Practical Application of the Principles of Epidemiology to Study Design and Data Analysis
Joseph H. Abramson
Suppose that examinations of 200 workers in a hospital reveal that 24 are carriers of methicillin-resistant Staphylococcus aureus (MRSA). Does this mean a prevalence of 12% in the hospital’s personnel?
A study of adults undergoing mandatory health examinations (1) revealed that MRSA carriage (based on nasal swabs) was about twice as high among nonsmokers (4.3%) as among smokers (2.2%); the difference was statistically significant (p = .019). Does this mean that smoking protects against MRSA carriage?
Suppose this study had not found a significant difference, that is, p > .05, would this mean that smoking has no effect on the prevalence of MRSA carriage?
Suppose that a program to encourage hand washing by personnel is followed by a reduced rate of S. aureus infections among patients. Does this mean that the program reduced the incidence of these infections?
Suppose we are told that a review of the literature has found 16 controlled trials that show that a certain treatment for MRSA is efficacious and 4 that do not (a highly significant difference: p = .007). Can we conclude that the treatment works?
The answer to all five of these questions is “No.”
Why?
Read on.
MAKING SENSE OF DATA
Bias is the bugbear of epidemiologists (2). Bias does not here refer only to preconceived opinions and preference but (as defined by the Dictionary of Epidemiology) to any “error in the conception and design of a study—or in the collection, analysis, interpretation, reporting, publication, or review of data—leading to results that are systematically (as opposed to randomly) different from truth” (3). Its commonest forms, in any kind of study, are information bias, which is caused by shortcomings in the collecting, recording, coding, or processing of data, and selection bias, which is the distortion produced by the manner in which subjects are selected for study or by the loss of subjects who have been selected. In an analytical study, bias may also be caused by confounding.
This chapter deals with ways of minimizing or dealing with biases and uncertainties, both when planning and conducting a study and when handling its results, in order to make the study as valid as possible, with reference both to the study’s soundness (its internal validity) and, when relevant, to its generalizability or applicability in other contexts (its external validity).
The focus is on epidemiological studies, that is, on studies of the occurrence, distribution, and determinants of health-related states or events in specified populations. This is a rubric that embraces all studies in the field of healthcare epidemiology and infection control, except maybe some laboratory studies.
Separate consideration will be given to epidemiological studies of various types, namely, descriptive studies and analytical observational studies, and (more briefly) ecological and multilevel studies, program reviews, trials, and meta-analyses. Descriptive studies may be cross-sectional ones that describe a situation at or around a given time (“snapshots”) or longitudinal ones (such as surveillance procedures) that describe changes or events in an ongoing way or during a given period (“motion pictures”). Descriptive studies of disease occurrence may be termed prevalence studies if they are cross-sectional and incidence studies if they extend over a period. Changes may also be appraised by comparing the findings of repeated cross-sectional studies. Analytical observational studies include analytical crosssectional studies, which examine the associations between variables (e.g., between suspected causal factors and their assumed effects) that exist at or about a given time; cohort studies, which are follow-up studies of people with various degrees of exposure to supposed causal factors; case-control studies, which compare the characteristics and prior experiences of people with and without a given disease or other outcome; and ecological and multilevel studies, which use data about groups or populations as such, unlike other studies, which are based only on data about the individuals in the groups that are studied. Program reviews are observational or analytical studies of the operation and outcome of healthcare procedures or programs, clinical trials and program trials may be seen as epidemiological experiments that test the value of healthcare procedures or programs,
and meta-analyses are critical reviews and syntheses of different studies of the same topic.
and meta-analyses are critical reviews and syntheses of different studies of the same topic.
These types of epidemiological studies are not mutually exclusive. A study may have multiple objectives. It may, for example, be both descriptive and analytical, as in a study of colonization of group B streptococcus in pregnant women and neonates that was not confined to a description of prevalence and susceptibility to antimicrobial agents, but extended to an exploration of the effects (on the colonization rate in the newborn) of possible risk or protective factors, such as prolonged labor or the administration of antibiotics to the mother (4).
For each type of study, we consider the main biases and uncertainties that may arise and briefly enumerate the steps that can be taken when planning the study and when analyzing and interpreting the findings, so as to minimize these biases and uncertainties or permit account to be taken of their effects.
Although a number of statistical procedures are mentioned and numerical examples based on them are cited, these procedures are not explained. It is assumed that readers either have statistical consultants or collaborators, or themselves have a sufficient grounding in statistical principles to be able to make intelligent use of statistical software. A number of multipurpose commercial programs (such as those listed on Chapter 15, pp. 216-217) are available, but they have to be learned and may be difficult for an unversed nonstatistician to use. The Internet offers many simple interactive programs (“Web pages that perform statistical calculations”) (5), and a plethora of shareware and freeware statistical programs is available for downloading (6). The user-friendly WinPepi programs (7) for epidemiologists, for example—which can be downloaded free from www.brixtonhealth.com with their extensive and fully referenced manuals—can perform all the statistical procedures mentioned in this chapter (except Cox regression analysis and multilevel analyses). WinPepi was used to provide all the numerical examples cited in the text.
