(1)
Institute of Economic Growth, Delhi University, Delhi, India
Abstract
As was noted in Chap. 1, a good amount of literature at the all India or by states now already exists to suggest that health expenditure in India and some other low-income countries in Asia is considerably large (Bonu et al. 2007; Gottret and Schieber 2006; O’Donnell et al. 2008; Xu et al. 2003, etc.). A great deal of this expenditure—almost three-quarters or in some cases even more—is borne privately by households in many of these countries, in particular those with inadequate health-care systems. In a large number of cases, OOP spending on health causes serious implications for low-income households and affects their sustained living by affecting their normal expenditure pattern, particularly on a host of important nonfood items. A number of these issues have begun to receive much wider attention in India over the past few years, particularly after the seminal report by the NCMH (Ministry of Health and Family Welfare 2005). There has also been a growing concern over these years regarding major policy failures on the part of the centre and state governments in providing adequate resources—physical, financial and human—to meet health-care needs of the people, in particular the poor and the needy. This Commission has also explicitly recognised in its report the prevalence of a very high OOP spending on health in several low-income states—in particular by the households in lowest income deciles—and its role in pushing a significant fraction of households to face poverty and debt trap (see Section 2 of the Commission’s Report, 2005). More or less, a similar inference was drawn in Chap. 3 of this study indicating a large fraction of households sliding below poverty level after incurring OOP expenses on health. Many of them had to borrow from private moneylenders with high repayment liabilities leading to asset divestments.
As was noted in Chap. 1, a good amount of literature at the all India or by states now already exists to suggest that health expenditure in India and some other low-income countries in Asia is considerably large (Bonu et al. 2007; Gottret and Schieber 2006; O’Donnell et al. 2008; Xu et al. 2003, etc.). A great deal of this expenditure—almost three-quarters or in some cases even more—is borne privately by households in many of these countries, in particular those with inadequate health-care systems. In a large number of cases, OOP spending on health causes serious implications for low-income households and affects their sustained living by affecting their normal expenditure pattern, particularly on a host of important nonfood items. A number of these issues have begun to receive much wider attention in India over the past few years, particularly after the seminal report by the NCMH (Ministry of Health and Family Welfare 2005). There has also been a growing concern over these years regarding major policy failures on the part of the centre and state governments in providing adequate resources—physical, financial and human—to meet health-care needs of the people, in particular the poor and the needy.1 This Commission has also explicitly recognised in its report the prevalence of a very high OOP spending on health in several low-income states—in particular by the households in lowest income deciles—and its role in pushing a significant fraction of households to face poverty and debt trap (see Section 2 of the Commission’s Report, 2005). More or less, a similar inference was drawn in Chap. 3 of this study indicating a large fraction of households sliding below poverty level after incurring OOP expenses on health. Many of them had to borrow from private moneylenders with high repayment liabilities leading to asset divestments.
Recently, India has received considerable attention from the world community for its potential to sustain high growth over the coming years. Alongside, however, there has also been a growing concern about serious disparities and unequal distribution of the nation’s wealth (Asian Development Bank 2007). Health disparities and asymmetrically higher burden of health-care expenditure on poorer households have, in particular, remained a major concern in many of the recent studies with focus on issues relating to poverty and unequal sharing of welfare strategies initiated by the governments. The NCMH (2005) has clearly stated in its report that the ‘inequity in the access to and distribution of public health services has been a concern because of the extent of impoverishment households face on account of ill-health and catastrophic illness in particular’ (page 71).
