We saw in Chapter 6 that larger studies are less likely to get the wrong results due to chance (or random sampling error) than smaller studies; however, the example in Box 7.1 shows that a large sample size is not sufficient to ensure we get the right results. The enormous presidential poll conducted by the Literary Digest didn’t get the right answer because it included the ‘wrong’ people, i.e. they were not representative of everybody in the voting population. Furthermore, in epidemiology we frequently rely on records that have been collected for some other purpose, and we have already discussed some of the problems inherent in this in Chapter 3. Even when the data we use have been collected specifically for our research they are unlikely to be completely free of error. We often have to rely on people’s memories, but how accurate are they? And biological measurements such as blood pressure and weight are often subject to natural variation as well as being affected by the performance of the measurement system that we use.
In the run-up to the 1936 presidential election in America, the Literary Digest conducted a poll of more than two million voters and confidently predicted that the Republican candidate, Alf Landon, would win. On the day it was the Democrat candidate, Franklin D. Roosevelt, who won a landslide victory. The Digest had correctly predicted the winner of the previous five elections, so what went wrong in 1936?
The Digest sent polling papers to households listed in telephone directories and car registration records. In 1936, however, telephone and car ownership were more common among more affluent households and these were the people who were also more likely to vote Republican. The generally less-affluent Democrat voters were thus under-represented in the sample of voters polled. In contrast, a young George Gallup conducted a much smaller poll of a few thousand representative voters and correctly predicted the Roosevelt win. As a result of this fiasco the Digest folded but Gallup polls are still conducted today.
People live complicated lives and, unlike laboratory scientists who can control all aspects of their experiments, epidemiologists have to work with that complexity. As a result, no epidemiological study will ever be perfect. Even an apparently straightforward survey of, say, alcohol consumption in a community can be fraught with problems. Who should be included in the survey? How do you measure alcohol consumption reliably? All we can do when we conduct a study is aim to minimise error as far as possible, and then assess the practical effects of any unavoidable error. A critical aspect of epidemiology is, therefore, the ability to recognise potential sources of error and, more importantly, to assess the likely effects of any error, both in your own work and in the work of others. In this chapter we will point out some of the most common sources of such error in epidemiological studies and how these can best be avoided. We also want to emphasise from the outset that some degree of error is inevitable, but this need not invalidate the results of a study.
Sources of error in epidemiological studies
In an epidemiological study we usually want to measure the proportion of people with a particular characteristic or identify the association between an exposure and an outcome. To do this we have to recruit individuals into the study, measure their exposure and/or outcome status and then, if appropriate, calculate a measure of association between the exposure and outcome. We also want the results we obtain to be as close to the truth as possible. (Note that, although we will discuss error in the context of exposure and disease, when we talk about an exposure we mean anything from a gene to a particular behaviour, and the outcome need not be a disease but could be any health-related state.)
As you will discover, there are dozens of different names that have been given to the kinds of error that can occur in epidemiological studies. Fortunately, in practice, all types of error can be classified into one of two main areas: they relate either to the selection of participants for study or comparison, or to the measurement of exposure and/or outcome. These errors can in turn be either random or systematic. Random error or poor precision is the divergence, by chance alone, of a measurement from the true value. Systematic error occurs when measurements differ from the truth in a non-random way.
We will now discuss the main types of both selection and measurement error in more detail and will also consider the effects that they may have on the results of a study. Remember that in practice it is impossible to eliminate all error and the most important thing is therefore to consider the likely practical effects of any remaining error.
Selection bias
Depending on how we select subjects for our study, and how many we select, we can introduce both random and systematic sampling errors into our study. As you saw in the previous chapter, even if the people selected for a study are generally representative of the population that we wish to learn about (the target population), we may still get the wrong result just because of random sampling error, i.e. by chance, and this is especially likely when we take only a small sample. In contrast, the example in Box 7.1 shows how the results of even a large study can be biased if the sample of people selected for the study systematically differ from the population that we wish to learn about in some way.
