Fig. 1
An intracranial multi-contact platinum iridium electrode (Dixi, Beçancon, France)
Selecting the brain structures to be explored, and the arrangement and locations of the electrodes, requires a careful analysis of all the data collected during the noninvasive presurgical work-up and the formulation of one or more hypotheses concerning the location of the EZ and the trajectories of the seizure discharges. After electrode implantation, a first visual analysis is made of all of the SEEG signals, one electrode at a time, and then a synthetic montage is created using bipolar derivations defined on the basis of the clinical characteristics of the ictal phenomena. The final montage shows all of the selected contacts and, together with MRI, allows the definition of different cortical zones depending on their particular patterns of electrical activity [16].
At least one spontaneous seizure needs to be recorded in order to make an accurate cortical neurophysiological map (see Fig. 2 for a representative example). In a subset of patients, electrical stimulation through intracranial electrodes can also be used to localize eloquent cortical areas, map functions, and, when necessary, elicit seizures. At the end of a SEEG recording, all of the clinical, anatomical, functional, and electrical data are compared in order to obtain the best definition of the EZ [17, 18].


Fig. 2
A spontaneous seizure in right frontal lobe epilepsy (red arrow). The low-voltage fast activity is well located in electrodes O, G, and Z
2.2 Intracranial EEG Signal Analysis
There is a very wide range of methods of analyzing intracranial EEG signals [19, 20] that have been successfully used to study different questions in the field of epilepsy, such as seizure prediction, analysis of the source epileptic activity, and automatic recognition of epileptic transients or seizures [21–23].
In general terms, signal processing methods can be divided into the two main categories of univariate and multivariate methods. The most widely used univariate techniques are based on frequency domain analysis because obvious changes in EEG frequency content are very frequently associated with seizures. Over the last few years, considerable attention has been given to identifying (low-voltage) fast discharges or oscillations in the beta and/or gamma bands that could be a specific electrophysiological signature of epileptogenic areas [24–27]. More recently, technological improvements in digital EEG acquisition systems has made it possible to detect bursts of activity at very high frequencies, up to 500 Hz at the time of seizure onset [28]. On the basis of these observations, EEG activity above 80 Hz (high-frequency oscillations or HFOs) has been increasingly studied in subdural or intracranial EEG recordings with the aim of finding markers of epileptogenicity and identifying the area originating clinical seizures [29–31]. The findings of these studies suggest that HFOs may be potentially useful biomarkers for identifying epileptogenic areas, but their main drawback is that it is not possible to differentiate physiological and pathological HFOs clearly. A further step towards identifying the EZ was made with the introduction of the epileptogenicity index [32], a quantitative measure that is aimed at characterizing the degree of epileptogenicity of the explored brain structures. This index is based on the combination of the spectral (the appearance of fast oscillations replacing background activity) and temporal properties of intracranial EEG signals (the timing of their appearance in relation to seizure onset) and has been successfully used in different types of focal epilepsy [33, 34].
Although univariate indexes seem to be capable of localizing the structures involved early in the ictal process that produce rapid discharges at seizure onset, different approaches based on multivariate signal processing methods and study of the interactions and interdependences between multiple simultaneously recorded signals have been recently introduced. This has led to the concept of brain connectivity (see next section), which describes and quantifies the interdependence and degree of coordination between activities from local, distinct, or distant brain regions.
3 Brain Connectivity
Over the last 20 years, increasing efforts have been made to develop methods aimed at evaluating brain connectivity [35–37]. This has led to the development of various procedures that have different advantages and disadvantages and make different assumptions about the underlying relationship between the analyzed signals. However, the common hypothesis underlying these approaches is that the temporal evolution of the correlation between pairs of signals recorded in different brain regions reflects the connectivity between the regions themselves.
The concept of brain connectivity can be subdivided into three main categories: (1) anatomical (or structural) connectivity, which indicates the set of physical or structural connections linking neurons and may range from local circuits to large-scale pathways connecting distant brain regions; (2) functional connectivity, defined as the temporal correlation expressed in terms of the statistical dependence of spatially remote neuronal populations; and (3) effective connectivity, which refers to the influence that one neural system exerts over another, thus taking into account the direction of the information flow from one region towards another [38, 39]. Effective connectivity can be directly estimated on the basis of the signals (i.e., data driven) or be based on a model specifying causal links (i.e., model based) [14].
