The Frequentist Approach
The hypothesis tests described in this book are based on the frequentist approach to probability (Chapter 7) and inference that considers the number of times an event would occur if we were to repeat the experiment a large number of times. This approach is sometimes criticized for the following reasons.
- It uses only information obtained from the current study, and does not incorporate into the inferential process any other information we might have about the effect of interest, e.g. a clinician’s views about the relative effectiveness of two therapies before a clinical trial is undertaken.
- It does not directly address the issues of greatest interest. In a drug comparison, we are usually really interested in knowing whether one drug is more effective than the other. However, the frequentist approach tests the hypothesis that the two drugs are equally effective. Although we conclude that one drug is superior to the other if the P-value is small, this probability (i.e. the P-value) describes the chance of getting the observed results if the drugs are equally effective, rather than the chance that one drug is more effective than the other (our real interest).
- It tends to over-emphasize the role of hypothesis testing and whether or not a result is significant, rather than the implications of the results.
The Bayesian Approach
An alternative, Bayesian 1, approach to inference reflects an individual’s personal degree of belief in a hypothesis, possibly based on information already available. Individuals usually differ in their degrees of belief in a hypothesis; in addition, these beliefs may change as new information becomes available. The Bayesian approach calculates the probability that a hypothesis is true (our focus of interest) by updating prior opinions about the hypothesis as new data become available.
Conditional Probability
A particular type of probability, known as conditional probability, is fundamental to Bayesian analyses. This is the probability of an event, given that another event has already occurred. As an illustration, consider an example. The incidence of haemophilia A in the general population is approximately 1 in 10 000 male births. However, if we know that a woman is a carrier for haemophilia, this incidence increases to around 1 in 2 male births. Therefore, the probability that a male child has haemophilia, given that his mother is a carrier, is very different to the unconditional probability that he has haemophilia if his mother’s carrier status is unknown.
Bayes Theorem
Suppose we are investigating a hypothesis (e.g. that a treatment effect equals some value). Bayes theorem converts a prior probability, describing an individual’s belief in the hypothesis before the study is carried out, into a posterior probability, describing his/her belief afterwards. The posterior probability is, in fact, the conditional probability of the hypothesis, given the results from the study. Bayes theorem states that the posterior probability is proportional to the prior probability multiplied by a value, the likelihood of the observed results which describes the plausibility of the observed results if the hypothesis is true (Chapter 32).
Diagnostic Tests in a Bayesian Framework
Almost all clinicians intuitively use a Bayesian approach in their reasoning when making a diagnosis. They build a picture of the patient based on clinical history and/or the presence of symptoms and signs. From this, they decide on the most likely diagnosis, having eliminated other diagnoses on the presumption that they are unlikely to be true, given what they know about the patient. They may subsequently confirm or amend this diagnosis in the light of new evidence, e.g. if the patient responds to treatment or a new symptom develops.
When an individual attends a clinic, the clinician usually has some idea of the probability that the individual has the disease – the prior or pre-test probability. If nothing else is known about the patient, this is simply the prevalence (Chapters 12 and 38) of the disease in the population. We can use Bayes theorem to change the prior probability into a posterior probability. This is most easily achieved if we incorporate the likelihood ratio (Chapter 32), based on information obtained from the most recent investigation (e.g. a diagnostic test result), into Bayes theorem. The likelihood ratio of a positive test result is the chance of a positive test result if the patient has disease, divided by that if he or she is disease-free. We discussed the likelihood ratio in this context in Chapter 38 and showed that it could be used to indicate the usefulness of a diagnostic test. We now use it to express Bayes theorem in terms of odds (Chapter 16):
where
The posterior odds is simple to calculate but, for easier interpretation, we convert the odds back into a probability using the relationship
This posterior or post-test probability is the probability that the patient has the disease, given a positive test result. It is similar to the positive predictive value (PPV, Chapter 38) but the clinician will not be able to determine the PPV unless he or she has access to the test results from a sample of patients, each of whom has a definitive diagnosis from a gold standard test (see Table 38.1). Furthermore, the main factor that affects the PPV is the prevalence, and there may be reasons why an individual’s underlying risk of disease is known to be higher or lower than the overall population prevalence. Thus, in this situation, even if the clinician could calculate the PPV, it may not give a reasonable indication of his or her belief, after the test result is known, that the patient has the disease. Therefore, it is preferable to calculate the post-test probability in this situation.
A simpler way to calculate the post-test probability is to use Fagan’s nomogram (see Fig. 45.1); by connecting the pre-test probability (expressed as a percentage) to the likelihood ratio and extending the line, we can evaluate the post-test probability.