Selection bias occurs when there is a systematic difference between the people who are included in a study and those who are not, or when study and comparison groups are selected inappropriately or using different criteria. Unlike random sampling error, we cannot reduce selection bias by simply increasing the size of the study sample – the problem persists no matter how large the sample.
The issue of selection bias is a major problem in simple descriptive studies such as prevalence surveys. If the sample of people included in the survey is not representative of the wider population the results of the survey can be very wrong, as the Literary Digest found in their biased opinion poll which under-represented the views of poorer Americans. In analytic studies, selection bias can add to the differences between groups being compared, thereby moving them further from the ideal of complete exchangeability that we discussed in Chapter 4. This will potentially lead to biased measures of association (OR, RR, AR or PAR). It is a particular concern in case–control studies because the participants are recruited as two separate groups and it can be difficult to ensure that the final control group makes an appropriate comparison group for the cases.
A similar problem can arise in cohort studies when the exposed and unexposed groups are recruited separately, for example when the exposed group comprises workers in a particular occupation or military group and a separate unexposed group has to be identified for comparison. However, in many cohort studies, such as the Framingham and Nurses’ Health studies that we discussed in Chapter 4, we recruit a single group of participants and then classify them according to their exposure. In this situation the question of how individuals were recruited is usually less important in terms of the validity of the study results (what is often called internal validity). However, it can influence the generalisability or external validity of the findings because they may apply only to the sorts of people who took part. In some situations, however, selection bias (at the point of recruitment) can also bias the effect estimates from a cohort study. As an example, consider a cohort study examining the effect of children’s socioeconomic status (SES) on their risk of injury. If the families of lowest SES are more likely to refuse to participate, then this group may be under-represented in the total cohort. In this situation, measurement of the risk of injury within the low SES group and comparisons with those of higher SES should still be accurate; the low SES group will just be smaller than it might have been had more low SES families participated. If, however, those families of lower SES who refuse to take part are also those whose children are at highest risk of injury, i.e. if participation is associated with both the exposure (SES) and the outcome (injury), then the study will underestimate the true amount of injury in this group. It will then also underestimate the effect of low SES on injury risk because the really high-risk children in that group were not included.
As for cohort studies, selection bias at recruitment and exposure assignment is not usually a major issue for internal validity in clinical trials, although it can occur if the allocation process is predictable and the decision whether or not to enter a person into the trial is influenced by the expected treatment assignment. For example, if alternate patients are assigned to receive active drug or placebo, a physician may decide not to enter sicker patients into the trial if he or she thought they were not going to be given the active drug. This selection bias will affect the internal validity of the study and is another reason why the allocation process should be truly random and ideally neither the investigators nor the participant should know what group the participant is in (see Chapter 4).
For both cohort and intervention studies the more important issue is to avoid or minimise ‘loss to follow-up’ because selection bias can arise if those who remain in a study are different from those who do not, i.e. the issue is selection out of the study population rather than selection in.
Some specific sources of selection bias
Some common ways in which selection bias can arise include the following.
Volunteers
It is well known that people who volunteer to participate in surveys and studies (i.e. they spontaneously offer their involvement rather than being selected in a formal sampling scheme) are different from those who do not volunteer. In particular, volunteers are often more health-conscious and, as a result, volunteer groups will often contain a lower proportion of, say, smokers than the general population. Advertisements calling for volunteers for a survey or study may also attract people who have a personal interest in the topic area. The prevalence of various diseases or behaviours in a volunteer group may thus be very different from that in the underlying population because of this self-selection into the study. This means that volunteer groups are completely unsuitable for surveys conducted to measure the prevalence of either health behaviours or diseases in the population and they are also likely to introduce bias into studies looking for associations between exposures and health outcomes. For this reason, epidemiological research rarely uses groups of haphazardly recruited volunteers and, if it does, it is advisable to pay close attention to whether this may have biased the results in some way.
Imagine a survey about a sensitive area such as sexual behaviour where participants were recruited via advertisements in women’s magazines. How representative do you think the results would be of all women?