There are numerous sets of publication guidelines for epidemiological studies—witness the title of a recent review (8)—and these checklists can serve as reminders of what kinds of data should be collected and what kinds of analyses should be done. Particularly useful are the STROBE (Strengthening the Reporting of Observational Studies in Epidemiology) (9) and (for randomized trials) CONSORT (Consolidated Standards of Reporting Trials) (10) guidelines.
Before embarking on a study of any kind, ethical matters must of course be considered. Confidentiality should be taken into consideration even if the study is based only on existing medical records, and informed consent should be obtained whenever special test procedures—even questioning—or interventions are required. Approval by an appropriate ethics committee may be needed.
DESCRIPTIVE STUDIES
Information bias
Epidemiological studies do not always have clear purposes. It is not always clear why the study was performed; that is, what it was hoped to achieve by performing it. But every epidemiological study should have clearly defined objectives, that is, an answer to the question “What knowledge is the study planned to yield?” These objectives dictate the variables to be measured, and these variables must be clearly defined.
We have been told, at the outset of this chapter, that a simple descriptive study, whose objective was presumably to measure the prevalence of MRSA carriage, revealed that 24 of 200 hospital workers were carriers of MRSA (1). A number of obvious questions come to mind.
What, for example, is meant by “carriers of MRSA”? First, what is the conceptual definition (the “dictionary definition” of the characteristic that it is hoped to measure)? Carriers of these bacteria with no evidence of acute infection, or all carriers? Persistent carriers only, or transient carriers also? And secondly, how was this concept translated into an operational (working) definition, expressed in terms of the method of examination? From what sites were swabs taken? If from nostrils, one or both? Once only, or repeatedly? What type of swabs? Were the swabs stored or used immediately? Which of the available tests for MRSA was used? Precisely what results, using these methods, were taken as evidence of MRSA carriage? (And, of course, were all the workers examined in the same prescribed manner?)
Once we know the conceptual and (especially) the operational definition of the variable, we can ask how valid the measurement was; that is, how well did it measure what the researcher wanted to measure? The validity of a measure or a method of measurement can be appraised by comparing the findings with a criterion (a reference standard or “gold standard”) that is known or believed to be close to the truth, if such a criterion is available. For a “yes-no” (dichotomous) variable, validity can then be calculated (see Chapter 3, pp. 54-55) and expressed as sensitivity and specificity. The sensitivity of the MRSA measure tells us what proportion of the true carriers (according to the gold standard) are detected by the examination, and its specificity tells us what proportion of noncarriers are correctly classified as noncarriers. The false-positive rate is 100% minus the specificity. It may also be enlightening to calculate the predictive value of the findings—when MRSA is detected by the measure, what is the probability that it is truly present (positive predictive value)? and when it is not detected, what is the probability that it is truly absent (negative predictive value)? But it must be remembered that these calculated predictive values (unlike sensitivity and specificity) are dependent on the prevalence of the condition. For example, if sensitivity and specificity are both 90%, the positive predictive value can be shown to be 79% if the true prevalence is 30 per 100, 55% if the true prevalence of MRSA is 12 per 100, and only 32% if the true prevalence is 5 per 100. If no “gold standard” is available, other methods of appraising validity can be used (11), for example, by checking the results against other (although not necessarily better) measures of the variable (convergent and discriminant validity), against related variables (construct validity), or against subsequent events (predictive validity). Often, reliance can be placed on common sense—that is, a judgment that the measure is obviously valid (face validity). If the validity of a measure is not known, it is sometimes decided
to appraise it in the course of the study (e.g., by using a “gold standard” measure in a subsample) or in a pretest.
to appraise it in the course of the study (e.g., by using a “gold standard” measure in a subsample) or in a pretest.
It may be helpful, although it is not essential, to also know how reliable (i.e., repeatable) the measure is; that is, whether the same result is obtained when the examination is repeated. High reliability does not necessarily mean that the measure is valid (what is more reliable—or less useful— than a broken watch?) But low reliability will always cast doubt on validity. Many measures of reliability are available. In this instance, use would probably be made of the kappa coefficient (see Chapter 3, p. 72) or the apparently preferable AC1 coefficient, which express the proportion of subjects who are classified in the same way each time, after allowing for the effect of chance agreement. If we were appraising a numerical measure, other indices of reliability would be appropriate, such as St Laurent’s gold-standard correlation coefficient (12) and the 95% limits of agreement (13).