As was highlighted in Chap. 1, over the past one decade or so, there have been several studies based on Indian data to examine catastrophic spending by poor and low-income households on health and some of its correlates (Bonu et al. 2007; Roy and Howard 2007; Ranson et al. 2006; Garg and Karan 2009; Peters et al. 2002). Most of these studies are, however, based on earlier rounds of the National Sample Surveys on household consumption or health expenditure. The study by Bonu et al. (2007) has however relied on most recent NSS (61st round conducted during the 12 months of July 2004 to June 2005) to investigate the incidence, intensity and important correlates of catastrophic health-care payments in India (NSSO 2006a, b). None of these studies have however tried to make use of data drawn from smaller towns and villages like the one collected by us with a particular focus on economically low-performing states and the slum community. An attempt is therefore made in the rest of this chapter to examine the catastrophic nature of spending made by a cross-section of households from the low-income districts of two major states and the capital city of Delhi. The focus of this chapter is largely directed towards three critical issues. These are:
Size of health expenditure by households in relation to their (i) total consumption budget comprising goods and services purchased from market and (ii) nonfood consumption expenditure.
Catastrophic health expenditure by households based on multiple cut-offs or threshold norms. Both total and nonfood consumption expenditures are used to define catastrophe.
Correlates of catastrophic expenditure.
A limitation encountered by studies using head count of catastrophic spending on health ought not to be overlooked. In many cases, this otherwise very useful concept does not include the households unable to access health-care services due to extreme poverty or lack of understanding about certain ailments. There may also be households with a trade-off between OOP health-care spending and the risks of falling into impoverishment. A few may decide to bargain medical treatment against the risk of any further slippage into living standard or long-term consumption poverty. Catastrophe analyses unfortunately exclude all such factors.
5.1 Share of OOP Health-Care Spending in Total and Nonfood Consumption Budget
As noted, this section summarises the magnitude and distribution of OOP health-care spending by a sample of households drawn from selected rural and urban areas of UP (total 1,000 households—750 rural and 250 urban), Rajasthan (650—500 rural and 150 urban) and Delhi (360—102 from identified slums and 258 from non-slums). There are two basic underpinnings that have helped to evolve this entire chapter. First, it tries to highlight further the cascading role played by the OOP payments in squeezing finances available to lower-quintile households and tamper with their budget allocations to different goods and services consumed by the family. Given the asymmetrical nature of intra-household (intra-family) distribution of resources, there are strong possibilities that the aged, women and other weaker members in the family with poor bargaining strength may get disproportionately affected (Agarwal 1990). The second objective obviously is to bring further evidence in support of an emerging consensus among analysts favouring added public resources to improve health care in order to cushion low-income households and bring down the risks of their falling below poverty threshold. Risk pooling measures a bigger proportion of population that must also be paid serious attention with measures to ensure a quicker implementation (Joglekar 2008). Yet another important issue relates to growing drug prices and cascading effect of patenting laws that make medicines simply unaffordable to people in many poor and low-income countries including India.
Tables5.1a, 5.1b, 5.2a and 5.2b distribute households by the mean of their OOP health share in monthly consumption expenditure—both total and nonfood.2 These bivariate tables are further extended to highlight differentials across the observed socioreligious groups including SC, ST, OBC and upper castes as well as the two dominant religious categories in most survey areas, namely, Hindus and Muslims (see Tables 5.1b and 5.2b). As before, these results are presented without going into further desegregations to avoid small-sample biases and ensure sufficient number of observation within each response category.