Most of the methods of estimating connectivity, particularly those focusing on functional connectivity, are bivariate (pair-wise) [40], which means that they are capable of evaluating the presence of interactions by considering only pairs of signals. However, this can lead to the identification of not only spurious interactions because it does not distinguish direct and indirect relationships but also spurious connections due to the effects of sources common to both signals [20]. Many recent papers have extensively described the advantages and disadvantages of bivariate measures in the fields of functional and effective connectivity [41, 42].
On the contrary, multivariate measures make it possible to explore a wide-ranging network by considering a whole set of signals in the same model. Interest in this field is growing because the quantitative analysis of complex networks is becoming a promising neuroscientific tool. Most of the estimators (mainly nonlinear approaches) belong to the family of methods measuring functional connectivity (see [20, 43] for a review). However, as previously stated, although they are useful in characterizing brain networks, they do not give any information concerning the direction of the interactions or the influence that one system has over another (causality).
In an attempt to overcome these limitations, directed connectivity measures have been developed with the aim of evaluating effective causality. The first definition of causality was given by Wiener in 1956 [44]: “If we can predict the first signal better by using the past information from the second one than by using the information without it, then we call the second signal causal to the first one.” This general definition was formalized by Granger (1969) in terms of predictions in the context of stochastic processes and multivariate regression models of time series. Granger causality [45] was first defined in the field of econometrics for two processes x(t) and y(t). If the knowledge of the past of both x(t) and y(t) reduces the variance of the prediction error of y(t) in comparison with the knowledge of the past of y(t) alone, then the Granger signal x(t) causes the signal y(t). The lack of reciprocity makes it possible to evaluate the direction of the information flow between the signals. Granger’s definition of causality can be easily implemented by means of autoregressive (AR) models and extended to the case of multivariate systems [14, 46].
A number of directed connectivity measures have been developed on the basis of the concept of Granger causality derived from the multivariate autoregressive (MVAR) modelling of multichannel EEG signals [14, 46] and successfully used to study the propagation of seizure activity in patients with focal epilepsies [13, 47–49]. Among them, directed transfer function (DTF) [50] and partial directed coherence (PDC) [51] are effective connectivity measures based on MVAR coefficients transformed into the frequency domain. PDC is particularly interesting because of its ability to distinguish direct and indirect causality flows in the estimated connectivity pattern.
4 Connectivity and EZ Localization
A number of studies of intracranial EEG recordings have developed and applied methods aimed at identifying and quantifying the coupling between the explored areas. These studies were based on the evidence showing that the structures involved in seizure generation are characterized by EEG abnormal transient coupling and changes in connectivity, particularly at the time of seizure onset [52–54]. Recent studies have found enhanced connectivity in the regions including the EZ, thus revealing the existence of highly interconnected nodes or “hubs” that may play a crucial role in the onset and propagation of ictal activity [13, 48, 55]. Moreover, it has been demonstrated that the activity recorded from distant regions shows altered connectivity patterns during seizures [56] and that network topology greatly changes during ictal events [13, 48, 57].
The earliest studies performed in the 1970s [58] used coherence (one of the most widely used bivariate measures) to localize epileptic foci in cats on the basis of the multi-electrode data obtained during a generalized seizure. Subsequently, Takigawa et al. [59] used directed coherence, an index that is capable of detecting the direction of information flow, to investigate bidirectional communication patterns between the frontal and occipital cortex in the scalp EEGs of epileptic patients.
Among the linear multivariate approaches, the en bloc or adapting version of DTF has been used to identify the epileptogenic focus during seizures [47, 49] and to investigate the dynamic changes in effective connectivity at the time of seizure onset [60–62]. Jung et al. [63] used DTF to estimate the ictal onset zone in patients with Lennox–Gastaut syndrome and demonstrated that areas with high average outflow values corresponded well with the surgical resection areas identified by means of conventional methods. Overall, these studies have shown that the multivariate approach is a suitable means of exploring the temporal dynamics of the mechanisms leading to ictal events [64, 65].
