There are three types of analytic study: cohort, casecontrol, and cross-sectional. The goal of analytic epidemiologic studies is to discover a statistical association between cases of disease and possible causes of disease, called exposures. A first step in any such study is the careful definition of terms used, especially defining what clinical and laboratory characteristics are required to indicate a case of disease.
The Cohort Study and Relative Risk
Prospective Cohort Study There are several subtypes of cohort study, but all have certain common features and are analyzed the same way. In the prospective cohort study, we identify a group of subjects (e.g., persons or patients) who do not have the disease of interest. Then, we determine which subjects have some potential risk factor (exposure) for disease. We follow the subjects forward in time to see which subjects develop disease. The purpose is to determine whether disease is more common in those with the exposure (“exposed”) than in those without the exposure (“nonexposed”). Those who develop disease are called “cases,” and those who do not develop disease are “noncases” or “controls.”
A classic example of a prospective cohort study is the Framingham study of cardiovascular disease, which began in 1948 (
3). Framingham is a city about 20 miles from Boston with a population of about 300,000, which was considered to be representative of the US population. A random sample of 5,127 men and women, age 30 to 60 years and without evidence of cardiovascular disease, was enrolled in 1948. At each subject’s enrollment, researchers recorded gender and the presence or absence of many exposures, including smoking, obesity, high blood pressure, high cholesterol, low level of physical activity, and family history of cardiovascular disease. This cohort was then followed forward in time by examining the subjects every 2 years and daily checking of the only local hospital for admissions for cardiovascular disease.
Note several features of this study. The study was truly prospective in that it was started before the subjects developed disease. Subjects were followed over many years and monitored to determine if disease occurred, that is, if they became “cases.” This is an incidence study, in which only new cases of disease were counted (because persons with cardiovascular disease in 1948 were not eligible for enrollment). In an incidence study, it is necessary to specify the study period, that is, how long the subjects were allowed to be at risk before we looked to see whether they had developed disease.
The Framingham study allowed investigators to determine risk factors for a number of cardiovascular disease outcomes, such as anginal chest pain, myocardial infarction (heart attack), death due to myocardial infarction, and stroke. One finding of this study was that smokers had a higher rate of myocardial infarction than nonsmokers. An advantage of this study design is that it is very flexible, in that the effect of many different exposures on many different outcome variables can be determined. The disadvantages are the time, effort, and cost required.
Relative Risk Performing hospital surveillance for surgical site infections (SSIs) is an example of a prospective cohort study. Assume that during one year at hospital X, 100 patients had a certain operative procedure. Of these, 40 were wound class 2 to 3 and 60 were class 0 to 1. Note that wound class was determined before it was known which patients were going to develop SSI; this makes it a prospective cohort study. A subgroup or sample of patients was not selected; that is, the entire group was studied. When the patients were followed forward in time, the following was found: of 40 patients with class 2 to 3 procedures, 10 developed SSI; of 60 patients with class 0 to 1 procedures, 3 developed SSI.
Cohort study data are commonly presented in a 2 × 2 table format. The general form of the 2 × 2 table is shown in
Table 2-1, and the 2 × 2 table for this SSI example is shown below. Notice that the columns denote whether disease (SSI) was present and the rows whether exposure (wound class 2-3) was present. In this example, exposed means being class 2 to 3 and nonexposed means being class 0 to 1. In the 2 × 2 table below, the total number of cases is 13, total noncases is 87, total exposed is 40, total nonexposed is 60, and total patients is 100.
In the exposed group, the proportion ill = 10/40 = 0.25 or 25%. In the nonexposed group, the proportion ill = 3/60 = 0.05 or 5%. We compare the frequency of disease in the exposed versus nonexposed groups by calculating the relative risk (often called risk ratio). The relative risk of 5.0 means that patients in wound class 2 to 3 were five times more likely to develop SSI than were patients in wound class 0 to 1.
