Chapter Contents
Internal and External Validity 26
Bias 26
Selection Bias 27
Are the Groups Similar in all Important Respects? 27
Information Bias 28
Has Information Been Gathered in the Same Way? 28
Is the Information Bias Random or in One Direction? 29
Confounding 29
Is an Extraneous Factor Blurring the Effect? 29
Oral Contraceptives and Myocardial Infarction (and Smoking) 29
IUDs and Infertility (and Sexually Transmitted Disease) 29
Directed Acyclic Graphs 30
Control for Confounding 30
Restriction 31
Matching 31
Stratification 31
Multivariable Analysis 32
Propensity Scores 32
Sensitivity Analysis 33
Instrumental Variables 33
Chance 33
Judging Associations 34
Bogus, Indirect, or Real? 34
Conclusion 35
Readers of medical literature need to consider two types of validity, internal and external. Internal validity means that the study measured what it set out to; external validity is the ability to generalise from the study to the reader’s patients. Bias is a systematic distortion of the truth. With respect to internal validity, all observational research has some degree of selection bias, information bias, and confounding bias. Selection bias stems from an absence of comparability between groups being studied. The effect of information bias depends on its type. If information is gathered differently for one group than for another, bias results. By contrast, nondifferential misclassification (noise) tends to obscure real differences. Confounding is a mixing or blurring of effects: a researcher attempts to relate an exposure to an outcome but actually measures the effect of a third factor (the confounding variable). Confounding can be controlled in several ways: restriction, matching, stratification, multivariable techniques, and propensity scores. If a reader cannot explain away study results on the basis of selection, information, or confounding bias, then chance might be another explanation. Chance should be examined last, however, as these biases can account for significant, though bogus, results. Differentiation between spurious, indirect, and causal associations can be difficult. Considerations such as temporal sequence, strength and consistency of an association, and evidence of a dose–response effect lend support to a causal link. Unlike the physical sciences, the biological sciences lack absolute truths. In clinical research, all we have are hypotheses. Observational epidemiology is a young science and a blunt tool; its findings should always be viewed as tentative.
Clinicians face two important questions as they read medical research: is the report believable and if so, is it relevant to my practice? Uncritical acceptance of published research has led to serious errors and squandered resources. Based on observational studies, the American Heart Association used to recommend menopausal oestrogen therapy for prevention of heart disease. Taking vitamins B, C, E, and beta-carotene was thought to prevent heart disease. Fibre and folate intake were thought to protect against colorectal cancer. All these hypotheses were refuted by randomised controlled trials. In this chapter, we will discuss two types of validity, describe a simple checklist for readers, and offer some considerations by which to judge reported associations.
Internal and External Validity
Analogous to a laboratory test, a study should have internal validity (i.e., the ability to measure what it sets out to measure). Internal validity is ‘the degree to which a study is free from bias or systematic error’. Errors stemming from random, not systematic error, relate to precision. Given the choice between internal validity and precision, the former usually deserves priority. A valid study result with some imprecision is preferable to a precisely wrong answer due to a huge sample size and inadequate control of bias. Internal validity is the sine qua non of clinical research; extrapolation of invalid results to the broader population is not only worthless but potentially dangerous.
A second important concern is external validity: can results from study participants be extrapolated to the reader’s patients? External validity is defined as ‘the degree to which results of a study may apply, be generalised, or be transported to populations or groups that did not participate in the study’. Because a total enumeration or census approach to medical research is usually impossible, the customary tactic is to choose a sample, study it, and, hopefully, extrapolate the result to one’s practice. Gauging external validity is necessarily more subjective than is assessment of internal validity.
Internal and external validity entail important trade-offs. For example, randomised controlled trials are more likely than observational studies to be free of bias, but because they usually enrol selected participants, external validity can suffer. This problem of atypical participants is also termed distorted assembly. Participants in randomised controlled trials tend to be different (including being healthier) than those who choose not to take part, in part due to eligibility criteria. The filtering process for admission to randomised trials might, therefore, result in an eclectic population no longer representative of the general public.
Bias
Bias undermines the internal validity of research. Unlike the conventional meaning of the word (i.e., prejudice), bias in research denotes a systematic, rather than random, deviation from the truth. All observational studies have built-in bias; the challenge for investigators, editors, and readers is to ferret these out and judge how they might have affected results. Regrettably, randomised controlled trials that do not follow the rules of conduct are vulnerable to bias, and the methodological quality of trials is related to the observed results.
A simple checklist, such as that shown in Panel 3.1 , can be helpful when reading observational study reports.
Is Selection Bias Present?
