Chapter Contents
The Issue 206
A Proposed Statistical Solution 207
Multiple Endpoints 207
Composite Endpoints 208
Multiple Treatments (Multiarm Trials) 209
The Issue of Multiplicity Adjustment in Multiarm Trials 210
The Role of Adjustments for Multiplicity 211
What Readers Should Look for 212
Conclusion 212
Multiplicity problems emerge from investigators looking at many additional endpoints and treatment group comparisons. Thousands of potential comparisons can emanate from one trial. Investigators might only report the statistically significant comparisons, an unscientific practice if unwitting and fraudulent if intentional. Researchers must report all the endpoints analysed and treatments compared. Some statisticians propose statistical adjustments to account for multiplicity. Simply defined, they test for no effects in all the primary endpoints undertaken versus an effect in one or more of those endpoints. In general, statistical adjustments for multiplicity provide crude answers to an irrelevant question. However, investigators should use adjustments when the clinical decision-making argument rests solely on one or more of the primary endpoints being significant. In these cases, adjustments somewhat rescue scattershot analyses. Readers need to be aware of the potential for underreporting of analyses.
Many analytical problems in trials stem from issues related to multiplicity. Investigators sometimes address the issues responsibly; however, others ignore or remain oblivious to their ramifications. Put colloquially, some researchers torture their data until they speak. They examine additional endpoints, manipulate group comparisons, do many subgroup analyses, and undertake repeated interim analyses. Difficulties usually manifest at the analysis phase because investigators add unplanned analyses. Literally thousands of potential comparisons can emanate from one trial, in which case many statistically significant results would be expected by chance alone. Some statisticians propose adjustments in response, but unfortunately those adjustments frequently create more problems than they solve.
Multiplicity problems stem from several sources. Here we address multiple endpoints and multiple treatments. In the next chapter, we address subgroup and interim analyses ( Chapter 20 ). The perspectives on multiplicity are contentious and complex. In proposing approaches to handle multiplicity, any position alienates many ( Panel 19.1 ). Multiplicity issues stir hot debates.

Some statisticians favour adjustments for multiple comparisons, whereas others disagree.
Several recent publications show that the multiple comparisons debate is alive and well. I . . . observe that it is hard to see views such as the following being reconciled . . .
No adjustments are needed for multiple comparisons.
Bonferroni adjustments are, at best, unnecessary and, at worst, deleterious to sound statistical inference.
. . . Type I error accumulates with each executed hypothesis test and must be controlled by the investigators.
Methods to determine and correct type 1 errors should be reported in epidemiologic and public health research investigations that include multiple statistical tests.
The Issue
Multiplicity portends troubles for researchers and readers alike for two main reasons. First, investigators should report all analytical comparisons implemented. Unfortunately, they sometimes hide the complete analysis, handicapping the reader’s understanding of the results. Second, if researchers properly report all comparisons made, statisticians proffer statistical adjustments to account for multiple comparisons. Investigators need to know whether they should use such adjustments, and readers need to know whether to expect them.
Multiplicity can increase the overall error in significance testing. The type I error (α), under the hypothesis of no association between two factors, indicates the probability of the observed association from the data at hand being attributable to chance. It advises the reader of the likelihood of a false-positive conclusion. ( Chapter 11 ) The problem emerges when multiple independent associations are tested for significance. If d is the number of comparisons, then the probability that at least one association will be found significant is (1 − [1 – α] d ). Frequently, investigators in medical research set α at 0.05. Thus, if they test 10 independent associations, assuming the universal null hypothesis of no association in all 10, the probability of at least one significant result is 0.40 (i.e., 1 − [1 − 0.05] 10 . Stated alternatively the cumulative chance of at least one false-positive result out of the 10 comparisons is 40%. Nevertheless the probability of a false positive for every single comparison remains 0.05 (5%) whether one or a million are tested.
A Proposed Statistical Solution
Most statisticians would recommend reducing the number of comparisons as a solution to multiplicity. Given many tests, however, some statisticians recommend making adjustments such that the overall probability of a false-positive finding equals α after making d comparisons in the trial. Authors usually attribute the method to Bonferroni and simply state that to test comparisons in a trial at α, all comparisons should be performed at the α /d significance level, not at the α level. Thus, for an α of 0.05, with 10 comparisons, every test would have to be significant at the 0.005 level. Analogously, some investigators retain the same individual α threshold but multiply every observed p value by d. Thus, with 10 comparisons, an observed p = 0.02 from a trial would yield an adjusted p = 0.20. Of note, the Bonferroni adjustment inflates β error, thereby reducing statistical power.