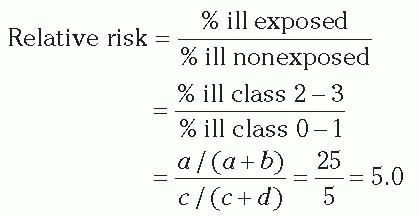
Retrospective Cohort Study A retrospective cohort study is started after disease has developed. A study period
(start date and stop date) is decided upon. Using patient records, we look back in time to identify a group (cohort) of subjects that did not have the disease at the start time. We then use patient records to determine whether each cohort member had a certain exposure. Again using patient records, we determine which cohort members developed disease during the study period. Finally, we calculate the percent with disease in those with the exposure and those without the exposure and compare the two.
The following is an example of a retrospective cohort study based on the SSI example above. Hospital X noted that the overall SSI rate of 13% was higher than in previous years. We want to determine whether a new surgeon (surgeon A) was responsible for the increase. The prospective surveillance system did not routinely record the surgeon performing each procedure, so we pull the records from each procedure and record whether or not surgeon A was involved. We find that surgeon A operated on 20 patients,3 of whom later developed SSI. Among the 80 other patients, 10 developed SSI. The percent ill in the exposed group (surgeon A) = 3/20 = 15%. The percent ill for other surgeons (nonexposed) = 10/80 = 12.5%. The relative risk = 15%/12.5% = 1.2.
The interpretation is that patients operated on by surgeon A were 1.2 times (or 20%) more likely to develop disease than patients operated on by other surgeons. Factors to consider in deciding whether surgeon A is truly a cause of the problem are presented below (see Interpretation of Data, Including Statistical Significance and Causal Inference).
To review, this was a retrospective cohort study, since data on the exposure were collected from patient records after we knew which patients had developed SSI. The retrospective nature of data collection is sometimes irrelevant and sometimes a problem. For certain types of data, such as length of hospital stay or death, retrospective data collection will be as good as prospective. However, determining other factors, such as which ancillary personnel treated a given patient, may be difficult to do after the fact, and retrospective studies using such data may be less valid.
Observational Versus Experimental Studies Epidemiologic studies are generally observational; that is, the investigator collects data but does not intervene in patient care. Patients, physicians, nurses, and random processes all play a part in determining exposures in the hospital. The goal of observational studies is to simulate the results of an experimental study (see Quasi-Experimental Studies)
In an experimental study, a group (cohort) of subjects is identified and the investigator assigns some of them to receive treatment A (exposed) and the remainder to receive an alternate treatment B (nonexposed). The patients are followed forward in time, the cases of disease are recorded, and the rates of illness and relative risk are calculated as usual. The experimental study is a special type of a prospective cohort study where the two exposure groups are assigned by the investigator.
Cohort Studies With Subjects Selected Based on Exposure In this type of cohort study, subjects are selected based on exposure. We select two subgroups: one that is exposed and one that is nonexposed. Both groups are followed forward in time to see how many develop disease. Consider the SSI example and surgeon A above. We study all 20 patients operated on by surgeon A (exposed); of the 80 patients operated on by other surgeons, we randomly select 40 (nonexposed). Thus, only 60 patients of the original group of 100 are included in this study.
Note that this is a type of cohort study, not a case-control study. In a case-control study, the subjects are chosen based on whether or not they have disease. In this study, subjects were chosen based on whether or not they had exposure.
The disadvantage of this type of cohort study, where the subjects are selected based on exposure, is that only one exposure (i.e., the exposure that you selected subjects on) can be studied. However, this type of study is very useful for studying an uncommon exposure. In the SSI surveillance example used above, consider the situation if there had been 500 surgical procedures, and surgeon A had performed only 20 of them. If you performed a cohort study of the entire group, you would have to review 500 charts, which would waste time and effort. Instead, you could perform a cohort study of the 20 procedures performed by surgeon A (exposed), and 40 randomly selected procedures performed by other surgeons (nonexposed). The second alternative would be much more efficient.
