Introduction
In the past decade, mounting evidence suggests that surgical performance affects postoperative outcomes, as superior quality of surgery reduces the risk of complications, shortens the length of hospital stay, and results in better long-term functional and oncological outcomes. Thus surgical training is the foundation for high-quality surgery. The recent transition in surgical training from Halstead’s traditional apprenticeship model, famously known as “see one, do one, teach one,” to a more standardized and quantifiable training method has led to a rapidly growing interest in surgical assessment and performance measures.
Competency refers to the bare minimum performance level required for a surgeon to operate independently, whereas proficiency implies some level of performance above the bare minimum. Mastery refers to a rare, exceptional performance that is the highest level of achievement within a certain domain. This chapter focuses on performance measures at a proficiency level.
Measures of performance
Several surrogates of performance have been used in the past, with the previous “gold standard” being surgical outcomes (e.g., complication rates, readmission rates, oncologic outcomes, functional outcomes). Although assessing outcomes seems reasonable and clinically relevant, it is limited by several shortcomings. Due to its retrospective nature, poor surgical performance can be identified only after an adverse event occurs rather than before or as it occurs. In addition, the heterogeneity of patient and disease characteristics makes comparisons between surgeons or institutions difficult. For example, high-risk patients are often transferred from community hospitals to tertiary centers for treatment, which may make simple surgical outcome comparisons between community hospitals and tertiary centers unfair. Another popular method of estimating surgical skills was based on prior case load; however, caseload is not always necessarily accurate as surgeons’ learning curves vary widely. Thus better surgical assessment methods are needed to accurately and objectively gauge surgeon performance.
To meet this need, multiple assessment methods have been developed and can be broadly classified into three main categories: manual assessment, computer-generated metrics, and surgeon biometrics ( Fig. 10.1 ). Burgeoning studies have shown these methods to be capable of differentiating expertise, demonstrating surgeons’ learning curves, and predicting surgical outcomes, thereby showing great potential for measuring robotic surgery performance for training, certification, and accreditation purposes.

Artificial intelligence in robotic surgery and education
Artificial intelligence (AI) has gained lots of interest in robotic surgery due to the plethora of data available from just a single surgery. In 2019, approximately 1,229,000 da Vinci robotic-assisted surgeries were done globally, compared with just 753,000 in 2016. , With multiple new robotic systems on the horizon, robotic surgery will likely grow even faster than before. The blossom of robotic surgery provides a unique and natural venue for incorporating AI, especially into surgical education and proficiency measurement. The combination of intraoperative instrument motion-tracking (kinematics), video recording, and AI has created an unprecedented opportunity to objectively and instantaneously assess a surgeon’s performance and provide customized feedback to train the next generation of robotic surgeons.
In this chapter, we will first review current proficiency measures used to assess surgeon proficiency in robotic surgery, then elaborate on the current applications of AI in robotic surgical education, and finally discuss future directions.
Manual assessment of robotic surgical education
Manual assessment requires expert evaluators to watch either a live or previously recorded surgical performance and then manually rate different surgical skills, with each domain anchored by objective grading criteria. Manual assessment can be broadly divided into two main categories: global skills assessments and procedure-specific assessments ( Table 10.1 ). As implied by the names, global skills assessments evaluate only general robotic surgical skills and can be applied across many procedures, whereas procedure-specific assessments evaluate surgical skills in a specific procedure or procedural step. Manual assessments have demonstrated the ability to differentiate surgeons based on previously used surrogates of skill and proficiency (i.e., prior surgeon case load) and are now commonly used as the standard with which newer assessment tools are compared. A summary of manual assessments is demonstrated in Table 10.1 .
