CHAPTER 21 Assessment of Tests
SENSITIVITY AND SPECIFICITY
The formula for sensitivity is:
The formula for specificity is:
SnNOut: high Sensitivity, Negative test rules it Out.
SpPIn: high Specificity, Positive test rules it In.
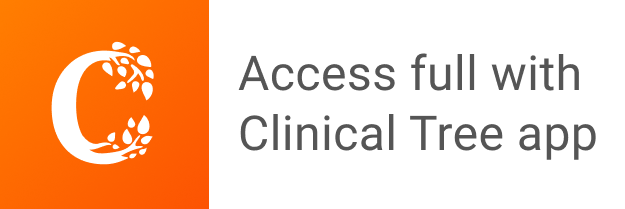
CHAPTER 21 Assessment of Tests
The formula for sensitivity is:
The formula for specificity is:
SnNOut: high Sensitivity, Negative test rules it Out.
SpPIn: high Specificity, Positive test rules it In.