Key Concepts
All observations of subjects in a study are evaluated on a scale of measurement that determines how the observations should be summarized, displayed, and analyzed.
Nominal scales are used to categorize discrete characteristics.
Ordinal scales categorize characteristics that have an inherent order.
Numerical scales measure the amount or quantity of something.
Means measure the middle of the distribution of a numerical characteristic.
Medians measure the middle of the distribution of an ordinal characteristic or a numerical characteristic that is skewed.
The standard deviation is a measure of the spread of observations around the mean and is used in many statistical procedures.
The coefficient of variation is a measure of relative spread that permits the comparison of observations measured on different scales.
Percentiles are useful to compare an individual observation with a norm.
Stem-and-leaf plots are a combination of frequency tables and histograms that are useful in exploring the distribution of a set of observations.
Frequency tables show the number of observations having a specific characteristic.
Histograms, box plots, and frequency polygons display distributions of numerical observations.
Proportions and percentages are used to summarize nominal and ordinal data.
Rates describe the number of events that occur in a given period.
Prevalence and incidence are two important measures of morbidity.
Rates must be adjusted when populations being compared differ in an important confounding factor.
The relationship between two numerical characteristics is described by the correlation.
The relationship between two nominal characteristics is described by the risk ratio, odds ratio, and event rates.
Number needed to treat is a useful indication of the effectiveness of a given therapy or procedure.
Scatterplots illustrate the relationship between two numerical characteristics.
Poorly designed graphs and tables mislead in the information they provide.
Computer programs are essential in today’s research environment, and skills to use and interpret them can be very useful.
Presenting Problems
Pulmonary embolism (PE) is a leading cause of morbidity and mortality. Clinical features are nonspecific and a certain diagnosis is often difficult to make. Attempts to simplify and improve the diagnostic process in evaluating patients for possible PE have been made by the introduction of two components: determination of pretest probability and d-dimer testing. Pretest probability is determined by developing explicit criteria for determining the clinical probability of PE. d-dimer assays measure the formation of d-dimer when cross-linked fibrin in thrombi is broken down by plasmin.Elevated levels of d-dimer can be used to detect deep venous thrombosis (DVT) and PE. Some d-dimer tests are very sensitive for DVT and a normal result can be used to exclude venous thromboembolism.
Kline and colleagues (2002) wished to develop a set of clinical criteria that would define a subgroup of patients with a pretest probability of PE of greater than 40% (high-risk group). These patients would be at too great a risk of experiencing a PE to have the diagnosis excluded on the basis of d-dimer testing. However, patients with a lower pretest probability (low-risk group), in whom a normal result can help rule out the diagnosis of PE, might be suitable candidates for d-dimer testing. Data were available for 934 patients with suspected PE at seven urban emergency departments (ED) in the United States. The investigators measured a number of potential risk factors for PE, and we look at some basic attributes of the observations in this chapter. A random sample of shock index observations on 18 patients is given in the section titled, “Calculating Measures of Central Tendency” and the entire data set is in a folder on the CD-ROM [available only with the book] entitled “Kline.”
The aging of the baby-boomers is leading to important demographic changes in the population, with significant implications for health care planners. Over the next 30 years in the United States, the proportion of people over the age of 75 years is expected to increase greatly. With the aging of the population, functional decline resulting in disability and morbidity is a major challenge to health care systems.
Hébert and coworkers (1997) designed a study to measure disability and functional changes over a 2-year period in a community-dwelling population age 75 years and older. A nurse interviewed 655 residents in their homes in Quebec, Canada. The Functional Autonomy Measurement System (SMAF), a 29-item rating scale measuring functional disability in five areas, was administered together with a questionnaire measuring health, cognitive function, and depression. Each individual was interviewed again 1 and 2 years later by the same nurse. The SMAF scale rates each item on a 4-point scale, where 0 is independent and 3 is dependent. Functional decline was defined by an increase of 5 points or more on the questionnaire, and improvement as a change within ± 4 points. (The final analysis included 572 subjects, 504 of whom completed both follow-up interviews and 68 of whom died during the study.)
The authors wanted to summarize the data and estimate declines in functional status. They also wanted to examine the relationship between changes in scores over the two 1-year periods. Data are given in the section titled “Displaying Numerical Data in Tables & Graphs” and on the CD-ROM [available only with the book] in a folder entitled “Hébert.”
A large study in a primary care clinic found that one in 20 women had experienced domestic violence (DV) in the previous year and one in four had experienced it sometime during her adult life. Children from violent homes often suffer behavioral, emotional, and physical health consequences either because they themselves are abused or because they have witnessed the abuse of their mother. Pediatricians have a unique opportunity to screen mothers because DV-battered women may be more likely to obtain medical care for their children than for themselves.
Lapidus and colleagues (2002) at the Connecticut Children’s Medical Center conducted a survey to assess DV education and training and the use of DV screening among a representative statewide sample of pediatricians and family physicians. They mailed self-administered surveys to 903 physicians identified as active members of the American Academy of Pediatrics and the American Academy of Family Physicians. The survey requested information on physician demographics and issues relating to DV. Domestic violence was defined as “past or current physical, sexual, emotional, or verbal harm to a woman caused by a spouse, partner, or family member.” Overall, 49% of the physicians responded to the survey after a total of three mailings. The authors looked at the distribution of responses and calculated some measures of predictive factors. We will revisit this study in the chapter on survey research. In this chapter, we illustrate frequency tables and odds ratios calculated by these investigators. Data are on the CD-ROM [available only with the book] in a folder entitled “Lapidus.”