Table 5.1a
OOP health expenditure as a percentage of the total consumption expenditure: sample households (%)
OOP payments as % of HHDs’ total PCMCE | Total sample | UP | Rajasthan | Delhi | ||||
---|---|---|---|---|---|---|---|---|
Rural | Urban | Rural | Urban | Rural | Urban | Slums | Non-slum | |
Panel 1 | ||||||||
N | 1,250 | 760 | 750 | 250 | 500 | 150 | 102 | 258 |
Mean | 14.9 | 10.6 | 15.2 | 10.5 | 14.5 | 11.3 | 13.8 | 9.0 |
SD | 0.1635 | 0.1446 | 0.1674 | 0.1552 | 0.1575 | 0.1449 | 0.1586 | 0.1251 |
CV | 109.6 | 136.7 | 110.0 | 148.5 | 108.9 | 128.5 | 115.0 | 138.8 |
Panel 2 | ||||||||
Quintile means | ||||||||
Poorest 20 % | 9.4 | 7.9 | 9.7 | 6.3 | 8.9 | 12.2 | 5.9 | 7.3 |
2nd quintile | 10.2 | 9.6 | 10.6 | 7.4 | 9.4 | 14.4 | 12.7 | 8.8 |
3rd quintile | 13.8 | 9.6 | 13.2 | 9.4 | 14.7 | 9.4 | 12.2 | 9.3 |
4th quintile | 17.5 | 12.6 | 18.3 | 19.0 | 16.4 | 6.4 | 13.0 | 11.8 |
Richest 20 % | 23.7 | 13.1 | 27.2 | 29.9 | 20.0 | 19.8 | 25.6 | 8.0 |
Table 5.1b
OOP health expenditure as a percentage of the total consumption expenditure: socioreligious groups (%)
OOP payments as % of HHDs’ total PCMCE | Social groups | Religions | ||||
---|---|---|---|---|---|---|
SC | ST | OBC | Upper caste | Hindu | Muslim | |
Panel 1 | ||||||
N | 455 | 249 | 777 | 529 | 1,789 | 188 |
Mean | 15.8 | 13.8 | 13.4 | 10.7 | 13.5 | 12.3 |
SD | 0.1742 | 0.1355 | 0.1614 | 0.1440 | 0.1588 | 0.1559 |
CV | 110.3 | 98.4 | 120.6 | 134.5 | 117.8 | 126.5 |
Panel 2 | ||||||
Quintile means | ||||||
Poorest 20 % | 9.3 | 9.2 | 9.0 | 8.9 | 9.3 | 7.6 |
2nd quintile | 10.8 | 14.5 | 11.6 | 7.4 | 11.2 | 12.1 |
3rd quintile | 17.6 | 20.1 | 13.7 | 10.2 | 14.9 | 10.3 |
4th quintile | 18.4 | 19.6 | 16.1 | 11.1 | 15.8 | 14.6 |
Richest 20 % | 26.9 | 14.5 | 20.2 | 11.7 | 16.4 | 19.0 |
Table 5.2a
OOP health expenditure as a percentage of nonfood expenditure: sample households (%)
OOP payments as % of HHDs’ nonfood exp. | Total sample | UP | Rajasthan | Delhi | ||||
---|---|---|---|---|---|---|---|---|
Rural | Urban | Rural | Urban | Rural | Urban | Slums | Non-slums | |
N | 1,250 | 760 | 750 | 250 | 500 | 150 | 102 | 258 |
Mean | 31.2 | 19.8 | 32.6 | 20.0 | 29.1 | 21.2 | 27.9 | 15.5 |
SD | 0.2540 | 0.2208 | 0.2615 | 0.2323 | 0.2411 | 0.2329 | 0.2461 | 0.1784 |
CV | 81.4 | 111.7 | 80.2 | 116.3 | 82.9 | 109.9 | 88.1 | 115.2 |
Table 5.2b
OOP health expenditure as a percentage of nonfood expenditure: socioreligious groups (%)
OOP payments as % of HHDs’ nonfood exp. | Social groups | Religions | ||||
---|---|---|---|---|---|---|
SC | ST | OBC | Upper caste | Hindu | Muslim | |
N | 455 | 249 | 777 | 529 | 1,789 | 188 |
Mean | 32.0 | 30.2 | 27.4 | 20.1 | 27.1 | 26.7 |
SD | 0.2599 | 0.2324 | 0.2550 | 0.2193 | 0.2490 | 0.2490 |
CV | 81.2 | 77.1 | 93.1 | 108.9 | 91.7 | 93.1 |
Tables 5.1a and 5.1b provide the share of OOP health spending in total consumption budget—the latter furnishes similar information separately with a break-up by two religious and four social groups. Our results in many cases fail to compare with a few of the earlier studies, suggesting an average of about 5 % of the total consumption budget (and 10 % of the nonfood consumption budget) on OOP health care in India (van Doorslaer et al. 2007; Bonu et al. 2007). Our data indicate a considerably higher OOP mean spending on medical bills in all the three states and their selected villages or towns. Also, this lack of comparison continues in relation to both total and nonfood consumption budgets.