Assessment Method | Brief Description | Skills Domains Assessed |
---|---|---|
Global Skills Assessments | ||
OSATS | Evaluates technical proficiency in open surgery |
|
GOALS | Expanded on OSATS to evaluate technical proficiency in laparoscopic surgery |
|
GEARS | First robotic surgery-specific assessment tool; currently the most widely used to measure robotic surgery proficiency |
|
R-OSATS | Combines elements of OSATS and GOALS to assess proficiency across four standardized dry lab tasks |
|
ARCS | Developed by Intuitive Surgical to assess distinct console skills not assessed by GEARS |
|
Procedure-Specific Assessments | ||
RACE | Developed to assess specific technical skills in vesicourethral anastomosis in RARP |
|
PACE | Developed to assess RARP-specific skills in standardized steps | 5-point Likert scale with performance description for each domain of the seven standardized steps |
CASE | Developed to assess RARC-specific skills in standardized steps | 5-point Likert scale with performance description for each domain of the eight standardized steps |
RARP Assessment Score | Developed to assess technical skill in critical steps identified by HFMEA analysis | Standardized steps divided into substeps to identify hazards and create a 17-stage system (17 processes and 41 subprocesses) |
Global skills assessments
Various global skills assessments have been created or adapted for robotic surgical performance evaluation over the years. The three most commonly used are Objective Structured Assessment of Technical Skill (OSATS), Global Operative Assessment of Laparoscopic Skills (GOALS), and Global Evaluation and Assessment of Robotic Skills (GEARS).
Objective structured assessment of technical skill
OSATS was first introduced in 1996 as one of the first surgical technical skills assessment tools and was originally designed for laboratory use. , It has since been used across many settings, including simulated, laboratory, and live operating environments, and for many surgical subspecialties, including urology, to evaluate robotic surgical performance.
OSATS has been used to accurately differentiate between novice and expert surgeons and to capture improvement in surgical skills after training programs. , OSATS has also shown potential correlations with important surgical outcomes, as OSATS scores correlated with anastomosis patency in live rat models undergoing robot-assisted microvascular surgery. However, correlations to surgical outcomes have been primarily in the laboratory or simulator setting, and further research must be done to identify correlations after live robotic surgery.
Although OSATS has been used and adapted to evaluate robotic surgery proficiency, it was originally designed for open surgery in a laboratory setting. As a result, it does not encompass all of the distinct skills required for robotic surgery, such as depth perception, bimanual dexterity, camera control, and master manipulator workspace.
Global operative assessment of laparoscopic skills
GOALS was developed in 2005 by Vassilou et al. to specifically evaluate laparoscopic surgical skills and has since been adapted for robotic surgical assessment in the live surgery and laboratory setting. Because GOALS was created for minimally invasive surgery, it evaluates robotic surgery skills better than OSATS; however, it still lacks certain skills unique to robotic surgery such as camera control and master manipulator workspace. , Like OSATS, GOALS has been used to reliably assess surgeon performance and distinguish expert and trainee performance, including in specific procedural steps such as a ureteral anastomosis.
Global evaluative assessment of robotic skills
Although GOALS was an improvement from OSATS in assessing robotic surgeon performance, it still lacks some of the skills specific for robotic surgery. Thus GEARS was developed by Goh et al. in 2012 as an expansion of GOALS and is the first robotic surgery-specific assessment tool. GEARS expanded upon GOALS to include many of the robotic surgery-specific skills that were missing in previous assessment tools such as camera control, robotic control, and operator workspace. , Because of its specificity for robotic surgery, yet generic and flexible grading scheme, GEARS has become the most widely used and extensively studied manual assessment tool to evaluate robotic surgery performance, especially in urology.
Like the previously described global assessment tools, GEARS can accurately distinguish surgeons by skill level based on previously used surrogates, such as prior caseloads, and consistently determine rank order of robotic surgeons, particularly those of lower skill level. , Unlike the previously described assessments, GEARS is the only global skills assessment tool that has been correlated to clinical outcomes after live surgery. In studying GEARS in robot-assisted radical prostatectomy (RARP), Goldenberg et al. found that GEARS scores of the overall cases as well as in specific steps (bladder neck dissection and vesicourethral anastomosis) were independent predictors of 3-month continence recovery. , GEARS scores have also been correlated with urethral catheter replacement rates and readmission rates after RARP.
Other global skills assessments
Although GEARS is specific for robotic surgery and widely used, it still does not encompass every skill required for robotic surgery. Thus several other robotic surgery-specific assessment tools have been proposed to address these missing domains. Siddiqui et al. proposed an adapted system by combining elements from OSATS and GOALS to create an assessment tool specifically for robotic surgery, termed Robotic-Objective Structured Assessment of Technical Skills (R-OSATS). , R-OSATS has demonstrated the ability to differentiate between various expertise levels, including faculty, fellows, and junior and senior residents, and to determine a minimum cutoff score for competence. ,
Recently, Intuitive Surgical Inc. (Sunnyvale, CA) developed the Assessment of Robotic Console Skills (ARCS) to assess robotic console manipulation skills, including optimization of field of view and workspace and basic energy pedal skills, different skills than those measured by GEARS. In their validation study, ARCS accurately distinguished surgeons based on previous caseloads, making it another promising assessment tool in the future.
