The limits of memory
The sheer quantity of medical information, even within a single speciality, is often beyond the power of one person to comprehend. People, that is, the human brain, simply cannot cope with the amount of information that they need to function safely and effectively. For instance, more than 600 drugs require adjustment of doses for multiple levels of renal dysfunction; an easy task for a computer, but one which will inevitably be performed poorly by a person (Bates and Gawande, 2003). Machines can therefore act as a kind of extended memory, which we can access at will, to overcome the transience and limitations of human memory storage. However, these are not the only problems of memory; there are other limiting factors which are not always appreciated.
In his review of memory’s strengths and imperfections, Daniel Schachter (1999) identified ‘seven sins’ of memory, each of which has application and relevance to clinical work. The first three are sins of omission, the next three instances of distortion or inaccuracy and the final one concerns memories we would rather forget. I have added examples of how these ‘sins’ might manifest in a clinical environment:
- Transience, meaning that information fades over time, or is at least less accessible. A doctor might forget that a patient has poor renal function when prescribing.
- Absent-mindedness, meaning inattention and consequent weak memory traces. A nurse might read and remember 500 when the label says 50.
- Blocking, temporary inaccessibility of memories, the so-called tip of the tongue phenomenon. A doctor might be unable to recall a drug dosage even though they had given the drug many times before.
- Misattribution involves attributing a recollection or idea to the wrong source, such as thinking that a particular scene from a film came from another with a similar theme. When seeing a patient in a clinic, a doctor might recall and act on a medical history that in fact applies to a different patient.
- Suggestibility. Studies of eye witness testimony have shown that we easily and unknowingly adjust our memories to accord with new information and become convinced that our new ‘memories’ are veridical. An example would be unintentionally convincing your patient that they had had an angiogram, even though they did not.
- Bias involves retrospective distortions and unconscious inferences that are related to current knowledge and beliefs; we adjust our memory of events to accord with our current experience, whether good or bad. For instance, remembering incorrectly that you had noticed previously that a cancer patient showed early signs of the disease consistent with your current diagnosis.
- Persistence refers to pathological memories: information or events we wish we could forget but cannot. The distressing memory of your worst mistake that comes to mind at unexpected moments.
Our memory then, while generally highly effective and efficient in daily life, may lead us astray in a number of ways. An example of an instance in which relying on memory led to disaster is shown in Box 13.1. There are, of course, many other lessons to be taken from this story of wrong site surgery, particularly about personal responsibility, hierarchy and communication. However, the fallibility of memory is a core theme; relying on remembering that the biopsy was taken from the right side in the face of evidence from the medical record that it came from the left is, to put it charitably, not entirely sensible. Schachter points out though that we should not necessarily conclude that memory is hopelessly flawed. Most of these features which make us fallible in some circumstances are also adaptive. Forgetting unnecessary information, such as where you parked your car the day before yesterday, is highly adaptive. Jorge Luis Borges’ story, Funes the Memorious, imagines a man who forgets nothing; Funes is paralysed by reminiscence. Real life examples exist of mnemonists with perfect recall who are unable to function at an abstract level through being inundated with detail. A perfect memory in a computer is marvellous; in a person it could be a liability.
BOX 13.1 Hemivulvectomy for vulvar cancer: the wrong side removed
A 33-year-old female with microinvasive vulvar carcinoma was admitted to a teaching hospital for a unilateral hemivulvectomy. After the patient was intubated for general anaesthesia, the trainee reviewed her chart and noted that the positive biopsy was from the left side. As the trainee prepared to make an incision on the left side of the vulva the attending surgeon stopped him and redirected him to the right side. The trainee informed the attending surgeon that he had just reviewed the chart and learned that the positive biopsy had come from the left. The attending surgeon informed the trainee that he himself performed the biopsies and recalled that they were taken from the right side. The trainee complied and performed a right hemivulvectomy.
The next day, the Chief of Pathology called the trainee to enquire about the case. The specimen he received was labelled ‘right hemivulvectomy’ and did not reveal any evidence of cancer. The pre-operative biopsies the pathologist had reviewed had been positive, however they were labelled ‘left vulvar biopsy’. He wondered if there had been a labelling error.
The trainee informed the pathologist that the right side had been removed, and then informed the surgeon about the error. The attending surgeon denied that any error had been made; he insisted that the original biopsies had been mislabelled. The surgeon did not inform the patient of the error. When the patient returned for routine follow-up the surgeon performed a vulvar colposcopy and biopsied the left side. Microinvasive cancer was noted in the biopsies. Shortly thereafter, the patient underwent a second hemivulvectomy to treat her vulvar cancer.