Other techniques based on nonlinear approaches have also been validated and used to study epileptic networks. One of these widely used in the field of intracranial EEG is the h2 nonlinear regression index, which was introduced by Pijn and Lopes da Silva [66] and subsequently extended to SEEG by Wendling et al. [67]. This approach has mainly been used to investigate the coupling between the temporal neocortex and limbic structures in patients with temporal lobe epilepsy (TLE), and the results have shown that the regions involved in the EZ establish preferential functional links during seizures. Furthermore, the authors identified several subtypes of TLEs on the basis of the different interactions between temporal and neocortical structures (see [68, 69] for a review of the work done in this field).
Although all of these methods are based on measuring statistical coupling between pairs of signals, they have different underlying theoretical principles and there is no agreement about how they should be carried out. In a recent study, Wendling et al. [70] used simulations to evaluate the advantages and disadvantages of three different families of bivariate methods: linear and nonlinear regression, phase synchronization, and generalized synchronization. Their main conclusion was that there is no “universal” method insofar as none of the methods performed better in all situations, mainly because the results depend on the frequency content of the signals and some methods may be insensitive to the coupling parameters of the system.
Most of the studies of connectivity-based EZ localization have so far mainly concentrated on the ictal and pre-ictal phases, when abnormal critical patterns are more easily detectable; only a few were carried out with the aim of localizing the EZ on the basis of interictal intracranial EEG recordings. Bettus et al. [71] studied the interictal EEG patterns recorded in patients with drug-resistant mesial temporal lobe epilepsy (MTLE) using the h2 nonlinear correlation index and found that the EZ was characterized by decreased power in the theta band and a general increase in signal interdependences in comparison with the results obtained in a group of patients whose seizures originated in other areas. Wilke et al. [48] used adapting DTF, betweenness centrality, and indices derived from graph theory to identify critical network nodes during ictal and interictal electrocorticogram (EcoG) recordings of patients with intractable epilepsy. They observed patterns of altered network interactions (mainly in the gamma band) not only during seizures but also during interictal spike activity and random non-ictal periods.
5 Application in Focal Cortical Dysplasia
In our recent study [13], we evaluated the changes in dynamic connectivity patterns in the SEEG recordings of ten patients with Taylor-type focal cortical dysplasia (type II FCD) under interictal, pre-ictal, and ictal conditions using the PDC and indices derived from graph theory (in- and out-density, and betweenness centrality) in order to characterize the synchronization and connectivity properties of the SEEG signals recorded from inside the dysplasia (i.e., the lesional zone) and distinguish them from those of other regions involved in ictal activity or not.
Type II FCD is characterized by the presence of cortical dyslamination, and dysmorphic neurons and balloon cells. We selected this type of focal epilepsy in order to validate the appropriateness of our approach because this type of dysplasia is intrinsically epileptogenic [72], the EZ normally overlaps the dysplasia, and the clear-cut borders of the anatomical lesion allow its precise exeresis and a good surgical outcome.
To estimate PDC an MVAR model has to be identified. An MVAR model describes a multivariate dataset as a linear combination of its own past plus uncorrelated white noise.
Given a set S = {x n (k), 1 ≤ m ≤ M} of M simultaneously observed stationary time series, the MVAR model with order p is defined as
where A 1, A 2, … A p are the coefficient matrices (dimensions M × M), with the coefficients a ij (r) describing the linear interaction of x j (k − r) on x i (k), and w i (k) represents a random (Gaussian) white noise driving innovation.
![$$ \left[\begin{array}{lll} {x}_1(k)\hfill \\{\hfill} \vdots \hfill \\{\hfill} {x}_M(k)\hfill \end{array}\right]={\displaystyle \sum_{r=1}^p}{A}_r\left[\begin{array}{lll} {x}_1\left(k-r\right)\hfill \\{\hfill} \vdots \hfill \\{\hfill} {x}_M\left(k-r\right)\hfill \end{array}\right]+\left[\begin{array}{lll} {w}_1(k)\hfill \\{\hfill} \vdots \hfill \\{\hfill} {w}_M(k)\hfill \end{array}\right], $$](https://i0.wp.com/basicmedicalkey.com/wp-content/uploads/2017/06/A7657_2013_61_Chapter_Equa.gif?w=960)
The PDC, for each pair of channel i and j, are calculated from the Fourier transform of the MVAR coefficients as
where
with Δt the sampling interval and I the identity matrix of dimension M.


