Fig. 1
Schematic illustration of the operational architectonics methodology including steps for EEG segmentation, extraction of segments’ features, and synchronization of segments. Further explanations are provided in the text. RTP—rapid transitional processes (boundaries between quasi-stationary EEG segments); SC—momentary synchro-complexes (synchronization of RTPs between several but always the same local EEGs at the particular time instants)
The choice of window durations and of the “false alerts” rule was made during a few preliminary modelling studies (for a review see [56]) to guarantee the following two goals: (a) to detect as many real RTPs as possible and (b) to keep the level of “contamination” by “false” RTPs in the results reasonably low.
The identified quasi-stationary segments of EEG activity could be characterized by several attributes that reflect different characteristics of transient neuronal assemblies [83, 86]. These attributes are (Fig. 1, middle panel) as follows:
(1)
Average amplitude within each segment (microvolts)—as generally discussed above, mainly indicates volume or size of the neuronal assembly; the more the neurons recruited into an assembly through local synchronization of their activities, the higher the amplitude corresponding to this assembly’s oscillation in the EEG [16, 201].
(2)
Average length of segments (milliseconds)—illustrates the functional life-span of a neuronal assembly or the duration of operation produced by this assembly; because a transient neuronal assembly functions during a particular time interval, this period is reflected in EEG as a stabilized interval of quasi-stationary activity [180].
(3)
Coefficient of amplitude variability within segments (%)—shows the stability of local neuronal synchronization within a neuronal assembly [194].
(4)
Average amplitude relation among adjacent segments (%)—indicates neuronal assembly growth (recruitment of new neurons) or disassembling (functional elimination of neurons) [180].
(5)
Average steepness among adjacent segments, estimated in the close area of RTP (%)—shows the speed of neuronal assemblies growing or disassembling [180].
Methodological Results Related to EEG Segmentation
Experimental results revealed a relatively large number of EEG segments per minute for nearly all narrow-frequency bands [73, 83, 139, 180, 200, 202]. For example, there are about 60 quasi-stationary segments for delta activity (~1,000 ms average duration), 120 quasi-stationary segments for theta activity (~500 ms), 200 quasi-stationary segments for alpha activity (~300 ms), 250 quasi-stationary segments for beta-1 activity (~250 ms), 300 quasi-stationary segments for beta-2 activity (~200 ms), and 350 quasi-stationary segments for gamma activity (~170 ms) per each 1-min local EEG during restful wakefulness with closed eyes. Similar relations between durations of quasi-stable states within different frequency bands have been shown in independent research [57]. This range of temporal durations fits very well with the biophysical processes underlying the assemblies’ formation, often iteratively on time scales corresponding to 100–900 ms range [34]. As it has been pointed out by David et al. [203] a neuronal assembly’s operation should be longer than the time for spike transmission between neurons either directly or through a small number of synapses (from few milliseconds to several tens of milliseconds), and it must be shorter than the time it takes for a cognitive act to be completed (which is from several hundreds of milliseconds to a few seconds).
Within such segments, the degree of synchronization (alignment of EPSP/IPSP times in multiple neurons making up the neuronal assembly) can vary in time, so it is important to consider how much variability can be tolerated and still be able to consider assembly member neurons to be “acting together” [204]. Our data have shown that the coefficient of within-segment amplitude variability was relatively low for all studied frequency bands (thus indicating the formation of neuronal assemblies [91]; rest with closed or open eyes), and it was substantially higher (up to 30 %) for the randomly altered EEG (see Note 9) (see also [139]). Such a considerable increase in the values of within-segment amplitude variability in the “random” EEG indicates the absence of stabilized epochs and thus lack of neuronal assembly formation [56]. More precisely it represents an estimation of the maximum possible rate of relative alterations in the amplitude variability for a given EEG [53].
Additionally, it has been shown that average amplitude relations among adjacent segments were substantially lower for the randomly altered EEG when compared to real EEG (rest with closed or open eyes; for an example, see [139]), thus indicating that RTPs are true authentic boundaries of EEG quasi-stationary segments which reflect the episodes of relative stabilization of neuronal activity within separate neuronal assemblies separated by such RTPs [53, 56, 139].
OA theory proposes that the simple operations of neuronal assemblies that we experience subjectively as simple patterns/features of complex thought/image/scene and/or observe objectively as elemental operations of behavior are created and executed in emergent neural fields of self-organized activity of neuronal assemblies [91]. If this is so, then one should observe an RTP in local EEGs (reflecting a reset of a new operation) every time a novel task (or experimental input) is induced. In other words, if RTPs are true EEG markers of transitional moments between local neuronal assembly operations (indicating their start and end), then under experimental conditions the number of RTPs should be much higher at the exact moment of systematic change from one cognitive/mental operation to another (induced start of a new task) when compared to other task-free time coordinates within the same condition or a control condition that does not include systematic change of operations according to an experimental protocol. Indeed, experimental studies have confirmed this hypothesis [73, 200] (for illustration see Fig. 4 in [56]). These findings clearly indicate that RTPs are the true markers of beginnings and ends of brain–mind operations.
Generally, it has been shown that segmental description of local EEG signals within the multichannel EEG recording is very sensitive to a both spontaneous (stimulus independent) and induced (stimulus dependent) brain states [73, 139, 180, 200, 205, 206] (for a review see [86]) as well as pharmacological influence [83, 151, 153, 154] and various pathological conditions [73, 102, 152] (for a review see [86]).
Summarizing this subsection we would like to stress that application of OA methodology to an EEG signal allows researchers to monitor local electric fields of transient neuronal assemblies with millisecond time resolution via extracellularly placed (at the scalp) electrodes (see Note 10) and can be used to interpret many facets of neuronal communication and computation [180]. For example, such parameters as size, life-span, stability, and speed of neuronal assembly growth or disassembly can now be studied robustly as a function of subject’s states, behavioral acts, pharmacology usage, and neurological or psychiatric pathology (for a review see [86]). The main advantage of such methodology over others, used for the investigation of EEG activity, is that not only are the underlying biophysical mechanisms taken into account but also the neurophysiologic processes related to the EEG and reflected in its spatial and temporal nonstationarity are considered [91].
From the first studies of RTP dynamics [52, 73, 139, 200, 208] it has been noticed that when an RTP in EEG amplitude occurs at any one site (separate EEG channel), it tends to simultaneously occur at several sites of the multichannel EEG recording, marking the onset of a spatiotemporal EEG pattern (see Note 11). Peculiarities of such synchronization are discussed in the following subsection.
3.2 Synchronization of Operations of Neuronal Assemblies and EEG Signals
The second (high) level of OA theory describes the temporal synchronization of different brain operations (operational synchrony) that are executed simultaneously by different local and transient neuronal assemblies [91]. Indeed, as it has been observed in the previous subsection, individually each neuronal assembly presents only a partial aspect of the whole object/scene/concept [211], while the wholeness of “perceived” or “imagined” is brought into existence by joint (synchronized) operations of many functional and transient neuronal assemblies in the brain (see Note 12) (for a thorough discussion see [91, 217]; see also [218]). The recombination of neuronal assemblies in new configurations makes it possible to present subjectively a nearly infinite number of different qualities, patterns, objects, scenes, and concepts—even those, with which we have never been acquainted before.
