html xmlns=”http://www.w3.org/1999/xhtml” xmlns:mml=”http://www.w3.org/1998/Math/MathML” xmlns:epub=”http://www.idpf.org/2007/ops”>
Bias is the single biggest potential problem in clinical research. Bias means that the results observed are consistently influenced away from the true result. Random variation can also affect the results, but bias is different because it consistently influences the results in one direction. If a study is biased, it cannot be valid. Bias is like an infection, which can kill an otherwise healthy study. Even the accusation that bias might exist can cause the validity of the study to be questioned and the results discounted. Therefore, it is critical that a study include measures to avoid not only bias but also the potential appearance of bias. We do not know of any investigators who would consciously bias a study to get specific results, but there are subtle ways in which bias can sneak in to a study. In Chapter 18 we discuss methods to avoid biases.
17.1 Common Sources of Bias
The following sections describe the most common types of bias that can occur during a study. Although these are described separately, there is considerable overlap and interaction between the different categories of bias. A caregiver’s perception of how a participant is doing may affect the participant’s perception of what is being done to her, and if the caregiver is biased, then the participant’s responses may become biased. Some of the biases described apply primarily to interventional studies, while other biases are more often associated with observational studies. Some problems in a study only become biases when they occur differentially between groups. Most importantly, many biases can infect any type of study.
We include publication bias, although it is not a design bias, because it is so often referred to in the literature.
17.1.1 Prognostic Bias
Prognostic bias (also called allocation bias) occurs in an interventional study when participants are selected or assigned to treatment based on certain characteristics. The characteristics in this discussion are different from those specified in the inclusion and exclusion criteria (Chapter 12); instead, they are characteristics that would influence the results of the study. Assignment of healthier appearing participants to the new treatment would create a bias in favor of the new treatment. Although this specific bias should not occur in a properly implemented randomized interventional study, it can arise when the study is not randomized. Importantly, randomization implies that the treatment assignment of a participant is independent of the participant’s characteristics and can be known only after the participant is enrolled in the study (Section 20.1).
In a comparative study of high-dose oral fluconazole versus standard of care therapy for cryptococcal meningitis in AIDS patients, the investigators might use the oral fluconazole in all participants able to take oral medications, while participants unable to take oral medications received the standard of care (an IV therapy). Thus, healthier participants would be assigned to the oral fluconazole group rather than to the standard of care group. Such a study would be very biased.
Prognostic bias is much more common in observational studies. A case-control study can be biased when the control population is different from the cases in ways that affect the outcome. In Chapter 26 we state that controls should be as similar to the cases as possible, with the only differences being those of outcome. If the controls have healthier lifestyles or a healthier environment than the cases, then the study is biased in favor of a larger difference between the groups, which could be attributed falsely to exposures. If the controls are worse on these factors, then the study is biased in the other direction: a difference due to the exposures of interest may be obscured by the adverse effect of the controls’ general environment.
In a case-control study of adult males with a congenital hormone imbalance, data was collected from a multi-item questionnaire and the focus was on social and cognitive problems. The cases were to be compared to a group of “healthy” men – those without the imbalance or related problems – with the same age distribution. The investigators recruited employees from their institution for controls. When the data was examined, it was seen that almost all the controls in the study had a bachelor’s degree or at least some kind of training certificate and therefore probably did not have cognitive problems. Therefore, the study was biased in favor of finding a difference in cognitive function between cases and controls.
A cohort study may be biased by the selection of the cohort or by differences between groups in a comparative cohort study. A bias can occur in comparing ethnic groups when one group has a higher socioeconomic index than the other, which may result in a healthier lifestyle, improved access to health care, or other factors that would influence their outcome. This is similar to the type of bias noted earlier in this section for case-control studies. Even within a single cohort, if the subgroup of unexposed participants differs from the subgroup of exposed participants in ways other than the exposures of interest, then the result may be biased either for or against a particular result.
The Million Women Study was a cohort study to determine if there was a link between hormone replacement therapy (HRT) and breast cancer. The cohort was comprised of women who were invited to enroll when they had routine mammography. They were followed up for breast cancer incidence and death. The study showed an increased risk of cancer in women who used HRT. However, other investigators observed that women who have routine mammography are not representative of the general population; they are more likely to be using HRT and also more likely to be at risk for breast cancer. Thus the study was biased toward finding an association that may not exist in the general population.
In this famous example an investigator tried to show that high doses of vitamin C were an effective treatment for cancer. The investigators treated 100 advanced cancer patients with vitamin C, beginning the treatment at the time when they were diagnosed as “untreatable,” and recorded their survival history. The control group of 1,000 patients was selected from records of patients from the same hospital who were not treated with vitamin C, and the researchers computed their survival time from when they were diagnosed as untreatable. However, further investigation showed that the two groups were not comparable. In addition to not being matched by important prognostic factors, the average time from the initial diagnosis to “untreatable” status was shorter in the patients treated with vitamin C. Therefore, they would have begun vitamin C treatment earlier after the diagnosis of their disease, and thus the study was biased toward their living longer. Subsequent properly designed analyses showed no benefit of vitamin C in cancer treatment.
Historical controls can be a source of prognostic bias due to the fact that circumstances change over time. Changes in the diagnostic criteria, environmental factors, and population demographics may introduce systematic differences even though the major criteria appear to be the same. The methods for measuring key parameters and the standards for defining normal laboratory measurements may change over time.
