While it is important to be able to read and interpret individual papers, as we have noted previously the results of a single study are never going to provide the complete answer to a question. To move towards this we need to review the literature more widely. There can be a number of reasons for doing this, some of which require a more comprehensive approach than others. If the aim is simply to increase our personal understanding of a new area then a few papers might provide adequate background material. Traditional narrative reviews, which give less emphasis to complete coverage of the literature and tend to be more qualitative, have value for exploring areas of uncertainty or novelty, but it is harder to scrutinise them for flaws. In contrast, a major decision regarding policy or practice should be based on a systematic review and perhaps a meta-analysis of all the relevant literature and it is this systematic approach that we will focus on here.
What is a systematic review?
A systematic review should be a helpful synthesis of all of the relevant data – highlighting patterns but not hiding differences. Although its primary data units are whole studies rather than individuals, it should still have a clearly formulated research question and be conducted with the same rigour as its component studies. So how should we go about conducting a systematic review? This is a major undertaking and excellent guidelines are widely available for would-be reviewers (see e.g. the Cochrane Collaboration website www.cochrane.org) so we will not attempt to cover all of the issues here. But, in brief, it involves:
identifying all potentially relevant primary research studies that address the question of interest and including or excluding them according to predetermined criteria;
abstracting the data in a standard format and critically appraising the included studies;
summarising the findings of the studies, this might include a formal meta-analysis to combine the results of all of the studies into a single summary estimate; and
an overall evaluation of the evidence with appropriate conclusions.
It follows from this that such a review should be structured in the same way as a primary paper: an introduction to show why the research question is of interest; a methods section to explain how studies were identified, included/excluded and appraised, and how the data were abstracted; the results where patterns are highlighted and differences assessed; and, finally, a discussion where the results are interpreted, threats to validity considered and causal conclusions drawn. Box 11.1 shows a condensed excerpt from the methods section of a Cochrane systematic review of the use of antibiotics for treating acute laryngitis, giving a sense of the detailed approach required. In the next few pages we will discuss the various stages of the review process as a guide to both reading and writing a systematic review.
Objectives
To assess the effectiveness and safety of different antibiotic therapies in adults with acute laryngitis.
Search strategy
We searched the Cochrane Central Register of Controlled Trials (CENTRAL 2014, Issue 11), MEDLINE (January 1966 to November week 3, 2014), EMBASE (1974 to December 2014), LILACS (1982 to December 2014) and BIOSIS (1980 to December 2014). The CENTRAL and MEDLINE search strategies are provided. We imposed no language or publication restrictions.
We employed other strategies including the searching of references of review articles and books related to infections of the respiratory tract, and handsearches of journals, etc. We searched grey literature such as conference abstracts/proceedings, published lists of theses and dissertations, etc., and other literature outside of the main journal literature, where possible.
Trial selection
Randomised controlled trials (RCTs) comparing any antibiotic therapy with placebo or another antibiotic in the treatment of acute laryngitis. The primary outcome was an improvement in recorded voice score assessed by an expert panel.
Data extraction
Two review authors (names provided) independently:
retrieved the articles and assessed their eligibility from the title and abstracts,
assessed the full text of all studies identified as possibly relevant,
assessed the risk of bias (see Box 9.2) for each study and resolved disagreements by discussion.
Data synthesis
We used Review Manager 5.3 to create ‘Summary of findings’ tables. We produced a summary of the intervention effect and a measure of quality for each of the above outcomes using the GRADE approach (see ‘Drawing conclusions’, below).
Identifying the literature
The first challenge when conducting a systematic review is to identify all of the relevant literature. The potential sources of data are numerous. MEDLINE is probably the most commonly used source for epidemiological papers. At June 2016 it contained more than 23 million references to articles published since 1946 in 5,600 life sciences journals worldwide. It is freely accessible through the US National Library of Medicine search engine PubMed® at www.ncbi.nlm.nih.gov/pubmed/. EMBASE® is not so widely available but has some advantages over MEDLINE in that it includes many additional journals as well as conference abstracts. Another valuable database, particularly for systematic reviews of trials of the effects of healthcare interventions, is that of the Cochrane Collaboration (CENTRAL, www.cochrane.org). There are also many other electronic databases that may be valuable sources of literature depending on the question you are researching (e.g. PsycINFO® has an obvious specialised focus).