Cohort Studies—Summary Cohort studies can be prospective or retrospective, observational or experimental. They usually include a whole group of subjects, but studying two subgroups selected based on exposure is also possible. The 2 × 2 table layout and calculations are the same for all types of cohort studies. All have in common that subjects are chosen without regard to whether they develop disease.
The Case-Control Study and Odds Ratio
In a case-control study, we choose subjects for study based on whether they have disease. Since we have to know which subjects developed disease before we select them, case-control studies are always retrospective. We usually study those with disease (cases) and choose a sample of those without disease (controls). We usually study one to four controls per case. The more controls, the greater the chance of finding statistically significant results. However, there is little additional benefit from studying more than four controls per case. Controls are usually randomly selected from subjects present during the study period who did not have disease.
Example: Case-Control Study of Surgical Site Infections This is the same example presented in the section on cohort study and relative risk. At hospital X, 100 patients had a certain operative procedure, 40 class 2 to 3 (exposed) and 60 class 0 to 1 (nonexposed), and 13 developed SSI. To perform a case-control study, we select the 13 patients with SSI (cases) and also study 26 patients who had surgical procedures but did not have SSI (controls). We studied two controls per case, but could have studied fewer or more controls. The controls were randomly chosen from all patients who had the surgical procedure under study but did not develop SSI. From their
medical records, we find which of the subjects had class 2 to 3 procedures and which had class 0 to 1 procedures. Our data showed that, of 13 cases, 10 had class 2 to 3 procedures. Of 26 noncases, 9 had class 2 to 3 procedures. The 2 × 2 table for this example is as follows:
In a case-control study, we cannot determine the percent ill in the exposed or nonexposed groups, or the relative risk. In this example, note that the percent ill among class 2 to 3 is NOT = 10/(10 + 9) = 52.6%. However, we can validly calculate the percent of cases that were exposed, 10/13 = 76.9%, and the percent of noncases that were exposed, 9/26 = 34.6%. Note that the cases were much more likely to have the exposure than were the controls. Most importantly, we can calculate the odds ratio (also called the relative odds;
Table 2-1) as follows:

We can interpret the odds ratio as an estimate of the relative risk. Using the case-control method, we estimated that patients in class 2 to 3 were 5.6 times more likely to develop SSI than were patients in class 0 to 1. Note that the odds ratio is similar to, but slightly higher than, the relative risk (5.0) we calculated previously. If the frequency of disease is not too high, that is, is less than approximately 10%, the odds ratio is a good approximation of the relative risk.
The meanings of the letters (i.e.,
a,
b,
c, and
d) used to represent the 2 × 2 table cells are different in cohort versus case-control studies (
Table 2-1). For example, in a cohort study,
a denotes the number of cases of disease among exposed persons; in a case-control study,
a denotes the number exposed among a group of cases. Although this distinction may not be clear to the novice, it will suffice to keep in mind that in a case-control study, it is not valid to calculate percent ill or relative risk, but it is valid to calculate an odds ratio.
A more in-depth explanation of the odds ratio is as follows. In a case-control study, we actually measure the odds of exposure among those with disease and the odds of exposure among those without disease. The ratio of these two odds is the exposure odds ratio; if equal to 2.0, this would be interpreted as “the odds of exposure are twice as high in those with disease versus those without disease.” However, the exposure odds ratio is not a very useful quantity. Fortunately, it can be proven mathematically that the exposure odds ratio equals the disease odds ratio. Therefore, using our example of 2.0, we can say that the odds of disease are twice as high in those exposed versus those not exposed, which is closer to being useful. Finally, we use the odds ratio as an approximation of the relative risk (where the frequency of disease is not too high) and say simply that those with exposure are twice as likely to get disease.
Selection of Controls Selection of controls is the critical design issue for a case-control study. Controls should represent the source population from which the cases came; represent persons who, if they had developed disease, would have been a case in the study; and be selected independently of exposure
(4). It is always appropriate to seek advice when selecting controls, and may be worthwhile to select two control groups to compare the results obtained with each.