Such synchronization of operations gives rise to a completely new level of brain abstractness—metastable brain states (see Note 13) [108]. These metastable brain states or functional operational modules (OMs), as we name them, accompany the realization of brain complex (and composite) macro-operations (e.g., cognitive percepts, phenomenal objects, and reflective thoughts; for a review see [91, 217]), whereas each of them is instantiated by the particular volumetric spatial–temporal pattern in the electromagnetic field [54–56]. Within the OA theory, the complex operation or operational act has internal structure where each element in its turn also has its own internal structure and so on until the simplest elemental operations are reached (Fig. 2). In other words, there is a more complex operation/operational act that subsumes the simplest ones. It is critical that any complex operation/operational act is not just a conjunction of simplest operations (or operational acts)—but it is also an operation (or operational act) in its own right with emergent properties that are not evident in the subordinate constituents. Such architecture has a clear nested hierarchy [224] and thus could serve as the needed ingredient of brain organization that would allow a conscious mind to be expressed and present the features of consciousness (referred to as neural states, mental unity, qualia, and mental causation), which are discussed in detail elsewhere [54, 91, 217] (see also [170–172]).


Fig. 2
Schematic presentation of the compositionality of operations. Further explanations are provided in the text
Synchronization of EEG Segments: Structural Synchrony Measure
Because the beginning and end of discrete operations of local neuronal assemblies are marked by the sharp changes in local EEG amplitude (RTPs), the simultaneous occurrence of these RTPs found in different local EEG signals within the multichannel EEG recording could provide evidence of neuronal assembly synchronization (located in different brain areas) that participate in the same functional act as a group (Fig. 1, bottom panel), e.g., executing a particular complex operation responsible for a subjective presentation of complex objects, scenes, concepts, or thoughts [54, 91, 217]. Fingelkurts and Fingelkurts [200] were the first who came up with this hypothesis as well as suggested the quantitative method for estimation of such unique type of brain synchrony. The method is named “structural synchrony” (or index of structural synchrony, ISS) since it refers to a synchrony between structures of local EEG signals, though qualitatively it estimates the synchrony among operations of multiple neuronal assemblies; thus, it corresponds to an “operational synchrony” [73]. Later this method has been modified to expand its functionality [56].
The details of this technique are beyond the scope of this chapter; therefore, we concentrate on the essential aspects only. In brief, each RTP in the reference EEG channel (the channel with the minimal number of RTPs from any pair or set of EEG channels) is surrounded by a short “window” (Δt, ms). Any RTP from another (test) EEG channel is considered to coincide if it fell within this window (Fig. 1, bottom panel). It is important to note that such coincidence of RTPs is related to a specific type of signal coupling—the structural synchronization of discrete events—which completely ignores the level of signal synchronization in the intervals (segments) between the coinciding RTPs (see Note 14) [122, 200]. Therefore, this approach explicitly uses the definition of functional connectivity agreed upon in neuroimaging community [226]: Functional connectivity is defined as the temporal correlation between spatially remote neurophysiological events [227]. As it has been noted by Plenz [191], such measure would in fact represent an unbiased estimate of synchronization, because in the absence of any knowledge about the underlying spatial organization of the involved neuronal assemblies, it uses temporal proximity of RTPs to estimate nonrandom interaction [56]. Importantly, ISS could capture both the near-instantaneous synchronous RTPs, comparable to zero phase-locked synchronization between two sites, as well as successively synchronized RTPs, comparable with delayed phase-locked synchronization [122].
To arrive at a direct estimate at the 5 % level of statistical significance (p < 0.05) of the ISS, computer simulation of RTP coupling is undertaken based on random shuffling of time segments marked by RTPs (500 independent trials). As a result of this procedure, the stochastic (random) levels of RTP coupling (ISSstoch), together with the upper and lower thresholds of ISSstoch significance (5 %), are calculated. The ISS tends towards zero if there is no synchronization between EEG segments derived from different EEG channels and has positive or negative values where such synchronization exists. Positive values (higher than upper stochastic level) indicate “active” coupling of EEG segments where synchronization of EEG segments is observed significantly more often than expected by chance (as a result of random shuffling during a computer simulation), whereas negative values (lower than low stochastic level) mark “active” decoupling of segments where synchronization of EEG segments is observed significantly less than expected by chance (as a result of random shuffling during a computer simulation). Dynamics of ISS and its positive and negative levels are shown in Fig. 3. The strength of EEG structural synchrony is proportional to the actual value of ISS: the higher this value, the greater the strength of functional connection.


Fig. 3
Schematic illustration of the index of structural synchrony (ISS) and its stochastic levels. As an example, the calculations of ISS are shown for 16 EEG channels. The Y-axis displays the ISS values found in the experiment (illustrated as bars). The X-axis displays the 120 possible pair combinations of 16 EEG channels. The data presented for a healthy subject in a rest condition with eyes closed
Since most methods that estimate synchronization look at pairs of recording channels only, a global picture of synchronization in multichannel EEG recording is usually obtained by averaging the pairwise synchronization between every possible pair of channels. The main problem with such an approach is its inability to detect the naturally arising synchronization patterns across space and time [228] (for a review see [91]). In contrast to the majority of other synchronization methods (that have a number of other limitations) (see Note 15) the OA methodology uses algorithmic instruments that permit it to estimate synchrony of events in more than two EEG channels.
Structural synchrony can be identified in several (more than two) EEG channels, thus identifying synchrocomplexes (SC). An SC is a set of EEG channels in which each channel forms a paired combination (with valid values of ISS) with all other EEG channels within the same SC, meaning that all pairs of channels in an SC have to have statistically significant ISS values linking them together (Fig. 1, bottom panel). The number of cortical areas (indexed by the synchronized EEG channels) recruited in an SC is described as “the order of areas recruitment” [73, 200, 210]. Therefore, all SCs could be divided into a set of categories based on the number of cortex areas involved: SC2—SC with second order of areas recruitment, SC3—SC with third order of areas recruitment, SC4—SC with forth order of areas recruitment, and so on. Notice that any given SC is considered as a member of its own category (for example, SC3) only if the correspondent RTPs coincided in time among correspondent number of EEG channels (in this example, 3). However, any three SCs2 which could comprise the particular SC3 but which do not coincide in time between each other are not considered as producing SC3 type and, therefore, are not counted as SC3 but instead as SC2. The same logic is applied to any other SC category larger than SC2.
Notice that synchronized RTPs mark transitions between different segments in the EEG signal, which are usually of different type in the various EEG channels. Therefore, the described RTP-based measure of functional connectivity, in contrast to conventional approaches, is free from similarities of the EEG signals in different channels. In this context, the simultaneous stabilization of RTPs across several cortical areas is supposed to reflect the formation of a steady cooperation of operations among those scattered in different cortical areas neuronal assemblies independent of the particular characteristics of these operations [91]. At the more conceptual level, such synchrony presents the relevant suboperations or phenomenal qualia about the external objects (or scenes) reflected in the wave packets of quasi-stationary EEG segments derived from different cortical areas for integration and formation of a unified macroscopic (complex) phenomenal object, scene, or thought as the culminating act of perception, imagination, or thinking (for a detailed discussion see [91, 217]). In this context SCs have a direct relation to the OM estimation.
Operational Module (OM) Estimation
The criterion for defining an OM is a sequence of the same type of SCs of the same category during given epoch of analysis. In other words, the constancy and continuous existence of each OM persist across a sequence of discrete and concatenated segments of stabilized (coupled) local EEG activities (indexed by the same SCs) that constitute that particular OM (Fig. 4; [55, 56]). Conceptually, the continuity of any given OM exists as long as the set of neuronal assemblies located in different brain areas maintains synchronicity between their discrete operations (see Note 16). Analogous to SCs, OMs can be of different order of areas recruitment (indexed by the number of synchronized EEG channels).