Procedure-specific assessments
Assessing surgeon performance in a specific procedure is important to ensure quality procedures and requires more than just global skills assessments. Thus procedure-specific assessments have been developed to evaluate a surgeon’s knowledge of and surgical skill in a procedure or a specific procedural step. These assessment tools often deconstruct the overall surgery into standardized steps to evaluate performance and provide detailed feedback. Procedure-specific assessment tools have been primarily developed through two processes: the Delphi process and the Healthcare Failure Mode Effect Analysis (HFMEA).
The delphi process
The Delphi process is a forecasting process method used to reach a group consensus through multiple rounds of questionnaires among a panel of experts. Every round, responses are recorded and shared with the rest of the panel and experts will adjust their answers based on the rest of the panel’s responses. This process occurs for multiple rounds until a group consensus is reached. Several procedure-specific assessments have been developed through the Delphi method.
The Robotic Anastomosis Competency Evaluation (RACE) tool assesses specific skills across five domains relevant to the vesicourethral anastomosis in an RARP. In its validation study, RACE scores distinguished trainees of different experience levels (beginner, novice, and expert) with moderate interrater reliability.
The Prostatectomy Assessment and Competency Evaluation (PACE) assesses performance across seven domains of skills for each of the standardized RARP steps. In its validation study, PACE demonstrated varying levels of reliability in differentiating expertise depending on the step.
The Cystectomy Assessment and Competency Evaluation (CASE) assesses surgical performance during critical steps of a robot-assisted radical cystectomy (RARC) with moderate interrater reliability. In its validation study, experts outperformed trainees in all steps, but significance was not reached.
The Pelvic Lymphadenectomy Appropriateness and Completion Evaluation (PLACE) was developed to assess the completeness of the pelvic lymph node dissection after RARC.
Healthcare failure mode effect analysis
HFMEA is a human risk analysis used to identify critical steps in a procedure associated with important clinical outcomes. By identifying these critical steps, assessment tools developed through HFMEA can potentially provide technical skills assessments of clinically relevant skills or steps. The RARP Assessment Score was developed by an international group using HFMEA. They identified critical steps to focus RARP training and then used the RARP Assessment Score to demonstrate a learning curve necessary for competency in technical skills. A similar tool was created through HFMEA for robot-assisted partial nephrectomy. , Currently, no studies have assessed the correlation between assessments developed through HFMEA and surgical outcomes.
Limitations of manual assessments
Although manual assessments require nothing more than a video recorder, they are limited by the tremendous amount of time required of expert evaluators to manually rate each video as well as the potential subjective bias of human evaluators. Although manual assessment tools are anchored by objective criteria, certain domains and scores are open for interpretation, making standardization among evaluators challenging at times.
Crowd-sourced evaluation is a promising solution to these limitations. Crowd-sourced evaluation involves using the public, regardless of medical training or background, to evaluate surgical skill and technique. Studies have shown that crowd-sourced results can achieve similar results and consistency as expert evaluator results. Crowd-Sourced Assessment of Technical Skills (C-SATS) is an online crowd-sourcing community that has shown promising results across various studies.
Limited by the major time commitment required to manually rate surgeon performance, it is impossible for training surgeons to receive constant or regular feedback if only expert evaluators are involved. However, through crowdsourced evaluations, trainees can receive detailed feedback more frequently, perhaps even after every surgical performance, allowing them to continually focus their training on specific skills and objectively gauge their learning curve. Crowdsourcing is again limited by subjective biases inherent to human evaluators, and further research must be done to determine exactly how crowdsourcing should be used in surgical assessment.
Computer-generated metrics
During robotic surgery, instrument kinematic data and system events data can be recorded automatically, thereby providing far more information than was ever possible in open or laparoscopic surgery. Various studies have demonstrated that these metrics can be used to distinguish expertise, measure proficiency, and predict surgical outcomes.