An example of incorrect selection of controls is provided by a case-control study of coffee and pancreatic cancer (
3,
5). The cases were patients with pancreatic cancer, and controls were selected from other inpatients admitted by the cases’ physicians but without pancreatic cancer. The finding was that cases were more likely to have had the exposure (coffee drinking) than the controls, which translated into a significant association between coffee drinking and pancreatic cancer. The problem was that the controls were not selected from the source population of the cases (cases did not arise from hospital inpatients) and thus were not representative of noncases. The physicians admitting patients with cancer of the pancreas were likely to admit other patients with gastrointestinal illness; these control patients were less likely to be coffee drinkers than the general population, possibly because they had diseases that prompted them to avoid coffee. A better control group might have been healthy persons of similar age group to the cases.
More contemporary examples of problematic control selection are studies of the association between vancomycin receipt and vancomycin resistance (
6). Cases are often hospitalized patients who are culture positive for vancomycin-resistant enterococci. Controls have often been selected from patients who were culture positive for vancomycin-sensitive enterococci. Using this control group, case-patients will be more likely to have received vancomycin than the controls, resulting in a significant association and elevated odds ratio. The problem is that controls were not representative of the source population and were less likely to have received vancomycin than other patients, since vancomycin would have suppressed or eliminated vancomycin-sensitive microorganisms. Better control groups would be hospital patients similar in age and severity of illness to the cases.
A potential problem is that hospital patients without a positive culture may include some patients who had the microorganism but were not cultured. Inclusion of these patients as controls would bias the odds ratio to 1.0 (null result). An alternative method is to limit controls to those with at least one clinical culture performed. However, this may not be preferable since it results in selection of sicker controls (“severity of illness bias”) and also biases the odds ratio toward 1.0
(7). Another way to look at this issue of potential “contamination” of the control group with unrecognized cases is as follows: in a study design called the case-cohort study, cases are compared with subjects chosen from all patients (i.e., from both cases and noncases); then, the
ad/
bc statistic equals the relative risk rather than the odds ratio; therefore, inadvertent inclusion of noncases in the control group when performing a casecontrol study may “bias” the odds ratio toward the relative risk and thus be advantageous.
Incidence Versus Prevalence
Incidence includes only new cases of disease with onset during a study period; the denominator is the number of subjects without disease at the beginning of the study period. Incidence measures the rate at which people without the disease develop the disease during a specified period of time; it is used to study disease etiology (risk).
Prevalence includes both new and old cases that are present at one time and place, measuring the proportion of people who are ill. The commonest measure of prevalence is point prevalence, which is the proportion of individuals who are ill at one point in time. Point prevalence is a unitless proportion. A different measure of prevalence, period prevalence, is the proportion of persons present during a time period with disease. Period prevalence has been criticized as an undefined mixture of both prevalent and incident cases without quantitative use, but is occasionally seen.
Prevalence studies are the ideal way to measure disease burden and plan for needed resources. For example, if we wanted to know how many isolation rooms would be needed for patients with resistant microorganisms, we would want to know average prevalence, that is, the total number of patients with recognized drug-resistant microorganisms of either new or old onset in the hospital at any given time.
Prevalence can also be used as a simple, quick, and dirty way to measure disease frequency and risk factors, but such estimates may be biased by length of stay. It is often said that prevalence equals incidence times duration. That is, prevalence is higher if either incidence is higher or if the duration of the illness is longer. In hospital studies, prevalence is greatly influenced by length of stay and mortality. For example, assuming that ascertainment of vancomycin-resistant enterococci is stable, the prevalence of vancomycin-resistant enterococci in a hospital may decrease because of an effective prevention program, or because patients with this microorganism are being discharged sooner or dying more commonly than had been the case previously.
Point prevalence and incidence density are mathematically linked; in a steady-state or dynamic population, one can be derived from the other. Prevalence can be derived from incidence density and distributions of durations of disease, and incidence density may be derived from prevalence and distributions of durations to date of disease
(8,
9,
10,
11).