses the way physicians use probability in speaking to patients, and Goodman (1999) discusses interesting aspects of the history of probability in an accompanying editorial.
Probability concepts are helpful for understanding and interpreting data presented in tables and graphs in published articles. In addition, the concept of probability lets us make statements about how much confidence we have in such estimates as means, proportions, or relative risks (introduced in the previous chapter). Understanding probability is essential for understanding the meaning of P values given in journal articles.
We use two examples to illustrate some definitions and rules for determining probabilities: Presenting Problem 1 on meningococcal disease (Table 4–1) and the information given in Table 4–2 on gender and blood type. All illustrations of probability assume the observation has been randomly selected from a population of observations. We discuss these concepts in more detail in the next section.
Time Period | |||||||
---|---|---|---|---|---|---|---|
Preepidemic 1987–1992 | Early Epidemic 1993–1994 | Recent Epidemic 1995–1996 | |||||
Count | Column % | Count | Column % | Count | Column % | ||
Sex | Men | 75 | 50 | 59 | 50 | 75 | 53 |
Women | 75 | 50 | 58 | 50 | 66 | 47 | |
Race | White | 120 | 80 | 94 | 80 | 110 | 78 |
African American | 5 | 3 | 0 | 0 | 1 | 1 | |
Hispanic | 2 | 1 | 10 | 9 | 11 | 8 | |
Native American | 2 | 1 | 2 | 2 | 0 | 0 | |
Asian | 0 | 0 | 0 | 0 | 2 | 1 | |
Unknown | 21 | 14 | 11 | 9 | 17 | 12 | |
Site of infection | Sepsis | 66 | 44 | 45 | 38 | 40 | 28 |
Meningitis | 39 | 26 | 32 | 27 | 39 | 28 | |
Both | 39 | 26 | 32 | 27 | 34 | 24 | |
Unknown | 6 | 4 | 8 | 7 | 28 | 20 | |
Died during epidemic | No | 141 | 94 | 108 | 92 | 132 | 94 |
Yes | 9 | 6 | 9 | 8 | 9 | 6 |
In probability, an experiment is defined as any planned process of data collection. For Presenting Problem 1, the experiment is the process of determining the site of infection in patients with meningococcal disease. An experiment consists of a number of independent trials (replications) under the same conditions; in this example, a trial consists of determining the site of infection for an individual person. Each trial can result in one of four outcomes: sepsis, meningitis, both sepsis and meningitis, or unknown.
The probability of a particular outcome, say outcome A, is written P(A). The data from Table 4–1 have been condensed into a table on the site of infection with total numbers and are given in Table 4–3. For example, in Table 4–3, if outcome A is sepsis, the probability that a randomly selected person from the study has meningitis without sepsis as the site of infection is
Time Period | |||||
---|---|---|---|---|---|
Preepidemic 1987–1992 | Early Epidemic 1993–1994 | Recent Epidemic 1995–1996 | Total | ||
Site of infection | Sepsis | 66 | 45 | 40 | 151 |
Meningitis | 39 | 32 | 39 | 110 | |
Both | 39 | 32 | 34 | 105 | |
Unknown | 6 | 8 | 28 | 42 | |
Total | 150 | 117 | 141 | 408 |
In Presenting Problem 2, the probabilities of different outcomes are already computed. The outcomes of each trial to determine blood type are O, A, B, and AB. From Table 4–2, the probability that a randomly selected person has type A blood is
The blood type data illustrate two important features of probability:
1. The probability of each outcome (blood type) is greater than or equal to 0.
- 2. The sum of the probabilities of the various outcomes is 1.
Events may be defined either as a single outcome or a set of outcomes. For example, the outcomes for the site of infection in the meningitis study are sepsis, meningitis, both, or unknown, but we may wish to define an event as having known meningitis versus not having known meningitis. The event of known meningitis contains the two outcomes of meningitis alone plus both (meningitis and sepsis), and the event of not having known meningitis also contains two outcomes (sepsis and unknown).