(REPRODUCED FROM BRITISH MEDICAL JOURNAL, DAVID W BATES. “USING INFORMATION TECHNOLOGY TO REDUCE RATES OF MEDICATION ERRORS IN HOSPITALS”. 320, NO. 7237, [737–740], 2000, WITH PERMISSION FROM BMJ PUBLISHING GROUP LTD.)
The fallibility of memory is an everyday experience, which is generally not too embarrassing to admit. Using devices to compensate, whether a shopping list, a diary or a computer, comes easily to us. Our judgements and decisions however, are more precious to our self esteem and there is much more resistance to allowing guidelines and protocols, whether on paper or instantiated in software, to take over human decisions. This was memorably expressed by Franc¸ois, Duc de al Roche Foucauld in 1666 in his Maximes, when he pointed out that ‘Everyone complains about their memory, but no one complains about their judgement’
In other spheres, such as navigation, judgement has given way to measurement and calculation and now to computation. My grandfather, flying in the First World War, navigated by compass and flying along railway lines, dipping down to inspect the countryside from time to time. My father, flying a Sunderland flying boat in the Second World War, made careful calculations of direction, wind speed and compass bearing, taking into account the discrepancy of true and magnetic north and the error introduced in the compass reading by the metal hull of the aeroplane. Today, an onboard computer just sorts it all out.
Research on judgement (weighing the options) and decision making (choosing amongst the options) has yielded different perspectives on human abilities. On the one hand, the naturalistic decision-making school, most powerfully and persuasively represented by Gary Klein (1998), has shown how experts can rapidly assess a dangerous situation and, far from analysing and choosing, seem to just ‘know’ what to do. A firefighter can just see that the fire is in the basement and the building above is about to collapse; a physician takes one look at a patient and sees that they are dangerously hypoglycaemic. Klein describes this as ‘recognition primed decision making’, rapid, adaptive and effective. This is the classic image of the expert physician who assesses a complex set of symptoms and immediately perceives the correct diagnosis. It is difficult, though not impossible, to imagine replacing this kind of intuitive brilliance with the stolid, systematic approach of a computer. In principle, these decisions could be handled by a machine; in practice, the time spent entering the relevant data might be the limiting factor.
Consider, however, some other common medical scenarios, such as assessing the risk of suicide. A psychiatrist must consider past history, diagnosis, previous attempts at self harm, declared intention and family support available, then weigh all these factors and decide whether the patient can return to the community. Or, consider a paediatric cardiac surgeon weighing up the risks of operating on a tiny baby: anatomy of the heart, pulmonary artery pressure, findings from the echocardiogram and a host of other features may be considered to assess the likely short- and long-term outcomes for the child of operating now, operating in six months or not operating at all. Both of these decisions involve complex calculations, weighing of different factors and combining them to produce a judgement between two or more choices. People must assemble the information, but would a machine or an algorithm make a better decision? In fact, numerous studies have shown that we vastly overestimate our power to make such judgements and that we also overestimate the number of factors that we take into account. Using statistical methods and models is nearly always superior to using unaided human judgement (Box 13.2) (Hastie and Dawes, 2001). This phenomenon, of the superiority of statistical over clinical and other expert judgement, was first documented by Paul Meehl in 1954. In the most recent update of his findings, (Grove and Meehl, 1996), Meehl concluded that empirical comparisons show that the mechanical (statistical, whether computer or calculated) method is almost invariably equal or superior to the human judgement.
BOX 13.2 Clinical and statistical prediction
A world expert on Hodgkin’s disease and two assistants rated nine characteristics of biopsies taken from patients and assessed overall severity’ as a predictor of longevity. In fact, when experts judged the disease to be more severe, patients actually lived slightly longer; the judgement trend was in the wrong direction. In contrast, a multiple regression model based on the same nine characteristics showed a clear, though not strong, reliable association between actual and predicted longevity.
30 experienced psychologists and psychiatrists predicted the dangerousness of 40 newly admitted psychiatric patients. The experts were provided with 19 cues, mostly derived from the judgements of psychiatrists who interviewed the patients on admission. The human judges predicted the likelihood of violent assault on another person in the first week of hospitalization with an accuracy of 0.12; the most accurate human judge scored 0.36. In contrast, a linear statistical model achieved an accuracy of 0.82 with the same data.