The question that was asked at the start of this chapter was “Suppose that examinations of 200 workers in a hospital reveal that 24 are carriers of methicillin-resistant Staphylococcus aureus (MRSA). Does this mean a prevalence of 12% in the hospital’s personnel?” If there is no misclassification, the prevalence is obviously 12% in these workers— that is, in the very unlikely event that the sensitivity and specificity of the measure are both 100%. But if there is misclassification—and there almost always is—the prevalence is unlikely to be 12%. Suppose, for example, that only 5 of the 200 subjects (2.5%) truly have MRSA and that the measure of MRSA has a sensitivity and specificity of 90%. Then it can be expected that 90% of the 5 will be found to have MRSA (4.5 true positives), and so will 10% of the other 195 (19.5 false positives), so that the total number who apparently have MRSA will be 24 (12% of the 200). In other words, a true prevalence of 2.5% will yield an apparent prevalence of 12%. And, conversely, an apparent prevalence of 12% points to a true prevalence of only 2.5%. Taking account of misclassification, the prevalence of MRSA in these 200 workers would thus be only 2.5%. An appropriate computer program, such as WinPepi, can easily do this reverse calculation, if fed the sensitivity, specificity, and apparent prevalence.
Descriptive epidemiological studies are usually concerned with more than one dependent variable and may involve independent variables as well, since they often aim to describe the findings in different subgroups, for example, different age groups or occupational groups, or in patients with different diagnoses. A failure to define appropriate working definitions for any of the variables, sufficiently valid for the purposes of the study, may result in a study flawed by information bias.
Any deficiencies in the collection of data may bias the results. The case-finding procedures used in an outbreak investigation, for example, may be inadequate however clearly a case is defined. Information bias may also be caused by missing data and by deficiencies in the recording or management of data, for example, by errors or omissions in the recording of findings or in the transfer of data to a computer for analysis.
In a longitudinal descriptive study, information bias may be caused by any changes that occur with time in disease definitions, case notification systems, or case-finding methods.
Data collected by observation (e.g., clinical or laboratory examinations) are generally more valid than data collected by interviewing or questioning (except of course in studies of feelings or attitudes). Numerous factors may reduce the validity of data based on questions— faulty memory (recall bias), a tendency to give socially acceptable responses, the interviewer’s attitude, the wording of the question, etc. (see Chapter 5, pp. 87-90). Medical records too are often disappointing as a source of valid data unless they have been planned and maintained as a basis for research; a high proportion of the healthcareassociated infections detected by a surveillance program may not appear in the diagnostic record (14). All records maintained solely for administrative purposes may be problematic with respect to their completeness or accuracy. An examination of the feasibility of appraising the immunization status of hospital workers in England, for example, revealed that only 85% of hospital trusts knew the exact number of staff employed, and only 68% had records of all immunizations (15).
The above considerations apply to all epidemiological studies, not only to descriptive ones—namely, the need for defined study objectives, for clear operational definitions expressed in terms of the methods of study, and (if face validity does not suffice) for assurance of the validity of these methods. Information on the validity of methods may be available from other studies, but this should be handled circumspectly, since sensitivity and specificity may be affected by the characteristics of the sample in which validity was appraised and may by chance be different in different samples.
Selection Bias and Sampling Variation
To return to the MRSA example, we have not been told how the 200 workers were selected. Can the findings be validly applied to “the hospital’s personnel,” or do they apply only to a not necessarily representative group of 200 workers? Were the 200 a random sample, chosen from the total personnel by using random numbers or a computer program that uses an algorithm that makes an as-good-as-random selection? Or, were they an equally representative systematic sample, selected (for example) by taking every fifth person in a list of all personnel? Or, on the other hand, were they a haphazard and possibly unrepresentative sample; for example, were they the more easily persuaded workers encountered in a particular part of the hospital at the time of the study and possibly only junior personnel to boot (because what researcher would want to get up the noses of senior physicians or nurses, and administrators?).
In whatever way the sample was selected—even if it was selected randomly—it would be helpful to be assured that the characteristics of the workers who were studied were in fact similar to those of the total personnel if the latter information is available. Was the sample sufficiently similar in age, sex, occupation, etc. to the population from which it was drawn to allay concerns about selection bias?
To reduce sampling variation, use is often made of stratified random sampling. Representativeness with regard to age and sex, for example, can be enhanced if the sample is made up of separate random samples selected from each age-sex stratum.
A common source of selection bias is the loss of subjects, that is, the loss of members of the selected sample, as a result of refusal, failure to find subjects, mishaps in the laboratory, etc. Were the 200 workers who were studied in the MRSA study the total selected sample, or were they part of a selected sample of (say) 300? If the latter, it would be helpful to know whether and how the workers who were lost differed from those who were included. May the reasons for noninclusion be connected with the variable under study?