Fig. 4
Schematic illustration of operational modules (OMs) and operational space–time (OST). Each OM exists in its own OST, which is “blind” to other possible time and space scales present simultaneously in the brain “system”: all neural assemblies that do not contribute to a particular OM are temporarily and spatially “excluded” from the OST of that particular OM. Further explanations are provided in the text. RTP—rapid transitional processes (boundaries between quasi-stationary EEG segments); SC—momentary synchro-complexes (synchronization of RTPs between several but always the same local EEGs at the particular time instants); F3—the left frontal cortical area; F4—the right frontal cortical area; O1—the left occipital cortical area; O2—the right occipital cortical area; T4—the right temporal cortical area; Pz—the central parietal cortical area. As an example, it is shown that neural assemblies in these areas could synchronize their operations on three different (even though partially intertwined) spatial–temporal scales, thus forming three separate OMs each having its own operational space–time (bottom panel, 3D objects)
The notion of operational space–time (OST) applies here [229]. Intuitively, OST is the abstract (virtual) space and time which is “self-constructed” in the brain each time a particular OM emerges (see Note 17). Formally, the OST concept holds that for a particular complex operation, the spatial distribution of the locations of a particular set of neuronal assemblies together with their synchronous activity at repetitive instants of time (indexed by SCs) comprises the OM. These distributed locations of that set of neuronal assemblies are discrete, and their proximity as well as the activity in the “in-between area(s),” delimited by the known locations of neuronal assemblies, are not considered in the definition (only the exact locations of a particular set of neuronal assemblies are relevant). Also, between the moments in time that particular locations of the neuronal assemblies synchronize, there can be smaller subset(s) of these locations synchronized between themselves or with other neural locations, though these do not relate to the same space–time of the same OM (although they may relate to some other OM). Therefore several OMs, each with its own OST, can coexist at the same time within the same volumetric electromagnetic field [91]. The sketch of this general idea (based on experimental data) is presented in Fig. 4.
According to OA theory, the metastable OMs at an OST level somehow “freeze” and “classify” the ever-changing and multiform stream of our cognition and conscious experiences [91]. In this sense, the succession of complex cognitive operations, phenomenal images, or thoughts is presented by the succession of discrete and relatively stable OMs, which are separated by RTPs, i.e., abrupt changes of OMs (see Note 18) (see Fig. 5). As it has been shown experimentally [52, 83, 84, 139, 148, 153, 202, 208, 232], at the critical point of transition in a mental state, e.g., during changes from one task/thought to another, the OM undergoes a profound reconfiguration which is expressed through the following process [91]: A set of local bioelectrical fields (which constitute an OM) produced by transient neuronal assemblies located in several brain areas rapidly loses functional couplings with one another and establishes new couplings within another set of local bioelectrical fields, thus demarcating a new OM in the volumetric OST continuum of the brain. Using this mechanism the brain determines who “talks” to whom at any particular moment. In this sense, the brain can “rewire” itself dynamically and functionally on a milliseconds time scale without changing the synaptic hardware (see Note 19) [57, 235].


Fig. 5
Schematic diagram depicting dynamics of operational modules (OMs). Relatively stable complex OMs (outlined by the red line) undergo abrupt changes simultaneously with changes in cognitive task. Such abrupt changes marked as rapid transitional periods (RTPs). Grey shapes illustrate individual OMs. Red line illustrates complex OMs. For interpretation of the references to color in this figure legend, the reader is referred to the Web version of this chapter. This scheme is based on data published in ref. 247
Methodological Results Related to EEG Structural Synchrony
Already first experimental studies [52, 73, 139, 200, 208, 232] had shown that OMs (specific spatiotemporal configuration of stabilized segments among local EEG fields) indeed exist, thus confirming the discovery of a new and previously unknown type of brain functional connectivity. It was found that such OMs are characterized by different order of recruitment of cortical areas: from any two to the whole cortex. Surprisingly, analysis has shown [200, 232] that independently from the condition or the functional state all independent OMs of second order of cortical areas recruitment that do not participate in any OM of a higher order of areas recruitment have negative ISS values. This means that any two cortical sites tend to actively decouple their operations if they are not bound to a third site or several other areas concurrently. In other words, if two areas of the cortex are operationally synchronized, they also tend to be synchronized with some other area(s).
Recent calculations showed that the power-law statistics governs the probability that a particular number of cortical areas are recruited into an OM (defined as the temporal RTP coincidences among different EEG channels). This ubiquitous dependency is characterized by a fractal relation between different levels of resolution of the data, a property also called self-organized criticality [236]. Thus, it has been shown that OMs are indeed driven by a renewal process with power index μ ≈ 2 [237–239] (see Note 20), which is in line with Beggs and Plenz’s [244] avalanche research. An avalanche is defined as a spontaneous and abrupt burst of activity observed on variable numbers of electrodes for different periods of time separated by silent or quasi-stable periods [191]. Neuronal avalanche behavior is an indication that the cortex is working in a critical condition. Critical systems exhibit scale-free, power-law dynamics at many levels of description during transitions between order and disorder, and their universal behavior is independent of the specific realization of system components [245].
It has been shown that independent OMs of the second order of areas recruitment which have negative ISS values constitute only 14 % from total number of all possible OMs formed by two cortical areas that are part of triple, quadruple, and higher degree OMs [73, 200]. These higher degree OMs have only positive values of ISS. Thus, one may conclude that the brain “prefers” to synchronize three or more cortical areas in order to execute ongoing complex operations; otherwise, neuronal assemblies tend to work autonomously. Our analysis also revealed that such stabilized spatiotemporal OM configurations have transient dynamics which is expressed as a series of sudden transitions between OMs [73, 139, 200, 208]. What is the life-span of OMs with different order of areas recruitment?
From an information-theory point of view, one may suppose that large (covering most or the whole cortex) long-lasting OMs are not efficient in the healthy brain [178] because context-dependent information transfer is necessarily more transitory and would require dynamic reconfiguration of OMs as well as existence of many OMs. This intuition has been confirmed experimentally [73]: it has been shown that the average life-span of OMs is longest for OMs with second order of areas recruitment (~30 s in average) and shortest for OMs that span most or the whole cortex (~100 ms in average) (see Note 21). Thus, one may conclude that the brain operates as a highly dynamic system where large spatial–temporal patterns of stabilized activity (indexed as OMs) are formed only for a very brief episode and quickly dissolve allowing the brain (as a whole) to have more degrees of freedom to form new OMs needed to execute newly immediately emerged and ever-changing operations. Therefore, the dynamics of cortex functional organization is usually dominated by local interactions between brain regions. Here it is important to note that some types of OMs of particular categories are surprisingly stable and persist across all studied experimental conditions in all studied subjects [73, 232, 247]. Initially we have interpreted such OMs as responsible for long-lasting, complex brain operations and body “housekeeping” tasks [229]. However, it later became evident [98] that, as a whole, these highly stable OMs constituted a set of cortical areas that has been named the “default mode network” (DMN) [248]. Nowadays researchers tend to associate the DMN either with stimulus-independent thought [249, 250] or with the “autobiographical” self [249, 251], or being a “self,” or having self-consciousness [170, 252]. Indeed, as we have discussed in other work [98], a subject that experiences phenomenal self-consciousness always feels directly present in the center of an externalized multimodal perceptual reality [253, 254]. This is why the set of particular OMs that constitute a DMN and altogether specify the sense (probably in an implicit form) of “being a self” is always active, even during realization of some cognitive (or other) tasks and conditions (including dreaming), independently of their complexity [98].