(REPRODUCED FROM HASTIE R. & DAWES R.M. RATIONAL CHOICE IN AN UNCERTAIN WORLD: THE PSYCHOLOGY OF JUDGMENT AND DECISION MAKING, 2001, WITH PERMISSION FROM SAGE PUBLICATIONS INC, CALIFORNIA)
The field of judgement and decision making is vast and the issue of human ability and fallibility much debated. My intention is simply to show that, in some instances at least, there is good reason for thinking that the computational aspects of some medical decisions might be more consistently and accurately carried out by a computer than by a person, however expert. Decision support therefore may, if used appropriately, have a major impact on patient safety.
One of the key problems for the future then will be discovering where technology can help and where we need to rely on human judgement. As Bates et al. (2001) point out, human beings are erratic and err in unexpected ways, yet we are also resourceful and inventive and can recover from errors and crises. In comparison, machines, at least most of those currently in use, are dependable but also dependably stupid. An almost perfect instruction, quite good enough for any human operator, can completely disable a machine. Human beings also have the capacity to respond to an ‘unknown unknown’, that is an event that could not have been predicted (Bates et al., 2001).
At the moment it seems safe to say that there is excessive reliance in healthcare on human memory and other fallible processes; computers, memory and decision aids of all kinds are grossly underused. The boundaries of the human machine interface will change over time, as we develop more powerful and sophisticated systems and accept that clinical expertise, essential though it is, does not necessarily bring reliability and consistency to routine operations. In some areas however, there have already been considerable advances; a notable example is the use of computerized systems in the process of medication administration.
Using information technology to reduce medication errors
Medication errors arise from a variety of causes. Almost half result, in some degree, from clinicians lacking information about the patient or the drug. This may be because they do not know the information themselves, because test results are missing or because other patient or drug specific information is not available. Other common problems are that handwritten orders are illegible, do not contain all necessary information, are transcribed incorrectly or contain errors of calculation (Bates, 2000). Several medication technology systems have been addressing these and other problems, operating at various stages of the medication and delivery process (Figure 13.1). They show great promise but, as David Bates warns, are not a panacea:
Information technologies …. may make some things better and others worse; the net effect is not entirely predictable, and it is vital to study the impact of these technologies. They have their greatest impact in organizing and making available information, in identifying links between pieces of information, and in doing boring repetitive tasks, including checks for problems. The best medication processes will thus not replace people but will harness the strengths of information technology and allow people to do the things best done by people, such as making complex decisions and communicating with each other.
(BATES, 2000)
The system that has probably had the largest impact on medication error is computerized physician order entry (CPOE), in which medication orders are written online. This improves orders in several ways. First, they are structured, so they must include a drug, dose and frequency; the computer, unlike a person, can refuse to accept any order without this information. They are always legible, and the clinician making the order can always be identified if there is a need to check back. Finally, all orders can be routinely and automatically checked for allergies, drug interactions, excessively high or low doses and whether the dosage is appropriate for the patient’s liver and kidney function. Clinical staff may fear that these advantages may be offset by the time lost in typing rather than writing orders. However, Hollingworth et al. (2007) found that increases were only marginal (12 seconds per order) and there was little disruption to workflow. We should also note that the overall effect on the system, as opposed to the individual prescriber, could well be greater efficiency because fewer orders have to be corrected and fewer adverse drug events occur.
Bates et al. (1998) showed that the introduction of a computerized order entry system resulted in a 55% reduction in medication errors. This system provided clinicians with information about drugs, including appropriate constraints on choices (dose, route, frequency) and assistance with calculations and monitoring. With the addition of higher levels of decision support, in the form of more comprehensive checking for allergies and drug interactions, there was an 83% reduction. Other studies have shown, for instance, improvement of prescribing of anticoagulants, heparin and anti-infective agents and reductions in inappropriate doses and frequency of drugs given to patients with renal insufficiency (Kaushal, Shojania and Bates, 2003). Evidence of the value of CPOE continues to accumulate. In a recent meta-analysis of complex prescribing in vulnerable patients, Floor van Rosse et al. (2009) reviewed 12 studies in paediatric and adult intensive care settings and found that CPOE reduced medication errors but that the impact on clinical outcomes remained equivocal. In a review of CPOE, Kaushal, Shojania and Bates (2003) caution that while these systems show great promise, most studies have examined ‘home-grown’ systems, and have only considered small numbers of patients in specific settings. Much more research is needed to compare different applications, identify key components, examine factors relating to acceptance and uptake and anticipate and monitor the problems that such systems may induce.