Even if the sample in the MRSA study was a representative randomly selected one, we cannot be sure of the 12%. There are a very large number of alternative random samples that might have been chosen, and the findings in different random samples of workers would, by chance (random sampling variation), obviously differ. We cannot be certain that the true prevalence in the total personnel is 12%, just because the prevalence in one representative sample is 12%. The best we can do is to use a computer program to obtain a confidence interval, which we can interpret, with a given level of confidence, as expressing the range within which the prevalence probably falls. In this instance, we can be 95% sure that the prevalence is between 8% and 17%. If a lesser level of confidence satisfies us, the range is narrower—the 90% confidence interval is from 9% to 16%. If we want to be more certain of the result, we can compute the 99% confidence interval, which is wider, namely, from 7% to 19%. The confidence interval depends on the size of the sample; it is wider if the sample is small, and narrower if it is large. If the sample size was only 50, uncertainty would be greater, the 95% confidence interval being from 5% to 23% instead of from 8% to 17%. If the sample size was 1000, the 95% confidence interval would be narrow—from 10% to 14%.
If the sample was randomly selected and we ignore possible misclassification, we can thus conclude with 95% confidence that the prevalence in the hospital’s personnel is between 8% and 17%. But if we assume a sensitivity and specificity of 90% (in which instance the adjusted prevalence is only 2.5%), the 95% confidence interval for prevalence ranges from just above 0% to 9%.
The sample size required in a descriptive study depends on the desired width of the confidence interval—if a more precise result is wanted, a larger sample is required. The basic requirements for the calculation of sample size (or for the computer program that calculates it) are a guess, a wish, and a precaution. If the aim is to measure a proportion or rate, a guess must be made at its expected value; to be on the safe side, a proportion of 0.5, or 50%, can be assumed—this is a “worst-case scenario” that maximizes the required sample size. If the aim is to measure a mean value, the expected standard deviation is required. The wish is for a narrow confidence interval— that is, a specified acceptable error (i.e., half the width of the confidence interval) at a given (say 95%) level of confidence. The precaution (required by some computer programs) is allowance for the expected loss of members of the chosen sample because of refusal or for other reasons; taking this into account ensures an adequate sample size despite the losses, but of course does not remove the possibility of bias caused by selective losses. The size of the population from which the sample is drawn may also influence the required sample size, but only if the population is very small.
In a study that sets out to measure more than one dependent variable, the sample size requirement will usually differ for different variables (with different expected frequencies). It then becomes necessary to either select the largest sample size or decide on a compromise that will sacrifice precision with respect to the less important variable or variables.
Planning a Descriptive Study
When planning a descriptive study (or any epidemiological study, for that matter), thought should be given to both information bias and selection bias.
To minimize information bias, clear operational definitions are required for all variables and (if categorical scales are used) for their categories; valid methods of measurement should be used, and they should be applied in a standard way. Validity should be measured if necessary. Quality control measures (including checks on correct performance and on reliability) should be built in; and data cleaning (16), including the correction of errors where possible, should be performed both before and during or after entry of data to the computer. Data entry can be made easier and more accurate by using software, such as the freeware programs EpiData (see p. 202) and Epi Info (see pp. 201, 202) that provides help in the design of a data entry form, a data entry screen, and a data set and can apply rules and calculations during data entry, for example, by restricting data to legitimate values. A record should be kept of the amount of missing data.
In a surveillance program (see Chapter 89), which is an ongoing descriptive study of health data (permitting, inter alia, the detection of outbreaks) or healthcare data, standardized working definitions and standardized methods of reporting and recording are especially important and may be particularly difficult to enforce because of the involvement of a large and constantly changing body of observers.
Especially in studies that set out to describe beliefs, perceptions, or practices regarding health or healthcare, consideration should be given to the use of qualitative methods, whose findings are described in words rather than numbers, as well as the usual quantitative methods. These methods, based on (for example) observations, conversations and in-depth interviews, or focus group sessions, can provide useful insights concerning beliefs and behavior (although not their numerical prevalence) and ways of exploiting or changing them. Reluctance of health workers or members of the public to be immunized (e.g., against swine flu) and unwillingness of parents to have their children immunized can best be combated if the motivations for and against immunization are understood. If done properly, qualitative research is as rigorous as quantitative research, but it needs special skills and generally requires the involvement of professionals who have had the requisite training. The designs used in “mixed-method” studies that integrate qualitative and quantitative data collection and analysis include the use of qualitative data as a basis for planning quantitative data-collection methods, the comparison and integration of qualitative and quantitative findings, and the quantitative analysis of qualitative data (17).
