Key Concepts
Selecting the appropriate diagnostic procedure depends, in part, on the clinician’s index of suspicion.
The threshold model for testing contains two decision points: when the index of suspicion is high enough to order a diagnostic procedure, and when the index of suspicion is so high that results from a procedure will not influence subsequent actions.
Two components of evaluating diagnostic procedures are sensitivity and specificity.
Sensitivity is a procedure’s ability to detect a disease if one is present.
Specificity is a procedure’s ability to give a negative result if no disease is present.
Two errors are possible: false-positive results occur when the procedure is positive but no disease is present; false-negative results occur when the procedure is negative but a disease is present.
Sensitivity and specificity must be combined with the clinician’s index of suspicion to properly interpret a procedure.
The 2 × 2 table method provides a simple way to use sensitivity and specificity to determine how to interpret the diagnostic procedure after it is done.
After sensitivity and specificity are applied to the clinician’s index of suspicion, the probability of a disease based on a positive test and the probability of no disease with a negative test can be found. They are the predictive values of a positive and negative test, respectively.
A likelihood ratio is the ratio of true-positives to false-positives; it is used with the prior odds of a disease (instead of the prior probability) to determine the odds after the test is done.
A decision tree may be used to find predictive values.
Bayes’ theorem gives the probability of one outcome, given that another outcome has occurred. It is another way to calculate predictive values.
A sensitive test is best to rule out a disease; a specific test is used to rule in a disease.
ROC (receiver operating characteristic) curves are used for diagnostic procedures that give a numerical result, rather than simply being positive and negative.
Decision analysis, often using decision trees, is an optimal way to model approaches to diagnosis or management.
Outcomes for the decision analysis may be costs, quality-of-life adjusted survival, or subjective utilities measuring how the patient values different outcomes.
The optimal decision from a decision tree may be analyzed to learn how sensitive the decision is to various assumptions regarding probabilities, costs, etc.
Decision analysis can be used to compare two or more alternative approaches to diagnosis or management (or both).
Decision analysis can be used to compare the timing for diagnostic testing.
Journal articles should not publish predictive values without reminding readers that these values depend on the prevalence or index of suspicion.
Presenting Problems
A 57-year-old man presents with a history of low back pain. The pain is aching in quality, persists at rest, and is made worse by bending and lifting. The pain has been getting progressively worse, and in the past 6 weeks has been awakening him at night. Within the past 10 days he has noticed numbness in the right buttock and thigh and weakness in the right lower extremity. He denies fever, but has had a slight loss of appetite and a 10-lb weight loss over a period of 4 months. He has no prior history of low back pain and his general health has been good. The physical examination reveals a temperature of 99.6°F, tenderness in the lower lumbar spine, a decrease in sensation over the dorsal and lateral aspect of the right foot, and weakness of right ankle eversion. Deep tendon reflexes were normal.
Based on your review of the literature, the patient’s history and physical examination, you suspect the man has a 20–30% chance of a spinal malignancy. You must decide whether to order an erythrocyte sedimentation rate (ESR) or directly order imaging studies, such as a lumbar MRI. Joines and colleagues (2001) compared several strategies for diagnosing cancer in patients with low back pain. They reported the sensitivity and specificity for different diagnostic procedures, including an ESR ≥ 20 mm/h and several imaging studies. They reported a sensitivity of 78% and specificity of 67% for an ESR ≥ 20 mm/h and sensitivity and specificity of 95% for lumbar MRI. They developed several diagnostic strategies, or decision trees, for investigating the possibility of cancer in primary care outpatients with low back pain and determined the cost for each diagnostic strategy using data from the year 2000 Medicare reimbursements. Strategies were arranged in order of cost per patient and compared with the number of cases of cancer found per 1000 patients. We use information from their study to illustrate sensitivity and specificity of a diagnostic procedure and again to illustrate the use of decision trees to compare strategies.