- ▪
In a cohort study, are participants in the exposed and unexposed groups similar in all important respects except for the exposure?
- ▪
In a case-control study, are cases and controls similar in all important respects except for the disease in question?
Is Information Bias Present?
- ▪
In a cohort study, is information about outcome obtained in the same way for those exposed and unexposed?
- ▪
In a case-control study, is information about exposure gathered in the same way for cases and controls?
Is Confounding Present?
- ▪
Could the results be accounted for by the presence of a factor (e.g., age, smoking, sexual behaviour, diet) associated with both the exposure and the outcome but not directly involved in the causal pathway?
If the Results Cannot Be Explained By These Three Biases, Could They Be the Result of Chance?
- ▪
Is the difference statistically significant, and, if not, did the study have adequate power to find a clinically important difference?
- ▪
What are the relative risk or odds ratio and 95% CI?
- ▪
Does the size of the treatment effect warrant attention, or is it likely due to bias ( Chapter 7 )?
Several glossaries have catalogued biases in clinical research. Sackett’s original compilation included 35 different biases. Since that early effort, the list of potential biases has grown. More recent lists include 69 to 74. We are lumpers, not splitters, and prefer to group all these possible biases into three widely accepted categories: selection, information, and confounding. The common theme for all three is ‘different’. Something ‘different’ systematically distorts the planned comparison.
Selection Bias
Are the groups similar in all important respects?
Selection bias stems from a disparity between groups being studied. For example, in a cohort study, the exposed and unexposed groups differ in some important respect aside from the exposure. Some use the term ‘selection bias’ to indicate that a nonrepresentative sample has been chosen for study. Because this problem would not affect the internal validity of an analytic study, choosing a nonrepresentative sample should be considered a problem with external validity and not a type of bias.
Membership bias is a type of selection bias: people who choose to be members of a group (e.g., joggers) might differ in important respects from others. For instance, both cohort and case-control studies initially suggested that exercising after myocardial infarction prevented repeat infarction. However, a randomised controlled trial failed to confirm this benefit. Those who chose to exercise might have differed in other important ways from those who did not exercise, such as diet, smoking, and presence of angina.
The protective effect of menopausal oestrogen therapy against coronary heart disease consistently found in observational studies was likely due to membership bias: women who chose to be oestrogen takers were healthier in other ways than those who did not. While the Women’s Health Initiative trial has been widely criticised for methodological flaws, the lack of cardioprotection from oestrogen in women more than 10 years into menopause has been corroborated in other trials. However, women who started oestrogen within a decade of menopause enjoyed a reduction in both coronary heart disease and death. Timing made a big difference, a feature missed in the original analysis.
In case-control studies, selection bias implies that cases and controls differ importantly aside from the disease in question. Two types of selection bias have earned eponyms: Berkson and Neyman bias. Also known as an admission-rate bias, Berkson bias (or paradox) results from differential rates of hospital admission for cases and controls. The formal definition is ‘a form of selection bias that arises when the variables whose association is under study affect selection of subjects into the study’. Alternatively, knowledge of the exposure of interest might lead to an increased rate of admission to hospital. For example, doctors who care for women with salpingitis might be more likely to hospitalise those with a telltale IUD string noted on pelvic examination than those without. In a hospital-based case-control study, this would stack the deck (or gynaecology ward) with a high proportion of intrauterine device (IUD)-exposed cases, spuriously increasing the odds ratio.
Neyman bias is an incidence–prevalence bias. It arises when a gap in time occurs between exposure and selection of study participants. This bias crops up in studies of diseases that are quickly fatal, transient, or subclinical. Neyman bias creates a case group not representative of cases in the community. For example, a hospital-based case-control study of myocardial infarction and snow shovelling (the exposure of interest) would miss individuals who died in their driveways and thus never reached a hospital; this might lower the odds ratio of infarction associated with this exposure.
Other types of selection bias include unmasking (detection signal) and nonrespondent bias. An exposure might lead to a search for an outcome, as well as the outcome itself. For example, women with leg pain and known to be taking oral contraceptives are more likely to get a diagnostic workup than are other women. In observational studies, nonrespondents are different from respondents. In Denmark, nonresponders to health surveys have higher mortality rates of alcohol morbidity and mortality than do responders. In the Netherlands, adolescent responders to a survey smoked less, drank less alcohol, and had better health status than did nonresponders.
Loss to follow-up can undermine cohort studies. If participants are lost at random, computer models suggest that even large proportions lost do not bias the results. However, when losses were not random (presumably the usual real-life situation), even small proportions of loss to follow-up introduced serious bias. This underscores the importance of diligent procedures to minimise such losses. Here, an ounce of prevention can be worth a ton of cure.
