Measurement Variability and Error
A biological variable measured on each of a number of individuals will always exhibit a certain amount of variability. The measurements are likely to vary between individuals (inter-individual variation) as well as within the same individual (intra-individual variation) if the measurement on that individual is repeated, either immediately or some time later. Much of this variability arises because of differences in associated factors, e.g. genetic, environmental or lifestyle factors. For example, blood pressure measurements may vary between individuals if these individuals differ in terms of their sex, age, weight or smoking status and within an individual at different times of the day. We refer to this type of variability as measurement variability. We define measurement error as that which arises when there is a difference between the observed (or ‘measured’) values and true values of a variable (note that although we refer to the ‘true’ measurement here, it is rarely possible to obtain this value). Measurement error may be:
- Systematic – the observed values tend to be too high (or too low) because of some known or unknown extraneous factor affecting the measurements in the same way (e.g. an observer overestimating the values). Systematic errors lead to biased estimates, raising concerns about validity, and should be reduced as far as possible by, for example, standardizing conditions, training observers and/or calibrating the instrument (i.e. verification by comparison with a known standard).
- Random – the observed values are sometimes greater and sometimes less than the true values but they tend to balance out on average. For example, random errors may occur because of a lack of sensitivity of the measuring instrument. Random error is governed by chance although the degree of error may be affected by external factors (e.g. the pH in fresh blood samples may exhibit greater random error when these samples are at room temperature rather than on ice).
Both measurement variability and error are important when assessing a measurement technique. Although the description of error in this section has focused on laboratory measurements, the same concepts apply even if we are interested in other forms of measurement, such as an individual’s state of health on a particular day, as assessed by a questionnaire.
Reliability
There are many occasions on which we wish to compare results which should concur. In particular, we may want to assess and, if possible, quantify the following two types of agreement or reliability:
- Reproducibility (method/observer agreement). Do two techniques used to measure a particular variable, in otherwise identical circumstances, produce the same result? Do two or more observers using the same method of measurement obtain the same results?
- Repeatability. Does a single observer obtain the same results when she or he takes repeated measurements in identical circumstances?
Reproducibility and repeatability can be approached in the same way. In each case, the method of analysis depends on whether the variable is categorical (e.g. poor/average/good) or numerical (e.g. systolic blood pressure). For simplicity, we shall restrict the problem to that of comparing only paired results (e.g. two methods/two observers/duplicate measurements).
Categorical Variables
Suppose two observers assess the same patients for disease severity using a categorical scale of measurement, and we wish to evaluate the extent to which they agree. We present the results in a two-way contingency table of frequencies with the rows and columns indicating the categories of response for each observer. Table 39.1 is an example showing the results of two observers’ assessments of the condition of tooth surfaces. The frequencies with which the observers agree are shown along the diagonal of the table. We calculate the corresponding frequencies which would be expected if the categorizations were made at random, in the same way as we calculated expected frequencies in the Chi-squared test of association (Chapter 24), i.e. each expected frequency is the product of the relevant row and column totals divided by the overall total. Then we measure agreement by
which represents the chance corrected proportional agreement, where:
- m = total observed frequency (e.g. total number of patients)
- Od = sum of observed frequencies along the diagonal
- Ed = sum of expected frequencies along the diagonal
- 1 in the denominator represents maximum agreement.
κ = 1 implies perfect agreement and κ = 0 suggests that the agreement is no better than that which would be obtained by chance. There are no objective criteria for judging intermediate values. However, kappa is often judged as providing agreement1 which is:
- poor if κ < 0.00
- slight if 0.00 ≤ κ ≤ 0.20
- fair if 0.21 ≤ κ ≤ 0.40
- moderate if 0.41 ≤ κ ≤ 0.60
- substantial if 0.61 ≤ κ ≤ 0.80
- almost perfect if κ > 0.80.
Although it is possible to estimate a standard error and confidence interval2 for kappa, we do not usually test the hypothesis that kappa is zero since this is not really pertinent or realistic in a reliability study.
Note that kappa is dependent both on the number of categories (i.e. its value is greater if there are fewer categories) and the prevalence of the condition, so care must be taken when comparing kappas from different studies. For ordinal data, we can also calculate a weighted kappa3 which takes into account the extent to which the observers disagree (the non-diagonal frequencies) as well as the frequencies of agreement (along the diagonal). The weighted kappa is very similar to the intraclass correlation coefficient (see next section and Chapter 42).