Factor VIII is one of the procoagulants of the intrinsic pathway of coagulation. Hemophilia A, a disease affecting about 1 in 10,000 males, is a hereditary hemorrhagic disorder characterized by deficient or defective factor VIII. Acquired hemophilia is a much rarer hemorrhagic disorder af fecting 1 person per million each year and char acterized by spontaneous development of an autoantibody directed against factor VIII. Patients often present with ecchymosis, hematomas, hematuria, or compressive neuropathy. The hemorrhagic complications are fatal in 14–22% of patients. Underlying diseases, including autoimmune diseases and malignancies, are often associated with acquired hemophilia.
Optimal treatment is not yet established and, because the disease is so rare, no randomized controlled trials of treatment have been undertaken. A retrospective study of 34 patients with acquired hemophilia due to factor VIII inhibitors was conducted along with an extensive literature review to clarify the clinical characteristics of this disease and plan a prospective study of optimal treatment (Bossi et al, 1998). Information from the study is given in the section titled “Tables and Graphs for Nominal and Ordinal Data.” The investigators want to summarize data on some risk factors for men and women separately.
Premature birth, especially after fewer than 32 weeks of gestation, is associated with a high incidence of respiratory distress syndrome and a form of chronic lung disease known as bronchopulmonary dysplasia. Lung disease is the principal cause of morbidity and mortality in premature infants.
Thyroid hormones stimulate fetal lung development in animals. Little thyroid hormone is transferred from mother to fetus, but thyrotropin-releasing hormone (TRH) given to the mother increases fetal serum concentrations of thyroid hormone. Several studies have shown that the antenatal administration of TRH reduces the incidence and severity of respiratory distress syndrome, chronic lung disease, and death in these high-risk infants. Two other studies showed no benefit from treatment with TRH.
Ballard and coinvestigators (1998) wanted to reassess the efficacy and safety of antenatal administration of TRH in improving pulmonary outcome in preterm infants. Most of the earlier studies were relatively small, and one had not been blinded. Also, changes in neonatal care implemented in the past decade, particularly the use of surfactant, improved the chances of survival of premature infants.
The study enrolled 996 women in active labor with gestations of at least 24 but fewer than 30 weeks into a randomized, double-blind, placebo-controlled trial of antenatalTRH. The women receiving active treatment were given four doses of 400 μg of TRH intravenously at 8-h intervals. Those receiving placebo were given normal saline. Both groups received glucocorticoids, and surfactant was given to the infants when clinically indicated. There were 1134 live births (844 single and 290 multiple) and 11 stillbirths.
Infants born at 32 or fewer weeks gestation constituted the group at risk for lung disease; those born at 33 weeks or later were not at risk for lung disease. Outcomes included infant death on or before the 28th day after delivery; chronic lung disease, defined as the need for oxygen therapy for 21 of the first 28 days of life; and the development of respiratory stress syndrome, defined as the need for oxygen and either assisted ventilation or radiologic findings. The authors wanted to find the risk of developing these outcomes in the TRH group compared with the placebo group. Selected results from the study are given in the section titled “Number Needed to Treat.”
Purpose of the Chapter
This chapter introduces different kinds of data collected in medical research and demonstrates how to organize and present summaries of the data. Regardless of the particular research being done, investigators collect observations and generally want to transform them into tables or graphs or to present summary numbers, such as percentages or means. From a statistical perspective, it does not matter whether the observations are on people, animals, inanimate objects, or events. What matters is the kind of observations and the scale on which they are measured. These features determine the statistics used to summarize the data, called descriptive statistics, and the types of tables or graphs that best display and communicate the observations.
We use the data from the presenting problems to illustrate the steps involved in calculating the statistics because we believe that seeing the steps helps most people understand procedures. As we emphasize throughout this book, however, we expect that most people will use a computer to analyze data. In fact, this and following chapters contain numerous illustrations from some commonly used statistical computer programs, including NCSS contained on the CD-ROM [available only with the book] .
Scales of Measurement
The scale for measuring a characteristic has implications for the way information is displayed and summarized. As we will see in later chapters, the scale of measurement—the precision with which a characteristic is measured—also determines the statistical methods for analyzing the data. The three scales of measurement that occur most often in medicine are nominal, ordinal, and numerical.
Nominal scales are used for the simplest level of measurement when data values fit into categories. For example, in Presenting Problem 5 Ballard and colleagues (1998) use the following nominal characteristic to describe the outcome in infants being treated with antenatal TRH: the development of respiratory distress syndrome. In this example, the observations are dichotomous or binary in that the outcome can take on only one of two values: yes or no. Although we talk about nominal data as being on the measurement scale, we do not actually measure nominal data; instead, we count the number of observations with or without the attribute of interest.
Many classifications in medical research are evaluated on a nominal scale. Outcomes of a medical treatment or surgical procedure, as well as the presence of possible risk factors, are often described as either occurring or not occurring. Outcomes may also be described with more than two categories, such as the classification of anemias as microcytic (including iron deficiency), macrocytic or megaloblastic (including vitamin B12 deficiency), and normocytic (often associated with chronic disease).
Data evaluated on a nominal scale are sometimes called qualitative observations, because they describe a quality of the person or thing studied, or categorical observations, because the values fit into categories. Nominal or qualitative data are generally described in terms of percentages or proportions, such as the fact that 38% of the patients in the study of patients with acquired hemophilia (Bossi et al, 1998) developed hematuria. Contingency tables and bar charts are most often used to display this type of information and are presented in the section titled “Tables and Graphs for Nominal and Ordinal Data.”