Figure 45.1 Fagan’s nomogram for interpreting a diagnostic test result.
Adapted from Sackett, D.L., Richardson, W.S., Rosenberg, W. and Haynes, R.B. (1997) Evidence-based Medicine: How to Practice and Teach EBM. Churchill-Livingstone, London, with permission.

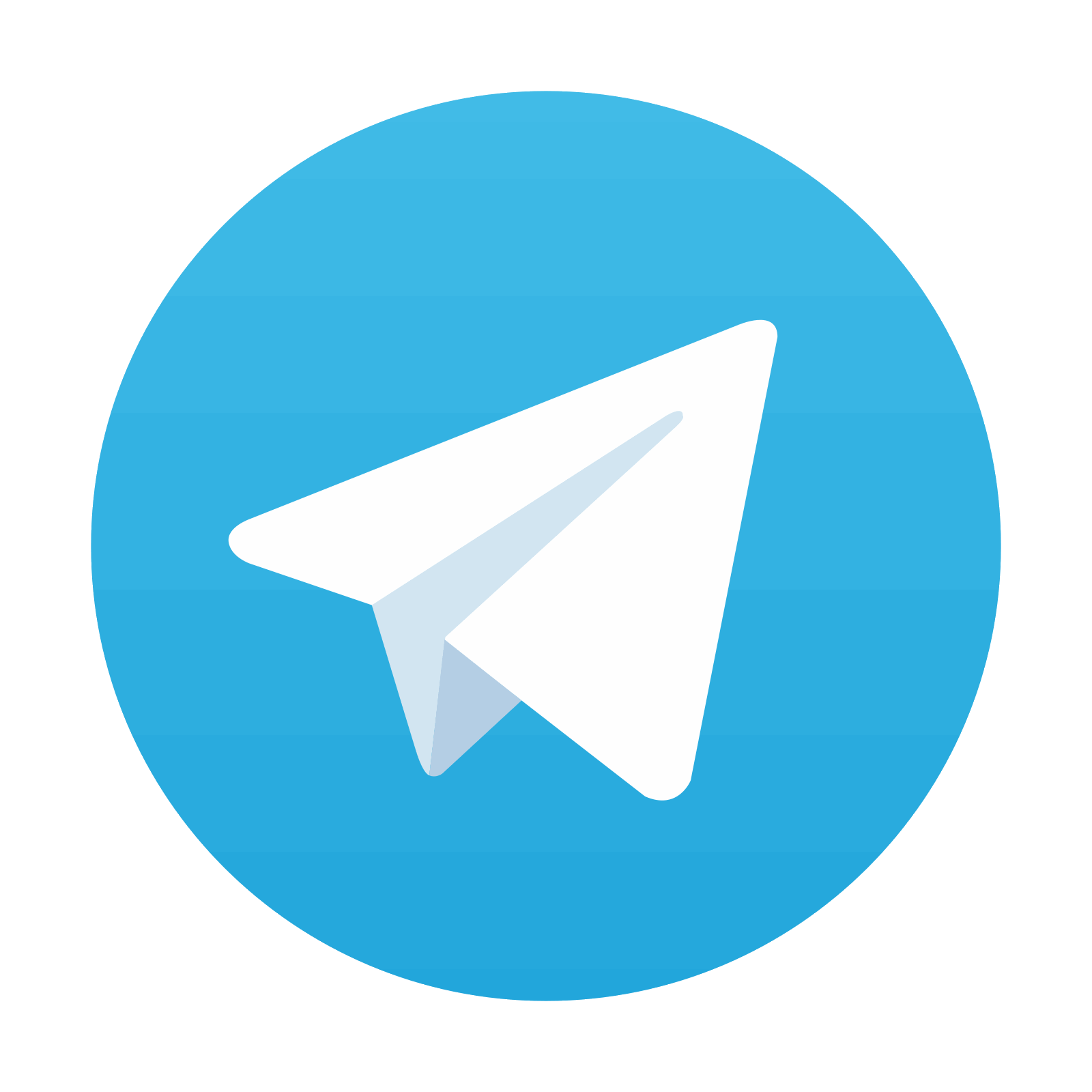
Stay updated, free articles. Join our Telegram channel

Full access? Get Clinical Tree
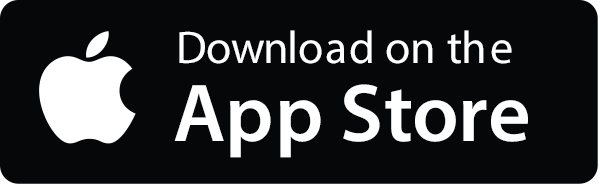