Coupling of neuronal assemblies is usually examined within single-frequency bands (meaning on a particular temporal scale). At the same time, as noted by Buzsáki and Draguhn [34], different oscillatory frequencies might carry different dimensions of brain integration, and the coupling of operations of neuronal assemblies between two or more temporal scales (frequency bands) could provide enhanced combinatorial opportunities for storing/processing/presenting complex spatiotemporal patterns [91, 255]. The central assumption behind such a view is that oscillations within different frequency bands reflect different types of cognitive processes [11, 39, 106, 256] and that different oscillations become synchronized if the task (or condition) demands require a co-activation or an integration of the respective cognitive processes [256, 257].
Considering the polyphonic character (mixture of different frequency oscillations) of the EEG field [11, 16, 39] and the hierarchical nature (different time scales) of segmental descriptions of local EEG fields [52–54, 56], OMs could coexist on and between different temporal (frequency oscillations) scales. In addition to topographical relationships, cross-frequency synchronization is capable of describing synchronous activity between different neuronal assemblies that may spatially overlap under the same EEG electrode.
The OA methodology enables researches to study such cross-frequency coupling in a direct manner (see Note 22) [56]. In contrast to many other methods (for a critique see [258]), it (a) requires no a priori assumptions about which frequency bands should be synchronized but rather relies on the natural statistical properties of the data to reveal cross-frequency synchronization; (b) is sensitive to transient changes in cross-frequency coupling over both time and frequency; (c) can simultaneously assess multiple cross-frequency synchronizations (i.e., different synchronizations in different frequency bands), and (d) is well suited for investigating possible cross-frequency coupling during dynamic and/or brief cognitive or perceptual events [210].
From the first studies in this field [73, 200, 255] it became evident that segmental flows among the EEG frequency components are more or less synchronized and depend on the character of information processing of brain activity. Interestingly, such synchrony is independent of narrow frequency bands’ closeness within broad EEG spectral pattern [73, 255]. For example, the ISS is not always higher in the alpha1–alpha2 pair of frequency bands (neighboring oscillations) when compared to delta–beta1 pair of frequency bands (non-neighboring oscillations). The principal finding is that ISS between basic EEG rhythms (delta, theta, alpha1, alpha2, beta1, beta2) decreases in each local cortical area as cognitive loading increases (see Note 23) [73]. Another finding concerns the occipital-frontal gradient: ISS values for the cross-frequency synchrony increase during rest condition in the direction from occipital to frontal cortical areas, while they decrease along the same direction during cognitive activity (see Note 24) [73] (for an illustration see [56]).
Together, these findings pointed to the existence of not only spatially nested neuronal assemblies, but also to a dynamically organized nested structure of OMs between different temporal scales [224] (see also [167, 256]). In such functionally nested architecture, the ongoing brain activity during an awake resting state is characterized by (1) increased coupling between operations of neuronal assemblies located within the same cortical area, though performing their operations on different temporal scales, and at the same time (2) decreased coupling between operations of neuronal assemblies located in different cortical areas, though performing their operations on the same temporal scale. In response to cognitive loading such dependency reverses: local cross-scale synchrony decreases while the topographical same-scale synchrony increases [73, 232].
Our research has shown [73, 200, 255] that OMs (being themselves the result of synchronized operations produced by distributed transitive neuronal assemblies) are capable of further operational synchronization between each other both within the same and across different temporal scales, thus forming a more abstract and complex OM which constitutes an integrated experience [91, 217]. In such operational architecture, each of the complex OMs is not just a sum of simpler OMs but rather a natural union of abstractions about simpler OMs. Therefore, OMs have a rich combinatorial complexity and the ability to reconfigure themselves rapidly, which is crucially important for the presentation of highly dynamic cognitive and phenomenal (subjective) experience [91, 217]. Yet the opposite process is also possible, where any complex OM can be partitioned into a set of sub-modules, and to that effect each sub-module may be further decomposed into sub-sub-modules, stripping these processes all the way down to basic operations. Such decomposition would be responsible for a segmentation of our subjective experience and focused attention. In other words, such operational architecture has the fractal property of hierarchical modularity, multi-scale modularity, or, as Meunier and co-workers called it, “Russian doll” modularity [259].
Taking into account the hierarchy of segmental description of EEG in different temporal and spatial scales, it could be suggested that the discrete structure of brain activity depicted in the EEG piecewise spatiotemporal stationary structure is the operational framework within which a variety of rapid “microscopic” variables can obey the “macroscopic” operational structure of brain activity [91]. Thus, the spatial and temporal nested hierarchy of discrete metastable states of neuronal assemblies can serve as the basis for functioning of such a potentially multivariable system like the brain [108].
What are the functional benefits that make such nested hierarchy of virtual modules of brain activity so attractive for brain functioning? One of the earliest propositions formulated by Simon [260] states that a “nearly decomposable” system that comprises multiple and sparsely interconnected modules allows more rapid adaptation of the system in response to changing environmental conditions (see also [261]). In respect to a central nervous system, there could be several important advantages: (a) Networks of functional and dynamic modules have the property of small-worldness which is advantageous because it favors locally segregated processing (with low wiring cost) of specialized functions (for example visual or auditory detection) due to the high clustering of couplings between nodes in the same module while at the same time support globally integrated operations of more generic functions (for example working memory) due to the short path length [259]. Such double tendencies intervened within the same modules demonstrate the principle of metastability [108]. (b) The topology of functional and dynamic modules is associated with rich nonlinear dynamical behavior: temporal scale separation due to fast intra-modular processes and slow inter-modular processes [262] as well as high dynamical complexity due to the coexistence of both segregated and integrated activity [108, 211, 263]. Meunier et al. [259] observed that the presence of modules allows some neuronal activity to remain locally encapsulated while at the same time maintaining a dynamical balance where dynamical activity is maintained between the extremes of rapidly “dying out” and invading the whole network, as marginally stable modules can be combined or divided while preserving stability [264–266]. (c) Another advantage of virtual modules is their optimality at performing tasks in a changing environment [259], where a certain set of simple (basic) operations are required and where a combination (coupling) of these “building operations” is needed to solve complex tasks [91, 217].
4 Implications of OA Methodology
In the previous section we have briefly observed the main tenets and general methodological results of operational architectonics framework for EEG analysis. The main advantage of such methodology is the fact that it allows researchers to study the inherent local and global processes in the EEG field at a common level of formal analysis. According to this analysis all multivariability and complexity of brain operations are reflected in the nested hierarchy of dynamic local fields of neuronal assemblies [91, 256]. In the following subsections we overview the implications of OA methodology for specific domains.
4.1 Implications for Cognitive Neuroscience
There are several major problems in cognitive neuroscience that attract many resources, with researchers aiming to resolve or clarify them. One such problem is a working memory. Even though it has been understood already for some time that memory (and working memory in particular) reflects a distributed property of large-scale cortical systems [35, 218, 267, 268], the particular contribution of neuronal assemblies located in different cortical areas during episodic encoding and retrieval processes is still uncertain and only partially understood [269].
The application of OA methodology to memorizing conditions revealed rich data about the neurophysiological mechanisms behind different stages of the memory task [73, 139, 200, 208, 232, 247]. The experiments were designed in such a way that it was possible to test resting, waiting, encoding, keeping-in-mind (retaining), and identification (retrieval) short-term periods of the memory task separately and in their natural chronology.