If a sample is to be used in a descriptive study, it should be a representative one (random or systematic), possibly selected after stratification, and large enough to ensure an acceptable degree of precision. Sampling generally requires a sampling frame, for example, a list of the subjects from whom the sample is to be selected. To sample newly diagnosed cases of a disease as they crop up, use may be made of systematic sampling, for example, every fourth case, or of a sampling scheme whereby a case is randomly selected from each successive block of (say, two or four) cases.
Efforts should be made to ensure full coverage of the sample. If the study is a longitudinal one, entailing repeated examination of the same subjects, it may be necessary to plan tracking procedures, including the collection of information about addresses, places of employment, and the whereabouts of family members.
To permit the assessment of sampling bias, so that its possible effect can be taken into account when interpreting the findings, the characteristics of the sample studied should be compared with those of the total study population, using whatever demographic or other information is available; and the characteristics of subjects lost from the sample (or those of a sample of the lost subjects) should, if possible, be compared with the characteristics of the sample studied. Records should of course be kept of the reasons for noninclusion in the sample, since they may point to possible bias.
In addition to these precautions to ensure the internal validity of the study, thought should be given to the usefulness of the results in other contexts, unless there is no intention to publish the results. There will usually be other health workers or researchers who will be interested in the applicability of the findings in their own healthcare services or populations, even if the study was planned to meet a specific local need. Care should therefore be taken to collect, and provide, any information about the group or population studied, or about the context, that may help others to decide on the relevance of the study findings elsewhere.
Analysis of a Descriptive Study
The analysis of a descriptive study is usually simple. The frequency distribution of each variable in the total study sample or its subgroups is tabulated; rates or proportions, preferably with their confidence intervals, are computed for “yes-no” or other categorical variables; and measures of central tendency and dispersion (see Chapter 3, pp. 50-51) are computed for metric (noncategorical) variables. Onesample significance tests (see Chapter 3, pp. 57-58 and pp. 61-62) can be used to see whether the rate or proportion, or the mean or median, conforms with some standard value or with an expected or other hypothetical value. And twosample significance tests (see Chapter 3, pp. 58-64) can be used to make comparisons with findings elsewhere.
The possible effect of misclassification can be appraised, as in the above MRSA example. Occasionally, the effect of information bias can be controlled in other ways. If, for example, there is a constant bias in laboratory results, due to a mistake in the preparation of a standard solution, it may be rectified by applying a correction factor.
The possible effect of selection bias should be taken into account when interpreting the findings, particularly if there was poor coverage of the sample. Sometimes it is possible to control selection bias by statistical manipulations during the analysis. If there was a low response rate in one sex, for example, the findings can be weighted in accordance with the sex composition of the total study population to obtain an estimate that compensates for this selectivity. A disadvantage is that this is based on the assumption that, in each sex, the subjects included and excluded are similar, which is not necessarily true.
In a longitudinal study, such as a surveillance program, the analysis is complicated by the need to describe changes with time. Numerous statistical procedures are available for the appraisal of trends (18), with or without controlling for seasonal variation and with or without controlling for deviations that may be caused by extraneous factors, such as fluctuations in diagnostic criteria. Outbreaks may be detected by changes from the “endemic” baseline values. But algorithms for the early detection of outbreaks usually use surveillance data from multiple sites.
A need sometimes arises to combine the results of two or more case-finding methods that yield different and incomplete, but overlapping, lists of cases. An estimate of overall prevalence can then be obtained by feeding the numbers, including the numbers of overlaps, into a computer program that can use the capture-recapture (or a similar) technique (19). This procedure, which is based on assumptions that are not always met (20), takes its name from its original use in estimating animal populations by capturing, marking, and releasing a batch of animals and then seeing how many of them are recaptured in the next batch of animals caught. Its earliest use in healthcare epidemiology was to estimate the number of hospital patients using methicillin (21), followed by its use in the surveillance of healthcare-associated infections (22), and it has since been used in many other studies of incidence or prevalence and of the effectiveness of ascertainment systems (23). In a capture-recapture study based on notifications of invasive neonatal group B streptococcus infections, made separately by pediatric wards and by microbiological laboratories, for example, the analysis led to the conclusion that the total number of cases was about double the total notified number (24). The capture-recapture technique may yield an overestimate if all cases have in fact been found or an underestimate if some types of case are “uncatchable” by any procedure.
ANALYTICAL OBSERVATIONAL STUDIES
The key feature of an analytical study is the examination and interpretation of associations between variables. This brings new possible biases and uncertainties in its train in addition to those besetting descriptive studies.