Information Bias
Has information been gathered in the same way?
Information bias, also known as observation, classification, ascertainment, and measurement bias, is defined as ‘a flaw in measuring exposure, covariate, or outcome variables that results in different quality (accuracy) of information between comparison groups’. In a cohort study or randomised controlled trial, information about outcomes should be obtained the same way for those exposed and unexposed. In a case-control study, information about exposure should be gathered in the same way for cases and controls.
Information bias can arise in many ways. For example, an investigator might gather information about an exposure for a case by bedside interview, but by telephone interview for a community control. The presence of a disease might prompt a more determined search for the putative exposure of interest for cases than for controls. To minimise information bias, detail about exposures in case-control studies should preferably be gathered by researchers unaware of whether the respondent is a case or a control. Similarly, in a cohort study with subjective outcomes, the observer should be unaware of the exposure status of each participant.
In case-control studies that rely on memory of remote exposures, recall bias is inescapable. Cases tend to search their memories to identify what might have caused their disease; healthy controls have no such motivation. Thus better recall among cases and underreporting among controls are routine. In a Swedish case-control study, the association between family history of cancer and lymphoma was consistently stronger based on self-report compared with registry data in Sweden. Many case-control studies have reported an increase in cancer risk after abortion. However, when Swedish investigators compared histories of prior abortions obtained by personal interview with centralised medical records, they documented systematic underreporting of abortions among controls (but not among cases). In cohort studies free from recall bias, induced abortion has had either a protective effect or no effect on risk of breast cancer.
Is the information bias random or in one direction?
The effect of information bias depends on its type. If information is gathered differentially for one group than for another, then bias results, raising or lowering the relative risk or odds ratio dependent on the direction of the bias. By contrast, nondifferential misclassification (i.e., noise in the system) tends to obscure real differences. For example, an ambiguous questionnaire might lead to errors in data collection among cases and controls, shifting the odds ratio towards unity, meaning no association.
Confounding
Is an extraneous factor blurring the effect?
Confounding is a mixing or blurring of effects. A researcher attempts to relate an exposure to an outcome but actually measures the effect of a third factor, termed a confounding variable. A confounding variable is associated with the exposure and it affects the outcome, but it is not an intermediate link in the chain of causation between exposure and outcome. More simply, confounding is a methodological fly in the ointment. Confounding is often easier to understand from examples than from definitions.
Oral contraceptives and myocardial infarction (and smoking)
Early studies of the safety of oral contraceptives reported a pronounced increased risk of myocardial infarction. This association later proved to be spurious, because of the high proportion of cigarette smokers among users of birth control pills. Here, cigarette smoking confounded the relation between oral contraceptives and infarction. Women who chose to use birth control pills also chose, in large numbers, to smoke cigarettes, and cigarettes, in turn, increased the risk of myocardial infarction. Pill users who smoke have a dramatically increased risk of infarction; pill users without cardiovascular risk factors have no increased risk. Although investigators thought they were measuring an effect of birth control pills, they were in fact measuring the confounding effect of smoking among pill users.
IUDs and infertility (and sexually transmitted disease)
Case-control studies of IUDs and infertility nearly drove this highly effective contraceptive off the US market in the 1980s. A reported doubling in the risk of tubal infertility related to IUD use led to dire warnings, a pharmaceutical company bankruptcy, an epidemic of lawsuits, and the disappearance of all copper IUDs. Methodological flaws included failure to control adequately for the potentially confounding effect of sexually transmitted diseases. However, nearly two decades passed until empirical evidence was available. A case-control study of tubal obstruction in Mexico City performed a chlamydia serology test on each case and control. Women who had used an IUD in the past had no increase in the risk of tubal obstruction; in contrast, nonusers with serological evidence of prior infection had a significant increase in risk (odds ratio 2.4; 95% CI 1.7–3.2).
Directed Acyclic Graphs
These causal diagrams, also termed causal graphs and path diagrams, are defined as ‘a graphical display of causal relations among variables, in which each variable is assigned a fixed location on the graph (called a node), and in which each direct causal effect of one variable on another is represented by an arrow with its tail at the cause and its head at the effect’. The descriptor ‘acyclic’ indicates no feedback loop; no variable can affect itself ( Fig. 3.1 ). These graphs are sometimes used to help identify when to control for variables in the causal mix. Free software is available for creating directed acyclic graphs (DAGs).