The most straightforward result was that bursts of abrupt OM formations were observed at the exact moments when one stage of a memory task was switching over to another in a controllable manner, for example, transition from encoding to retention [73, 200]. This finding clearly indicates that formation of virtual OMs is guided by the need to execute complex cognitive or mental operations. Since each complex operation is a coupling of several simpler ones [91, 217], one may expect to find many OMs of different complexity present within each stage of the memory task. This is indeed the case. The diversity of OMs grows as cognitive loading increases, reaching its maximum at the retention stage (Fig. 6, upper panel). It were OMs with the small and medium order of areas recruitment that contributed the most to such increase in OM diversity [73, 200, 232, 247]. This observation can be easily interpreted as an increased need for independent brain operations that parallel the need to anticipate, perceive, encode, and then keep-in-mind external stimuli during the studied increase of cognitive loading of a memory task. Indeed, in agreement with the general understanding, many neuronal assemblies with distributed parallel processing are active and must synchronize their operations in order to be able to execute the needed cognitive operations as a basis for successful memory performance [247] (see also [16, 257, 270, 271]).


Fig. 6
Schematic presentation of the dynamics of diversity and life-span of OMs (upper panel) and average operational synchrony among all basic frequency bands within each EEG channel (bottom panel) as a function of cognitive loading during different stages of working memory task. This scheme is based on data published in ref. 247
Interestingly, the increase in diversity of sets of OMs along the increase of cognitive loading is also accompanied by the increase of life-span of respected OMs (Fig. 6, upper panel). For example, the average life-span of OMs was minimal during resting stage (before stimuli presentation) and reached its maximum at the retention stage of the memory task [73, 232, 247]. It can be explained that during an increase in the cognitive loading, the growing number of complex cognitive operations also paralleled by an increase of their durations. Such prolonged duration of complex cognitive operations during encoding and especially retention stages of a memory task is probably an important condition for successful memorization [73, 232, 247]. However, detailed analysis revealed a more complex picture.
Even though the rate of successfully memorized stimuli in our experiments was quite high (up to 90 %) some trials for each subject were not successful—the item was not memorized correctly. Therefore, it was interesting to study the diversity and life-span of OMs as a function of high and low memorability in the same subjects. It has been found that the maximum diversity as well as longest life-span of OMs were found in trials with low memorization [73, 232], thus indicating that an excess of couplings of operations and too rigid dynamics of such coupled operations is incompatible with successful memorization. Interestingly, such an excess in the number and life-span of OMs was already present at the “waiting” stage (before the stimulus has been presented), where subjects only prepare to memorize the future coming stimuli [73, 232]. Thus, such an excess is not an event-related phenomenon but reflects a process mode that most likely can be interpreted in terms of a top-down process, for example the mentation (association thoughts) not related to the current task. The fact that such mentation could directly contribute to the process of forgetting has been documented by Klimesch et al. [272].
Thus, the findings presented here suggest that a particular number of specific medium-sized and with medium life-span OMs (that “cover” certain cortical areas) seems necessary to achieve successful memorization [73, 232, 247]. Indeed, although memory encoding, retention, and retrieval often share common regions of the cortex, the operational synchrony of these areas is always unique and presented as a mosaic of nested OMs for each stage of the short-term memory task [73, 232, 247]. When there are too few or too many OMs and their life-span is either too short or too long, then such conditions lead to cessation of efficient cognitive operation. Such dynamic balance of integrated and segregated processes as a necessary and sufficient condition to produce efficient cognitive activity (and eventually consciousness) is becoming increasingly recognized [91, 169, 184, 217, 220–222, 273].
As we have discussed above, operational synchrony can exist across a number of functional domains, with different frequency rhythms associated with each domain, thus indicating cross-frequency synchronization [256–258, 274, 275]. Application of OA methodology to different stages of a working memory task reveals the progressive decrease of average values of operational synchrony among all basic frequency bands (delta, theta, alpha1, alpha2, beta1, and beta2) in a cognitive load-dependent manner (Fig. 6, bottom panel) in each cortical location [73, 200, 232]. These data corroborated with the fact of parallel increase of operational synchrony between different cortical locations—spatial synchrony within the same frequency domain (Fig. 6, upper panel). Together these results clearly indicate that the decrease of cross-frequency synchrony in each cortical location releases the needed degrees of freedom of the neuronal assemblies (located in such cortical areas), allowing them to perform synchronously their operations on a particular temporal scale (frequency) between different cortical locations as a function of cognitive loading [73, 232].
At the same time, operational synchrony between some particular pairs of EEG frequencies increased as a function of cognitive loading during the various stages of working memory task [73, 200, 232]. These frequencies were mostly alpha1–beta1 and alpha2–beta1 and to a lesser extent delta–theta and theta–beta2 pairs that were positively correlated with memory loading in all frontal, central, temporal, parietal, and occipital regions. On the contrary, operational synchrony in the delta–alpha2 and alpha1–alpha2 pairs decreased as a function of cognitive loading during working memory task. A similar wide-band alpha–beta coupling has been observed using the method of a very-narrow-band EEG analysis to characterize the working memory encoding and retention [276] and also during pharmacological influences [277, 278]. Thus, the current findings support earlier suggestions for complementary roles of alpha and beta rhythms during working memory and cognitive loading [256, 275, 279, 280]. In line with this understanding, alpha and beta band network topologies (neuronal assemblies) have similar characteristics when compared to other frequency bands: they are more clustered and small-world-like [281]. Hence, operational synchrony between them is much more likely.
Coupling between theta and beta frequency bands has been previously observed in animals [282] and humans [283] during states of increased vigilance. These observations are in line with our more recent finding on increased coupling (indexed by operational synchrony) between theta and beta1 frequencies during increased cognitive load of a memory task [73, 200, 232]. The decreased coupling between alpha1 and alpha2 frequency bands during working memory most likely reflects a non-synchronous suppression of both components of alpha activity, which also have different functional meaning (“attention” for slow alpha and “engram consolidation” for the fast alpha; see [272, 284]).
Interestingly, the trials with high memorability were characterized by a very strong coupling between alpha2 and beta1 frequency bands, while trials with low memorability (in the same subjects) had strong synchrony in the alpha1–beta1 band pair [73, 200, 232]. These findings are in line with earlier studies of Klimesch [272] who demonstrated that subjects with faster alpha rhythm performed better on a memory task compared to subjects with slower alpha activity. An important difference though of data presented here is that the subjects were the same, but when the trial happened to be unsuccessful (subjects failed to memorize a given stimuli) it was alpha1 that was operationally synchronized with beta1, while during the successful trials (subjects memorized well) it was alpha2 that was synchronized with beta1 [73, 232].
There was also another characteristic feature of the unsuccessful trials: topographical operational synchrony between segmental structure of alpha activity in any given cortical area and segmental structure of theta activity in all other cortical areas had negative values of ISS, thus indicating unbinding of operations performed by neuronal assemblies operating on different temporal scales [73, 232]. For the successful trials such topographical alpha–theta operational synchrony on the contrary increased as a function of cognitive loading throughout the working memory task [73]. The reported results suggest that forgetting is associated with some sort of unbinding of operations performed by particular neuronal assemblies (for a similar conclusion see [285]). Most interestingly, such negative values of ISS (that indicate unbinding) between alpha and theta rhythms were observed over the entirety of the unsuccessful trials: pre-stimulus as well as post-stimulus. Thus, one may conclude that such alpha–theta cross-frequency decoupling is not an event-related phenomenon but rather reflects a process that can be interpreted in terms of top-down controlled processes [257]. Other situations, where operational synchrony exhibits negative ISS values, include (a) the failing of conscious binding of multisensory features into a coherent mental “object” [286] and (b) fading of consciousness altogether as in vegetative and to a smaller degree in minimally conscious patients [287, 288].