There are two potential problems with this type of recruitment. First, different magazines target different types of women so it is likely that the readers of one particular magazine will not be representative of all women. It is also likely that the women who choose to respond to a survey of this type will differ markedly from those who do not respond; for example, they may well be more confident and out-going and thus more likely to engage in less conventional sexual behaviours (Maslow and Sakoda, 1952). This exact issue plagued Kinsey who conducted some of the earliest work on sexual behaviour in the mid-1900s (Kinsey, 1948). He reported high levels of unconventional sexual behaviours in his study groups, but was roundly criticised for using samples of volunteers, prisoners and male prostitutes, thus raising concerns about the reliability of his results. Although Kinsey attempted to address these criticisms, the concerns remained and his results still cause controversy today.
Low response rates
What might be thought of as a type of volunteer bias, and one that again is a particular problem in surveys and case–control studies, is the problem of low response rates. People who have a particular disease are often highly motivated to take part in research into that disease. Controls, however, have no such motivation to participate and investigators are finding it increasingly hard to persuade healthy people to take part in research with the result that control participation rates are now often around 50%. Even if potential controls for a study are selected at random, if a large proportion do not agree to take part then the remaining group may no longer be a true random sample of the population and the results may be biased. Box 7.2 shows an example from a study looking at passive smoking and heart attack where the authors assessed and reported the likely extent of error in their estimates of smoking rates in the control group. This degree of thoroughness is commendable but, unfortunately, rarely seen due to logistical constraints. Note also how this information can be used to make a tentative practical assessment of the likely bias this error may have introduced into the estimate of the effect of passive smoking on heart disease.
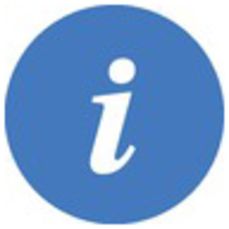
In a case–control study of the effects of passive smoking on the risk of heart attack or coronary death, the investigators put a lot of effort into trying to achieve a high response rate from controls. Potential controls were initially invited to attend a study centre where they would have blood collected and physical measurements taken as well as completing a risk factor questionnaire. Participants who did not respond to this invitation were sent a shorter questionnaire to complete at home and some people who still did not respond were then visited and interviewed at their homes. There were thus three types of people among the control group: the willing volunteers who replied to the initial invitation, the slightly less willing who replied to the shorter home questionnaire and the even more reluctant who agreed to take part only when visited by an interviewer. The investigators then compared the prevalence of smoking in these three groups (Table 7.1).
Ease of recruitment | Never smoker (%) | Ex-smokers (%) | Current smokers (%) |
---|---|---|---|
Men (age 35–69 years) | |||
Full participation (willing) | 35 | 40 | 24 |
Short questionnaire (less willing) | 30 | 42 | 28 |
Home interview (reluctant) | 29 | 42 | 29 |
Women (age 35–69 years) | |||
Full participation (willing) | 67 | 19 | 14 |
Short questionnaire (less willing) | 66 | 13 | 21 |
Home interview (reluctant) | 53 | 16 | 31 |
The harder it was to persuade someone to take part in the study, the more likely they were to be a current smoker, especially for women. This suggests that those who refused completely probably had even higher smoking rates. The measured prevalence of smoking in the control group is therefore likely to be an underestimate of the true level of smoking in the whole population. Using the study data, the calculated odds ratio for the association between smoking and heart disease in men was 2.3. However, if the true proportion of current smokers in the population was actually 3% higher and the proportion of non-smokers 3% lower than in the study controls, then the true odds ratio would have been lower, about 1.8. The study would thus have overestimated the strength of the association.