Associations between variables are usually detected by observing that the value of the dependent variable (e.g., the mean value, proportion, or rate) is different when the value of the independent variable (e.g., a suspected causal factor) is different. The difference in the values of the dependent variable may lie in the same direction as the difference in the values of the independent variable (a positive association) or in the opposite direction (a negative or inverse association). The strength of the association is measured by the extent of the discrepancy between the two values of the dependent
variable (say, the two rates), as measured by the ratio of the two values or by the difference between the two values. The further the ratio is from 1, the stronger the association. The discrepancy can be in either direction, depending on whether the association is positive or negative; ratios of 8 and 0.125 (i.e., one-eighth) point to associations of the same strength but different in direction. If the difference between means, rates, or proportions is used, the further it is from zero (in either direction), the stronger the association.
variable (say, the two rates), as measured by the ratio of the two values or by the difference between the two values. The further the ratio is from 1, the stronger the association. The discrepancy can be in either direction, depending on whether the association is positive or negative; ratios of 8 and 0.125 (i.e., one-eighth) point to associations of the same strength but different in direction. If the difference between means, rates, or proportions is used, the further it is from zero (in either direction), the stronger the association.
These two methods of measurement (using a ratio or a difference) do not necessarily lead to similar conclusions about the strength of the association or the factors affecting it. Etiological studies generally use ratios and assume that exposure to a risk or protective factor has a multiplicative effect; that is, exposure multiplies the risk of the condition under study by a given amount. The effects of different exposures can then be combined by multiplying them by each other. A multiplicative model is used in logistic regression analysis (see Chapter 2, p. 44) and Cox regression analysis (see Chapter 2, pp. 44-45). On the other hand, if a study is concerned with the absolute magnitude of a problem or with the resources needed to deal with it, it is more appropriate to use the absolute difference between risks or mean values and assume that an exposure has an additive effect; that is, exposure increases (or decreases) the risk or mean value by a given absolute amount. The effects of different risk factors can then be combined by adding them. This is the model used in linear regression analysis.
The ratios commonly used as measures of the strength of an association are rate ratios, risk ratios, odds ratios, and hazard ratios.
A rate ratio is the ratio of two rates that have persontime denominators (e.g., rates per 1,000 patient-days or per 1,000 person-years). A subject who was observed for 10 days would contribute 10 patient-days to the total denominator, as would 2 subjects who were each observed for 5 days or 10 subjects who were each observed for 1 day. Incidence and mortality rates (sometimes referred to as incidence density or mortality density) are of this type.
A risk ratio (confusingly, often also called a rate ratio) is the ratio of risks, which are measures that use count denominators, that is, the size of the population at risk (e.g., 10 cases per 1,000 subjects), and not person-time denominators. Prevalence (the number of cases at a given time) and cumulative incidence (the number of new cases during a given period) are measures of this type, as are simple proportions and percentages (which express the number of cases or episodes per 1 subject or per 100 subjects, respectively).
An odds ratio (see Chapter 2, pp. 23-24) is the ratio of two odds. An odds is the probability that something is present or will occur, divided by the probability that it is not present or will not occur. If the proportion of people exposed to a risk factor who develop a disease is 0.8, the odds in favor of the disease in this group is 0.8 divided by 0.2, or 4 (4 to 1). If the proportion of people not exposed to the risk factor who develop the disease is 0.2, the odds in favor of the disease in this group are 0.2 divided by 0.8, or 0.25. The odds ratio expressing the strength of the association is the ratio of these two odds, that is, 4 divided by 0.25, or 16.
Odds ratios have useful statistical properties, but may be hard to understand and are easily misunderstood. In the above example, use of the odds ratio, which is 16, gives an impression of a much stronger association than would be indicated by the risk ratio of 4 (0.8 in the exposed group divided by 0.2 in the nonexposed group).
Case-control studies yield odds ratios only (unless ancillary information is available), and not rate ratios or risk ratios. But if the condition under study is rare, there is little difference between the odds ratio and the risk ratio, and the odds ratio can be used as a substitute for the risk ratio. Under certain conditions, depending on the manner of selection of controls, the odds ratio observed in a case-control study can also be used as a proxy for the rate ratio (25).
Odds ratios are the ratios that are generally used in studies that employ logistic regression analysis, since the logistic coefficients provided by the analysis are the logarithms of odds ratios and can be converted to odds ratios by taking their antilogarithms. Some computer programs (like Win-Pepi) can use the logistic regression results to estimate risk ratios, risk differences, and other measures of effect that are less misleading than odds ratios sometimes are.
Hazard ratios are used in studies based on person-time denominators, particularly those using Cox regression analysis, where the hazard ratios are the antilogarithms of the computed coefficients.
Information Bias
As in a descriptive study, information bias may result from inadequate operational definitions of variables, inadequately standardized methods, errors in the recording or management of data, and (in a longitudinal study) from changes in disease definitions, case notification systems, or case-finding methods.