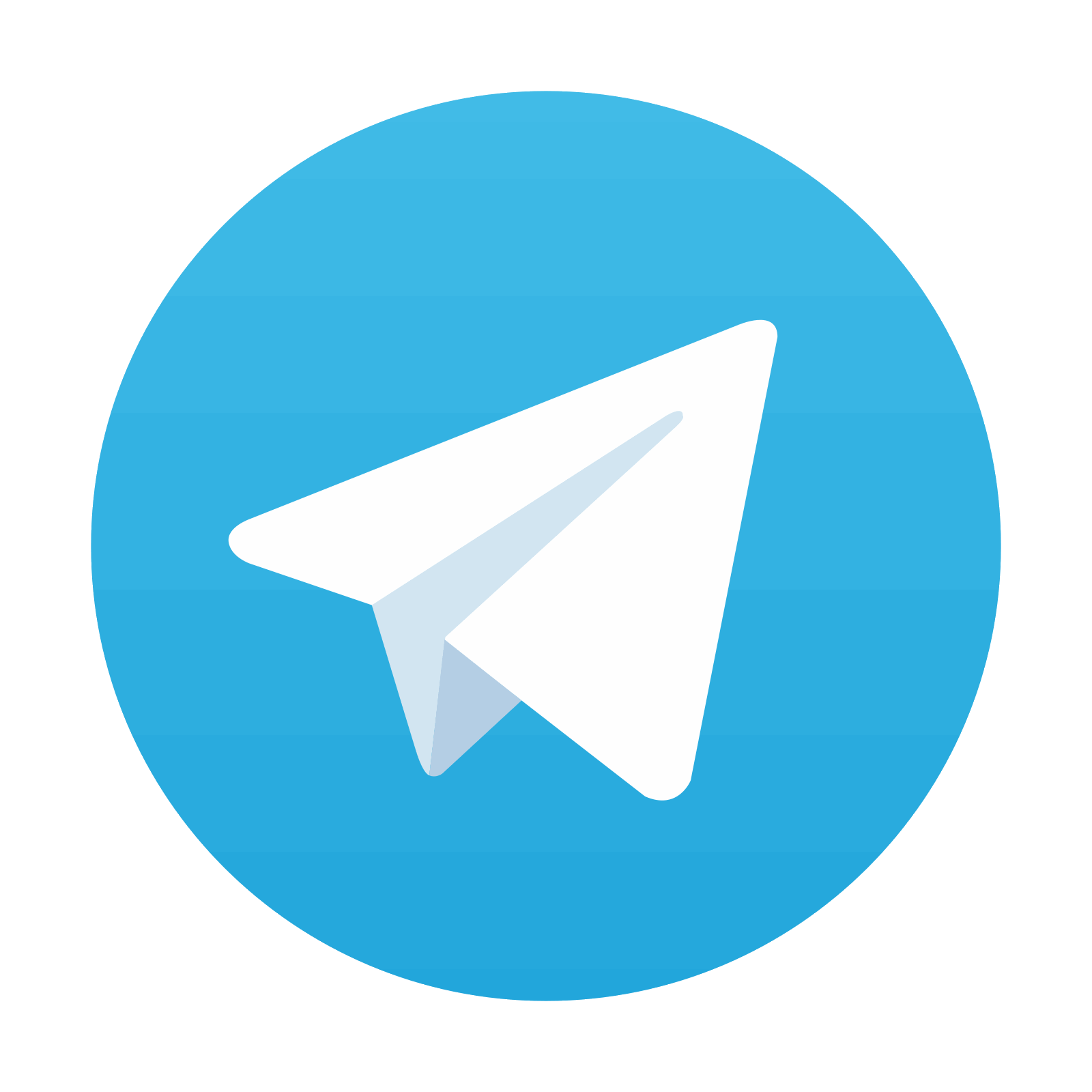
Stay updated, free articles. Join our Telegram channel

Full access? Get Clinical Tree
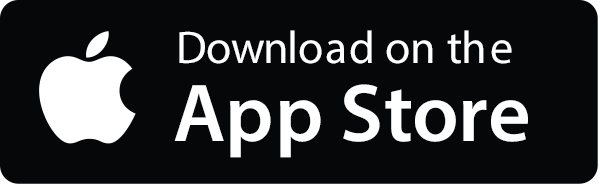