As part of a study of the effects of changes in the American diet, investigators wanted to determine if the prevalence of glucose abnormalities had changed. However, the standards for diagnosing Type II diabetes or impaired glucose tolerance from a fasting glucose or Oral Glucose Tolerance Test have changed over the years, with the threshold for diabetes or impaired glucose tolerance being reduced. Thus, the same glucose levels could cause an individual to be identified as “normal” in a historical control population but to be diagnosed as “abnormal” in the current population, biasing the study toward showing that the rate of abnormalities had increased in the current population.
17.1.2 Selection Bias
Selection bias can refer either to self-selection of individuals to be a participant in a research study or selection by the investigators of study participants to support a particular hypothesis, which would be a nonrandom sample. Selection bias is obviously related to prognostic bias: the major difference is that selection bias refers to bias in the overall study population, whereas prognostic bias generally refers to bias in selecting or assigning groups for comparison.
In 1998, a British researcher published a paper claiming that there was a link between the administration of the measles, mumps, and rubella vaccine and the appearance of autism and bowel disease. The paper reported on 12 children who were observed by this pediatrician before and after having the vaccination. This link could not be reproduced in other studies, and further investigation showed that the original paper had many flaws. These included the fact that 5 of the 12 children showed symptoms of neurological problems before they were given the vaccination. Since the researcher was also paid by lawyers who were acting for parents suing the providers of the vaccines, the integrity of the researcher was questioned and his choice of participants was seen as a case of selection bias.
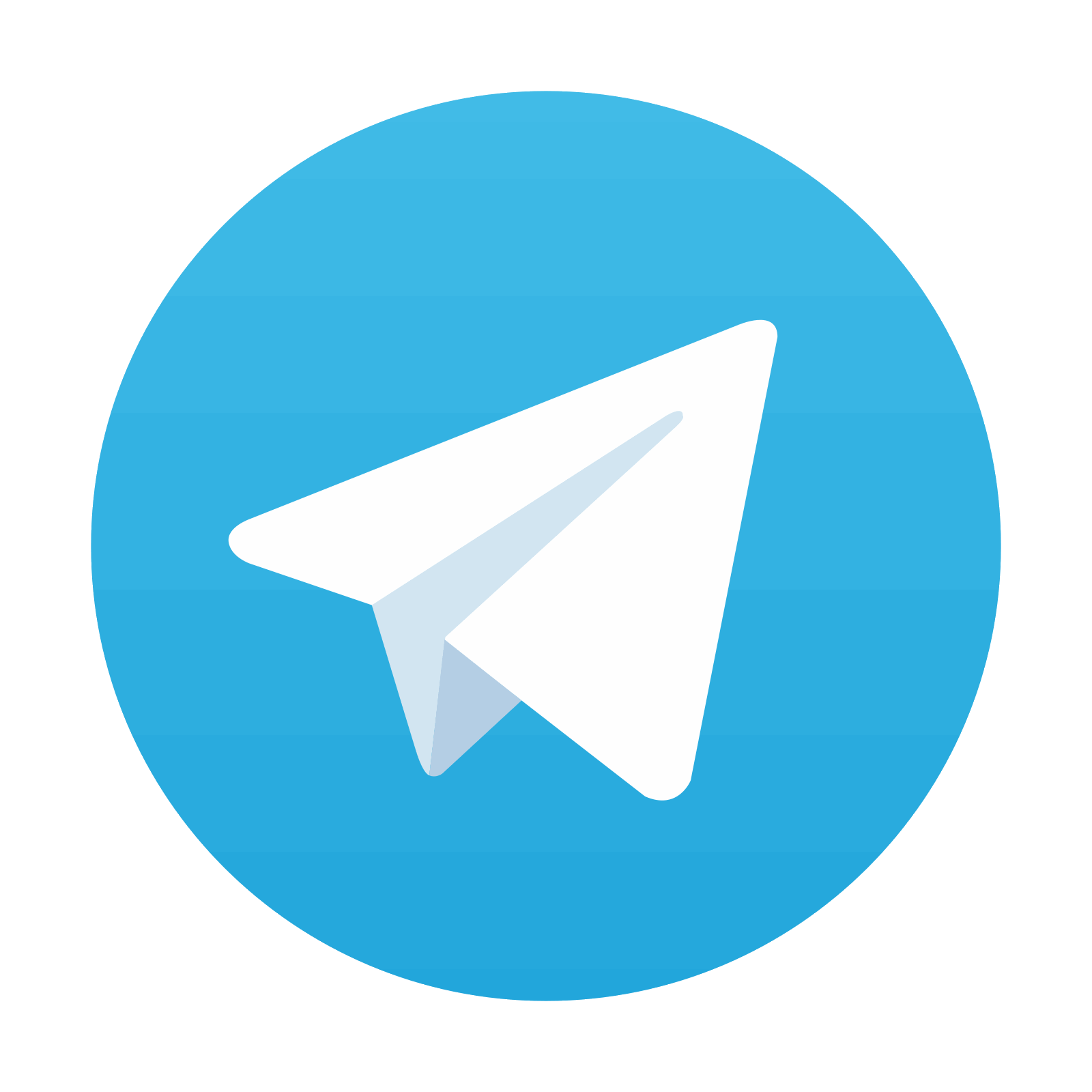
Stay updated, free articles. Join our Telegram channel

Full access? Get Clinical Tree
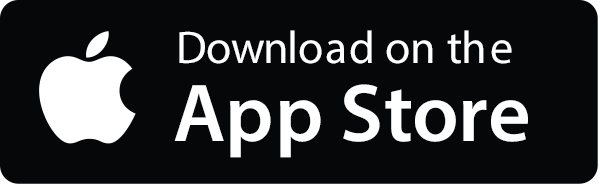