No electronic literature search is ever likely to be complete, so it is important to use multiple strategies. Once several relevant articles have been identified, it can help to check the papers that they cite, and also to look in the other direction, i.e. for papers that have cited them (e.g. via PubMed which lists other articles in PubMed that have cited the selected paper). Other sources include personal communication with experts in the field who may know of additional published articles (and unpublished material); theses, seminars, internal reports and non-peer-reviewed journals (sometimes described as the ‘grey literature’); and other electronic information including topic-specific Internet databases.
Publication and related biases
When searching the literature it is important to bear in mind that studies with positive and/or statistically significant findings may be more likely to be published than those without significant results. This publication bias is related not only to selective acceptance by journals, but also to selective submission to journals by researchers who may decide not to submit reports from research that either finds no association at all (i.e. a null finding) or in which the results are not statistically significant.
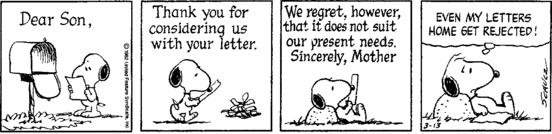
PEANUTS © 1982 Peanuts Worldwide LLC. Dist. By UNIVERSAL UCLICK.
It is possible to check the existence of publication bias by drawing what is called a funnel plot. In the absence of bias, this should be a roughly symmetrical inverted funnel shape.
Another problem is the preferential detection of articles in English. For an English speaker there are several barriers to the inclusion of non-English studies in a review, including the difficulties associated with translation and the fact that non-English articles may be published in local journals that are not indexed by major bibliographic databases. This is more likely to be the case for less exciting findings. It is also important to be aware that there may be multiple publications from one study and, if these are included as separate studies in the review, this could bias the conclusions.
Study inclusion and exclusion
Studies should be selected for inclusion in the review on the basis of predefined criteria. Depending on the research question, it might be appropriate to restrict the review to specific research designs, for example only randomised trials, or to those with specific methodological features. Such features might include:
the study size (e.g. only those studies with more than a certain number of cases),
the participants (e.g. a particular age range or sex),
a specific outcome or the way in which the outcome was measured (e.g. histological or serological confirmation),
the way in which the exposure was measured or classified (e.g. a particular type of blood test to measure an infection, more than two levels of alcohol intake) and
the duration of follow-up (e.g. more than 12 months).
Appraising the literature
The amount and types of literature generated by a search will vary enormously depending on the subject area. For a review of a specific treatment the studies may all be clinical trials, whereas an aetiological review is likely to include observational studies of all types from case reports to cohort studies, with few or no trials. As you have seen, different types of study answer different types of questions, or may be subject to different biases when answering the same question, so it is sensible to group them separately, at least to start with. This grouping may then provide a logical framework to help organise the data within the review.
Key information including aspects of the study design and conduct, the potential for error and the relevant results should be abstracted onto purpose-designed forms and the validity of the studies evaluated as outlined in Chapter 9. In an ideal world the appraisers should be blinded to the authors and the study results because this knowledge has been shown to influence judgements about validity. In practice this is not always possible, because the reviewers may already be too familiar with the literature. Rigorous systematic reviews like that described in Box 11.1 will often specify the need for multiple assessors to reduce the potential for bias.
A common approach to grading the quality of individual studies has been to classify study designs according to a hierarchy such that those at the top are considered to provide stronger evidence of an effect than those further down the scale (Table 11.1). You will notice that this ranking puts randomised trials at the top of the pile. While a classification of this type may be appropriate in the clinical context where RCTs are the norm, it is often not much help for an aetiological review. In 2003, the British Medical Journal published an entertaining systematic review of randomised controlled trials of ‘parachute use to prevent death and major trauma related to gravitational challenge’ (Smith and Pell, 2003). Not surprisingly, the authors failed to find any randomised trials for this particular preventive intervention. Despite the tongue-in-cheek nature of the report, the fact remains that not all interventions can be evaluated in RCTs and a lack of RCT evidence does not mean a lack of useful evidence for public health action. Even more important is the disregard for the quality of individual studies inherent in this approach. A well-designed and properly conducted cohort or case–control study could provide better evidence than a small or poorly conducted trial, but this rigid hierarchy would rate the evidence from the trial more highly. Box 11.2 considers the value of randomised and non-randomised designs in healthcare evaluation in more detail.