When an inherent order occurs among the categories, the observations are said to be measured on an ordinal scale. Observations are still classified, as with nominal scales, but some observations have more or are greater than other observations. Clinicians often use ordinal scales to determine a patient’s amount of risk or the appropriate type of therapy. Tumors, for example, are staged according to their degree of development. The international classification for staging of carcinoma of the cervix is an ordinal scale from 0 to 4, in which stage 0 represents carcinoma in situ and stage 4 represents carcinoma extending beyond the pelvis or involving the mucosa of the bladder and rectum. The inherent order in this ordinal scale is, of course, that the prognosis for stage 4 is worse than that for stage 0.
Classifications based on the extent of disease are sometimes related to a patient’s activity level. For example, rheumatoid arthritis is classified, according to the severity of disease, into four classes ranging from normal activity (class 1) to wheelchair-bound (class 4). Using the Functional Autonomy Measurement System developed by the World Health Organization, Hébert and coinvestigators (1997) studied the functional activity of elderly people who live in a community. Although order exists among categories in ordinal scales, the difference between two adjacent categories is not the same throughout the scale. To illustrate, Apgar scores, which describe the maturity of newborn infants, range from 0 to 10, with lower scores indicating depression of cardiorespiratory and neurologic functioning and higher scores indicating good functioning. The difference between scores of 8 and 9 probably does not have the same clinical implications as the difference between scores of 0 and 1.
Some scales consist of scores for multiple factors that are then added to get an overall index. An index frequently used to estimate the cardiac risk in noncardiac surgical procedures was developed by Goldman and his colleagues (1977, 1995). This index assigns points to a variety of risk factors, such as age over 70 years, history of an MI in the past 6 months, specific electrocardiogram abnormalities, and general physical status. The points are added to get an overall score from 0 to 53, which is used to indicate the risk of complications or death for different score levels.
A special type of ordered scale is a rank-order scale, in which observations are ranked from highest to lowest (or vice versa). For example, health providers could direct their education efforts aimed at the obstetric patient based on ranking the causes of low birthweight in infants, such as malnutrition, drug abuse, and inadequate prenatal care, from most common to least common. The duration of surgical procedures might be converted to a rank scale to obtain one measure of the difficulty of the procedure.
As with nominal scales, percentages and proportions are often used with ordinal scales. The entire set of data measured on an ordinal scale may be summarized by the median value, and we will describe how to find the median and what it means. Ordinal scales having a large number of values are sometimes treated as if they are numerical (see following section). The same types of tables and graphs used to display nominal data may also be used with ordinal data.
Observations for which the differences between numbers have meaning on a numerical scale are sometimes called quantitative observations because they measure the quantity of something. There are two types of numerical scales: continuousa (interval) and discrete scales. A continuous scale has values on a continuum (eg, age); a discrete scale has values equal to integers (eg, number of fractures).
aSome statisticians differentiate interval scales (with an arbitrary zero point) from ratio scales (with an absolute zero point); examples are temperature on a Celsius scale (interval) and temperature on a Kelvin scale (ratio). Little difference exists, however, in how measures on these two scales are treated statistically, so we call them both simply numerical.
If data need not be very precise, continuous data may be reported to the closest integer. Theoretically, however, more precise measurement is possible. Age is a continuous measure, and age recorded to the nearest year will generally suffice in studies of adults; however, for young children, age to the nearest month may be preferable. Other examples of continuous data include height, weight, length of time of survival, range of joint motion, and many laboratory values.
When a numerical observation can take on only integer values, the scale of measurement is discrete. For example, counts of things—number of pregnancies, number of previous operations, number of risk factors—are discrete measures.
In the study by Kline and colleagues (2002), several patient characteristics were evaluated, including shock index and presence of PE. The first characteristic is measured on a continuous numerical scale because it can take on any individual value in the possible range of values. Presence of PE has a nominal scale with only two values: presence or absence. In the study by Ballard and coworkers (1998), the number of infants who developed respiratory distress syndrome is an example of a discrete numerical scale.
Characteristics measured on a numerical scale are frequently displayed in a variety of tables and graphs. Means and standard deviations are generally used to summarize the values of numerical measures. We next examine ways to summarize and display numerical data and then return to the subject of ordinal and nominal data.
Summarizing Numerical Data with Numbers
When an investigator collects many observations, such as shock index or blood pressure in the study by Kline and colleagues (2002), numbers that summarize the data can communicate a lot of information.
One of the most useful summary numbers is an indicator of the center of a distribution of observations—the middle or average value. The three measures of central tendency used in medicine and epidemiology are the mean, the median, and, to a lesser extent, the mode. All three are used for numerical data, and the median is used for ordinal data as well.
Although several means may be mathematically calculated, the arithmetic, or simple, mean is used most frequently in statistics and is the one generally referred to by the term “mean.” The mean is the arithmetic average of the observations. It is symbolized by
(called X-bar) and is calculated as follows: add the observations to obtain the sum and then divide by the number of observations.
The formula for the mean is written Σ X / n, where Σ (Greek letter sigma) means to add, X represents the individual observations, and n is the number of observations.