Sometimes, we want to know the probability that an event will not happen; an event opposite to the event of interest is called a complementary event. For example, the complementary event to “having known meningitis” is “not having known meningitis.” The probability of the complement is
Note that the probability of a complementary event may also be found as 1 minus the probability of the event itself, and this calculation may be easier in some situations. To illustrate,
Two or more events are mutually exclusive if the occurrence of one precludes the occurrence of the others. For example, a person cannot have both blood type O and blood type A. By definition, all complementary events are also mutually exclusive; however, events can be mutually exclusive without being complementary if three or more events are possible.
As we indicated earlier, what constitutes an event is a matter of definition. Let us define the experiment in Presenting Problem 2 so that each outcome (blood type O, A, B, or AB) is a separate event. The probability of two mutually exclusive events occurring is the probability that either one event occurs or the other event occurs. This probability is found by adding the probabilities of the two events, which is called the addition rule for probabilities. For example, the probability that a randomly selected person has either blood type O or blood type A is
Does the addition rule work for more than two events? The answer is yes, as long as they are all mutually exclusive. We discuss the approach to use with nonmutually exclusive events in the section titled, “Nonmutually Exclusive Events and the Modified Addition Rule.”
Two different events are independent events if the outcome of one event has no effect on the outcome of the second. Using the blood type example, let us also define a second event as the gender of the person; this event consists of the outcomes male and female. In this example, gender and blood type are independent events; the sex of a person does not affect the person’s blood type, and vice versa. The probability of two independent events is the probability that both events occur and is found by multiplying the probabilities of the two events, which is called the multiplication rule for probabilities. The probability of being male and of having blood type O is
The probability of being male, 0.50, and the probability of having blood type O, 0.42, are both called marginal probabilitiesbecause they appear on the margins of a probability table. The probability of being male and of having blood type O, 0.21, is called a joint probability; it is the probability of both male and type O occurring jointly.
Is having an unknown site of infection independent from the time period of the epidemic in the Diermayer study? Table 4–3 gives the data we need to answer to this question. If two events are independent, the product of the marginal probabilities will equal the joint probability in all instances. To show that two events are not independent, we need demonstrate only one instance in which the product of the marginal probabilities is not equal to the joint probability. For example, to show that having an unknown site of infection and pre-epidemic period are not independent, find the joint probability of a randomly selected person having an unknown site and being diagnosed in the pre-epidemic period. Table 4–3 shows that
However, the product of the marginal probabilities does not yield the same result; that is,
We could show that the product of the marginal probabilities is not equal to the joint probability for any of the combinations in this example, but we need show only one instance to prove that two events are not independent.
Finding the joint probability of two events when they are not independent is a bit more complex than simply multiplying the two marginal probabilities. When two events are not independent, the occurrence of one event depends on whether the other event has occurred. Let A stand for the event “known meningitis” and B for the event “recent epidemic” (in which known meningitis is having either meningitis alone or meningitis with sepsis). We want to know the probability of event A given event B, written P(A | B) where the vertical line, |, is read as “given.” In other words, we want to know the probability of event A, assuming that event B has happened. From the data in Table 4–3, the probability of known meningitis, given that the period of interest is the recent epidemic, is
This probability, called a conditional probability, is the probability of one event given that another event has occurred. Put another way, the probability of a patient having known meningitis is conditional on the period of the epidemic; it is substituted for P(known meningitis) in the multiplication rule. If we put these expressions together, we can find the joint probability of having known meningitis and contracting the disease in the recent epidemic:
The probability of having known meningitis during the recent epidemic can also be determined by finding the conditional probability of contracting the disease during the recent epidemic period, given known meningitis, and substituting that expression in the multiplication rule for P(recent epidemic). To illustrate,
Remember that two or more mutually exclusive events cannot occur together, and the addition rule applies for the calculation of the probability that one or another of the events occurs. Now we find the probability that either of two events occurs when they are not mutually exclusive. For example, gender and blood type O are nonmutually exclusive events because the occurrence of one does not preclude the occurrence of the other. The addition rule must be modified in this situation; otherwise, the probability that both events occur will be added into the calculation twice.