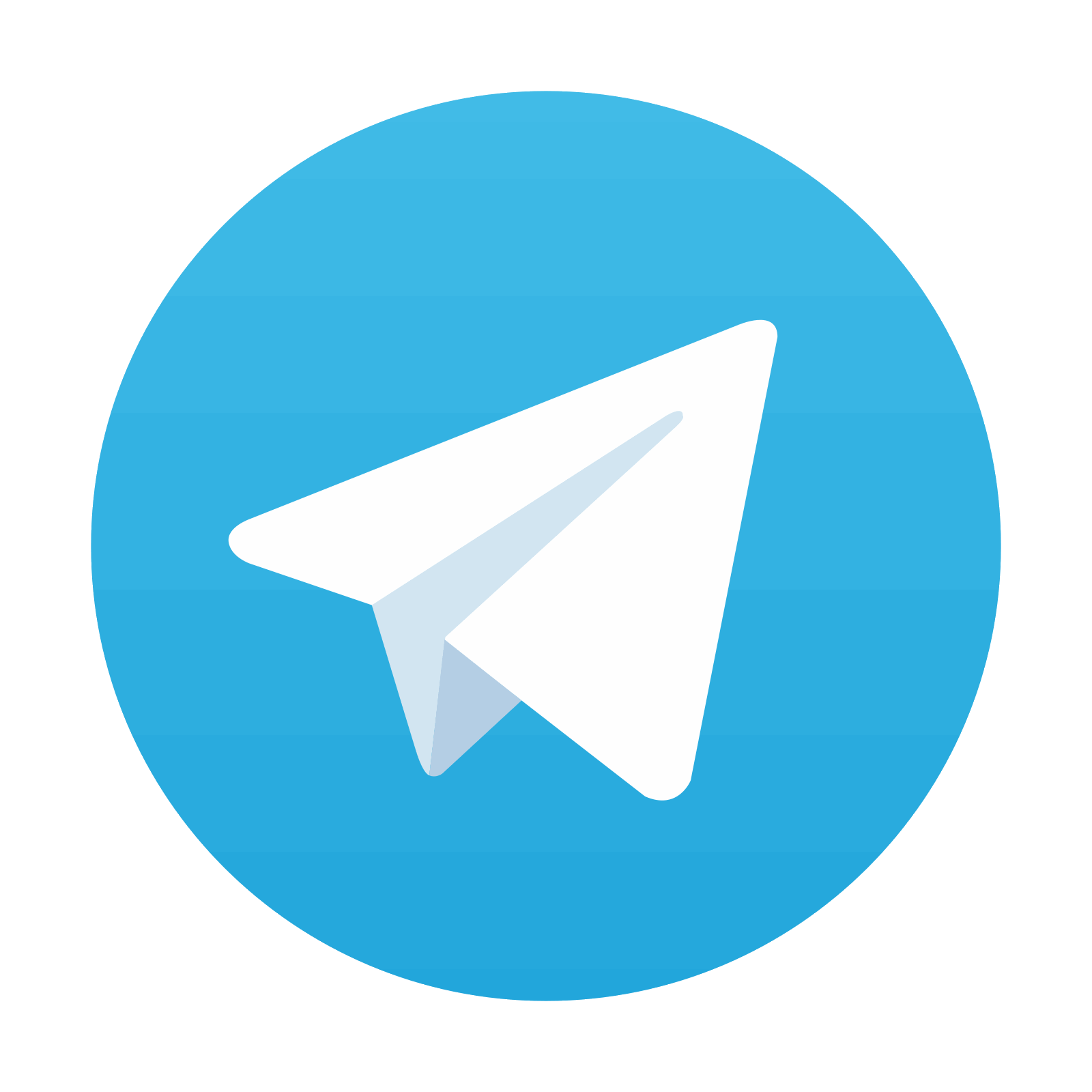
Stay updated, free articles. Join our Telegram channel

Full access? Get Clinical Tree
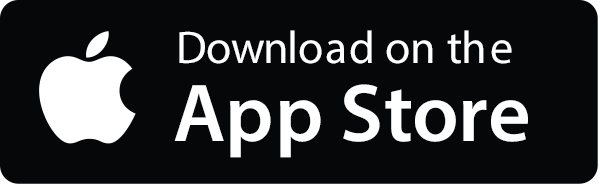

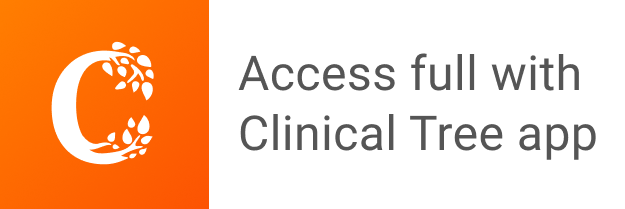