Table 3–1 gives the value of the shock index, systolic blood pressure, and heart rate for 18 randomly selected patients in the d-dimer study (Kline et al, 2002). (We will learn about random sampling in Chapter 4.) The mean shock index for these 18 patients is
Subject ID | Shock Index | Systolic Blood Pressure | Heart Rate |
---|---|---|---|
1 | 0.61 | 139 | 85 |
2 | 0.56 | 151 | 84 |
3 | 0.52 | 201 | 104 |
4 | 0.33 | 170 | 56 |
5 | 0.45 | 123 | 55 |
6 | 0.74 | 121 | 90 |
7 | 0.73 | 119 | 87 |
8 | 0.92 | 100 | 92 |
9 | 0.42 | 164 | 69 |
10 | 0.63 | 161 | 102 |
11 | 0.55 | 164 | |
12 | 0.50 | 138 | 69 |
13 | 0.75 | 118 | 89 |
14 | 0.82 | 130 | 106 |
15 | 1.30 | 109 | 142 |
16 | 1.29 | 92 | 119 |
17 | 0.85 | 126 | 107 |
18 | 0.44 | 139 | 61 |
The mean is used when the numbers can be added (ie, when the characteristics are measured on a numerical scale); it should not ordinarily be used with ordinal data because of the arbitrary nature of an ordinal scale. The mean is sensitive to extreme values in a set of observations, especially when the sample size is fairly small. For example, the values of 1.30 for subject 15 and is relatively large compared with the others. If this value was not present, the mean would be 0.612 instead of 0.689.
If the original observations are not available, the mean can be estimated from a frequency table. A weighted average is formed by multiplying each data value by the number of observations that have that value, adding the products, and dividing the sum by the number of observations. We have formed a frequency table of shock index observations in Table 3–2, and we can use it to estimate the mean shock index for all patients in the study. The weighted-average estimate of the mean, using the number of subjects and the midpoints in each interval, is
Shock Index | Count | Cumulative Count | Percent | Cumulative Percent | Graph of Percent |
---|---|---|---|---|---|
0.40 or less | 38 | 38 | 4.08 | 4.08 | / |
0.40 up to 0.50 | 104 | 142 | 11.16 | 15.24 | //// |
0.50 up to 0.60 | 198 | 340 | 21.24 | 36.48 | //////// |
0.60 up to 0.70 | 199 | 539 | 21.35 | 57.83 | //////// |
0.70 up to 0.80 | 155 | 694 | 16.63 | 74.46 | ////// |
0.80 up to 0.90 | 102 | 796 | 10.94 | 85.41 | //// |
0.90 up to 1.00 | 60 | 856 | 6.44 | 91.85 | // |
1.00 up to 1.10 | 37 | 893 | 3.97 | 95.82 | / |
1.10 up to 1.20 | 19 | 912 | 2.04 | 97.85 | / |
1.20 or higher | 19 | 932 | 2.15 | 100.00 | / |
The value of the mean calculated from a frequency table is not always the same as the value obtained with raw numbers. In this example, the shock index means calculated from the raw numbers and the frequency table are very close. Investigators who calculate the mean for presentation in a paper or talk have the original observations, of course, and should use the exact formula. The formula for use with a frequency table is helpful when we as readers of an article do not have access to the raw data but want an estimate of the mean.
The median is the middle observation, that is, the point at which half the observations are smaller and half are larger. The median is sometimes symbolized by M or Md, but it has no conventional symbol. The procedure for calculating the median is as follows:
- 1. Arrange the observations from smallest to largest (or vice versa).
- 2. Count in to find the middle value. The median is the middle value for an odd number of observations; it is defined as the mean of the two middle values for an even number of observations.
For example, in rank order (from lowest to highest), the shock index values in Table 3–1 are as follows:
0.33, 0.42, 0.44, 0.45, 0.50, 0.52, 0.55, 0.56, 0.61, 0.63, 0.73, 0.74, 0.75, 0.82, 0.85, 0.92, 1.29, 1.30
For 18 observations, the median is the mean of the ninth and tenth values (0.61 and 0.63), or 0.62. The median tells us that half the shock index values in this group are less than 0.62 and half are greater than 0.62. We will learn later in this chapter that the median is easy to determine from a stem-and-leaf plot of the observations.
The median is less sensitive to extreme values than is the mean. For example, if the largest observation, 1.30, is excluded from the sample, the median would be the middle value, 0.61. The median is also used with ordinal observations.
The mode is the value that occurs most frequently. It is commonly used for a large number of observations when the researcher wants to designate the value that occurs most often. No single observation occurs most frequently among the data in Table 3–1. When a set of data has two modes, it is called bimodal. For frequency tables or a small number of observations, the mode is sometimes estimated by the modal class, which is the interval having the largest number of observations. For the shock index data in Table 3–2, the modal class is 0.60 thru 0.69 with 199 patients.
Another measure of central tendency not used as often as the arithmetic mean or the median is the geometric mean, sometimes symbolized as GM or G. It is the nth root of the product of the n observations. In symbolic form, for n observations X1, X2, X3, . . Xn, the geometric mean is
The geometric mean is generally used with data measured on a logarithmic scale, such as the dilution of the smallpox vaccine studied by Frey and colleagues (2002), a presenting problem in Chapter 5. Taking the logarithm of both sides of the preceding equation, we see that the logarithm of the geometric mean is equal to the mean of the logarithms of the observations.
Use the CD-ROM [available only with the book] and find the mean, median, and mode for the shock index for all of the patients in the study by Kline and colleagues (2002). Repeat for patients who did and did not have a PE. Do you think the mean shock index is different for these two groups? In Chapter 6 we will learn how to answer this type of question.
Which measure of central tendency is best with a particular set of observations? Two factors are important: the scale of measurement (ordinal or numerical) and the shape of the distribution of observations. Although distributions are discussed in more detail in Chapter 4, we consider here the notion of whether a distribution is symmetric about the mean or is skewed to the left or the right.