Level | Evidence |
---|---|
I | Evidence from at least one properly randomised controlled trial |
II-1 | Evidence from well-designed controlled trials without randomisation |
II-2 | Evidence from well-designed cohort or case–control studies, preferably from more than one centre or research group |
II-3 | Evidence from comparisons over time or between places with or without the intervention; dramatic results in uncontrolled experiments could also be regarded as this level of evidence |
III | Opinions of respected authorities, based on clinical experience, descriptive studies and case reports, or reports of expert committees |
Most generic lists rank RCTs first in terms of study quality. For appropriate questions, i.e. about the effects of various interventions, this is reasonable, as you have seen. However, even for such questions caveats need to be applied. If a randomised trial is not competently conducted, or is too small, then its theoretical advantages disappear, and it can give misleading results (Schultz et al., 1995). There are also many situations in which a trial would be unfeasible, unethical, undesirable or unnecessary (Black, 1996), and trials are generally irrelevant for questions related to frequency or measurement validation (e.g. of the performance of a screening or diagnostic test).
Estimates of the effects of treatment may differ between randomised and non-randomised studies, but when direct comparisons have been made neither method has consistently given a greater effect than the other (McKee et al., 1999). Overall, it seems that dissimilarities between the participants in RCTs and non-randomised studies explain many of the differences; the two methods should therefore be compared only after patients not meeting the RCT eligibility criteria have also been excluded from the non-randomised study. Not surprisingly then, treatment effects measured in randomised and non-randomised studies are most similar when the exclusion criteria are the same and where potential prognostic factors are well understood and controlled for in the non-randomised setting. Taking this approach has helped reconcile some of the apparently major differences between the effects of menopausal hormone therapy (MHT) as found in RCTs (evidence of harm) and cohort studies (evidence of health benefits). Closer consideration of the details of the different studies suggests that differences in the ages at which women started taking MHT – around menopause (average age 51 years in the USA) in the cohort studies but at a mean age of more than 60 years in the trials – could explain many of the differences (Manson and Bassuk, 2007). It is also important to consider the precision of the RCT effect estimates – some are based on so few events (especially when death is the outcome of interest) that chance differences from the true underlying effect are quite likely. (For an example of these issues from studies comparing the effectiveness of various interventions to unblock coronary arteries see Britton et al., 1998.)
The generalisability (see Chapters 7 and 9) of the results of RCTs can also be questionable, given the highly selected nature of the participants: patients excluded from randomised controlled trials tend to have a worse prognosis than those included (McKee et al., 1999). When both randomised and non-randomised studies have been conducted and estimates of treatment effect are reasonably consistent for patients at similar risk, it allows more certain generalisation to the broader target populations of the non-randomised studies.
The need to move away from such a rigid approach has been well documented both for clinical research (Glasziou et al., 2004) and for health services research in general (Black, 1996). A preferable approach, adopted by decision-making bodies around the world such as the US Preventive Services Task Force (USPSTF) and the Canadian Task Force on Preventive Health Care (CTFPHC), is not to classify studies purely on the basis of their design but also according to the quality of the evidence they provide. As its name suggests, the USPSTF regularly reviews the evidence for and against a wide range of preventive interventions. They rate studies according to specific criteria for that design (based on the key questions of subject selection and measurement that we discussed in Chapters 4 and 7). A ‘good’ study would generally meet all of the specified criteria, a ‘fair’ study does not meet them all but is judged not to have a fatal flaw that would invalidate the results, and a fatally flawed study is classified as ‘poor’ (Harris et al., 2001). Another tool for assessing the quality of a study is the Cochrane ‘Risk of bias’ tool that we mentioned in Chapter 9 (Box 9.2) and was used by the authors of the review in Box 11.1.
Summarising the data
The next step in any review is to draw the data together to simplify their interpretation and to assist in drawing valid conclusions. It is important to look both for consistency of effects across studies (homogeneity) and for differences among studies (heterogeneity). Could differences be due to chance variation, or can they be explained by features of the studies or the populations they were conducted in? Graphs can be used to summarise the results of many studies in a simple format and in some situations the technique of meta-analysis can be used to combine the results from a number of different studies.