An especially insidious type of information bias, with effects that are not always easy to predict or control, may occur in an analytical study if the validity of a measure differs in different groups. For a “yes-no” variable, this effect is referred to as differential misclassification, as opposed to the nondifferential misclassification that occurs if validity, although not perfect, does not differ.
As an illustration, suppose that 20 of 100 men and 5 of 100 women report that they have had sexually transmitted diseases (STD). The observed risk ratio expressing the association between sex and a history of STD is then 4. If the sensitivity of the STD information is 80% in both sexes (with a faultless specificity of 100%)—that is, if most cases are reported and there are no false positives, and misclassification is the same in both sexes— our trusty software tells us that a true risk ratio of 5 would produce the observed risk ratio of 4. If there is nondifferential misclassification, the observed association is generally weaker than the true association. But now suppose that sensitivity is 80% in men and 40% in women. The true risk ratio that would give rise to an observed risk ratio of 4 would then be computed as 2. But if, on the other hand, sensitivity is 40% in men and 80% in women, the true risk ratio would be computed as 8. Differential misclassification can bias the result in either direction, and (without the aid of a computer) its effect is difficult to predict and difficult to compensate for.
In studies of the effect of a supposed risk factor on a disease, differential validity can express itself as diagnostic or exposure suspicion bias. Diagnostic suspicion bias can occur if the information about the disease comes from a subject, interviewer, or examiner whose report about the presence of the disease is colored by knowledge that there has been exposure to the risk factor and who is more likely to report the disease if there has been exposure. This is possible in a cohort study or a cross-sectional analytical study. Exposure suspicion bias can occur if the information about exposure comes from a subject, interviewer, or examiner whose report about the presence of the exposure is colored by knowledge of the presence of the disease. This is possible in a case-control study or a cross-sectional analytical study. Both forms of bias are less likely if there is effective blinding and if subjects, interviewers, or examiners are not aware of the study hypothesis.
Clearly, information on validity in different subgroups of the study population would be helpful when interpreting the findings.
In cohort studies, where information about exposure to risk factors is obtained at the outset of a follow-up period, this information may be biased if there are changes of exposure status during the follow-up period. Smokers may not remain smokers. This bias can be reduced by seeking and using information about these changes.
Selection Bias and Sampling Variation
The strength of associations observed in analytical studies is subject to sampling variation, and confidence intervals must be computed for the rate ratios, differences, or other measures used. The sample size required in order to obtain acceptably precise results can be calculated manually or by a computer program. If strength is measured by the ratio of two rates or proportions or odds, the calculation is based on the known or assumed value of one of the rates or proportions, the value of the ratio that it is wished to detect (at a given confidence level), and either the desired width of its confidence interval or the required power of a test to determine statistical significance. If strength is measured by a difference between two values, the calculation is based on the known or assumed standard deviations of the two values, the difference that it is wished to detect (at a given confidence level), and either the desired width of its confidence interval or the required power of a test to determine statistical significance. The expected loss of members of the chosen sample can also be taken into account. In a case-control study, the number of controls per case influences the required number of cases. Calculation of the required sample size is less simple if there are a number of independent variables.
The same possibilities of selection bias resulting from inappropriate sampling or incomplete coverage of the sample exist in analytical studies as in descriptive studies.
In addition, there are special issues to be considered in case-control studies and in cohort studies.
Case-Control Studies The study of associations in case-control studies is based on a comparison of cases (generally of a disease) with controls (who are free of the disease), with respect to their prior exposure to suspected risk or protective factors. To avoid bias, the controls should be drawn from the same population as the cases. They should represent the people who, if they had the disease in question, could have become cases in the study.
But many case-control studies are vitiated by the inappropriate selection of controls.
If the study includes all the cases occurring in a defined population, or a representative sample of them, suitable controls can be found by taking a representative sample of the individuals without the disease in the same population. This is relatively easy to do in a primary healthcare service that caters for a defined population, but it is not easy in a hospital-based study. Hospital cases with a given disease, for example, are usually drawn from an ill-defined catchment population. Even if the study is restricted to hospital cases living in a defined neighborhood, and it is practicable to select “community controls” drawn from the same neighborhood, it cannot be certain that the controls would have been treated in the same hospital if they had the disease. Population controls who are selected because of their relationship with the cases, for example, friends, neighbors, spouses, siblings, fellow workers, or classmates, may tend to resemble the cases in their circumstances, lifestyles, or (for blood relatives) genetic characteristics. In other words, there may be similarities between the cases and controls that have nothing to do with the disease and can lead to false conclusions about associations with the disease. Controls drawn from other patients in the same hospital also present problems. They do not have the disease in question, but they have other diseases, which may have their own associations with the risk or protective factors under consideration. Moreover, bias may be caused by differences between the hospital admission rates for different diseases (Berkson’s bias, admission rate bias). To minimize these problems, controls with similar diseases or clinical pictures may be selected (e.g., cancer controls for cancer cases, or women referred for breast biopsies of suspicious nodules, but not found to have breast cancer, as controls for cases found to have cancer or precancerous conditions). Patients admitted after traffic accidents or for elective surgery, blood donors, or hospital visitors are sometimes used as controls, in the hope that they represent the population base. It is usually found that the use of community controls overestimates the association between the disease and the risk factor, and the use of hospital controls underestimates it (26).