If outlying observations occur in only one direction—either a few small values or a few large ones—the distribution is said to be a skewed distribution. If the outlying values are small, the distribution is skewed to the left, or negatively skewed; if the outlying values are large, the distribution is skewed to the right, or positively skewed. A symmetric distribution has the same shape on both sides of the mean. Figure 3–1 gives examples of negatively skewed, positively skewed, and symmetric distributions.
The following facts help us as readers of articles know the shape of a distribution without actually seeing it.
- 1. If the mean and the median are equal, the distri bution of observations is symmetric, generally as in Figures 3–1C and 3–1D.
- 2. If the mean is larger than the median, the distribution is skewed to the right, as in Figure 3–1B.
- 3. If the mean is smaller than the median, the distribution is skewed to the left, as in Figure 3–1A.
In a study of the increase in educational debt among Canadian medical students, Kwong and colleagues (2002) reported the median level of debt for graduating students. The investigators reported the median rather than the mean because a relatively small number of students had extremely high debts, which would cause the mean to be an overestimate. The following guidelines help us decide which measure of central tendency is best.
- 1. The mean is used for numerical data and for symmetric (not skewed) distributions.
- 2. The median is used for ordinal data or for numerical data if the distribution is skewed.
- 3. The mode is used primarily for bimodal distributions.
- 4. The geometric mean is generally used for observations measured on a logarithmic scale.
Suppose all you know about the 18 randomly selected patients in Presenting Problem 1 is that the mean shock index is 0.69. Although the mean provides useful information, you have a better idea of the distribution of shock indices in these patients if you know something about the spread, or the variation, of the observations. Several statistics are used to describe the dispersion of data: range, standard deviation, coefficient of variation, percentile rank, and interquartile range. All are described in the following sections.
The range is the difference between the largest and the smallest observation. It is easy to determine once the data have been arranged in rank order. For example, the lowest shock index among the 18 patients is 0.33, and the highest is 1.30; thus, the range is 1.30 minus 0.33, or 0.97. Many authors give minimum and maximum values instead of the range, and in some ways these values are more useful.
The standard deviation is the most commonly used measure of dispersion with medical and health data. Although its meaning and computation are somewhat complex, it is very important because it is used both to describe how observations cluster around the mean and in many statistical tests. Most of you will use a computer to determine the standard deviation, but we present the steps involved in its calculation to give a greater understanding of the meaning of this statistic.
The standard deviation is a measure of the spread of data about their mean. Briefly looking at the logic behind this statistic, we need a measure of the “average” spread of the observations about the mean. Why not find the deviation of each observation from the mean, add these deviations, and divide the sum by n to form an analogy to the mean itself? The problem is that the sum of deviations about the mean is always zero (see Exercise 1). Why not use the absolute values of the deviations? The absolute value of a number ignores the sign of the number and is denoted by vertical bars on each side of the number. For example, the absolute value of 5, |5|, is 5, and the absolute value of –5, |-5|, is also 5. Although this approach avoids the zero sum problem, it lacks some important statistical properties, and so is not used. Instead, the deviations are squared before adding them, and then the square root is found to express the standard deviation on the original scale of measurement. The standard deviation is symbolized as SD, sd, or simply s (in this text we use SD), and its formula is
The name of the statistic before the square root is taken is the variance, but the standard deviation is the statistic of primary interest.
Using n – 1 instead of n in the denominator produces a more accurate estimate of the true population standard deviation and has desirable mathematical properties for statistical inferences.
The preceding formula for standard deviation, called the definitional formula, is not the easiest one for calculations. Another formula, the computational formula, is generally used instead. Because we generally compute the standard deviation using a computer, the illustrations in this text use the more meaningful but computationally less efficient formula. If you are curious, the computational formula is given in Exercise 7.
Now let’s try a calculation. The shock index values for the 18 patients are repeated in Table 3–3 along with the computations needed. The steps follow:
Patient | X | X – ![]() | (X –![]() |
---|---|---|---|
1 | 0.61 | –0.08 | 0.01 |
2 | 0.56 | –0.13 | 0.02 |
3 | 0.52 | –0.17 | 0.03 |
4 | 0.33 | –0.36 | 0.13 |
5 | 0.45 | –0.24 | 0.06 |
6 | 0.74 | 0.05 | 0.00 |
7 | 0.73 | 0.04 | 0.00 |
8 | 0.92 | 0.23 | 0.05 |
9 | 0.42 | –0.27 | 0.07 |
10 | 0.63 | –0.06 | 0.00 |
11 | 0.55 | –0.14 | 0.02 |
12 | 0.50 | –0.19 | 0.04 |
13 | 0.75 | 0.06 | 0.00 |
14 | 0.82 | 0.13 | 0.02 |
15 | 1.30 | 0.61 | 0.38 |
16 | 1.29 | 0.60 | 0.36 |
17 | 0.85 | 0.16 | 0.03 |
18 | 0.44 | –0.25 | 0.06 |
Sums | 12.41 | 1.28 | |
Mean | 0.69 |
- 1. Let X be the shock index for each patient, and find the mean: the mean is 0.69, as we calculated earlier.
- 2. Subtract the mean from each observation to form the deviations X – mean.
- 3. Square each deviation to form (X – mean)2.
- 4. Add the squared deviations.
- 5. Divide the result in step 4 by n – 1; we have 0.071. This value is the variance.
- 6. Take the square root of the value in step 5 to find the standard deviation; we have 0.267 or 0.27. (The actual value is 0.275 or 0.28; our result is due to round-off error.)