Numerical Variables
Suppose an observer takes duplicate measurements of a numerical variable on n individuals (just replace the word ‘repeatability’ by ‘reproducibility’ if considering the similar problem of method agreement, but remember to assess the repeatability of each method before carrying out the method agreement study).
Is There a Systematic Effect?
If we calculate the difference between each pair of measurements and find that the average difference is zero (this is usually assessed by the paired t-test but we might use the sign test or signed ranks test (Chapters 19 and 20)), then we can infer that there is no systematic difference between the pairs of results, i.e on average, the duplicate readings agree. If one set of readings represents the true values, as is likely in a method comparison study, this means that there is no bias.
Measures of Repeatability and the Bland and Altman Diagram
The estimated standard deviation of the differences (sd) provides a measure of agreement for an individual. However, it is more usual to calculate the British Standards Institution repeatability coefficient = 2sd. This is the maximum difference which is likely to occur between two measurements. Assuming a Normal distribution of differences, we expect approximately 95% of the differences in the population to lie between where
is the mean of the observed differences. The upper and lower limits of this interval are called the limits of agreement; from them, we can decide (subjectively) whether the agreement between pairs of readings in a given situation is acceptable. The limits are usually indicated on a Bland and Altman diagram which is obtained by calculating the mean of and the difference between each pair of readings, and plotting the n differences against their corresponding means4 (Fig. 39.1). The diagram can also be used to detect outliers (Chapter 3).
It makes no sense to calculate a single measure of repeatability if the extent to which the observations in a pair disagree depends on the magnitude of the measurement. We can check this using the Bland and Altman diagram (Fig 39.1). If we observe a random scatter of points (evenly distributed above and below zero if there is no systematic difference between the pairs), then a single measure of repeatability is acceptable. If, however, we observe a funnel effect, with the variation in the differences being greater (say) for larger mean values, then we must reassess the problem. We may be able to find an appropriate transformation of the raw data (Chapter 9) so that, when we repeat the process on the transformed observations, the required condition is satisfied.
Indices of Reliability
Intraclass Correlation Coefficient
An index of reliability commonly used to measure repeatability and reproducibility is the intraclass correlation coefficient (ICC, Chapter 42), which takes a value from zero (no agreement) to 1 (perfect agreement). When measuring the agreement between pairs of observations, the ICC is the proportion of the variability in the observations which is due to the differences between pairs, i.e. it is the between-pair variance expressed as a proportion of the total variance of the observations.
When there is no evidence of a systematic difference between the pairs, we may calculate the ICC as the Pearson correlation coefficient (Chapter 26) between the 2n pairs of observations obtained by including each pair twice, once when its values are as observed and once when they are interchanged (see Example 2).
If we wish to take the systematic difference between the observations in a pair into account, we estimate the ICC as
where we determine the difference between and the sum of the observations in each of the n pairs and:
is the estimated variance of the n sums
is the estimated variance of the n differences
is the estimated mean of the differences (an estimate of the systematic difference).
We usually carry out a reliability study as part of a larger investigative study. The sample used for the reliability study should be a reflection of that used for the investigative study. We should not compare values of the ICC in different data sets as the ICC is influenced by features of the data, such as its variability (the ICC will be greater if the observations are more variable). Note that the ICC is not related to the actual scale of measurement nor to the size of error which is clinically acceptable.
Lin’s Concordance Correlation Coefficient
It is inappropriate to calculate the Pearson correlation coefficient (Chapter 26) between the n pairs of readings (e.g. from the first and second occasions or from two methods/observers) as a measure of reliability. We are not really interested in whether the points in the scatter diagram (e.g. of the results from the first occasion plotted against those from the second occasion) lie on a straight line; we want to know whether they conform to the line of equality (i.e. the 45 ° line through the origin when the two scales are the same). This will not be established by testing the null hypothesis that the true Pearson correlation coefficient is zero. It would, in any case, be very surprising if the pairs of measurements were not related, given the nature of the investigation. Instead, we may calculate Lin’s concordance correlation coefficient5 as an index of reliability which is almost identical to the ICC. Lin’s coefficient modifies the Pearson correlation coefficient which assesses the closeness of the data about the line of best fit (Chapters 28 and 29) in the scatter plot by taking into account how far the line of best fit is from the 45 ° line through the origin. The maximum value of Lin’s coefficient is one, achieved when there is perfect concordance, with all the points lying on the 45 ° line drawn through the origin. The coefficient can be calculated as
where r is the estimated Pearson correlation coefficient (Chapter 26) between the n pairs of results (xi, yi), and and
are the sample means of x and y, respectively.