In Table 4–2, the probability of being male is 0.50 and the probability of blood type O is 0.42. The probability of being male or of having blood type O is not 0.50 + 0.42, however, because in this sum, males with type O blood have been counted twice. The joint probability of being male and having blood type O, 0.21, must therefore be subtracted. The calculation is
Of course, if we do not know that P(male and type O) = 0.21, we must use the multiplication rule (for independent events, in this case) to determine this probability.
Let us summarize the rules presented thus far so we can extend them to obtain a particularly useful rule for combining probabilities called Bayes’ theorem. Remember that questions about mutual exclusiveness use the word “or” and the addition rule; questions about independence use the word “and” and the multiplication rule. We use letters to represent events; A, B, C, and D are four different events with probability P(A), P(B), P(C), and P(D).
The addition rule for the occurrence of either of two or more events is as follows: If A, B, and C are mutually exclusive, then
If two events such as A and D are not mutually exclusive, then
aThe probability of three or more events that are not mutually exclusive or not independent involves complex calculations beyond the scope of this book. Interested readers can consult any introductory book on probability.
The multiplication rule for the occurrence of both of two or more events is as follows: If A, B, and C are independent, then
If two events such as B and D are not independent, then
The multiplication rule for probabilities when events are not independent can be used to derive one form of an important formula called Bayes’ theorem. Because P(B and D) equals both P(B | D) × P(D) and P(B) × P(D | B), these latter two expressions are equal. Assuming P(B) and P(D) are not equal to zero, we can solve for one in terms of the other, as follows:
which is found by dividing both sides of the equation by P(D). Similarly,
In the equation for P(B | D), P(B) in the right-hand side of the equation is sometimes called the prior probability, because its value is known prior to the calculation; P(B | D) is called the posterior probability, because its value is known only after the calculation.
The two formulas of Bayes’ theorem are important because investigators frequently know only one of the pertinent probabilities and must determine the other. Examples are diagnosis and management, discussed in detail in Chapter 12.
Although in everyday use the terms probability, odds, and likelihood are sometimes used synonymously, mathematicians do not use them that way. Odds is defined as the probability that an event occurs divided by the probability the event does not occur. For example, the odds that a person has blood type O are 0.42/ (1 – 0.42) = 0.72 to 1, but “to 1” is not always stated explicitly. This interpretation is consistent with the meaning of the odds ratio, discussed in Chapter 3. It is also consistent with the use of odds in gaming events such as football games and horse races.
Likelihood may be related to Bayes’ theorem for conditional probabilities. Suppose a physician is trying to determine which of three likely diseases a patient has: myocardial infarction, pneumonia, or reflux esophagitis. Chest pain can appear with any one of these three diseases; and the physician needs to know the probability that chest pain occurs with myocardial infarction, the probability that chest pain occurs with pneumonia, and the probability that chest pain occurs with reflux esophagitis. The probabilities of a given outcome (chest pain) when evaluated under different hypotheses (myocardial infarction, pneumonia, and reflux esophagitis) are called likelihoods of the hypotheses (or diseases).
Populations & Samples
A major purpose of doing research is to infer, or generalize, from a sample to a larger population. This process of inference is accomplished by using statistical methods based on probability. Population is the term statisticians use to describe a large set or collection of items that have something in common. In the health field, population generally refers to patients or other living organisms, but the term can also be used to denote collections of inanimate objects, such as sets of autopsy reports, hospital charges, or birth certificates. A sample is a subset of the population, selected so as to be representative of the larger population.
There are many good reasons for studying a sample instead of an entire population, and the four commonly used methods for selecting a sample are discussed in this section. Before turning to those topics, however, we note that the term “population” is frequently misused to describe what is, in fact, a sample. For example, researchers sometimes refer to the “population of patients in this study.” After you have read this book, you will be able to spot such errors when you see them in the medical literature. If you want more information, Levy and Lemeshow (1999) provide a comprehensive treatment of sampling.