But note the relatively large squared deviation of 0.38 for patient 15 in Table 3–3. It contributes substantially to the variation in the data. The standard deviation of the remaining 17 patients (after eliminating patient 15) is smaller, 0.235, demonstrating the effect that outlying observations can have on the value of the standard deviation.
The standard deviation, like the mean, requires numerical data. Also, like the mean, the standard deviation is a very important statistic. First, it is an essential part of many statistical tests as we will see in later chapters. Second, the standard deviation is very useful in describing the spread of the observations about the mean value. Two rules of thumb when using the standard deviation are:
- 1. Regardless of how the observations are distributed, at least 75% of the values always lie between these two numbers: the mean minus 2 standard deviations and the mean plus 2 standard deviations. In the shock index example, the mean is 0.69 and the standard deviation is 0.28; therefore, at least 75% lie between 0.69 ± 2(0.28), or between 0.13 and 1.25. In this example, 16 of the 18 observations, or 89%, fall between these limits.
- 2. If the distribution of observations is bell-shaped, then even more can be said about the percentage of observations that lay between the mean and ± 2 standard deviations. For a bell-shaped distribution, approximately:
- 67% of the observations lie between the mean ± 1 standard deviation
- 95% of the observations lie between the mean ± 2 standard deviations
- 99.7% of the observations lie between the mean ± 3 standard deviations
The standard deviation, along with the mean, can be helpful in determining skewness when only summary statistics are given: if the mean minus 2 SD contains zero (ie, the mean is smaller than 2 SD), the observations are probably skewed.
Use the CD-ROM [available only with the book], and find the range and standard deviation of shock index for all of the patients in the Kline and colleagues study (2002). Repeat for patients with and without a PE. Are the distributions of shock index similar in these two groups of patients?
The coefficient of variation (CV) is a useful measure of relative spread in data and is used frequently in the biologic sciences. For example, suppose Kline and his colleagues (2002) wanted to compare the variability in shock index with the variability in systolic blood pressure (BP) in the patients in their study. The mean and the standard deviation of shock index in the total sample are 0.69 and 0.20, respectively; for systolic BP, they are 138 and 0.26, respectively. A comparison of the standard deviations makes no sense because shock index and systolic BP are measured on much different scales. The coefficient of variation adjusts the scales so that a sensible comparison can be made.
The coefficient of variation is defined as the standard deviation divided by the mean times 100%. It produces a measure of relative variation—variation that is relative to the size of the mean. The formula for the coefficient of variation is
From this formula, the CV for shock index is (0.20/0.69)(100%) = 29.0%, and the CV for systolic BP is (26/138)(100%) = 18.8%. We can therefore conclude that the relative variation in shock index is considerably greater than the variation in systolic BP. A frequent application of the coefficient of variation in the health field is in laboratory testing and quality control procedures.
Use the CD-ROM [available only with the book] and find the coefficient of variation for shock index for patients who did and did not have a PE in the Kline and colleagues study.
A percentile is the percentage of a distribution that is equal to or below a particular number. For example, consider the standard physical growth chart for girls from birth to 36 months old given in Figure 3–2. For girls 21 months of age, the 95th percentile of weight is 12 kg, as noted by the arrow in the chart. This percentile means that among 21-month-old girls, 95% weigh 12 kg or less and only 5% weigh more than 12 kg. The 50th percentile is, of course, the same value as the median; for 21-month-old girls, the median or 50th percentile weight is approximately 10.6 kg.
Percentiles are often used to compare an individual value with a norm. They are extensively used to develop and interpret physical growth charts and measurements of ability and intelligence. They also determine normal ranges of laboratory values; the “normal limits” of many laboratory values are set by the 2½ and 97½ percentiles, so that the normal limits contain the central 95% of the distribution. This approach was taken in a study by Gelber and colleagues (1997) when they developed norms for mean heart variation to breathing and Valsalva ratio (see Exercise 2).
A measure of variation that makes use of percentiles is the interquartile range, defined as the difference between the 25th and 75th percentiles, also called the first and third quartiles, respectively. The interquartile range contains the central 50% of observations. For example, the interquartile range of weights of girls who are 9 months of age (see Figure 3–2) is the difference between 7.5 kg (the 75th percentile) and 6.5 kg (the 25th percentile); that is, 50% of infant girls weigh between 6.5 and 7.5 kg at 9 months of age.
The following guidelines are useful in deciding which measure of dispersion is most appropriate for a given set of data.
1. The standard deviation is used when the mean is used (ie, with symmetric numerical data).
2. Percentiles and the interquartile range are used in two situations:
a. When the median is used (ie, with ordinal data or with skewed numerical data).
b. When the mean is used but the objective is to compare individual observations with a set of norms.
3. The interquartile range is used to describe the central 50% of a distribution, regardless of its shape.
4. The range is used with numerical data when the purpose is to emphasize extreme values.
5. The coefficient of variation is used when the intent is to compare distributions measured on different scales.
Displaying Numerical Data in Tables & Graphs
We all know the saying, “A picture is worth 1000 words,” and researchers in the health field certainly make frequent use of graphic and pictorial displays of data. Numerical data may be presented in a variety of ways, and we will use the data from the study by Hébert and colleagues (1997) on functional decline in the elderly (Presenting Problem 2) to illustrate some of the more common methods. The subjects in this study were 75 years of age or older. We use a subset of their data, the 72 patients age 85 years or older who completed the Functional Autonomy Measurement System (SMAF). The total score on the SMAF for these subjects in year 1, year 3, and the differences in score between year 3 and year 1 in are given in Table 3–4.
