Introduction
Surgeons have been at the forefront of integrating robotic systems into routine clinical practice. Radical prostatectomy is now commonly performed using a surgical robot in the majority of large centers in developed nations. Surgeons continue to push the boundaries in integrating new technologies in their clinical practice and training to improve outcomes, safety, and quality in surgery. In this chapter the major new technologies that will drive further improvements in robotic surgery will be discussed.
Image guided robotic surgery
One of the major benefits of robotic systems, such as the da Vinci, is the improved visualization provided by the high definition three-dimensional (3D) camera ( Fig. 11.1 ). Indeed, a key area of development in the newer generations of the da Vinci system has been the optics of the endoscope, providing higher quality images and greater magnification. Advances in optics have been especially important for current robotic systems given the absence of haptic feedback and the need for the surgeon to rely on visual feedback to judge force and tension. Alongside ongoing improvements in the quality of the camera image and the size of the endoscope, image guided surgery (IGS) offers the opportunity to further drive improvements by combining preoperative imaging with the intraoperative endoscopic video. The aim is to provide further information to the surgeon, such as allowing them to “see” hidden internal structures. This overlay of images onto the operative field is known as augmented reality (AR). Image guidance was first used in neurosurgery to plan open procedures. Computed tomography (CT) and magnetic resonance imaging (MRI) images were used to create a 3D model of the patient to help surgical planning. The same models were also used to track instruments in real time during the procedure to avoid injury to critical structures.

The principle for all forms of IGS is to combine intraoperative images, usually from the endoscopic camera with other imaging modalities, providing the surgeon with maximal information. Additional imaging may be preoperative, cross-sectional modalities: commonly MRI; CT; or ultrasound (US) or intraoperative images, for example, near-infrared fluorescence (NIRF—e.g., the Firefly system; see below).
In order to allow construction of a 3D model, relevant images or parts of the images known as data segments must be identified. To date, this is most frequently performed manually which is very labor intensive. The additional images require careful alignment with the patient or live operative images through a process known as registration. Hereby, specific points are matched on the imaging and patient or intraoperative images. Commonly, certain anatomic landmarks are used, such as the tragus of the ear.
Alternatively, specific fiducial markers can be attached to the patient to aid registration. In neurosurgery, fiducial markers are commonly used in conjunction with stereotactic frames attached to the skull. Importantly, registration is classified as either rigid or nonrigid. In rigid systems, the subject does not change in shape or relative position, greatly simplifying registration. Orthopedic surgery and neurosurgery often involve rigid registrations given the fixed nature of the bony skeleton or the relative relationship between skull and brain.
In contrast, nonrigid systems, in which structures are liable to move and deform, are a much greater challenge. Registration models require more complex overlay of the images, which often needs to be performed manually. This introduces the further potential for human error. The last important component of IGS is the user interface. Information needs to be displayed clearly to the surgeon in a way that is not distracting. In robotic surgery, this is often provided as images superimposed onto the laparoscopic video feed. At the most basic level, preoperative images may be displayed on the surgeons’ display. Tile Pro technology makes this possible on the da Vinci.
IGS has been successfully applied to several areas of robotic surgery. NIRF has been used by a number of specialties. It offers enhanced anatomic views of the surgical field and helps to identify critical structures. The Firefly system was developed by Intuitive for use with the da Vinci Si and Xi systems. It uses a water-soluble dye, indocyanine green (ICG), that is detected by the NIRF camera.
ICG has the advantage of remaining largely within the vascular compartment and may be used for identification of both vessels and areas of vascular perfusion within soft tissues. The NIRF camera detects ICG by directing an 805 nm laser at the target anatomy. This causes emission of a photon of light with a wavelength of 830 nm from the ICG dye, which is detected by the camera.
The Firefly system has been used in a wide variety of robotic surgeries, including both benign and oncologic procedures. Robot assisted partial nephrectomy is one of the commonest procedures in which Firefly is used. ICG is used to identify the precise vascular anatomy and aid selective arterial clamping instead of main renal artery clamping.
In one study, ICG allowed selective clamping to be performed in 80% of patients. Other common uses of ICG/NIRF include identifying sentinel lymph nodes during extended pelvic lymph node dissection and identifying tumors during adrenal surgery. However, despite the range of applications, the level of evidence remains low, and the actual benefit to the patient and surgeon is yet to be determined.
As described above, the use of AR to enable the overlay of preoperative and intraoperative imaging is far more complex. Various applications have been trialed in robotic surgery. One of the first image guidance systems was developed by Thompson et al. in 2013 for robotic radical prostatectomy.
Preoperative MRI images were successfully overlaid onto the laparoscopic video images and used during 13 live surgeries. However, early models, such as that described by Thompson et al., used rigid 3D models that could not match the tissue deformation. Porpiglia et al. were able to develop the technology further by enabling deformation of the 3D MRI model to match the prostate deformation during surgery. The models were further used to identify areas of extracapsular extension (ECE).
A trial was conducted in which areas identified intraoperatively using the 3D MRI models were analysed histologically. One hundred percent of identified areas contained ECE in comparison to only 41% without use of the 3D MRI model. However, this technology remains in its infancy and further developments in nonrigid registration and automatic organ tracking will allow far greater clinical applications.