The electrocardiogram (ECG) is a valuable tool in the clinical prediction of an acute myocardial infarction (MI). In patients with ST segment elevation and chest pain typical of an acute MI, the chance that the patient has experienced an acute MI is greater than 90%. In patients with left bundle-branch block (LBBB) that precludes detection of ST segment elevation, however, the ECG has limited usefulness in the diagnosis of acute MI. An algorithm based on ST segment changes in patients with acute MI in the presence of LBBB showed a sensitivity of 78% for the diagnosis of an MI. A true-positiverate of 78% means that a substantial proportion of patients (22%) with LBBB who presented with acute MI would have a false-negativetest result and possibly be denied acute reperfusion therapy.
Shlipak and his colleagues (1999) conducted a historical cohort study of patients with acute cardiopulmonary symptoms who had LBBB to evaluate the diagnostic test characteristics and clinical utility of this ECG algorithm for patients with suspected MI. They used their results to develop a decision tree to estimate the outcome for three different clinical approaches to these patients: (l) treat all such patients with thrombolysis, (2) treat none of them with thrombolysis, and (3) use the ECG algorithm as a screening test for thrombolysis.
Eighty-three patients with LBBB who presented 103 times with symptoms suggestive of MI were studied. Nine individual ECG predictors of acute MI were evaluated. None of the nine predictors effectively distinguished the 30% of patients with MI from those with other diagnoses. The ECG algorithm had a sensitivity of only 10%. The decision analysis estimated that 92.9% of patients with LBBB and chest pain would survive if all received thrombolytic therapy, whereas 91.8% would survive if treated according to the ECG algorithm. Data summarizing some of their findings are given in the section titled, “Measuring the Accuracy of Diagnostic Procedures.” We use some of these findings to illustrate sensitivity and specificity.
Congestive heart failure (CHF) is often difficult to diagnose in the acute care setting because symptoms are nonspecific and physical findings are not sensitive enough. B-type natriuretic peptide is a cardiac neurohormone secreted from the ventricles in response to volume expansion and pressure overload. Previous studies suggest it may be useful in distinguishing between cardiac and noncardiac causes of acute dyspnea. Maisel and colleagues (2002) with investigators from nine medical centers conducted a multinational trial to evaluate the use of B-type natriuretic peptide measurements in the diagnosis of CHF. A total of 1586 patients with the primary complaint of shortness of breath were evaluated in the emergency departments of the participating study centers; physicians assessed the probability that the patient had CHF without knowledge of the results of measurement of B-type natriuretic peptide. They used receiver operating characteristic (ROC) curves to evaluate the diagnostic value of B-type natriuretic peptide and concluded it was the single best predictor of the presence or absence of CHF. It was more accurate than either the NHANES criteria or the Framingham criteria for CHF, the two most commonly used sets of criteria for diagnosing CHF.
Lead poisoning is an important disease among children. Exposure, often from house dust contaminated from crumbling old, lead-based paint, may be associated with a range of health effects, from behavioral problems and learning disabilities, to seizures and death. An estimated 21% of housing in the United States has deteriorated lead-based paint and is home to one or more children under 6 years of age. Nearly 900,000 children have blood lead (BPb) levels > 10 μg/dL, the level of concern established by the CDC. What are the costs and benefits of housing policy strategies developed to prevent additional cases of childhood lead poisoning?
Dr. M. J. Brown of the Harvard School of Public Health developed a cost–benefit analysis comparing two policy strategies for reducing lead hazards in housing of lead-poisoned children (2002). She used data from a historical cohort study she had undertaken previously that analyzed data on all lead-poisoned children in two adjacent urban areas over a 1-year period. The two areas were similar except that one employed a strict enforcement of housing code in residential buildings where lead-exposed children lived and the other had limited enforcement of codes. She used a decision tree model to compare costs and benefits of “strict” versus “limited” enforcement of measures to reduce residential lead hazards. Outcome measures were: (1) short-term medical and special education costs associated with an elevated BPb level in one or more additional children after the initial case and (2) the long-term costs of decreased employment and lower occupational status associated with loss of IQ points as result of lead exposure.
She found that the risk of finding additional children with lead poisoning in the same building was 4.5 times greater when the “limited” building code enforcement strategy was used. The cost to society of recurrent BPb level elevations in residential units where lead-poisoned children were identified was greater than the cost of abatement.