Bonferroni adjustment, however, usually addresses the wrong hypothesis. It assumes the universal null hypothesis which, simply defined, tests that two groups are identical for all the primary endpoints investigated versus the alternative hypothesis of an effect in one or more of those endpoints. That usually poses an irrelevant question in medical research. Clinically, a similar idea would be: ‘the case of a doctor who orders 20 different laboratory tests for a patient, only to be told that some are abnormal, without further detail’. ‘Controlling the probability that at least one component is rejected is usually too restrictive and rarely of interest to the researcher’. Indeed, Rothman wrote: ‘To entertain the universal null hypothesis is, in effect, to suspend belief in the real world, and thereby to question the premises of empiricism’.
Drug regulation with the need for clear dichotomous answers appropriately drives much of the activity in multiplicity adjustments. Adjustments fit the hypothesis-testing paradigm—approval or no approval—needed for drug regulation. In most published medical research, however, we encourage the presentation of interval estimation (e.g., relative risks with confidence intervals) for effects rather than just hypothesis testing (just a p value). Moreover, we suggest that the decision-making intent in most medical research discourages multiplicity adjustments.
Multiple Endpoints
Although the ideal approach for the design and analysis of randomised controlled trials relies on one primary endpoint, investigators frequently examine more than one. The most egregious abuse with multiplicity arises in the data-dredging that happens behind the scenes and remains unreported. Investigators analyse many endpoints but only report the favourable statistically significant comparisons. Failure to note all the comparisons made is unscientific if unwitting and fraudulent if intentional. ‘Post hoc selection of the endpoint with the most significant treatment difference is a deceitful trick that invariably overemphasises a treatment difference.’ Investigators must halt this deceptive practice.
Researchers should restrict the number of primary endpoints tested. They should specify a priori the primary endpoint or endpoints in their protocol. Focusing their trial increases the simplicity of implementation and the credibility of results. Furthermore, they should follow their protocol for their analysis. Deviations for data-dredging can be condoned but should be clearly labelled as explorations and fully reported. Disappointingly, trial reports frequently contain examinations of endpoints not included in the trial protocol but ignore planned primary analyses from the protocol. Safeguards to ensure that investigators have followed the protocol (such as The Lancet ’s protocol acceptance track and asking for protocols for all randomised controlled trials) provide assistance, but more extensive registering and publishing of protocols make sense. Lastly, investigators must report all the comparisons made.
Some authors have suggested that statistical adjustments for multiplicity should be applied much more frequently. They stated that up to 75% of trials with multiple primary outcomes should have adjusted for multiplicity in their analyses. However, they did not appear to have evidence that the investigators analysing the multiple outcomes were using a decision-making criterion (i.e., testing the universal null hypothesis) that would require adjustment.
Statistical adjustments for multiple endpoints might sabotage interpretation. For example, suppose investigators undertook a randomised controlled trial of a new antibiotic compared with a standard antibiotic for prevention of febrile morbidity after hysterectomy. They designated fever the primary outcome, and the results showed a 50% reduction (relative risk 0.50 [95% CI 0.25–0.99]; p = 0.048). Note the statistically significant result. Alternatively, suppose they had designated two primary endpoints: wound infection and fever. As typically happens in trials, the endpoints are highly correlated. So in addition to the 50% reduction in fever, the trial also found a 52% decrease in wound infection (0.48 [0.24–0.97]; p = 0.041). From some statisticians’ viewpoints, investigators should correct for multiple comparisons. As described earlier, the Bonferroni adjustment approach for multiple comparisons entails evaluating each primary endpoint at an adjusted statistical significance level boundary of α divided by the number of comparisons made. In this example with two comparisons (wound infection and fever), α would be divided by 2 or 0.05/2 = 0.025 for the 0.05 level of significance. Thus with adjustment, both endpoint comparisons become nonsignificant at the conventional 0.05 level and thus indeterminate (‘negative’). Seasoned clinical trialists, however, look at these results quite differently. The wound infection result enhances rather than debases the first result on fever. Clinicians understand biologically that the two endpoints are highly related. Adding the second endpoint on wound infection and observing similar results lends credence to the observed reduction in febrile morbidity. That adjustments would abolish the basic finding defies logic. Doing so would somewhat resemble a doctor finding an abnormally low haemoglobin level in a patient but no longer judging it worthy of treatment because the patient also had an abnormally low packed-cell volume (haematocrit).
Indeed, some statisticians would agree with not using formal adjustments for multiplicity in the aforementioned example. Even those predisposed to such adjustments recommend against them under certain delineated clinical decision-making scenarios. For example, if an investigator proposes to claim treatment effect if all the endpoints are significant or if most (defined in the protocol) are significant, then they assert that no adjustment for multiple endpoints is necessary.