To summarize this subsection, findings on OM dynamics clearly point to the fact that the binding of sensory feature representations into phenomenal (subjective) “objects” and active encoding, maintenance, and retrieval of these mental “objects” during working memory are critically dependent on dynamic millisecond-range synchronization of multiple operations performed by local neuronal assemblies that operate on different temporal (oscillations) scales nested within the same operational hierarchy [91, 256].
4.2 Implications for Clinical Practice
After years of research, modern neuroscience conceptualizes the human brain as a complex nested hierarchy of functionally specialized neuronal assemblies that interact with each other in a spatially and temporally coherent fashion [170–172, 256]. By means of such interactions, these neuronal assemblies and their larger synchronized conglomerates (OMs) shape physiological (normal) and pathological (diseased) behaviors [86]. Despite the wide spread of neuropsychiatric disorders [289, 290] and the progress in the basic neuroscience, there is only little advance in understanding the pathophysiology of such disorders and correspondent to it delay in the development of effective therapies [291]. We believe that this is due to a lack of a consistent paradigm of psycho- and neuropathology that would incorporate the novel knowledge from basic neuroscience. At the same time, such a novel paradigm that stresses the dynamic balance between isolated functions of local neuronal assemblies and globally coordinated activity between them is beginning to emerge [291]. It has been suggested that the loss of such a metastable balance in favor of either independent or hyper-ordered processing leads to pathological states that give rise to neuropsychiatric syndromes constituting a particular disorder [86, 151] (see also [291, 292]). This subsection reviews current knowledge about OMs’ dynamics in different pathologies derived from application of OA methodology in neurological and psychiatric conditions.
Chronic Opioid Addiction
It has been found that the number of OMs and strength of coupling within such OMs (estimated by an ISS) were significantly lower in chronic opioid abusers than in healthy controls [152]. This dependence was observed in alpha and beta frequency bands. Such disruption in the formation of virtual OMs is consistent with disorganization syndrome of cortex processes (suggested by Bressler [292]). Most likely, the disrupted operational connectivity between local and distributed neuronal assemblies is a candidate mechanism for the well-documented pattern of impairment in addicts, expressed as lack of integration of different cognitive functions for effective problem solving, deficits in abstract concept formation, set maintenance, set shifting, behavioral control, and problems in the regulation of affect and behavior [293–296]. From this perspective then, disorganization in the cortex activity of chronic opioid addicts is in favor of independent processing that was paralleled by increased volume, stability, and life-span of local neuronal assemblies in such addicts [152]. Furthermore, it has been found that the more years the subjects abuse opioids on a daily basis the more disintegration (indexed by ISS) takes place within the posterior section (low central, parietal, low temporal, and occipital areas) of the cortex. Hence, these findings give ground to suppose that longitudinal opioid abuse preferentially impairs the formation of OMs in the posterior cortex section. Areas located in this part of cortex are suggested to be responsible for a number of important functions [297]: perception, differentiation and somatosensory functions, memory functions and autobiographical records, as well as visual perception. Therefore one may suggest that the absence of appropriate relations between all these functions in opioid abuse patients due to a broken mechanism of OM formation leads such patients to specific maladaptive actions such as those directed at drug-seeking and drug-taking behavior which characterize addiction [152].
Opioid Withdrawal/Abstinence
Based on clinical and cognitive psychology studies it is known that when addicts crave for the drug, anxiety, nervousness, lack of inhibitory control, positive drug-related expectancies, and intrusive thoughts related to drugs are all simultaneously present [298, 299]. Consistent with the OA methodology framework, these complex cognitive functions are critically based on the dynamical interactions of operations performed by many cortical neuronal assemblies (see also [64, 271, 300, 301]). Considering that withdrawal/abstinence initiates a widespread parallel activation of cortical regions responsible for the cognitive operations mentioned above, one can expect to see a significant increase in formation of many OMs. Such increased operational synchrony among many transient neuronal assemblies would then explain the strong motivation of abstinent patients for the excessive drug craving, where the dynamics of local brain operations (functions) would be restrained by the large-scale context (removal of the aversive state) of mutually connected cortical areas. Application of OA methodology to EEG of abstinent patients fully confirms this hypothesis: a significant increase in formation of many OMs of different complexity (with a focus in the anterior part of the cortex) was found in opioid-dependent patients during withdrawal as compared to healthy controls [153]. It has also been documented that the complexity of OMs and strength of coupling within OMs (indexed by ISS) have had a predictive force towards the severity of abstinence: patients with strong withdrawal symptoms had higher number and strength of couplings among neuronal assemblies than patients with mild symptoms [153].
Major Depression
Even though the modern model of major depression stresses the key role of anterior asymmetry—the so-called cognitive anterior model of depression [302], from the point of view of metastable balance hypothesis outlined above, major depression could generally be viewed as a disorder of disturbed neuronal assemblies’ plasticity, leading to an inadequate relationship between multiple operations produced by these neuronal assemblies. Application of OA methodology to EEG of medication-free patients with major depression reveals a widespread and significant increase in formation of OMs when compared to healthy subjects, with greater number of small OMs in the left hemisphere and large OMs in the right hemisphere [148]. Such peculiarities of operational synchrony could be interpreted as signs of adaptive (over)compensation of the “depressed” brain in an attempt to achieve more adequate semantic context which is presented differently in left and right hemispheres [303] through establishing a new overall metastable brain state [108]. It has been suggested that in the process of such overcompensation, connections between neuronal representations of negative affect and different semantic concepts become strongly activated [148]. This hypothesis found confirmation in a semantic network model study [304, 305] in which both semantic and affective features are represented as nodes in the network. It was found that people who are depressed suffer from strongly activated connections between negative affective nodes and multiple semantic concepts, creating feedback loops that maintain depressive affect and mentation [306–308]. This is why depressed individuals tend to see even positive information as negative because it becomes associated with personally relevant negative information [309]. The observed type of a new metastable brain state with increased operational synchrony could be a possible mechanism underlying the maintenance of a depressive state [148].
Schizophrenia
Schizophrenia is another mental disorder whose pathophysiological mechanisms could be better understood if it is viewed as a disorder of metastable balance between large-scale integration (formation of OMs) and independent processing (local transient neuronal assemblies) in the cortex, favoring independent operations [156, 157, 291, 292]. Application of OA methodology to patients with schizophrenia [155] reveals a significant decrease of operational synchrony among remote neuronal assemblies (indexed as OM formation) in schizophrenic patients when compared to healthy subjects [86]. Such low level of operational synchrony may signify a well-documented pattern of mental impairment in schizophrenics that expresses a lack of integration of different cognitive functions for effective problem solving, deficits in abstract concept formation, set maintenance, set shifting, behavioral control, and problems in the regulation of affect and behavior [310, 311]. Since in this study negative and positive symptomology were mixed, one could not make a firm conclusion of how different symptomology would be reflected in the operational architectonics of EEG. One may suppose though that high values of positive symptomology in patients with schizophrenia would be paralleled by rather increased level of OM formation; this hypothesis needs to be tested in a separate study.