Table 5.1a (panel 1) gives the average share of OOP spending on health in total consumption of households located in rural and urban areas of both the states. Curiously, the mean OOP share of rural households is considerably large. Further, it exceeds the urban share as well. Among the rural households, for example, the mean OOP expenditure varies between 14 and 15 % of the total budget. The same in the urban areas is drawn between 10.5 and a little over 11 %. It may also be noticed from these results that the people from slums, on an average, spend a much larger share of their consumption budget than those from the non-slums (14 % by the slum residents compared to only 9 % by those from non-slums). It strongly suggests a regressive nature of spending if we could assume that all the non-slum households are essentially more affluent. This also reflects a significant departure on our part from the existing body of evidence that suggests that the poor pay less than the nonpoor.
We are nevertheless closer to the existing literature if we compare the mean OOP spending of households by consumption quintiles. While the magnitude of spending remained large, the OOP shares of the rich and poor differ significantly with highest quintile (or top 20 % of households according to their PCMCE) spending almost a quarter of their total consumption budget on health (Table 5.1a, panel 2). In contrast, the same for the bottom 20 % is about 10–12 % in rural and urban areas. The progressivism, as argued in the literature, is therefore maintained.
Table 5.1b provides OOP differentials among four social (SC, ST, OBC and upper castes) and two religious categories—Hindus and Muslims. Judged by social groupings, the lower-caste communities incur much higher OOP payments than their upper-caste counterparts. In terms of religion, though the two respective groups mutually differ, their differences at best remained marginal, i.e. less than a percentage point (Hindus 13.5 % of their total consumption expenditure, while for the Muslims it is given as 12.3 %). The progressivism among five consumption quintiles is also maintained.
Yet another important point to notice from Tables 5.1a and 5.1b is very high variations around the mean OOP. At almost every quintile level or socioreligious grouping, the coefficient of variation is more than 100 %, which tends to indicate extreme values at almost every level, quintile or social groups. It also amounts to suggest that there are households in each category with negligible spending on health services—inpatient or ambulatory.
The differences between the two sets of results—our own and those in the literature cited above—raise an interesting question: Do studies based on macro-data, often regarded as more policy friendly, really provide the realities faced by impoverished households from poor districts or geographical locations? In all fairness, perhaps both have their own merits and ought to be supplemented by each other.
With the mean of OOP payments as high in relation to total consumption expenditure as shown in Tables 5.1a and 5.1b, the same in relation to nonfood consumption expenditure can easily be guessed. It touches around 30 % of the total in rural areas and 20 % in urban areas (Tables 5.2a and 5.2b). In other words, mean of OOP in relation to nonfood expenditure is likely to stand double to that of the total consumption expenditure. The rest of the results follow exactly the same pattern exhibited above and therefore bear more or less similar explanation.
5.2 Catastrophic Health Spending: An Examination
With the mean of OOP health budget in total or nonfood consumption expenditure as high as was demonstrated in the preceding discussion, there is indeed every possibility that a large fraction of the low-income sample households must be facing a catastrophic situation, depending upon how the catastrophe is defined. Using the criterion employed in recent literature on catastrophic health spending—in particular by the WHO—this section is basically designed to examine a couple of these issues.3 It also provides a head count of households faced with a catastrophic situation by both their place of residence and socioreligious characteristics. Intensity of catastrophic health spending, described in the literature as mean payment overshoot (MPO), is also discussed based on our sample data.
5.2.1 Computation of Catastrophic Health Spending: Methodology
Catastrophic health-care payments are defined by analysts as a fraction of total or nonfood consumption expenditure exceeding a certain threshold level. A higher health-care share often severely endangers the consumption level of the entire family and brings it to an economic quandary (Garg and Karan 2009; Bonu et al. 2007; Kawabata et al. 2002). Two levels of threshold OOP spending are generally used to define the catastrophe:
Catastrophe 1: cut-off share of OOP health spending up to or beyond 10 % of the total family or household consumption budget.