Invasive carcinoma of the cervix occurs in about 15,000 women each year in the United States. About 40% ultimately die of the disease. Cervical carcinoma in situ is diagnosed in about 56,000 women annually, resulting in approximately 4800 deaths. Papanicolaou (Pap) smears play an important role in the early detection of cervical cancer at a stage when it is almost always asymptotic.
Although the American Cancer Society recommends annual Pap smears for at least 3 years beginning at the onset of sexual activity or 18 years of age, then less often at the discretion of the physician, only 12–15% of women undergo this procedure. The Pap smear is considered to be a cost-effective tool, but certainly imperfect—it has a sensitivity rate of only 75–85%. New technologies have improved the sensitivity of Pap testing but at an increased cost per test. Brown and Garber (1999) assessed the cost-effectiveness of three new technologies in the prevention of cervical cancer morbidity and mortality.
Introduction
“Decision making” is a term that applies to the actions people take many times each day. Many decisions—such as what time to get up in the morning, where and what to eat for lunch, and where to park the car—are often made with little thought or planning. Others—such as how to prepare for a major examination, whether or not to purchase a new car and, if so, what make and model—require some planning and may even include a conscious outlining of the steps involved. This chapter addresses the second type of decision making as applied to problems within the context of medicine. These problems include evaluating the accuracy of diagnostic procedures, interpreting the results of a positive or negative procedure in a specific patient, modeling complex patient problems, and selecting the most appropriate approach to the problem. These topics are very important in using and applying evidence-based medicine; they are broadly defined as methods in medical decision making or analysis. They are applications of probabilistic and statistical principles to individual patients, although they are not usually covered in introductory biostatistics textbooks.
Medical decision making has become an increasingly important area of research in medicine for evaluating patient outcomes and informing health policy. More and more quality-assurance articles deal with topics such as evaluating new diagnostic procedures, determining the most cost-effective approach for dealing with certain diseases or conditions, and evaluating options available for treatment of a specific patient. These methods also form the basis for cost–benefit analysis.
Correct application of the principles of evidence-based medicine helps clinicians and other health care providers make better diagnostic and management decisions. Kirkwood and colleagues (2002) discuss the abuse of statistics in evidence-based medicine.
Those who read the medical literature and wish to evaluate new procedures and recommended therapies for patient care need to understand the basic principles discussed in this chapter.
We begin the presentation with a discussion of the threshold model of decision making, which provides a unified way of deciding whether to perform a diagnostic procedure. Next, the concepts of sensitivity and specificity are defined and illustrated. Four different methods that lead to equivalent results are presented. Then, an extension of the diagnostic testing problem in which the test results are numbers, not simply positive or negative, is given using the ROC curves. Finally, more complex methods that use decision trees and algorithms are introduced.
Evaluating Diagnostic Procedures with the Threshold Model
Consider the patient described in Presenting Problem 1, the 57-year-old man who is concerned about increasing low back pain. Before deciding how to proceed with diagnostic testing, the physician must consider the probability that the man has a spinal malignancy. This probability may simply be the prevalence of a particular disease if a screening test is being considered. If a history and a physical examination have been performed, the prevalence is adjusted, upward or downward, according to the patient’s characteristics (eg, age, gender, and race), symptoms, and signs. Physicians use the term “index of suspicion” for the probability of a given disease prior to performing a diagnostic procedure; it is also called the prior probability. It may also be considered in the context of a threshold model (Pauker and Kassirer, 1980).
The threshold model is illustrated in Figure 12–1A. The physician’s estimate that the patient has the disease, from information available without using the diagnostic test, is called the probability of disease. It helps to think of the probability of disease as a line that extends from 0 to 1. According to this model, the testing threshold, Tt, is the point on the probability line at which no difference exists between the value of not treating the patient and performing the test. Similarly, the treatment threshold, Trx, is the point on the probability line at which no difference exists between the value of performing the test and treating the patient without doing a test. The points at which the thresholds occur depend on several factors: the risk of the diagnostic test, the benefit of the treatment to patients who have the disease, the risk of the treatment to patients with and without the disease, and the accuracy of the test.