Epilepsy
The epileptic brain presents a unique pathological condition that, despite the fact that it is the most common disorder of the nervous system [312], is still poorly understood. A recently emerging view that brain disorders and associated psychiatric problems are accompanied by disruption in the spatiotemporal structure of integrative brain activity [313], where this structure is either more irregular (uncorrelated randomness) or more regular (excessive order) than in normal healthy brain, could be of a particular help [151]. Application of OA methodology to an epileptic brain during the interictal (without signs of epileptiform abnormalities) periods in medication-free patients with chronic idiopathic generalized epilepsy reveals that (a) interictal EEG is characterized by OMs which contain more cortex areas than healthy control EEG and (b) brain oscillations of cortex regions involved in such OMs tend to have longer periods of temporal stabilization in interictal EEG when compared with control EEG [66]. Both findings suggest less dynamic performance of cooperative brain operations (dynamic rigidity) in patients with chronic epilepsy than in healthy subjects. Generally these findings pointed to a conclusion that chronic epilepsy should be determined not by a focus of pathological activity, but rather by an epileptic system, that contains a set of coupled distributed oscillatory states (or resonances) involved in a common activity [66].
Summarizing this subsection we could express the hope that the effort to look at many chronic disabling mental or neurological disorders as conditions with dynamical disruption in the spatiotemporal functional structure of integrative brain activity [64, 271, 291, 292, 300, 301, 313] would result in a new generation of evidence-based diagnosis and treatment strategies [314, 315].
4.3 Implications for Somnology Studies
In mammals sleep is associated with specific cortical EEG patterns, generally divided into rapid eye movement (REM) and non-rapid eye movement (NREM) (see Note 25) sleep [317]. During REM sleep EEG is desynchronized and hippocampal theta rhythms are present, while during NREM sleep EEG is characterized by the presence of slow (delta) waves, sleep spindles, and K-complexes [318]. As recently observed by Mignot [318], it is likely that sleep is a distributed process with some neuronal systems being the primary drivers. Interactions between sleep- or wake-specific neuronal assemblies must ensure that the processes occur in synchrony and in exclusion of each other to create stable states of wake, NREM, and REM, with limited time spent in transition states. This view is highly compatible with OA methodology, according to which many transient neuronal assemblies rapidly synchronize their operations in order to present the stability of a particular complex state/operation [91].
So far the OA methodology has been applied only to EEG from NREM sleep. Results show that during NREM sleep the size of neuronal assemblies gets smaller, while the life-span increased compared to wakefulness state with closed eyes [86]. Furthermore, NREM sleep was characterized with increased formation of OMs, though coupling strength within such modules was not strong [86]. These findings mark a weak communication among neuronal assemblies located in different cortex areas during NREM sleep, allowing some level of flexibility with quick reorganization of synchronized neuronal assemblies into different combinations (OMs) in order to present a large number of possible cognitive operations needed for proper learning and memory consolidation which are posited to take place during NREM sleep [319–321].
Additionally, dividing NREM sleep on the dream-present and dream-absent epochs reveals that NREM dream condition (which is usually presented with simple, static, isolated image(s) or thought(s) and by one modality [322]) is characterized by short-lived small neuronal assemblies, long-lived large neuronal assemblies, and a significant increase in OM formation when compared to dreamless NREM condition [91].
Further studies are needed to detail OA organization of the brain during REM and NREM conditions as well as during sleep pathologies.
4.4 Implications for Neuropharmacology
As we have discussed above, recent research emphasizes that the majority of brain disorders, and psychiatric/mental problems are accompanied by disruption in the temporal and metastable structure of brain activity [313], where this temporal structure could be either more irregular (uncorrelated randomness) or more regular (excessive order) than normal [291, 292, 323, 324]. From this perspective, it has been suggested that the future of psychopharmacology lies in its ability to design psychotropic drugs that can restore the normal temporal structure and metastable structure of brain activity [151]. This approach seems more physiologically adequate to integrative, nonstationary, and self-organized nature of brain processes and fits in with a novel understanding of the dynamical nature of brain diseases, where the so-called lesions in time become more evident especially in the early stages of the disease than lesions in structure [325]. In this context, it is important to study how different psychotropic drugs can modify temporal/metastable structure of brain activity in healthy subjects and patients.
Application of OA methodology to EEGs recorded under the influence of lorazepam (GABAA agonist) reveals that the number of OMs and the strength of coupling within such OMs significantly increased under the lorazepam administration [84]. It is important to note that it was a randomized, double-blind, cross-over, placebo-controlled study. In the same study, it was found that different-sized neuronal assemblies in alpha and beta frequency bands performed differently under lorazepam when compared with placebo [83]: For the alpha-generated neuronal assemblies, it was observed that large neuronal populations exhibited a total decrease in size, functional life-span, and stability under lorazepam administration when compared to placebo. In contrast, small neuronal assemblies were very stable. The functional life-span of all beta-generated neuronal assemblies was prolonged [83]. These findings are likely to reflect the prolongation of inhibitory neuronal operations that manifest in the form of slowing of cognitive performance under the lorazepam administration [326]. It has also been suggested that, probably, strong enhancement of GABAergic function by lorazepam “mimics” the conditions of an immature brain [84], where excitatory actions of GABA provide most of the initial activity, a primitive signal with poor information content that propagates to all brain structures [327].
In contrast, heroin imposes a full disruption in the OMs’ formation with a parallel decrease in coupling strength within the OMs [152]. As we have discussed above, such disruption of operational synchrony among neuronal assemblies located in different cortical areas is consistent with cortex disorganization syndrome (suggested by Bressler [292]). It is likely that heroin-induced disruption of operational synchrony may constitute the candidate mechanism for the well-documented pattern of cognitive impairment in heroin users: lack of integration of different cognitive functions, deficits in abstract concept formation and behavioral control, and problems in the regulation of affect and behavior [293, 294, 296].
Since methadone is sometimes used as a maintenance treatment for heroin-dependent patients [328], it was interesting to study its effect on the EEG operational architectonics in such patients. Application of the OA methodology to EEGs of patients who were on methadone treatment during many months showed that methadone restored the normal values of number and coupling strength of OMs [154] and consequently normalized the metastable organization of the cortex in all patients (for a discussion see [108]). This conclusion is consistent with the current theoretical view that normal brain function is the product of a large-scale network of coupled neuronal assemblies exhibiting transient and inherently metastable dynamics [122, 168, 219, 329].
Summarizing this subsection we hope that the application of OA approach for EEG analysis will contribute to a current effort in developing a more rational psychopharmacology that takes into consideration the novel views on brain/mental disorders.
4.5 Implications for Ontological Development Research
There are several critical questions in the developmental EEG studies: What is the physiological basis for the synchrony within oscillations in human EEG over the developmental life-span? If oscillations reflect important cognitive processes then do different synchronicity modes within and between oscillations contribute to the development of different aspects of human cognition? In order to get some insight into these questions, we reanalyzed results of OA methodology which was applied to EEGs of healthy 12-year-old teenagers [155] and healthy 30-year-old adults [86]. The results revealed that the formation of OMs and the strength of coupling within the observed OMs were smaller in teenagers when compared to adults (p < 0.01, Wilcoxon test). These findings are in agreement with current understanding that with age the repertoire of the states of individual neuronal assemblies located in different cortical regions becomes bigger due to increased specialization and that at the same time there is increased integration between distributed neuronal populations responsible for different cognitive operations in adults [330]. In general, these findings are in line with modern neo-Piagetian models of cognitive development from childhood to adulthood [331–335]. They are also consistent with previous EEG coherence studies [118, 336–338] which show that as the number of connections increases with age then there is accompanied increased coherence (synchronicity), increased stability, and decreased “chaos” [339].