There are at least six reasons to study samples instead of populations:
1. Samples can be studied more quickly than populations. Speed can be important if a physician needs to determine something quickly, such as a vaccine or treatment for a new disease.
2. A study of a sample is less expensive than studying an entire population, because a smaller number of items or subjects are examined. This consideration is especially important in the design of large studies that require a lengthy follow-up.
3. A study of an entire population (census) is impossible in most situations. Sometimes, the process of the study destroys or depletes the item being studied. For example, in a study of cartilage healing in limbs of rats after 6 weeks of limb immobilization, the animals may be sacrificed in order to perform histologic studies. On other occasions, the desire is to infer to future events, such as the study of men with prostate cancer. In these cases, a study of a population is impossible.
4. Sample results are often more accurate than results based on a population. For samples, more time and resources can be spent on training the people who perform observations and collect data. In addition, more expensive procedures that improve accuracy can be used for a sample because fewer procedures are required.
5. If samples are properly selected, probability methods can be used to estimate the error in the resulting statistics. It is this aspect of sampling that permits investigators to make probability statements about observations in a study.
6. Samples can be selected to reduce heterogeneity. For example, systemic lupus erythematosus (SLE) has many clinical manifestations, resulting in a heterogeneous population. A sample of the population with specified characteristics is more appropriate than the entire population for the study of certain aspects of the disease.
To summarize, bigger does not always mean better in terms of sample sizes. Thus, investigators must plan the sample size appropriate for their study prior to beginning research. This process is called determining the power of a study and is discussed in detail in later chapters. See Abramson (1999) for an introductory discussion of sampling.
The best way to ensure that a sample will lead to reliable and valid inferences is to use probability samples, in which the probability of being included in the sample is known for each subject in the population. Four commonly used probability sampling methods in medicine are simple random sampling, systematic sampling, stratified sampling, and cluster sampling, all of which use random processes.
The following example illustrates each method: Consider a physician applying for a grant for a study that involves measuring the tracheal diameter on radio graphs. The physician wants to convince the granting agency that these measurements are reliable. To estimate intrarater reliability, the physician will select a sample of chest x-ray films from those performed during the previous year, remeasure the tracheal diameter, and compare the new measurement with the original one on file in the patient’s chart. The physician has a population of 3400 radiographs, and we assume that the physician has learned that a sample of 200 films is sufficient to provide an accurate estimate of intrarater reliability. Now the physician must select the sample for the reliability study.
A simple random sample is one in which every subject (every film in the example) has an equal probability of being selected for the study. The recommended way to select a simple random sample is to use a table of random numbers or a computer-generated list of random numbers. For this approach, each x-ray film must have an identification (ID) number, and a list of ID numbers, called a sampling frame, must be available. For the sake of simplicity, assume that the radiographs are numbered from 1 to 3400. Using a random number table, after first identifying a starting place in the table at random, the physician can select the first 200 digits between 1 and 3400. The x-ray films with the ID numbers corresponding to 200 random numbers make up the simple random sample. If a computer-generated list of random numbers is available, the physician can request 200 numbers between 1 and 3400. To illustrate the process with a random number table, a portion of Table A–1 in Appendix A is reproduced as Table 4–4. One way to select a starting point is by tossing a die to select a row and a column at random. Tossing a die twice determines, first, which block of rows and, second, which individual row within the block contains our number. For example, if we throw a 2 and a 3, we begin in the second block down, third row, beginning with the number 83. (If, on our second throw, we had thrown a 6, we would toss the die again, because there are only five rows.) Now, we must select a beginning column at random, again by tossing the die twice to select a block and a column within the block. For example, if we toss a 3 and a 1, we use the third block (across) of columns and the first column, headed by the number 1. The starting point in this example is therefore located where the row beginning with 83 and the column beginning with 1 intersect at the number 6 (in bold type in Table 4–4).
