Chi-Squared Test: Large Contingency Tables
The Problem
Individuals can be classified by two factors. For example, one factor may represent disease severity (mild, moderate, severe) and the other factor may represent blood group (A, B, O, AB). We are interested in whether the two factors are associated. Are individuals of a particular blood group likely to be more severely ill?
Assumptions
The data may be presented in an r × c contingency table with r rows and c columns (Table 25.1). The entries in the table are frequencies; each cell contains the number of individuals in a particular row and a particular column. Every individual is represented once, and can only belong in one row and in one column, i.e. the categories of each factor are mutually exclusive. At least 80% of the expected frequencies are greater than or equal to 5.
Table 25.1 Observed frequencies in an r × c table.
Table 25.2 Observed frequencies and assigned scores in a 2 × k table.
Rationale
The null hypothesis is that there is no association between the two factors. Note that if there are only two rows and two columns, then this test of no association is the same as that of two proportions (Chapter 24). We calculate the frequency that we expect in each cell of the contingency table if the null hypothesis is true. As explained in Chapter 24, the expected frequency in a particular cell is the product of the relevant row total and relevant column total, divided by the overall total. We calculate a test statistic that focuses on the discrepancy between the observed and expected frequencies in every cell of the table. If the overall discrepancy is large, then it is unlikely the null hypothesis is true.
Because the approximation to the Chi-squared distribution is reasonable if the degrees of freedom are greater than one, we do not need to include a continuity correction (as we did in Chapter 24).
Refer χ2 to Appendix A3.
If the Assumptions are not Satisfied
If more than 20% of the expected frequencies are less than 5, we try to combine, appropriately (i.e. so that it makes scientific sense), two or more rows and/or two or more columns of the contingency table. We then recalculate the expected frequencies of this reduced table, and carry on reducing the table, if necessary, to ensure that the E ≥ 5 condition is satisfied. If we have reduced our table to a 2 × 2 table so that it can be reduced no further and we still have small expected frequencies, we use Fisher’s exact test (Chapter 24) to evaluate the exact P-value. Some computer packages will compute Fisher’s exact P-values for larger contingency tables.
Chi-Squared Test for Trend
The Problem
Sometimes we investigate relationships in categorical data when one of the two factors has only two categories (e.g. the presence or absence of a characteristic) and the second factor can be categorized into k, say, mutually exclusive categories that are ordered in some sense. For example, one factor might be whether or not an individual responds to treatment, and the ordered categories of the other factor may represent four different age (in years) categories 65–69, 70–74, 75–79 and ≥80. We can then assess whether there is a trend in the proportions with the characteristic over the categories of the second factor. For example, we may wish to know whether the proportion responding to treatment tends to increase (say) with increasing age.
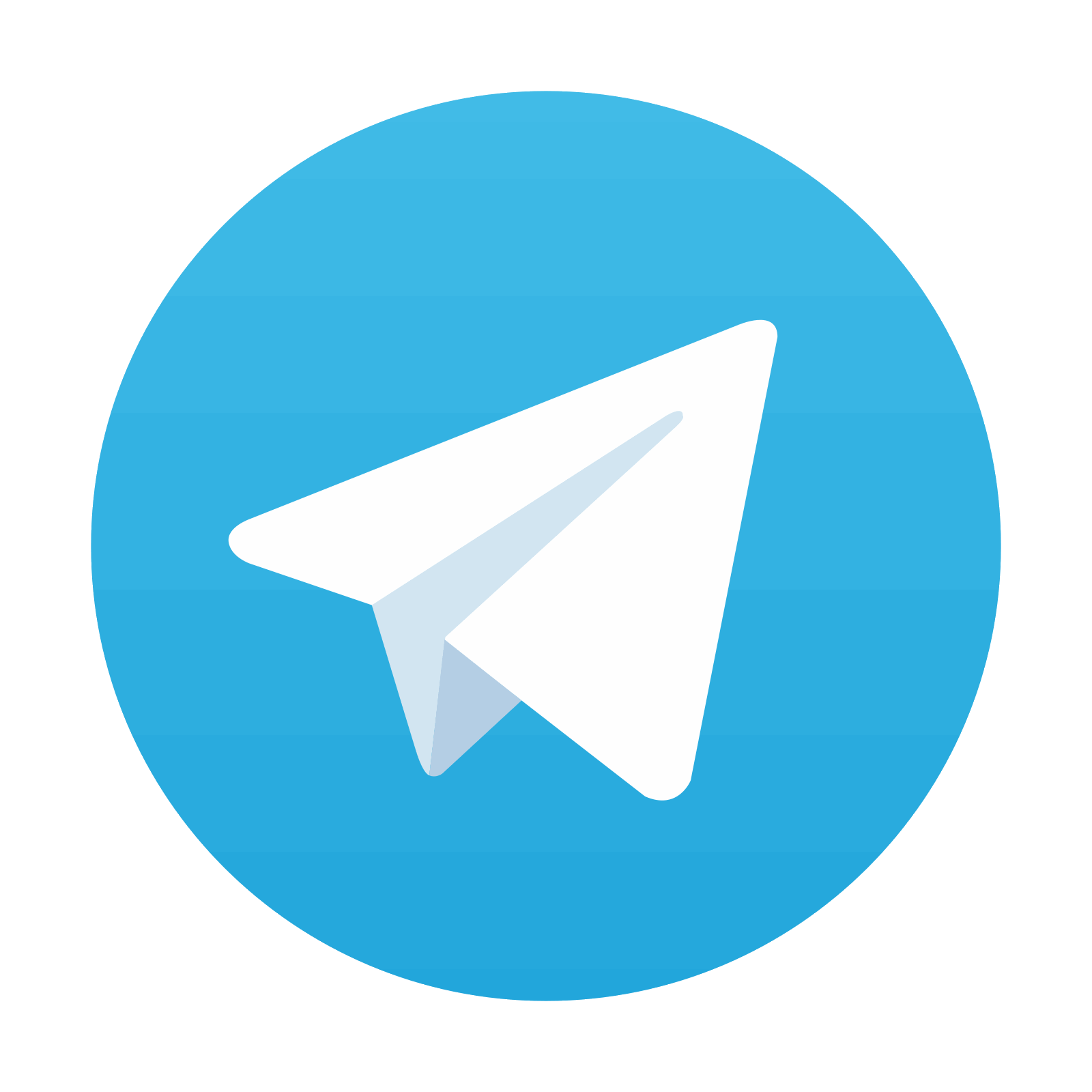
Stay updated, free articles. Join our Telegram channel

Full access? Get Clinical Tree
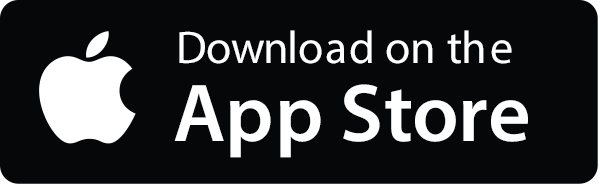