Another critical safety issue is that there can be a discrepancy between the drugs that patients are meant to be receiving and those that they are actually taking. Medicines reconciliation refers to the process of producing a definitive list of the drugs the patient should be taking and then checking them against the drugs they are actually taking; as you might imagine, points of transition, such as discharge from hospital, are particularly vulnerable to errors of this kind. Schnipper et al. (2009) looked for discrepancies between preadmission medication, medication during admission and medication at discharge. They found an average of 1.4 potential adverse drug events per admission – not very good odds for the patient. This rate was reduced by a third after the introduction of a Web-based computerized medicines reconciliation system, which enabled clinical staff to view and compare medication information from ambulatory (out of hospital) settings with hospital medication. The team also clarified responsibility for medicines reconciliation at different time points, reduced multiple history taking and used cross-checking between staff to increase compliance with the new systems, so there was much more to this intervention than simply the technology.
Looking further ahead, it is possible to envisage the use of many other technologies in the process of medication delivery. Most of these are in the early stages of development, are relatively untested and sometimes delayed by external constraints. Bar coding for instance, widely used in supermarkets, could be enormously useful but cannot be implemented until drug manufacturers have agreed common standards (Bates, 2000). Considerable advances have been made however, in the reliability and efficiency of blood sampling and blood transfusion (Box 13.3).
BOX 13.3 Bar coding and blood transfusion
The transfusion process is long, complex and laborious. Clinical staff are vulnerable to fatigue, distraction and error. The single most important factor in blood transfusion incidents is mis-identification of the patient. Observations showed that staff were frequently distracted whilst checking blood, by having to answer the phone or respond to questions from colleagues. The programme of improvement evolved in four distinct stages:
Blood sample collection and the pre-transfusion bedside check
The first stage addressed two bedside processes previously: blood sample collection for compatibility testing and pre-transfusion checking. A handheld bar code device was introduced, which checked all stages of the process. For instance, staff had to scan the bar coded patient identity tag before proceeding to the next stage. The results of the implementation of the electronic transfusion process were dramatic, reducing the number of process steps and bringing sustained and significant improvements in blood sample collection and pre-transfusion checking. For example, correct verbal identification of patients rose from 11.8 to 100%.
Bar coding the transfusion process
Baseline observations revealed high error rates at almost every step of the transfusion process. Bar coding checks to the transfusion process brought significant improvements. These included an increase from 8 to 100% in checking that the pack was in date and the blood group and unit number on the blood pack matched the compatibility label. Similar significant improvements were found in blood sample collection, the collection of blood from blood refrigerators, and the documentation of transfusion; the time taken to collect a blood unit reduced from an average of 3 minutes to 1 minute per unit.
Electronic remote blood issue
The issue of red cell units was traditionally carried out within the blood transfusion laboratories. Electronic remote blood issue (ERBI) allowed blood to be released to specific patients at blood refrigerators in wards, theatres and other sites. The results showed that ERBI reduced the time to make blood available for surgical patients and improved the efficiency of hospital transfusion. Before it took 24 minutes to get blood to the patient; afterwards 59 seconds. Unused requests for blood reduced significantly and the process significantly reduced the workload of both blood transfusion laboratory and clinical staff.
Implementation of the electronic transfusion process in three acute hospitals
The electronic transfusion processes were implemented across three acute hospitals, with 1500 inpatient beds between them. The implementation was planned in 10 phases, involving 6 or more clinical areas per phase each of 4 weeks duration. Careful advance planning made it feasible to provide the necessary infrastructure in stages (e.g. wristband printers, handhelds) and to provide intensive training to small groups of staff. The task of training all the staff was huge, involving 1300 doctors, 3200 nurses, as well as phlebotomists and porters. At the end of the first year of the implementation stage, the electronic process was being used for taking 88% of samples for the blood transfusion laboratory and for administering 83% of transfusions, later rising to 95% of both samples and transfusion. Reduction of red cell usage and reduction in the rate of sample rejection have produced significant savings.