Graphical display of results
One way to display the results of a number of different studies is in a figure called a forest plot. Figure 11.1 shows a forest plot from a systematic review of the relation between weight/BMI and ovarian cancer risk (Purdie et al., 2001). It shows the results of all 23 case–control studies whose authors had reported data on this association, ordered with the most recent study at the top and the oldest at the bottom. The odds ratio for each individual study is represented by the black square, with the size of the square indicating the size (or ‘weight’ – see ‘Meta-analysis’, below) of that particular study. The horizontal bar through each box shows the 95% confidence interval for the odds ratio and the vertical line indicates the point where there is ‘no effect’, i.e. an odds ratio of 1.0. When the confidence interval crosses this line (i.e. it includes the null value) it indicates that the result is not statistically significant (i.e. p ≥ 0.05). (Note the use of the logarithmic scale, which balances positive and inverse relative effects visually around the null; i.e. an OR of 2.0 would be the same distance from 1.0 as an OR of 0.5 in the opposite direction.)
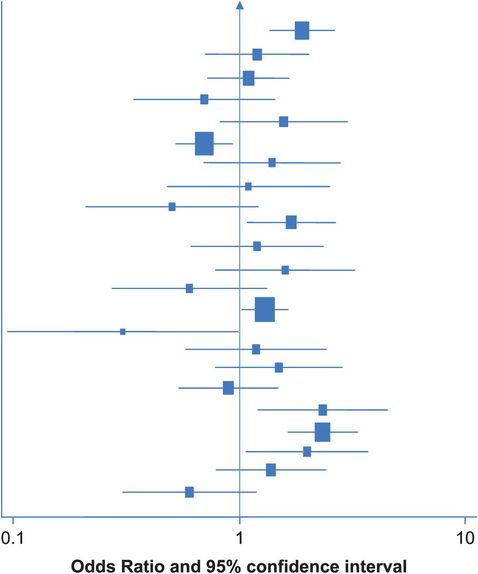
Diagrammatic representation of the results of 23 case–control studies evaluating the relation between extremes of weight/BMI and risk of ovarian cancer (Purdie et al., 2001).
Assessing heterogeneity
In this example the results of the 23 studies are scattered both sides of the line that marks an odds ratio of 1.0 (i.e. no effect), and they show no obvious pattern. The next step should be to evaluate this heterogeneity in more detail. Are there any differences between the studies that could explain some of the variation in their results?
There are formal statistical tests to check for heterogeneity between the results of different studies. For example Cochran’s Q-test and I2 statistics.
One major methodological difference between the studies in this example is subject selection: some were population-based and others were hospital-based. We touched on some of the problems inherent in hospital-based studies in earlier chapters – could this difference explain any of the variation in the study results? Other possibilities to consider might include the geographical areas where the research was done – for example, separating high- and low-risk countries, and the ages of the participants. In this case, if we separate the hospital- and population-based studies (Figure 11.2) we start to see some regularity. In each of the 11 population-based studies at the bottom of Figure 11.2, the OR is greater than 1.0 (although many of the individual results are not statistically significant), suggesting that obesity/higher weight is associated with an increased risk of ovarian cancer. In contrast, the results of the hospital studies still vary widely. In this situation it was felt that using hospital-based controls might not be appropriate, as obese people are more likely to have other health problems and thus end up in hospital so their use could lead to overestimation of the prevalence of obesity in the population and thereby to underestimation of the obesity–cancer association.
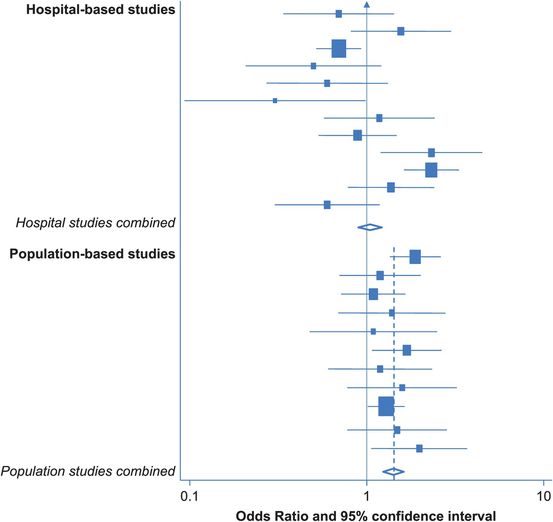
Diagrammatic representation of the results of 12 hospital-based and 11 population-based case–control studies evaluating the relation between extremes of weight/BMI and risk of ovarian cancer (Purdie et al., 2001).