Figure 12–1B illustrates the situation in which the test is quite accurate and has very little risk to the patient. In this situation, the physician is likely to test at a lower probability of disease as well as at a high probability of disease. Figure 12–1C illustrates the opposite situation, in which the test has low accuracy or is risky to the patient. In this case, the test is less likely to be performed. Pauker and Kassirer further show that the test and treatment thresholds can be determined for a diagnostic procedure if the risk of the test, the risk and the benefit of the treatment, and the accuracy of the test are known.
Measuring the Accuracy of Diagnostic Procedures
The accuracy of a diagnostic test or procedure has two aspects. The first is the test’s ability to detect the condition it is testing for, thus being positive in patients who actually have the condition; this is called the sensitivity of the test. If a test has high sensitivity, it has a low false-negative rate; that is, the test does not falsely give a negative result in many patients who have the disease.
Sensitivity can be defined in many equivalent ways: the probability of a positive test result in patients who have the condition; the proportion of patients with the condition who test positive; the true-positive rate. Some people use aids such as positivity in disease or sensitive to disease to help them remember the definition of sensitivity.
The second aspect of accuracy is the test’s ability to identify those patients who do not have the condition, called the specificity of the test. If the specificity of a test is high, the test has a low false-positive rate; that is, the test does not falsely give a positive result in many patients without the disease. Specificity can also be defined in many equivalent ways: the probability of a negative test result in patients who do not have the condition; the proportion of patients without the condition who test negative; 1 minus the false-positive rate. The phrases for remembering the definition of specificity are negative in health or specific to health.
Sensitivity and specificity of a diagnostic procedure are commonly determined by administering the test to two groups: a group of patients known to have the disease (or condition) and another group known not to have the disease (or condition). The sensitivity is then calculated as the proportion (or percentage) of patients known to have the disease who test positive; specificity is the proportion of patients known to be free of the disease who test negative. Of course, we do not always have a gold standard immediately available or one totally free from error. Sometimes, we must wait for autopsy results for definitive classification of the patient’s condition, as with Alzheimer’s disease.
In Presenting Problem 2, Shlipak and colleagues (1999) wanted to evaluate the accuracy of several ECG findings in identifying patients with an MI. They identified 83 patients who had presented 103 times between 1994 and 1997 with chest pain. It was subsequently found that 31 patients had an MI and 72 did not. The investigators reviewed the ECG findings and noted the features present; information is given in Table 12–1.
ECG Characteristic | Number of Positive Tests Presentations with MI | Number of Negative Tests Presentations without MI |
---|---|---|
ST elevation ≥1 mm in concordant leadsa | 2 | 72 |
ST depression ≥1 mm in leads V1, V2, V3a | 1 | 72 |
ST elevation ≥5 mm in discordant leadsa | 6 | 59 |
Let us use the information associated with ST segment elevation ≥ 5 mm in discordant leads to develop a 2 × 2 table from which we can calculate sensitivity and specificity of this finding. Table 12–2 illustrates the basic setup for the 2 × 2 table method. Traditionally, the columns represent the disease (or condition), using D+ and D– to denote the presence and absence of disease (MI, in this example). The rows represent the tests, using T+ and T– for positive and negative test results, respectively (ST segment elevation ≥ 5 mm or < 5 mm).
True-positive (TP) results go in the upper left cell, the T+D+ cell. False-positives (FP) occur when the test is positive but no ST segment elevation is present, the upper right T+D– cell. Similarly, true-negatives (TN) occur when the test is negative in patient presentations that do not have an MI, the T–D– cell in the lower right; and false-negatives (FN) are in the lower left T–D+ cell corresponding to a negative test in patient presentations with an MI.
In Shlipak and colleagues’ study, 31 patient presentations were positive for an MI; therefore, 31 goes at the bottom of the first column, headed by D+. Seventy-two patient presentations were without an MI, and this is the total of the second (D–) column. Because 6 ECGs had an ST elevation ≥ 5 mm in discordant leads among the 31 presentations with MI, 6 goes in the T+D+ (true-positive) cell of the table, leaving 25 of the 31 samples as false-negatives. Among the 72 presentations without MI, 59 did not have the defined ST elevation, so 59 is placed in the true-negative cell (T–D–). The remaining 13 presentations are called false-positives and are placed in the T+D– cell of the table. Table 12–3 shows the completed table.