Catastrophe 2: OOP health share up to or beyond 40 % of the total family or household nonfood consumption budget.
To simplify the argument, we have slightly deviated from the general practice and used a set of multiple threshold levels (z) for both types of catastrophes—5, 10, 15 and 25 % of the total consumption budget for catastrophe 1 and 15, 25, 40 and 60 % of nonfood consumption budget for catastrophe 2. Algebraically, the following steps are taken to obtain the head count of households with health-care budget share exceeding z:
Step 1:

Step 2:
(where N = 1, 2, …, 2,010 for the total sample and z (assigned with multiple values) denotes the threshold levels of both total and nonfood consumption expenditure). Similarly,
stands for out-of-pocket health payment budget of ith household (
is the mean OOP budget), and hhd i tot _ con refers to total consumption expenditure of the same household. Barring changes in z values, an identical procedure was adopted to measure catastrophe based on nonfood expenditure.
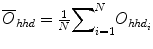


The entire calculations were made on the basis of the total and nonfood per capita monthly consumption expenditure (PCMCE).
5.2.2 Head Count of Catastrophic Spending: Some Results
Table 5.3 gives the socioreligious and state-wise distributions of households exceeding OOP thresholds in relation to their total consumption budget.4 The results are indeed alarming at their face value and pose major challenges for the health planners and institutions engaged in delivery of health-care services. These results clearly indicate that an overwhelming share of households in study areas is facing a serious catastrophic situation because of high OOP expenses on health. To illustrate, at the lowest threshold level, i.e. at 5 % level of total consumption expenditure, there are more than 67 % of the rural and 51 % of the urban households exceeding this limit. The same at the 10 % threshold level—which is generally considered as a catastrophic health spending by most of the analysts—turns out to be 49.5 % in rural areas and 32 % in urban areas. Moreover, our results further indicate that almost a fifth (18.5 %) of the rural households and over a tenth (11.6 %) of the urban households spend more than a quarter of their total consumption budget on health care.
Table 5.3
Catastrophic payment 1: households incurring OOP spending exceeding chosen threshold of the total consumption budget
Catastrophe payments | Sample size (N) | Catastrophe 1: overall consumption thresholds | CV (sd/mean)* 100 | ||||||
---|---|---|---|---|---|---|---|---|---|
Multiple z values | |||||||||
z = 5 % | z = 10 % | z = 15 % | z = 25 % | z = 5 % | z = 10 % | z = 15 % | z = 25 % | ||
Total sample | 2,010 | 61.1 | 42.9 | 29.9 | 15.9 | 79.82 | 115.43 | 153.34 | 230.30 |
Rural | 1,250 | 67.2 | 49.5 | 34.7 | 18.5 | 69.89 | 101.01 | 137.17 | 210.11 |
Urban | 400 | 51.1 | 32.0 | 21.8 | 11.6 | 97.98 | 145.96 | 189.29 | 276.52 |
Slum | 102 | 60.8 | 38.2 | 27.5 | 19.6 | 80.72 | 127.73 | 163.37 | 203.48 |
Non-slum | 258 | 48.4 | 30.2 | 18.6 | 6.2 | 103.35 | 152.21 | 209.57 | 389.66 |
UP | 1,000 | 62.2 | 44.6 | 32.2 | 17.6 | ||||
Rural | 750 | 66.8 | 49.5 | 35.5 | 19.5 | 70.55 | 101.14 | 134.98 | 203.53 |