Meta-analysis
Meta-analysis is a powerful technique that allows the results of a number of different studies to be combined. Each study is assigned a weight based on the amount of information it provides (e.g. the inverse of the standard error of the OR) and in general larger studies have greater weight. A weighted average of the individual study results can then be calculated. The assumption underlying this analysis is that all of the studies are estimating the same underlying effect and any variation between their results is due to chance. If their results are very different (i.e. they are heterogeneous), as in the hospital-based studies of BMI above, then this assumption may not be true and it might not be appropriate to combine the results.
The diamond at the bottom of Figure 11.2 represents the combined odds ratio for the 11 population-based studies; the centre indicates the point estimate and the ends show the 95% confidence interval. In this case, it indicates that being overweight increases the risk of ovarian cancer by 40% (pooled OR = 1.4; 95% CI 1.2–1.6). Notice that the diamond does not overlap the ‘no-effect’ line (i.e. the confidence interval does not include 1.0), so the pooled OR is statistically significant. If we draw a dotted line vertically through the combined odds ratio, it passes through the 95% confidence interval of each of the individual studies. This is an indication that the results of the studies are fairly homogeneous, but it is certainly not definitive. In this case a formal statistical test for heterogeneity gives a p-value of 0.63. If p were < 0.05, this would suggest that differences between the results of the individual studies were unlikely to be due to chance; however, the observed p-value is well away from this, suggesting there is no significant heterogeneity and thus supporting the ‘eyeball’ finding that the results are all fairly similar.
In contrast, if we combine the results of the 12 hospital-based studies, we find a combined OR of 0.9 (95% CI 0.9–1.2), but a line through this point would not pass through the confidence intervals of the individual studies. This suggests that the results of the hospital-based studies are heterogeneous, and this is confirmed by a statistical test for heterogeneity, which gives p < 0.001, which is highly statistically significant. In this situation it is inappropriate to combine the results into a single estimate of effect.
Pooled analysis
An even more rigorous but much more time-consuming approach is known as a pooled analysis or re-analysis. Instead of combining the summary results (OR or RR) from a number of different studies, the investigator obtains copies of the raw data from the original studies and re-analyses them in a consistent way. An excellent example is the Oxford-based Collaborative Group on Hormonal Factors in Breast Cancer which, since the mid-1990s, has been producing reports based on analyses of over 50,000 women with breast cancer and 100,000 without, from data provided by more than 50 separate studies. The collaboration’s first paper showed with great precision the very low absolute risk of breast cancer conferred by the majority of patterns of oral contraceptive pill use (Collaborative Group on Hormonal Factors in Breast Cancer, 1996). This report removed a great deal of the uncertainty that remained about this relation, despite many prior publications from individual studies.
Until recently, such pooled analyses were relatively uncommon: the effort required to obtain the original data, clean, recode and re-shape each data set to a common standard, conduct a new analysis and write the paper, all the while maintaining full approval of all contributing investigators, is monumental. However, the last few years have seen an explosion in the number of international consortia (and acronyms!) established specifically to bring together investigators from around the world to pool genetic and/or epidemiological data from different studies. This has been particularly true in the field of molecular epidemiology, where it seems likely that aside from a small number of ‘high-risk’ genes, such as the BRCA1 and BRCA2 genes identified for breast and ovarian cancer, the effects of any individual genetic variant on cancer risk are likely to be small and, as a result, very large numbers of individuals are needed to show an association with any certainty. Examples of these consortia include BCAC (Breast Cancer Association Consortium), OCAC (Ovarian Cancer Association Consortium), PANC4 (Pancreatic Cancer Case Control Consortium), E2C2 (Epidemiology of Endometrial Cancer Consortium), BEACON (Barrett’s and Esophageal Adenocarcinoma Consortium), DIAGRAM (DIAbetes Genetics Replication And Meta-analysis) Consortium … the list is ever-growing.