4.6 Implications for Personality Feature Studies
The dynamic balance between synchronization and desynchronization of cognitive operations is considered essential for normal brain function [86, 291, 292], as we have discussed above. If this is so, then such balance paralleled by EEG operational architecture balance should (a) have noticeable stability within individuals and (b) exhibit variability between individuals—thus being the “signature” of personality. This issue has not studied at a proper detail yet; however, some preliminary data is emerging. Applying the OA methodology to EEGs of monozygotic twins and comparing the OA indexes within the monozygotic pairs (genetically related subjects) and between members from different pairs (genetically unrelated subjects) revealed that the formation of OMs, their diversity, and types were nearly identical within members of the monozygotic pairs but differ significantly in participants from different monozygotic twin pairs [73, 200]. Specifically, the average Spearman rank correlation in the intra-pair and inter-pair similarities of OMs for the resting condition was 0.53 for genetically related and −0.13 for genetically unrelated subjects. These findings point to the existence of particular dynamic OM profiles that are individually predetermined and most likely have high heritability. The possibility of high genetic heritability for EEG functional connectivity has been documented in other independent studies [29, 337–340].
It has also been shown that such OM profiles correlate significantly with different cognitive, affective styles of subjects and their personality features [200]: for example, verbal type of thinking, normal level of anxiety and neuroticism, and phlegmatic temperament type (see Note 26) altogether characterize subjects with a high number of large OMs in the left hemisphere with a focus in anterior part of the cortex. Subjects with nonverbal/abstract type of thinking, diminished level of situational anxiety and neuroticism, and sanguine temperament type were characterized by a diverse set of large OMs in the right hemisphere with a focus in the posterior part of the cortex (Fig. 7, upper plane). Interestingly, such dependence between OM profiles and personality features was “hardwired” during rest condition only. During a particular cognitive task, the given OM profile tends to transform in order to execute a particular task. For example, if the initial personal OM profile was verbal but the cognitive task was visual (nonverbal), then during such visual task the verbal OM profile characteristics became similar to a nonverbal OM profile (Fig. 7, bottom plane), though the efficiency of subjects with such transformed profile during the visual cognitive task was slightly lower than the efficiency of subjects with initially present nonverbal OM profile [200]. These findings have been confirmed in the test–retest study which took place after 2 weeks.


Fig. 7
The position of OMs typical for individuals with different personality features. Circles indicate the locations of most frequently found OMs. Further explanations are provided in the text
Generally these data suggest that human individual cognitive abilities relate to a particular operational architectonics of the brain resting-state activity that is expressed as a nested spatial and temporal nonstationarity of EEG field which though possesses some degree of freedom that allows it to reorganize itself in order to execute a particular complex task or operation. Such a view is congruent with the hypothesis of Bekhtereva [343], who proposed that brain activity during the resting state may reflect the brain’s potential processing abilities and is correlated with individual differences in the cognitive process (for recent research see [344, 345]).
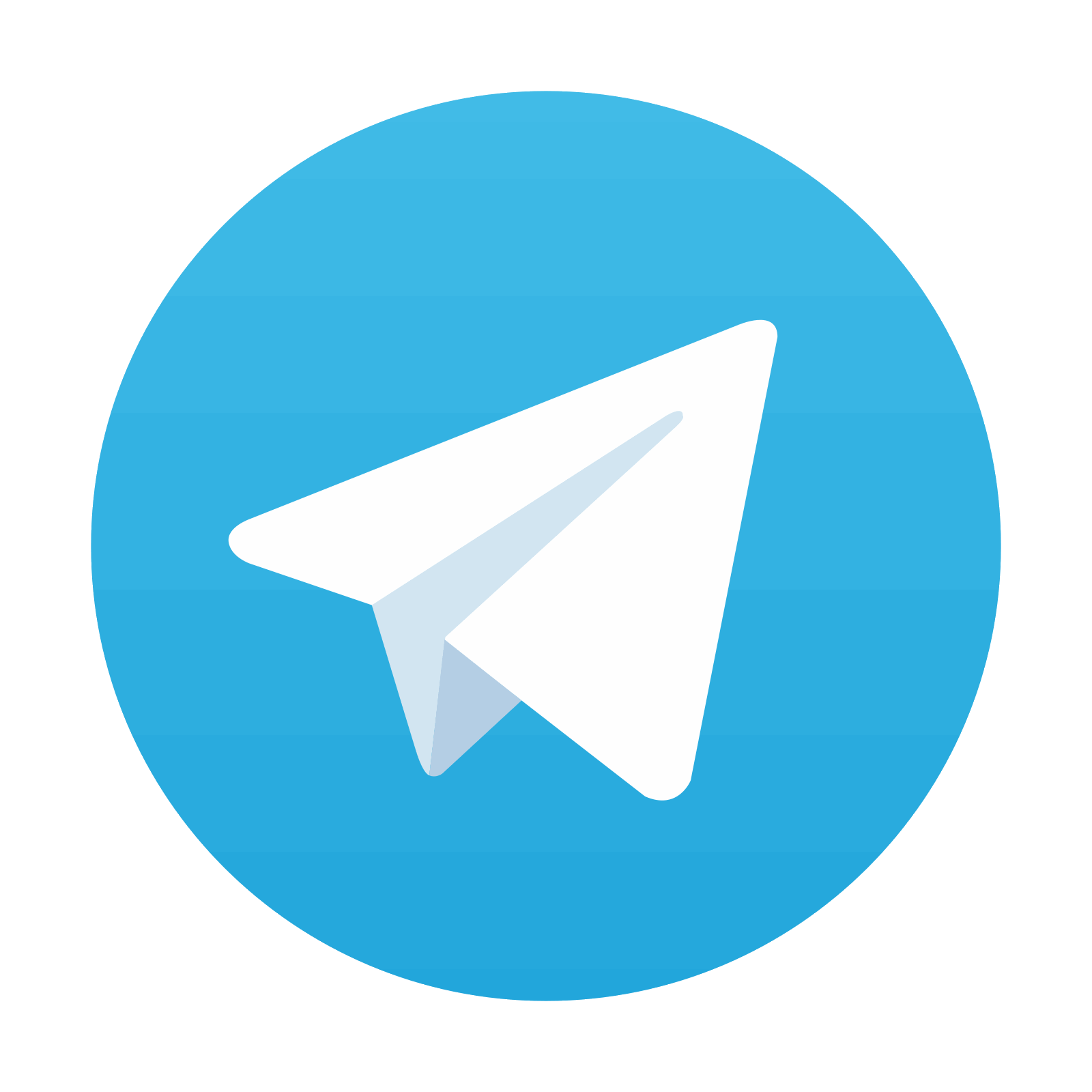
Stay updated, free articles. Join our Telegram channel

Full access? Get Clinical Tree
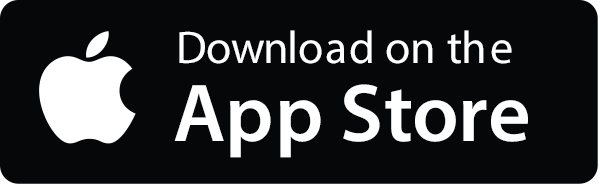