Furthermore, the Bonferroni adjustment, advocated most frequently for multiplicity, is an overcorrection at best. Moreover, it can be a severe overcorrection when the endpoints are associated with one another, which is frequently the case. Overcorrecting for p values hampers interpretation of results. The adjustment for multiple comparisons ‘mechanizes and thereby trivialises the interpretive problem, and it negates the value of much of the information in large bodies of data’. Clinical insights remain important. Investigators need to focus on the smallest number of endpoints that makes clinical sense and then report results on all endpoints tested. If more than one primary endpoint exists, investigators should address in their discussion whether additional endpoints reinforce or detract from the core findings. Formal adjustments for multiplicity frequently obscure rather than enhance interpretation.
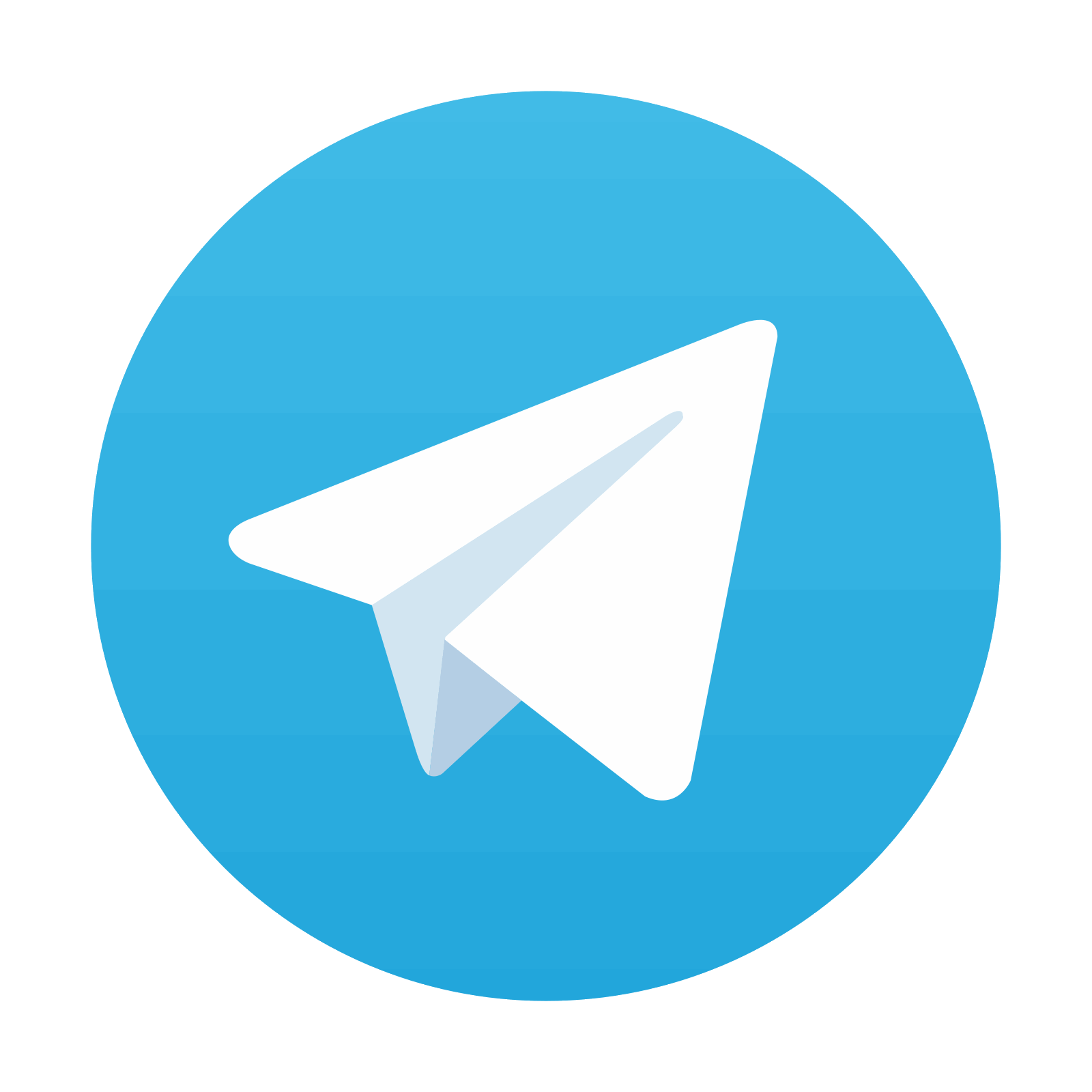
Stay updated, free articles. Join our Telegram channel

Full access? Get Clinical Tree
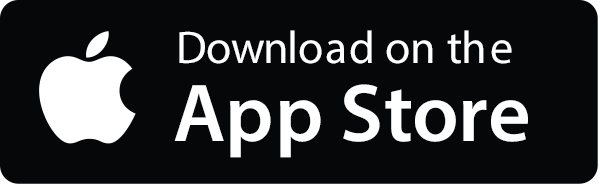