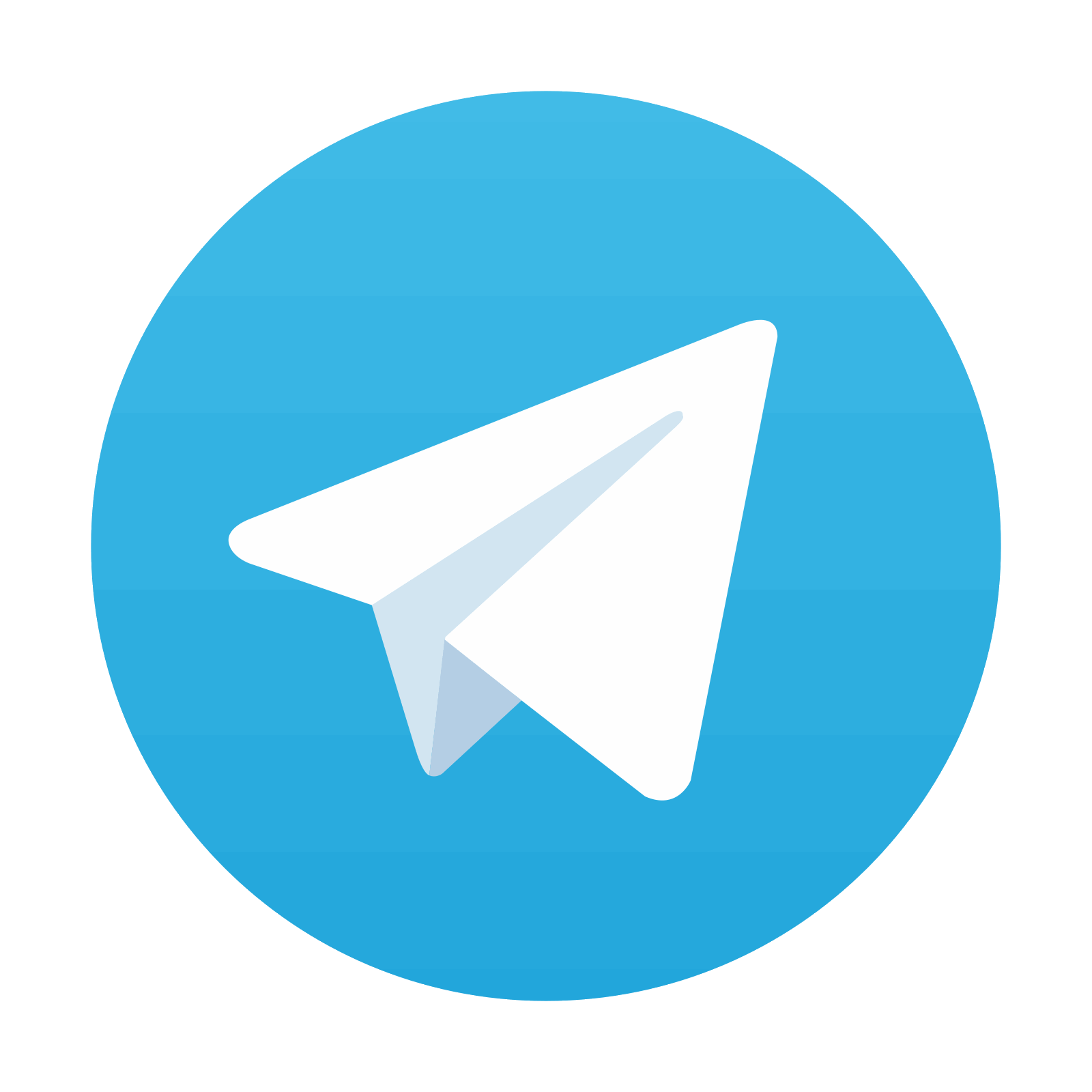
Stay updated, free articles. Join our Telegram channel

Full access? Get Clinical Tree
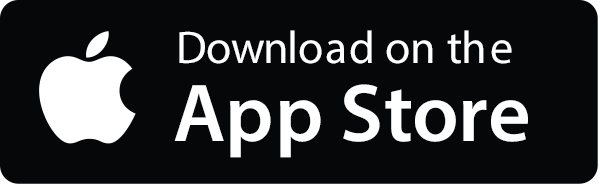