More Complex Situations
Sometimes you may come across more complex problems when assessing agreement. For example, there may be more than two replicates, or more than two observers, or each of a number of observers may have replicate observations. You can find details of the analysis of such problems in Streiner and Norman6.
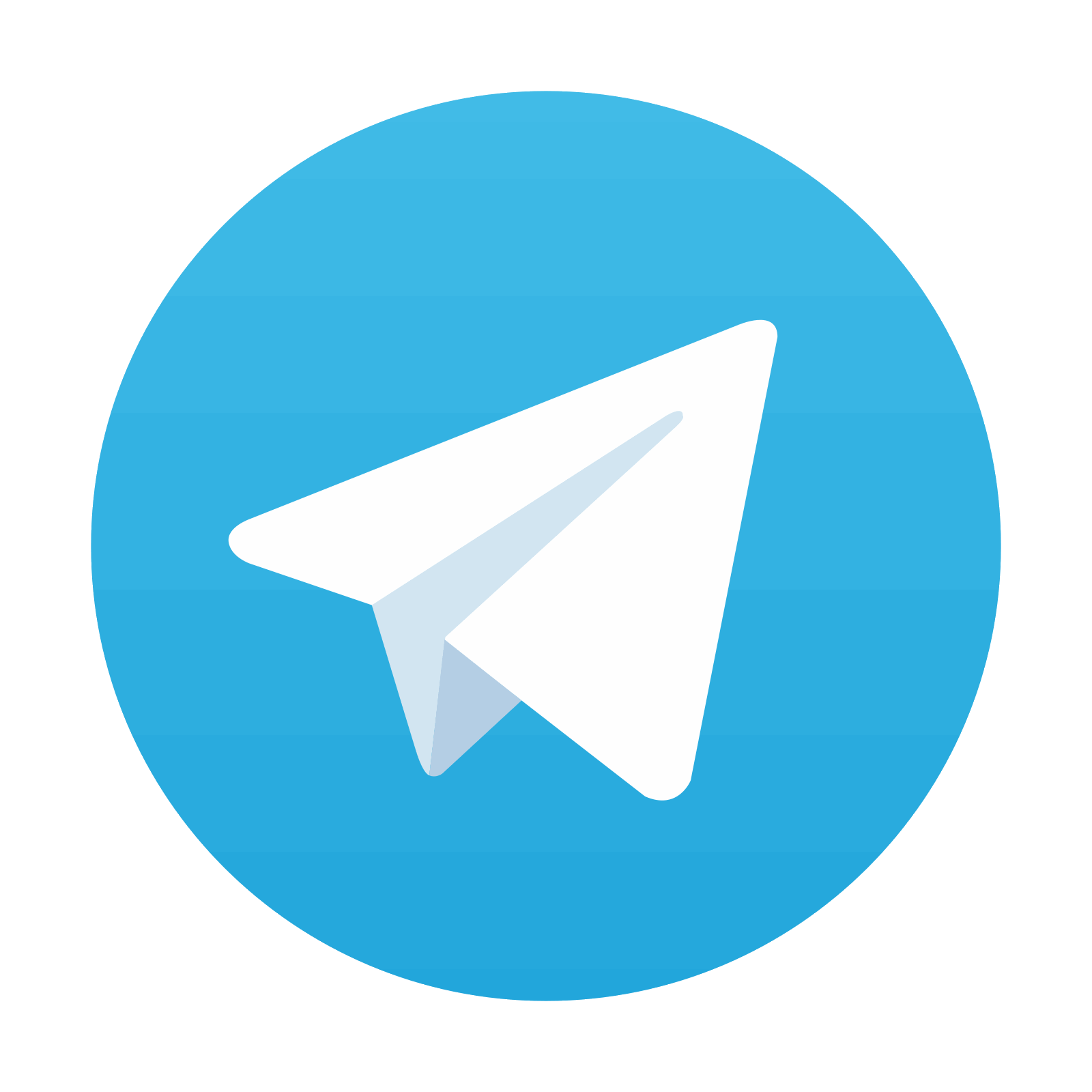
Stay updated, free articles. Join our Telegram channel

Full access? Get Clinical Tree
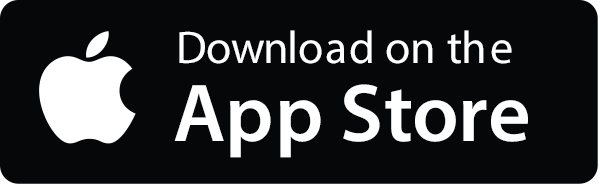