Using Table 12–3, we can calculate sensitivity and specificity of the ECG criterion for development of an MI. Try it before reading further. (The sensitivity of an ST elevation ≥ 5 mm in discordant leads is the proportion of presentations with MI that exhibit this criterion, 6 of 31, or 19%. The specificity is the proportion of presentations without MI that do not have the ST elevation, 59 of 72, or 82%.)
Using Sensitivity & Specificity to Revise Probabilities
The values of sensitivity and specificity cannot be used alone to determine the value of a diagnostic test in a specific patient; they are combined with a clinician’s index of suspicion (or the prior probability) that the patient has the disease to determine the probability of disease (or nondisease) given knowledge of the test result. An index of suspicion is not always based on probabilities determined by experiments or observations; sometimes, it must simply be a best guess, which is simply an estimate lying somewhere between the prevalence of the disease being investigated in this particular patient population and certainty. A physician’s best guess generally begins with baseline prevalence and then is revised upward (or downward) based on clinical signs and symptoms. Some vagueness is acceptable in the initial estimate of the index of suspicion; in the section titled, “Decision Analysis,” we discuss a technique called sensitivity analysis for evaluating the effect of the initial estimate on the final decision.
We present four different methods because some people prefer one method to another. We personally find the first method, using a 2 × 2 table, to be the easiest in terms of probabilities. The likelihood ratio method is superior if you can think in terms of odds, and it is important for clinicians to understand because it is used in evidence-based medicine. You can use the method that makes the most sense to you or is the easiest to remember and apply.
In Presenting Problem 1, a decision must be reached on whether to order an ESR or proceed directly with imaging studies (lumbar MRI). This decision depends on three pieces of information: (1) the probability of spinal malignancy (index of suspicion) prior to performing any tests; (2) the accuracy of ESR in detecting malignancies among patients who are subsequently shown to have spinal malignancy (sensitivity); and (3) the frequency of a negative result for the procedure in patients who subsequently do not have spinal malignancy (specificity).
What is your index of suspicion for spinal malignancy in this patient before the ESR? Considering the age and history of symptoms in this patient, a reasonable prior probability is 20–30%; let us use 20% for this example.
How will this probability change with the positive ESR? With a negative ESR? To answer these questions, we must know how sensitive and specific the ESR is for spinal malignancy and use this information to revise the probability. These new probabilities are called the predictive value of a positive test and the predictive value of a negative test, also called the posterior probabilities. If positive, we order a lumbar MRI and, if negative, a radiograph, according to the decision rules used by Joines and colleagues (2001). Then we must repeat the process by determining the predictive values of the lumbar MRI or radiograph to revise the probability after interpreting the ESR.
The first step in the 2 × 2 table method for determining predictive values of a diagnostic test incorporates the index of suspicion (or prior probability) of disease. We find it easier to work with whole numbers rather than percentages when evaluating diagnostic procedures. Another way of saying that the patient has a 20% chance of having a spinal malignancy is to say that 200 out of 1000 patients like this one would have spinal malignancy. In Table 12–4, this number (200) is written at the bottom of the D+ column. Similarly, 800 patients out of 1000 would not have a fetus with anomalies, and this number is written at the bottom of the D– column.
The second step is to fill in the cells of the table by using the information on the test’s sensitivity and specificity. Table 12–4 shows that the true-positive rate, or sensitivity, corresponds to the T+D+ cell (labeled TP). Joines and colleagues (2001) reported 78% sensitivity and 67% specificity for the ESR in detecting spinal malignancy. Based on their data, 78% of the 200 patients with spinal malignancy, or 156 patients are true-positives, and 200 – 156 = 44, are false-negatives (Table 12–5). Using the same reasoning, we find that a test that is 67% specific results in 536 true-negatives in the 800 patients without spinal malignancy, and 800 – 536 = 264 false-positives.