3D printing in robotic surgery
Alongside the use of AR to project 3D reconstructions onto the surgeon’s display, 3D printing has been increasingly incorporated into surgical practice. Also known as additive manufacturing, 3D printing was first developed in the automotive, aerospace, and architectural industries before being applied to the medical field. After segmentation of a radiological image, a 3D digital model is created before being printed using various methods. 3D printed models have been used in a variety of applications in surgery. The ability of models to demonstrate complex anatomy has been used in training for various procedures, such as partial nephrectomy and PCNL (see Chapter 8 for further details on the use of 3D printing for robotic surgical training), as well as patient education to understand their pathology. 3D models have also been directly applied to clinical practice, with models used to plan complex renal and prostate surgery. In particular for prostate surgery, translucent resin models were used to display exact location of the tumor within the prostate in an IDEAL phase 2a study that recruited 10 patients with T3 cancer. The model was used by the surgeon to plan nerve sparing approaches tailored to the tumor location ( Fig. 11.2 ). Interestingly, the models were also used to educate and obtain consent from the patients.

A major limitation of 3D printing has been the cost of producing models. While low-cost printers are becoming more widely available, the quality of models is also limited, and printers are often restricted to a single color. More recently, it has been shown that fused deposition modeling (FDM) offers low-cost but high-quality 3D models. FDM works by extruding a heated thermoplastic filament onto a platform in carefully programmed layers. While older models could only offer a single filament and hence single color, multifilament FDM printers can produce high-quality multicolored models shown to be equivalent to more expensive printing techniques when used to produce urological models.
Telecommunications and advanced robotic tools
As discussed in preceding chapters, major advances have been made in the field of surgical robotics. This process continues, resulting in ever more refined and powerful tools for the surgeon. In a number of key areas, there is potential for greater exploration. The lack of haptic feedback and the sense of touch is one of the largest drawbacks of current robotic systems. While improved optics can, to some extent, ameliorate this, it remains a major disadvantage.
Similarly, an early inspiration for robotic surgery was telemedicine and the opportunity for true remote surgery. One of the first clinical trials of telemedicine involving percutaneous access to the kidney was published as early as 2005. Yet since then, routine use of telemedicine in surgery remains limited to a select few cases. In both these areas, modern telecommunications offers solutions to the technical challenges.
The next revolution in telecommunications, the Internet of Things, is already underway with the growing connection and information sharing between huge numbers of objects. Underpinned by zero delay transmission, the Internet of Things offers huge potential to robotic surgery. Ultrasensitive sensors connected using extremely low latency 5G networks may be incorporated into robotic systems, providing the surgeon a fully immersive experience with high quality audio, visual, and haptic feedback. Low latency communications alongside artificial intelligence (AI) augmentation to help predict movements will enable surgeons to operate effectively with zero delay at great distances and offer the routine implementation of telesurgery. One of the first clinical trials of 5G surgery was conducted in China in 2019 when 5G technology allowed a surgeon in Hainan to operate on a patient 1400 miles away in Beijing.
Artificial intelligence in robotic surgery
The importance of integrating data in modern health care practice is increasingly being recognized. As described above, image guidance technologies are being developed to enable a surgeon to use preoperative imaging and other data effectively during surgery. A key driver for this is AI. The ability of machine learning (ML) algorithms used in AI to sort and classify data effectively and without ongoing human input enables the processing of large data sets. The transition from rigid to nonrigid models in AR offers great potential to increase the accuracy of the overlay and thereby the potential benefits to clinical practice.
The key to addressing the complexity of segmentation and registration are ML algorithms to automate the process. Furthermore, such an automated approach also offers the possibility to compensate for organ movement and interference by instruments. Porpiglia et al. have shown that it is possible to do this during robotic prostatectomy and robotic partial nephrectomy, even while continually adjusting the image to the motion of the organ during surgery.
Another important area of development is the automatic recognition of tissues and instruments with potential benefits across a number of areas of robotic surgery. Firstly, this will enable further improvements in the modeling of AR and registration of preoperative data sets. ML supported recognition software will also play an important role in development of automatic performance metrics.
As discussed in Chapter 10 , ML derived assessment of technical performance is important not only for training but also for quality assurance and even prediction of surgical outcomes and risk stratification. Not only could this be used to improve the clinical management of patients, but it will also aid logistical planning. ML has also been applied more directly, planning service delivery in robotic surgery. ML models have been developed to predict operative time based on patient factors, procedure, and even assistant expertise.
Intraoperative data collection will also increase in the future and likely feed into such autonomous systems for inferring the course of operations and aim to mitigate complications. For example, various data sources in the operating room currently working in isolation, such as anesthesia monitors and suction equipment, can be analyzed collectively.
More varied applications of AI in robotic surgery are also being developed. The possibility of intraoperative surgical margin status is being explored using ML analysis of shortwave Raman spectroscopy. Benchtop studies have shown the system to be able to differentiate renal cell carcinoma from benign tissues with a high degree of accuracy.
Autonomous surgery
The first autonomous surgical robots were developed over 30 years ago; however, until recently there has been very little further development. This contrasts sharply with industry, where autonomous functions have become commonplace. Autonomy can be defined as the “ability to perform intended tasks … without human intervention,” according to the International Organization for Standardization. It should be considered as a spectrum rather than a binary state. Yang et al. devised a classification system for medical robotics from 0 (no autonomy) to 5 (full autonomy— Table 11.1 ). Of note, the da Vinci system is classified as having level 1 autonomy since assistance is provided for tremor reduction.
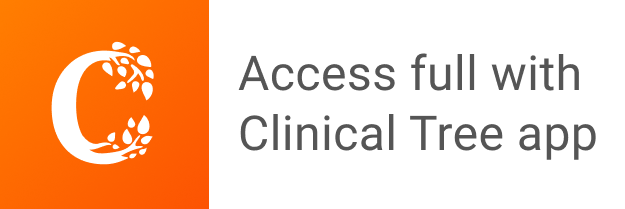