9 2 7 4 1 5 | 9 5 6 1 2 1 | 1 6 8 1 1 7 | 1 6 9 2 8 0 | 3 2 6 5 6 9 | 2 6 6 5 4 1 |
9 2 6 9 3 7 | 5 1 5 1 0 7 | 0 1 4 6 5 8 | 1 5 9 9 4 4 | 8 2 1 1 1 5 | 3 1 7 5 9 2 |
8 6 7 1 6 9 | 3 8 8 3 4 2 | 8 3 2 2 6 1 | 9 9 3 0 5 0 | 6 3 9 4 1 0 | 6 9 8 9 6 9 |
8 6 7 1 6 9 | 5 4 2 7 4 7 | 0 3 2 6 8 3 | 1 3 1 1 8 8 | 9 2 6 1 9 8 | 3 7 1 0 7 1 |
5 1 2 5 0 0 | 8 4 3 3 8 4 | 0 8 5 3 6 1 | 3 9 8 4 8 8 | 7 7 4 7 6 7 | 3 8 3 8 3 7 |
0 6 2 4 5 4 | 4 2 3 0 5 0 | 6 7 0 8 8 4 | 8 4 0 9 4 0 | 8 4 5 8 3 9 | 9 7 9 6 6 2 |
8 0 6 7 0 2 | 8 8 1 3 0 9 | 7 7 2 9 7 7 | 3 6 7 5 0 6 | 7 2 9 8 5 0 | 4 5 7 7 5 8 |
8 3 7 8 1 5 | 1 6 3 6 3 1 | 6 2 2 1 4 3 | 9 3 8 2 7 8 | 2 3 1 3 0 5 | 2 1 9 7 3 7 |
9 2 6 8 3 9 | 4 5 3 8 5 3 | 7 6 7 8 2 5 | 2 8 4 7 1 6 | 9 1 6 1 8 2 | 4 6 7 1 1 3 |
8 5 4 8 1 3 | 7 3 1 6 2 0 | 9 7 8 1 0 0 | 5 8 9 5 1 2 | 1 4 7 6 9 4 | 3 8 9 1 8 0 |
8 5 1 5 9 5 | 4 5 2 4 5 4 | 2 6 2 4 4 8 | 6 8 8 9 9 0 | 4 6 1 7 7 7 | 6 4 7 4 8 7 |
4 4 9 3 5 3 | 5 5 6 6 9 5 | 8 0 6 0 5 0 | 1 2 3 7 5 4 | 7 2 2 0 7 0 | 9 3 5 9 1 6 |
1 6 9 1 1 6 | 5 8 6 8 6 5 | 7 5 6 2 3 1 | 4 6 9 2 8 1 | 2 5 8 7 3 7 | 9 8 9 4 5 0 |
1 3 9 4 7 0 | 3 5 8 0 9 5 | 5 2 8 8 5 8 | 6 6 0 1 2 8 | 3 4 2 0 7 2 | 6 8 1 2 0 3 |
4 3 3 7 7 5 | 7 6 1 8 6 1 | 1 0 7 1 9 1 | 5 1 5 9 6 0 | 7 5 9 0 5 6 | 1 5 0 3 3 6 |
2 2 1 9 2 2 | 2 3 2 6 2 4 | 3 9 8 8 3 9 | 4 9 5 0 0 4 | 8 8 1 9 7 0 | 7 9 2 0 0 1 |
7 4 0 2 0 7 | 0 7 8 0 4 8 | 8 5 4 9 2 8 | 8 7 5 5 5 9 | 2 4 6 2 8 8 | 0 0 0 1 4 4 |
5 2 5 8 7 3 | 7 5 5 9 9 8 | 8 6 6 0 3 4 | 4 4 4 9 3 3 | 7 8 5 9 4 4 | 0 1 8 0 1 6 |
7 3 4 1 8 5 | 4 9 9 7 1 1 | 2 5 4 2 5 6 | 6 1 6 6 2 5 | 2 4 3 0 4 5 | 2 5 1 9 3 8 |
7 7 3 1 1 2 | 4 6 3 8 5 7 | 7 8 1 9 8 3 | 0 7 8 1 8 4 | 3 8 0 7 5 2 | 4 9 2 2 1 5 |
Because there are 3400 radiographs, we must read four-digit numbers; the first ten numbers are 6221, 7678, 9781, 2624, 8060, 7562, 5288, 1071, 3988, and 8549. The numbers less than 3401 are the IDs of the films to be used in the sample. In the first ten numbers selected, only two are less than 3401; so we use films with the ID numbers 2624 and 1071. This procedure continues until we have selected 200 radiographs. When the number in the bottom row (7819) is reached, we go to the top of that same column and move one digit to the right for numbers 6811, 1465, 3226, and so on.