Disease | ||
---|---|---|
Test | D+ | D– |
(TP) | (FP) | |
T+ | 156 | 264 |
(FN) | (TN) | |
T– | 44 | 536 |
200 | 800 |
The third step is to add across the rows. From row 1, we see that 156 + 264 = 420 people like this patient would have a positive ESR (Table 12–6). Similarly, 580 patients would have a negative ESR.
A. Completed Table | |||
---|---|---|---|
Disease | |||
Test | D+ | D– | |
(TP) | (FP) | ||
T+ | 156 | 264 | 420 |
(FN) | (TN) | ||
T– | 44 | 536 | 580 |
200 | 800 | 1000 | |
B. Step 4 | |||
Predictive value of a positive test | PV+ = TP/(TP + FP) = 156/420 | ||
= 0.371 | |||
Predictive value of a negative test | PV– = TN/(TN + FN) = 536/580 | ||
= 0.924 |
The fourth step involves the calculations for predictive values. Of the 420 people with a positive test, 156 actually have spinal malignancy, giving 156/420 = 37%. Similarly, 536 of the 580 patients with a negative test, or 92%, do not have spinal malignancy. The percentage 37% is called the predictive value of a positive test, abbreviated PV+, and gives the percentage of patients with a positive test result who actually have the condition (or the probability of spinal malignancy, given a positive ESR). The percentage 92% is the predictive value of a negative test, abbreviated PV–, and gives the probability that the patient does not have the condition when the test is negative. Two other probabilities can be estimated from this table as well, although they do not have specific names: 264/420 = 0.63 is the probability that the patient does not have the condition, even though the test is positive; and 44/580 = 0.08 is the probability that the patient does have the condition, even though the test is negative.
To summarize so far, the ESR is moderately sensitive and specific for detecting spinal malignancy when used with a low index of suspicion. It provides only a fair amount of information; it increases the probability of spinal malignancy from 20% to 37% when positive, and it increases the probability of no spinal malignancy from 67% to 92% when negative. Thus, in general, tests that have high sensitivity are useful for ruling out a disease in patients when the test is negative; for that reason, most screening tests have high sensitivity.
Now we repeat the previous reasoning for the subsequent procedure, assuming that the man’s ESR was positive; from Table 12–6, we know that the probability of spinal malignancy with a positive ESR is 37%. When a second diagnostic test is performed, the results from the first test determine the prior probability. Based on a positive ESR, 37%, or 370 out of 1000 patients, are likely to have spinal malignancy, and 630 are not. These numbers are the column totals in Table 12–7. Lumbar MRI was shown by Joines and colleagues to be 95% sensitive and 95% specific for spinal malignancy; applying these statistics gives (0.95)(370), or 351.5, true-positives and (0.95)(630), or 598.5, true-negatives. Subtraction gives 18.5 false-negatives and 31.5 false-positives. After adding the rows, the predictive value of a positive lumbar MRI is 351.5/383, or 91.8%, and the predictive value of a negative lumbar MRI is 97% (see Table 12–7).
A. Completed Table | |||
---|---|---|---|
Disease | |||
Test | D+ | D– | |
(TP) | (FP) | ||
T+ | 351.5 | 31.5 | 383 |
(FN) | (TN) | ||
T– | 18.5 | 598.5 | 617 |
370 | 630 | 1000 | |
B. Step 4 | |||
Predictive value of a positive test | PV+ = TP/(TP + FP) = 351.5/383 | ||
= 0.918 | |||
Predictive value of a negative test | PV– = TN/(TN + FN) = 598.5/617 | ||
= 0.970 |
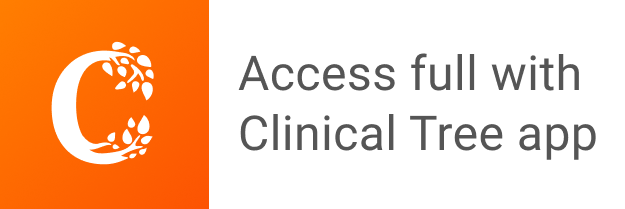