Loss to follow-up
In a case–control study the main concern with subject selection is with regard to who is included in the study. For both cohort and intervention studies the more important issue is to avoid or minimise selective losses from the cohort or study group. This can be a particular problem if more people are ‘lost to follow-up’ in one exposure group than another (i.e. loss is associated with exposure) and if loss is also related to the outcome of interest. For example, imagine a randomised clinical trial comparing a new drug with the current standard treatment. If the sickest people in the intervention group withdrew from the trial, the people remaining in the intervention group would be healthier than those in the standard treatment group and the new drug would appear to be more beneficial than it really was. The opposite situation would occur if those who were doing well were less likely to return for assessments and thus were more likely to be lost to follow-up. In a cohort study, participants with socially stigmatised behaviours (which these days can include smoking cigarettes) may be both less easy to follow-up and more likely to develop the health conditions being studied.
Ascertainment or detection bias
This can occur if an individual’s chance of being diagnosed as having a particular disease is related to whether they have been ‘exposed’ to the factor of interest. An example of this type of bias was seen in early studies of the association between oral contraceptive (OC) use and thromboembolism (a condition in which a blood clot develops in the legs and subsequently breaks off and moves to another part of the body, often the lungs). Doctors who were aware of the potential for this risk were more likely to hospitalise women with symptoms suspicious of thromboembolism if they were taking OCs. Early case–control studies, which were hospital-based, then overestimated the risk of thromboembolism associated with OC use. This was because the cases were more likely to be on OCs simply because of the way in which they were selected to be sent to hospital, because in the minds of their doctors this partly determined their diagnosis.
The healthy-worker effect
This is a well-documented type of selection bias that can occur in occupational studies. People who are working have to be healthy enough to do their job, so they tend to be more robust than the general population, which necessarily includes those who are disabled or seriously ill and hence unable to work. As a result, if occupational groups are compared with the general population – which is not uncommon in cohort studies of occupational hazards – they will almost always appear to be healthier overall. Comparisons within a workplace can also be flawed because different types of job often attract different types of people as well as requiring different levels of fitness. Imagine a study of the effects of heavy physical work on the occurrence of heart disease in which the investigators compared a group of manual labourers with a group of people of similar SES who had desk jobs. In this situation, people who had heart disease might be incapable of doing a manual job and therefore more likely to hold a desk job. The frequency of heart disease would thus appear to be higher in those with desk jobs, falsely suggesting that heavy work was protective against heart disease. Similar problems can arise in other groups where members are selected on the basis of physical capability, e.g. the armed forces (see Box 7.3).
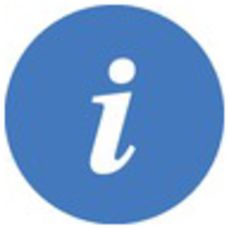
There is concern that men and women who saw active service in conflicts such as the Vietnam War have worse health than those who did not. Studies that have compared mortality rates among Vietnam veterans with those in the general population are hampered by the fact that the veterans had to pass a stringent medical examination at the time of their enlistment and so, at that time, were much more healthy than the average person. An analysis of mortality rates among male Australian Vietnam veterans found that, up until 1979, mortality among the veterans was actually 18% lower than in the general population (Table 7.2). It is highly unlikely that service in Vietnam would reduce a man’s subsequent risk of death, so this inverse association is likely to be due entirely to the healthy-worker (or in this case, healthy-warrior) effect. It is impossible to say how large this effect might be and to assess whether it could actually be masking an underlying increase in mortality in the veterans.
However, in the years from 1980 to 2001, overall mortality among the veterans was similar to that in the general population and cancer mortality was more than 20% higher among the veterans. With the increasing time interval since enlistment, the healthy-worker effect will have been wearing off for most causes and it now appears that the veterans do have higher rates of cancer death compared with the general population. The question of veterans’ health is now a major issue in many countries.
Control of selection bias
The question of selection bias has to be considered and then potential bias eliminated or minimised in the design and conduct of a study. Any error introduced here that leads to inappropriate comparisons cannot easily be removed in the data analysis although, as shown in the example in Box 7.2, it is sometimes possible to estimate the effects of any such bias; we will discuss this further below.