Urban | 250 | 48.4 | 30.0 | 22.4 | 12.0 | 103.46 | 153.06 | 186.50 | 271.34 |
Rajasthan | 650 | 64.5 | 46.0 | 31.1 | 16.5 | ||||
Rural | 500 | 67.8 | 49.6 | 33.6 | 17.0 | 68.98 | 100.90 | 140.72 | 221.18 |
Urban | 150 | 53.3 | 34.0 | 22.7 | 14.7 | 93.85 | 139.79 | 185.33 | 242.02 |
Delhi | 360 | 51.9 | 32.5 | 21.1 | 10.0 | 96.32 | 144.32 | 193.58 | 300.42 |
Social groups | |||||||||
SC | 455 | 67.5 | 50.3 | 35.6 | 20.9 | 69.51 | 99.45 | 134.63 | 194.88 |
ST | 249 | 69.9 | 50.6 | 34.1 | 16.1 | 65.79 | 99.00 | 139.18 | 229.04 |
OBC | 777 | 60.1 | 42.6 | 30.1 | 16.0 | 81.53 | 116.15 | 152.43 | 229.63 |
Upper caste | 529 | 52.9 | 33.3 | 22.5 | 11.3 | 94.39 | 141.76 | 185.79 | 279.85 |
Religious groups | |||||||||
Hindu | 1,789 | 62.0 | 43.7 | 30.5 | 16.3 | 78.23 | 113.51 | 150.92 | 226.49 |
Muslim | 188 | 55.9 | 38.8 | 27.7 | 13.8 | 89.15 | 125.85 | 162.15 | 250.28 |
How far it would be plausible to a make a generalisation of these results is indeed an issue on which opinion may differ; it nevertheless vindicates the views commonly held that countries with higher incidence of OOP health-care financing are pregnable with a greater risk of catastrophe (van Doorslaer et al. 2007).
Yet another significant observation arising from Table 5.3 is the higher fractions of rural households in both the states with catastrophic health payments (also see Fig. 5.1). The same for the urban areas is turning out to be much less. In other words, it tends to supplement the point suggesting inadequate rural health-care services provided by the government. Low-caste people, particularly the SC communities, are also in the quandary for the same reason. Curiously enough, the share of Muslim households incurring catastrophic spending on health is marginally lower than of the Hindus. How far is this responsible due to their insensitivities towards poor health or how far does it indicate their lack of resources to access health care may however not be judged on the basis of these results. Delhi slum residents are to some extent insulated because of better health-care infrastructure in and around the capital city, and as a result, a lesser proportion of them are found incurring catastrophic payments (Table 5.3). Deviations around the mean are relatively smaller at the higher threshold (z) levels and vice versa.
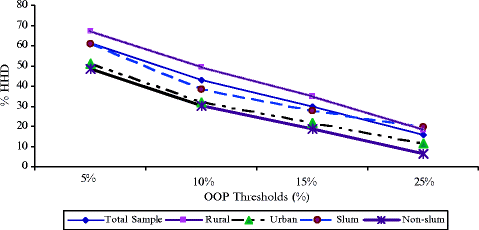
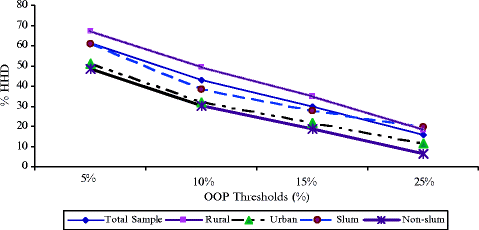
Fig. 5.1
Catastrophe head count: total consumption expenditure N = 2,010 (total sample)
Catastrophe head count 2, computed on the basis of non-sustenance (nonfood) budgets of sample households, repeats the same grim reality and reiterates further that the rural households are worst affected due to inadequate health-care infrastructure of the government (see Fig. 5.2). The lower-caste SC households are at their worst. Their big percentages are shown to incur catastrophic payments, causing them to suffer from serious and highly disproportionate loss of well-being (Table 5.4). Interestingly, the study areas chosen from both the major states (UP and Rajasthan) are mutually close to each other in terms of their population shares facing consumption catastrophe due to private health payments.
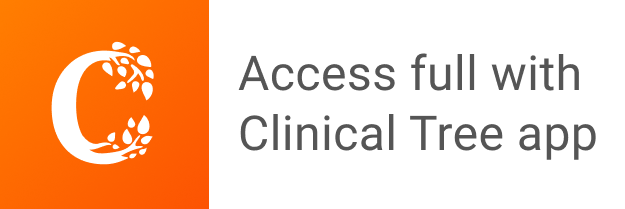