(TRANSFUSION, MURPHY, M. F., STAVES, J., DAVIES, A., FRASER, E., PARKER, R., CRIPPS, B., KAY, J., & VINCENT, C. “HOW DO WE APPROACH A MAJOR CHANGE PROGRAM USING THE EXAMPLE OF THE DEVELOPMENT, EVALUATION AND IMPLEMENTATION OF AN ELECTRONIC TRANSFUSION MANAGEMENT SYSTEM”. 49, NO. 5, 829–837, 2009. REPRODUCED WITH PERMISSION FROM WILEY-BLACKWELL)
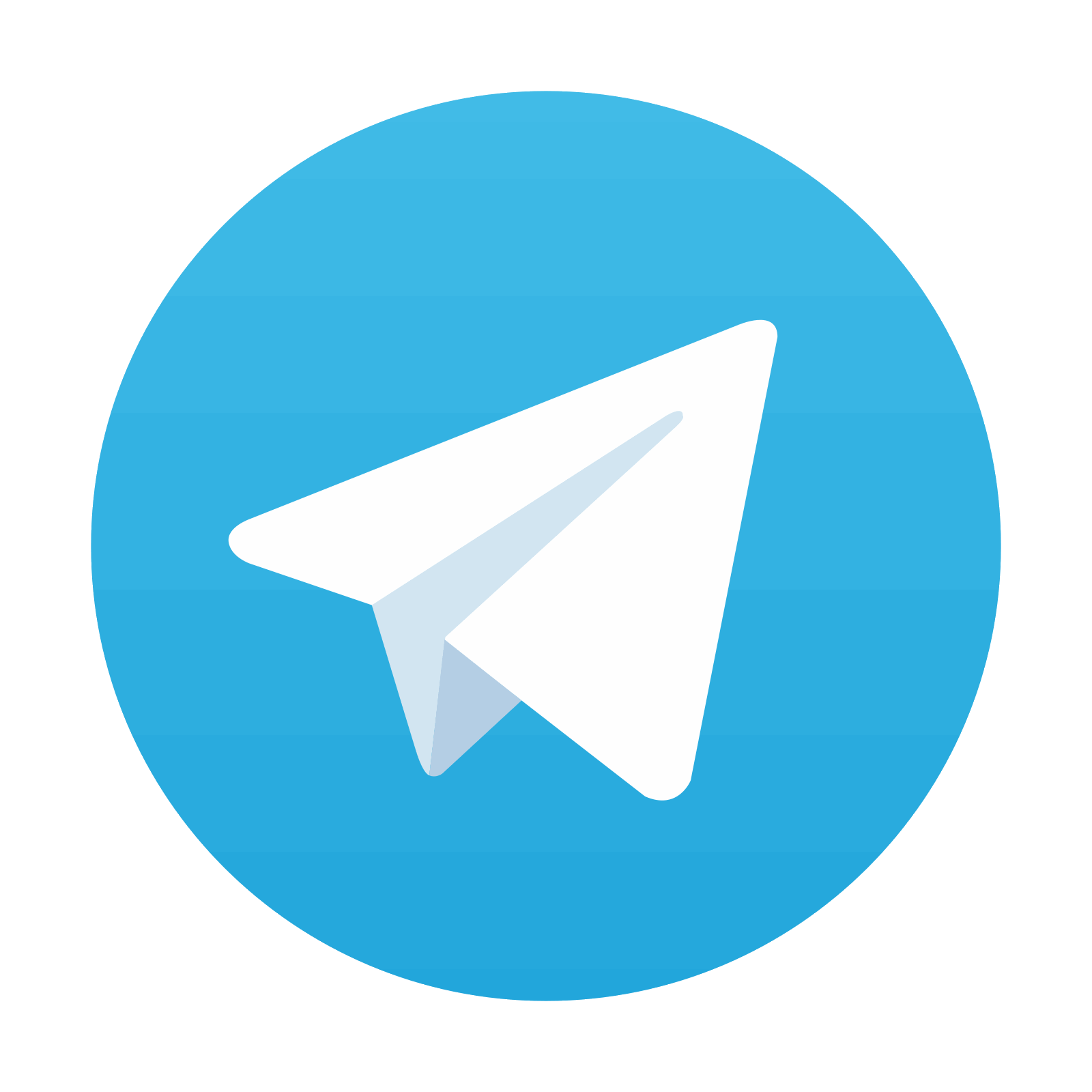
Stay updated, free articles. Join our Telegram channel

Full access? Get Clinical Tree
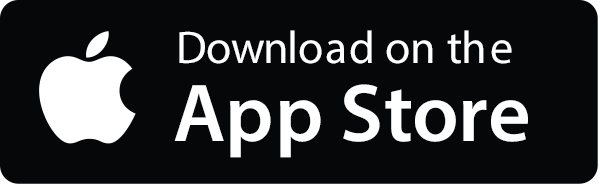