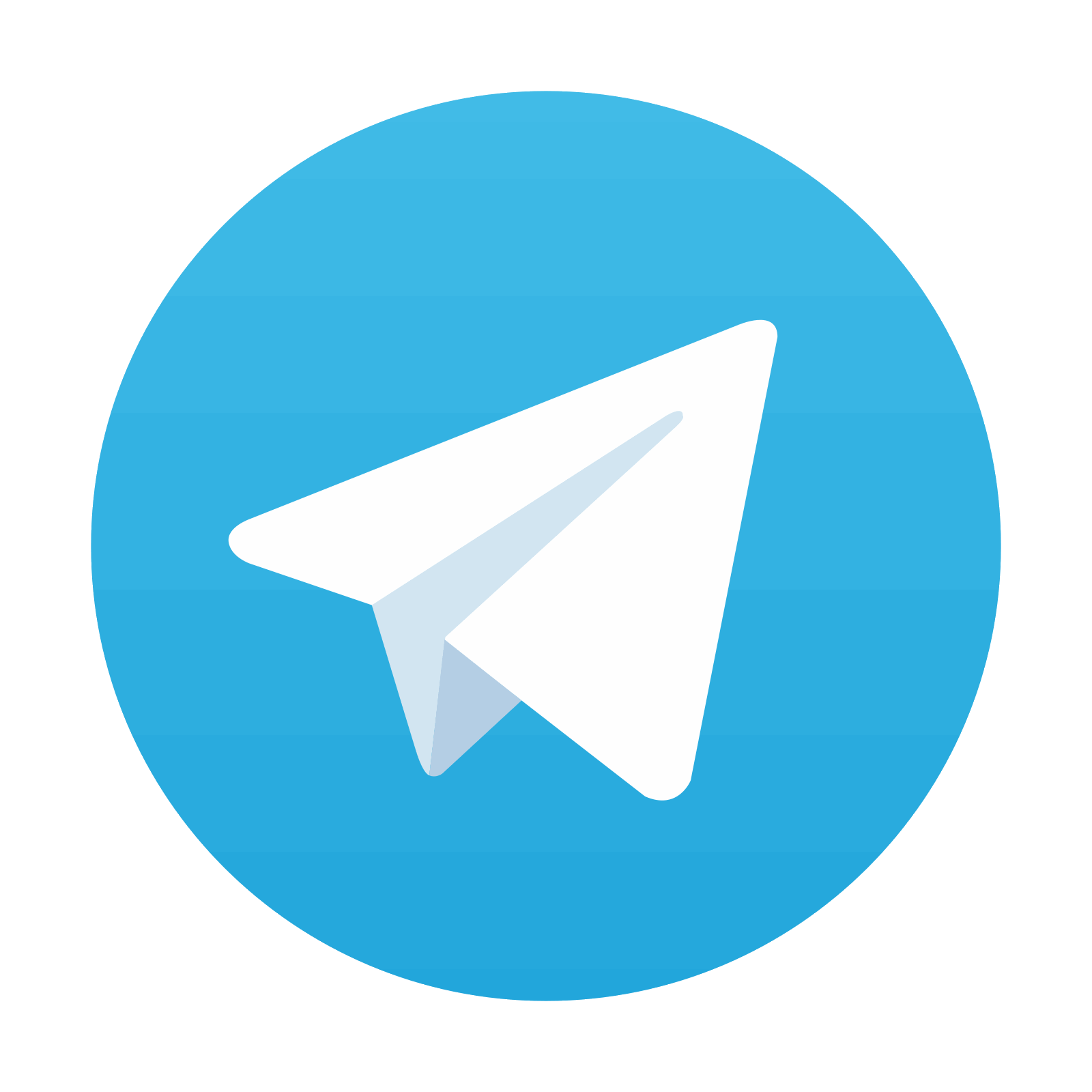
Stay updated, free articles. Join our Telegram channel

Full access? Get Clinical Tree
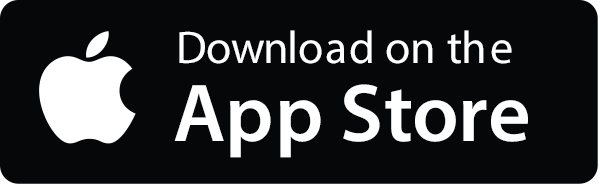