If a number less than 3401 occurs twice, the x-ray film with that ID number can be selected for the sample and used in the study a second time (called sampling with replacement). In this case, the final sample of 200 will be 200 measurements rather than 200 ra dio graphs. Frequently, however, when a number occurs twice, it is ignored the second time and the next eligible number is used instead (called sampling without replacement). The differences between these two procedures are negligible when we sample from a large population.
A systematic random sample is one in which every kth item is selected; k is determined by dividing the number of items in the sampling frame by the desired sample size. For example, 3400 radiographs divided by 200 is 17, so every 17th x-ray film is sampled. In this approach, we must select a number randomly between 1 and 17 first, and we then select every 17th film. Suppose we randomly select the number 12 from a random number table. Then, the systematic sample consists of radiographs with ID numbers 12, 29, 46, 63, 80, and so on; each subsequent number is determined by adding 17 to the last ID number.
Systematic sampling should not be used when a cyclic repetition is inherent in the sampling frame. For example, systemic sampling is not appropriate for selecting months of the year in a study of the frequency of different types of accidents, because some accidents occur more often at certain times of the year. For instance, skiing injuries and automobile accidents most often occur in cold-weather months, whereas swimming injuries and farming accidents most often occur in warm-weather months.
A stratified random sample is one in which the population is first divided into relevant strata (subgroups), and a random sample is then selected from each stratum. In the radiograph example, the physician may wish to stratify on the age of patients, because the trachea varies in size with age and measuring the diameter accurately in young patients may be difficult. The population of radiographs may be divided into infants younger than 1 year old, children from 1 year old to less than 6 years old, children from 6 to younger than 16 years old, and subjects 16 years of age or older; a random sample is then selected from each age stratum. Other commonly used strata in medicine besides age include gender of patient, severity or stage of disease, and duration of disease. Characteristics used to stratify should be related to the measurement of interest, in which case stratified random sampling is the most efficient, meaning that it requires the smallest sample size.
A cluster random sample results from a two-stage process in which the population is divided into clusters and a subset of the clusters is randomly selected. Clusters are commonly based on geographic areas or districts, so this approach is used more often in epidemiologic research than in clinical studies. For example, the sample for a household survey taken in a city may be selected by using city blocks as clusters; a random sample of city blocks is selected, and all households (or a random sample of households) within the selected city blocks are surveyed. In multicenter trials, the institutions selected to participate in the study constitute the clusters; patients from each institution can be selected using another random-sampling procedure. Cluster sampling is somewhat less efficient than the other sampling methods because it requires a larger sample size, but in some situations, such as in multicenter trials, it is the method of choice for obtaining adequate numbers of patients.
The sampling methods just discussed are all based on probability, but nonprobability sampling methods also exist, such as con venience samples or quota samples. Nonprobability samples are those in which the probability that a subject is selected is unknown and may reflect selection biases of the person doing the study; they do not fulfill the requirements of randomness needed to estimate sampling errors. When we use the term “sample” in the context of observational studies, we will assume that the sample has been randomly selected in an appropriate way.