In any study, it is important to have a clear definition of the population group that you want to study (the target population). This need not be everybody, but could be a specific subgroup of the whole population, and study participants should then be selected to represent this group. In a descriptive study it is essential to ensure that the study population really is representative of the target population or any measures of disease (incidence or prevalence) may be biased. In a case–control study the critical issues are defining the case group clearly and selecting an appropriate control group. Ideally all cases from the defined population would be included, but if only a sample is used they should be truly representative of all cases arising in the population. The controls should also be selected to be representative of the same population. (We discussed options for control selection in Chapter 4.) It is then important to ensure high participation rates among both cases and controls.
A good study will also have clearly defined eligibility criteria to determine whether specific individuals are included. For example, in a study of myocardial infarction, specific criteria developed by the World Health Organization might be used to define a case or, in a study of cancer, only those patients with histologically confirmed cancer might be eligible. Additional eligibility criteria might require people to fall within a certain age range (e.g. children are usually excluded from studies of adult diseases), reside in a defined area or be admitted to specific hospitals. Box 7.4 gives typical eligibility and exclusion criteria, here for a case–control study of ovarian cancer.
Eligibility criteria for cases for a study of ovarian cancer could be as follows:
A histologically confirmed diagnosis: the cancer must be confirmed by a pathologist.
Incident: the woman must have no previous history of ovarian cancer.
Primary ovarian cancer: the cancer must originate in the ovary; metastases (cancers that have spread from another anatomical site) would thus be excluded.
Age 18–79: studies often exclude children for practical reasons and in this case ovarian cancer is very rare in children. Older adults are also commonly excluded, particularly if exposure information is to be collected by questionnaire or interview because the problems of recall increase with age.
Resident in a specific geographical area: women who just happen to be diagnosed with ovarian cancer while visiting that region will be excluded.
Comparable eligibility criteria for the controls might then be the following:
Women aged 18–79.
Resident in the same specific geographical area.
No previous history of ovarian cancer.
No history of bilateral oophorectomy (i.e. they must have at least one ovary and so be at risk of developing ovarian cancer).
Exclusion criteria might include the following:
Women who are unable to give informed consent (for example, they have dementia).
Women who are too sick to participate (this decision might be made by the treating doctor).
Women who do not speak English (if the main study documents are all in English it might not be financially viable to translate them into other languages).
Note that the eligibility criteria describe the target population, i.e. all women who are eligible to take part in the study. For practical reasons some eligible women might later be excluded from the study. It is important to note that if large numbers of women are excluded, regardless of how good the reasons for this, then the resulting study sample might no longer be representative of the whole population. For example, the exclusion of very sick women might mean that cases of advanced cancer are under-represented in the study group. If advanced cancers differ somehow from early cancers in terms of their aetiology then this might affect the overall results. In experiments testing new treatments, older and sicker patients are often excluded, making it more likely that adverse drug effects will be missed, only to appear once the wider population is exposed to the drug.
Exclusion criteria in trials: In 1999 rofecoxib (Vioxx) was introduced as a new anti-inflammatory drug for management of osteoarthritis. It was withdrawn in 2004 because users had elevated risks of cardiovascular disease. One reason the adverse effects were not picked up sooner was that many of the early trials only enrolled people at low risk of cardiovascular disease (Krumholz et al., 2007).
In a cohort study or trial, one of the most important criteria for a high-quality study is to ensure complete follow-up of all participants because, as you have seen, the more people who are ‘lost to follow-up’ with unknown health status, the more likely it is that the results will be biased. It is therefore important to have measures to maximise retention of people within the study and, if possible, to follow-up those who drop out of the study. Data linkage, which we discussed in Chapter 3, can be helpful here because if the outcome of interest is likely to be captured in routine health records it may be possible to obtain this information for all of the people in the study even if they have dropped out of the study or can no longer be contacted individually. For example, studies with cancer incidence or mortality as an outcome can often use population-based cancer or death registers to obtain this information.