Instrument kinematic metrics
Instrument kinematic metrics generally measure instrument motion such as traveling distance, moving velocity, acceleration/deceleration, EndoWrist ️ articulation, and jerk (a derivative of acceleration with respect to time). , As early as 2006, Narazaki et al. showed that experts required less instrument traveling distance and demonstrated better bimanual dexterity when performing dry laboratory tasks.
In the following decade, new kinematic metrics (e.g., velocity, EndoWrist articulation) were validated for measuring proficiency, mostly in the dry laboratory setting. In 2018, Hung and colleagues validated these long-established metrics for the live robotic surgery setting, showing that expert surgeons perform significantly better during RARP than novices in nearly all aforementioned metrics. The same group further showed that these metrics could predict surgical quality, short-term surgical outcomes (i.e., length of hospital stay), and long-term functional recovery (i.e., continence recovery) after RARP. ,
However, one drawback of instrument kinematic metrics is that metrics are sometimes dependent on patient conditions. For example, Chen et al. showed that bony pelvic dimensions significantly impacted kinematic metrics during RARP. Similarly, Ghodoussipour et al. found that R.E.N.A.L. scores (a measure of difficulty for robot-assisted partial nephrectomy) impacted intraoperative metrics.
System event metrics
System event metrics measure robot activity other than instrument motion, such as camera movement, master clutch use, third instrument swap, and energy application, and have been validated for differentiating surgeons based on experience. , Unlike instrument kinematic metrics, system event metrics provide potentially actionable feedback regarding usage of camera, third arm, and instrument energy application (i.e., optimize camera view more frequently).
Grip force
Grip force is another instrument-based metric that has been used to measure proficiency. Studies involving grip force have been carried out only in the laboratory setting because the current da Vinci robotic system does not contain instrument force sensors. Results have shown that experienced robotic surgeons tend to apply less instrument force than novices when performing dry laboratory exercises or simulation tasks. In addition, dry laboratory training courses have been shown to improve instrument grip force in trainees. A new robotic system, Senhance, has incorporated force sensors into the system to potentially provide haptic feedback, raising much interest in validating this metric for the clinical setting.
Surgeon biometrics
Surgeon-derived biometrics provide another approach to assessing surgeon proficiency. In contrast to the computer-generated metrics that focus on external actions, biometrics focus on the internal responses of surgeons.
Eye tracking
Eye tracking has been widely used in sports and aviation for skills assessment, and recent innovations have allowed for wearable eye trackers to record eye movement and pupillary response during surgery in order to provide user-friendly and understandable feedback. Eye tracking has also been used to assess a surgeon’s visual recognition skills (e.g., identification of anatomical landmarks) and cognitive workload.
While operating, the surgeon’s eyes constantly make rapid voluntary movements (i.e., saccades) to scan ambient regions and then fixate on certain points to extract information (i.e., fixation periods). The sequential periods of rapid saccades and steady fixations define a surgeon’s gaze pattern. By overlaying a surgeon’s gaze pattern onto corresponding surgical video recordings, studies have found that experts spend more time fixating on target structures (i.e., target-locking gaze strategy) instead of searching or tracking instruments compared with novices (i.e., gaze-switching strategy). Thus a surgeon’s ratio of attention to target versus ambient structures can be potentially used as a measure of expertise and proficiency.
Apart from analyzing gaze patterns, eye-tracking metrics have also correlated with surgeon expertise or technical scores (e.g., GEARS). For example, the index of cognitive activity, derived from pupil signals representing the cognitive workload, decreases as a surgeon becomes more proficient. , However, like kinematic metrics, these eye-tracking metrics are also impacted by task difficulty. , , A detailed summary of eye metrics used to assess surgeon proficiency is demonstrated in Table 10.2 .
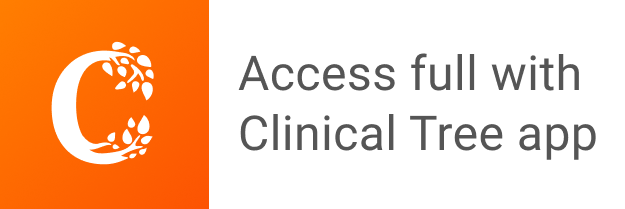