Age | Sex | SMAF at Time 1 | SMAF at Time 3 | Difference (Time 3 – Time 1) |
---|---|---|---|---|
90 | F | 28 | 20 | –8 |
88 | F | 8 | 11 | 3 |
88 | F | 6 | 9 | 3 |
90 | F | 22 | 18 | –4 |
88 | M | 6 | 7 | 1 |
86 | F | 9 | 9 | 0 |
86 | M | 23 | 15 | –8 |
85 | F | 12 | 40 | 28 |
88 | F | 9 | 30 | 21 |
86 | F | 5 | 15 | 10 |
95 | F | 20 | 16 | –4 |
88 | F | 3 | 26 | 23 |
87 | F | 22 | 24 | 2 |
86 | F | 20 | 20 | 0 |
86 | M | 0 | 1 | 1 |
93 | F | 30 | 34 | 4 |
87 | F | 13 | 23 | 10 |
94 | F | 47 | 52 | 5 |
86 | F | 1 | 20 | 19 |
85 | F | 3 | 50 | 47 |
87 | M | 4 | 57 | 53 |
89 | F | 12 | 14 | 2 |
87 | F | 1 | 4 | 3 |
87 | F | 13 | 16 | 3 |
85 | F | 1 | 1 | 0 |
85 | F | 35 | 30 | –5 |
88 | F | 22 | 19 | –3 |
88 | M | 1 | 1 | 0 |
86 | F | 2 | 17 | 15 |
88 | M | 3 | 3 | 0 |
86 | F | 21 | 39 | 18 |
85 | F | 2 | 2 | 0 |
85 | M | 7 | 8 | 1 |
88 | M | 8 | 10 | 2 |
85 | F | 7 | 5 | –2 |
89 | F | 11 | 20 | 9 |
87 | F | 1 | 0 | –1 |
88 | F | 12 | 19 | 7 |
87 | F | 19 | 56 | 37 |
94 | F | 21 | 16 | –5 |
86 | M | 17 | 26 | 9 |
85 | F | 27 | 21 | –6 |
85 | M | 4 | 2 | –2 |
85 | F | 9 | 5 | –4 |
85 | M | 7 | 34 | 27 |
87 | F | 38 | 34 | –4 |
85 | F | 13 | 22 | 9 |
85 | F | 4 | 4 | 0 |
85 | F | 17 | 27 | 10 |
90 | F | 23 | 27 | 4 |
86 | M | 12 | 13 | 1 |
88 | M | 30 | 29 | –1 |
85 | M | 27 | 26 | –1 |
87 | F | 26 | 47 | 21 |
86 | M | 44 | 46 | 2 |
85 | F | 21 | 23 | 2 |
86 | M | 17 | 57 | 40 |
88 | M | 10 | 19 | 9 |
85 | F | 15 | 22 | 7 |
86 | F | 4 | 6 | 2 |
88 | F | 10 | 12 | 2 |
88 | M | 18 | 22 | 4 |
87 | M | 12 | 20 | 8 |
85 | M | 37 | 47 | 10 |
85 | F | 17 | 14 | –3 |
89 | F | 14 | 19 | 5 |
85 | F | 11 | 14 | 3 |
87 | F | 4 | 6 | 2 |
86 | F | 16 | 26 | 10 |
90 | F | 5 | 6 | 1 |
85 | F | 48 | 51 | 3 |
88 | M | 9 | 17 | 8 |
Stem-and-leaf plots are graphs developed in 1977 by Tukey, a statistician interested in meaningful ways to communicate by visual display. They provide a convenient means of tallying the observations and can be used as a direct display of data or as a preliminary step in constructing a frequency table. The observations in Table 3–4 show that many of the differences in total scores are small, but also that some people have large positive scores, indicating large declines in function. The data are not easy to understand, however, by simply looking at a list of the raw numbers. The first step in organizing data for a stem-and-leaf plot is to decide on the number of subdivisions, called classes or intervals (it should generally be between 6 and 14; more details on this decision are given in the following section). Initially, we categorize observations by 5s, from –9 to –5, –4 to 0, 1 to 5, 6 to 10, 11 to 15, 16 to 20, and so on.
To form a stem-and-leaf plot, draw a vertical line, and place the first digits of each class—called the stem—on the left side of the line, as in Table 3–5. The numbers on the right side of the vertical line represent the second digit of each observation; they are the leaves. The steps in building a stem-and-leaf plot are as follows:
Stem | Leaves |
---|---|
–9 to –5 | 8 8 |
–4 to 0 | 4 0 |
+1 to +5 | 3 3 1 |
+6 to +10 | 0 |
+11 to +15 | |
+16 to +20 | |
+21 to +25 | 1 |
+26 to +30 | 8 |
+31 to +35 | |
+36 to +40 | |
+41 to +45 | |
+46 to +50 | |
+51 to +55 | |
+56 to +60 |
- 1. Take the score of the first person, –8, and write the second digit, 8, or leaf, on the right side of the vertical line, opposite the first digit, or stem, corresponding to –9 to –5.
- 2. For the second person, write the 3 (leaf) on the right side of the vertical line opposite 1 to 5 (stem).
- 3. For the third person, write the 3 (leaf) opposite 1 to 5 (stem) next to the previous score of 3.
- 4. For the fourth person, write the –4 (leaf) opposite –4 to 0 (stem); and so on.
- 5. When the observation is only one digit, such as for subjects 1 through 7 in Table 3–4, that digit is the leaf.
- 6. When the observation is two digits, however, such as the score of 28 for subject 8, only the second digit, or 8 in this case, is written.