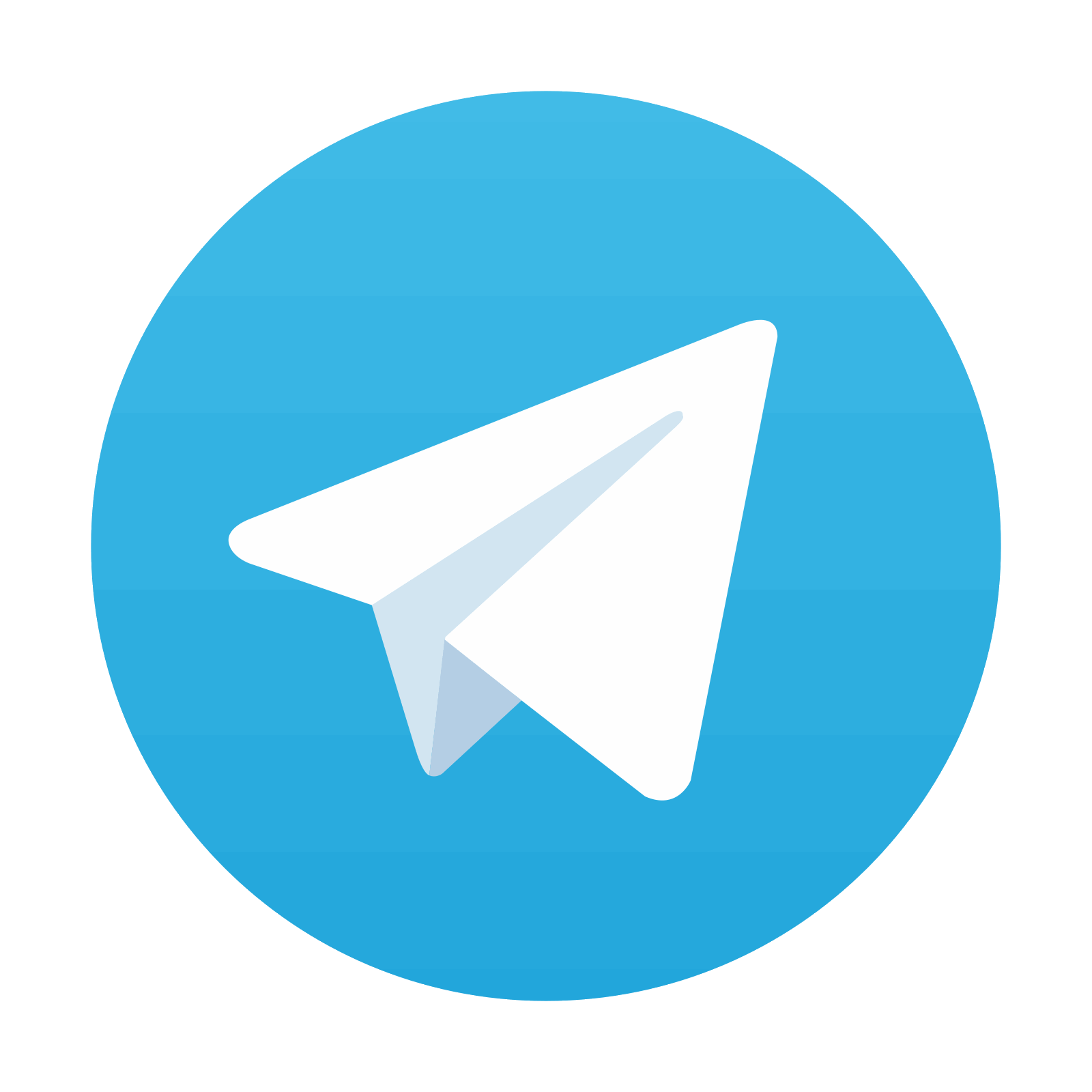
Stay updated, free articles. Join our Telegram channel

Full access? Get Clinical Tree
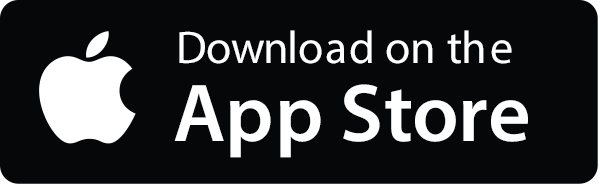