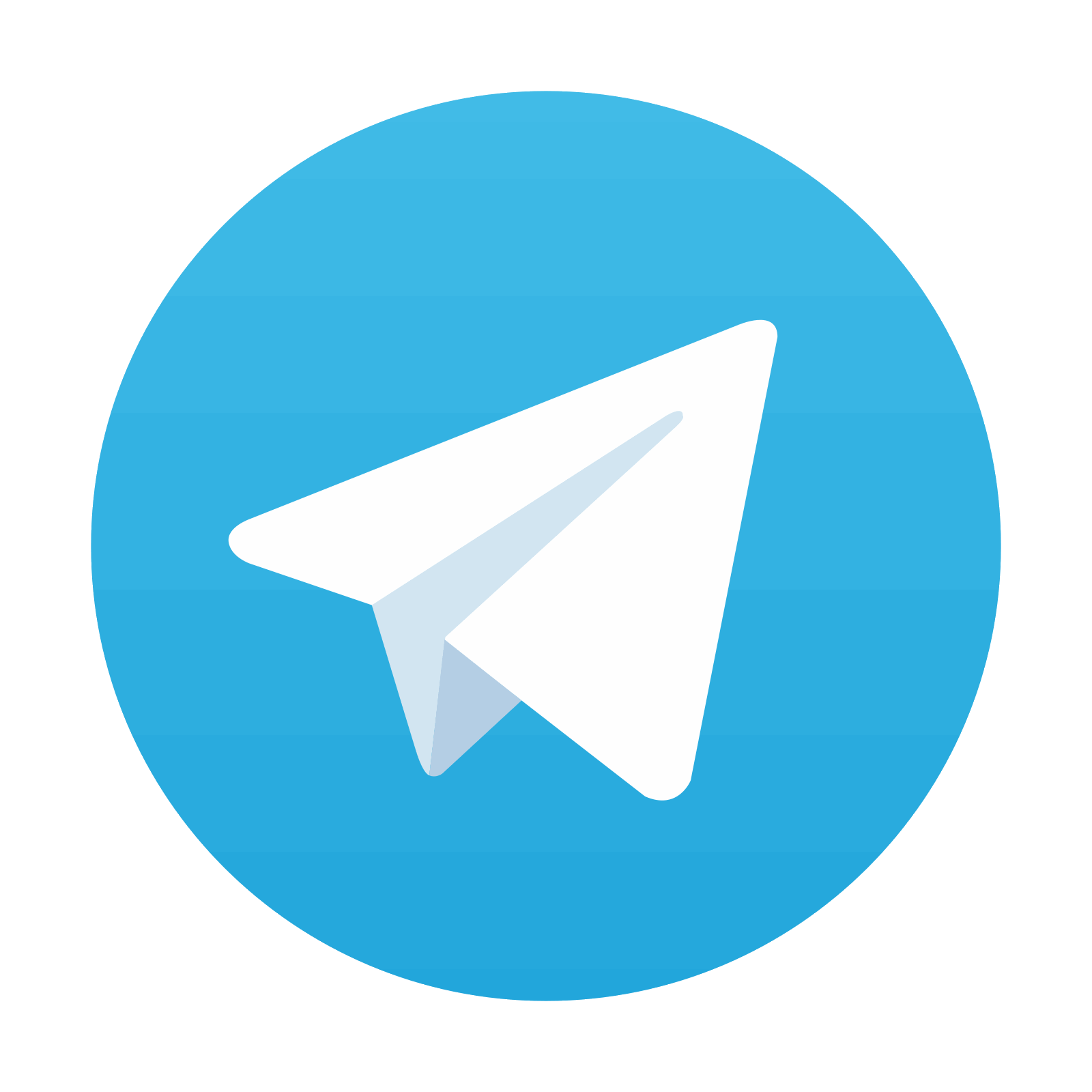
Stay updated, free articles. Join our Telegram channel

Full access? Get Clinical Tree
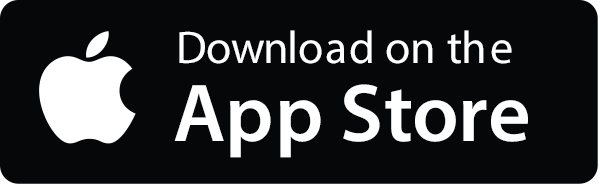