The leaves for the first ten people are given in Table 3–5. The complete stem-and-leaf plot for the score changes of all the subjects is given in Table 3–6. The plot both provides a tally of observations and shows how the changes in scores are distributed. The choice of class widths of 5 points is reasonable, although we usually prefer to avoid having many empty classes at the high end of the scale. It is generally preferred to have equal class widths and to avoid open-ended intervals, such as 30 or higher, although some might choose to combine the higher classes in the final plot.
Stem | Leaves |
---|---|
–9 to –5 | 8 8 5 5 6 |
–4 to 0 | 4 0 4 0 0 3 0 0 0 2 1 2 4 4 0 1 1 3 |
+1 to +5 | 3 3 1 2 1 4 5 2 3 3 1 2 4 1 2 2 2 2 4 5 3 2 1 3 |
+6 to +10 | 0 0 9 7 9 9 0 9 7 8 0 8 0 |
+11 to +15 | 5 |
+16 to +20 | 9 8 |
+21 to +25 | 1 3 1 |
+26 to +30 | 8 7 |
+31 to +35 | |
+36 to +40 | 7 0 |
+41 to +45 | 7 |
+46 to +50 | |
+51 to +55 | 3 |
+56 to +60 |
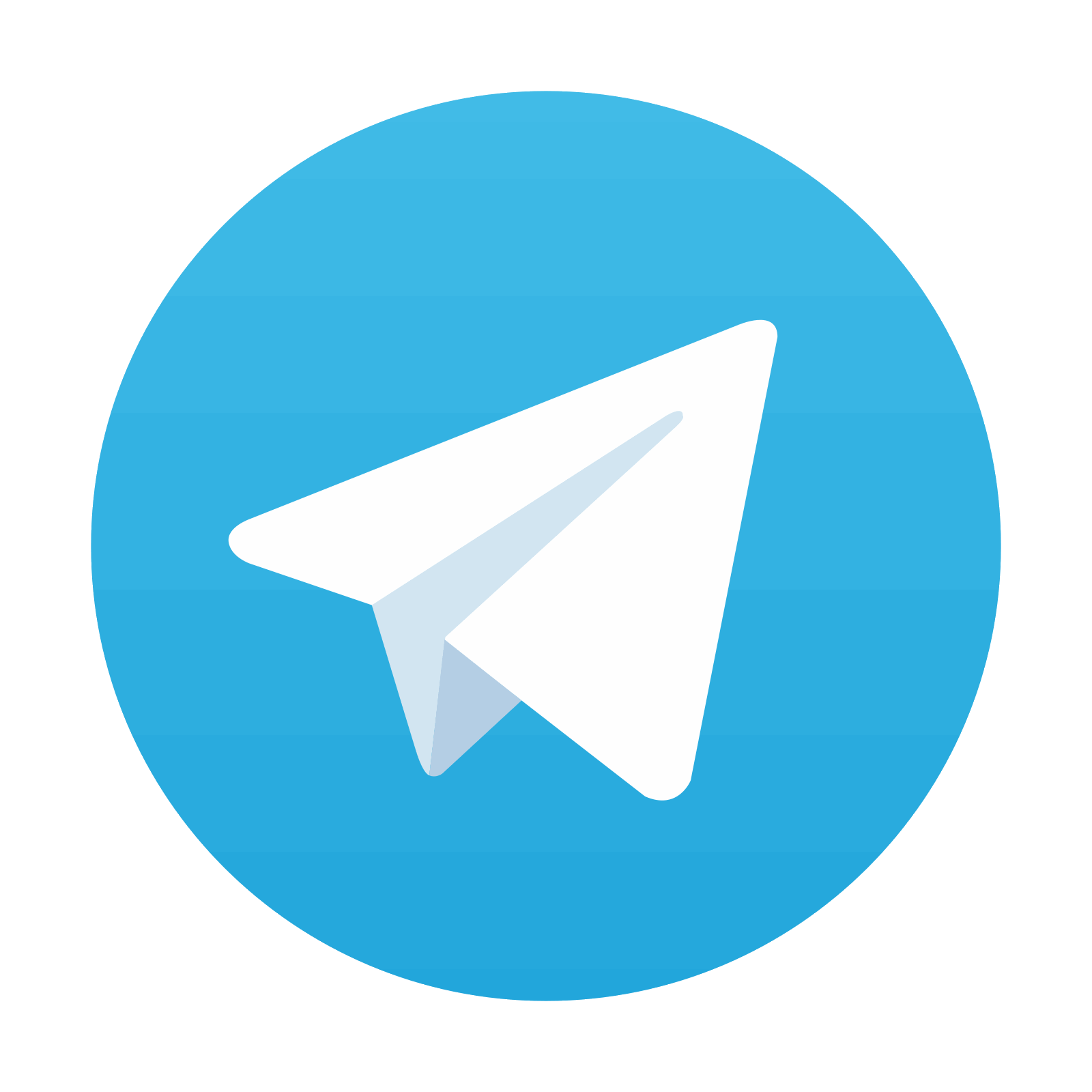
Stay updated, free articles. Join our Telegram channel

Full access? Get Clinical Tree
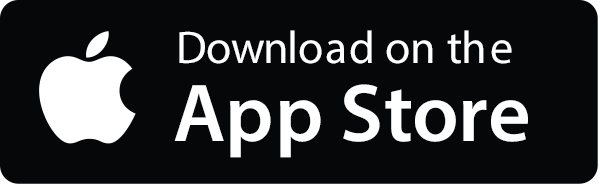
