Some Basic Concepts and Terms
The modern patient safety movement has emphasized medication errors, handoff errors, infections, and surgical errors; all of these areas are amenable to technological (e.g., computerized order entry), procedural (e.g., double checks), and policy (e.g., “sign-your-site”) solutions. Diagnostic errors have been less well emphasized, in part because they are more difficult to measure and to fix.
Interestingly, this relative inattention to diagnostic errors began from the very start of the patient safety movement.1 In the landmark Institute of Medicine report To Err is Human,2 the term “medication errors” is mentioned 70 times, while “diagnostic errors” comes up only twice. This is ironic, since diagnostic errors accounted for 17% of preventable errors in the Harvard Medical Practice Study,3 the source of the IOM estimate of 44,000 to 98,000 yearly deaths from medical mistakes (the “jumbo jet a day” figure that helped launch the safety movement; Chapter 1). A systematic review of autopsy studies conducted over four decades found that nearly 1 in 10 patients suffered a major antemortem diagnostic error, a figure that has fallen only slightly (the best recent estimate is about 5%) despite all of today’s advanced imaging and laboratory techniques.4 Diagnostic error remains the most common reason for a paid malpractice claim in ambulatory patients.5
At first glance, diagnostic errors would seem to represent human failings—pure failures of cognition. And it is true that, perhaps more than any other area in patient safety, the training and skills of the diagnostician remain of paramount importance. However, in keeping with our modern understanding of patient safety, there are system fixes that can decrease the frequency and consequences of diagnostic mistakes. In this chapter, I will discuss the cognitive as well as the process failures (such as failure to transfer the results of a crucial laboratory or radiologic study to the correct provider in a timely way) that give rise to diagnostic errors.
Missed Myocardial Infarction: A Classic Diagnostic Error
Annie Jackson, a 68-year-old African-American woman with mild diabetes, high blood pressure, and elevated cholesterol, presented to the emergency department after 30 minutes of squeezing chest discomfort. An ECG was quickly obtained. The ER physician, Dr. Zoe Bennett, studied the tracing and saw some nonspecific changes in the ST and T segments—not entirely normal but not the ST-segment elevations that are classic for acute myocardial infarction (MI). On exam, she found mild tachycardia, clear lungs, and mild tenderness over the lower part of the patient’s sternum. She considered the latter discovery quite reassuring (after all, such tenderness would be more characteristic of a musculoskeletal process than a heart attack), but also ordered a troponin (a biomarker released by damaged heart cells). It came back mildly elevated, again not in the range specific for MI but not normal either. Nevertheless, she made a diagnosis of costochondritis (inflammation of the sternum–rib joint), prescribed an anti-inflammatory agent and bed rest, and released Ms. Jackson from the emergency department. She died later that night, a victim of an untreated MI.
We can only guess which cognitive error caused Dr. Bennett to release Annie Jackson from the ER.6 Perhaps because Ms. Jackson was a woman, she underestimated her chance of having a heart attack. She almost certainly relied too heavily on chest wall tenderness for her diagnosis—it is unusual, but not unheard of, in MI patients. She also overemphasized the lack of clear evidence on the ECG and troponin tests. Although they were “nonspecific,” both were clearly abnormal and thus justified admission. Maybe she was just exhausted after a long day at work.7
We do know, however, that this particular error—sending patients home with heart attacks—is distressingly common and frequently lethal. Nearly 1 in 25 patients with MIs are mistakenly sent home, and these patients have a much higher death rate than MI victims who are correctly diagnosed and hospitalized.8 Because missed MI is the best-studied diagnostic error, I will use it to make several broader points about these errors.
In the 1970s, researchers began studying the problem of missed MIs and quickly concluded that many errors were related to patient characteristics. Physicians were more likely to send patients home despite worrisome histories or abnormal data when the patients were in groups traditionally believed to be at lower risk for MI, such as younger patients (under age 55) and women. Nonwhites were also mistakenly sent home more often, raising the question of racial bias, conscious or unconscious, among caregivers.8 In one particularly sobering study, 720 physicians were shown videotapes of actors playing patients with chest pain that could have been heart related.9 Four actors, each speaking precisely the same script, appeared on the videos: a white man, a white woman, a black man, and a black woman. Regardless of their own race and ethnicity, the physicians were far more likely to recommend cardiac catheterization for the white male than for the black female. Similar variations in diagnostic and therapeutic practices have been seen elsewhere in medicine, catalyzing vigorous efforts to address these “healthcare disparities.”10
Researchers found that physician-specific differences were also at play. For example, one study showed that senior physicians were significantly more likely than their younger colleagues to correctly hospitalize chest pain patients (those with real MIs).11 Were the older physicians better diagnosticians? Perhaps not—the older physicians were also more likely to hospitalize patients without heart attacks. In other words, with experience came risk aversion. Another study showed that risk aversion was not simply a function of age. One hundred and nineteen physicians completed a questionnaire assessing their attitudes toward risk. Doctors who appeared to be risk seekers (e.g., those who like fast cars and sky diving) were four times more likely to send the same chest pain patient home than the risk avoiders.12
One could easily look at cases like Annie Jackson’s and see careless doctoring, a blown diagnosis, and a fatal mistake. But by now, hopefully, you’re approaching this case with a more systems-focused mindset, thinking: How can we improve our ability to diagnose patients who come to the ER with a constellation of symptoms, findings, and risk factors that may yield ambiguous results but can be life-threatening? Too often, without a systematic approach, the clinical decision—to admit or discharge the patient—is based on the physician’s faulty reasoning, which in turn may be traced to poor training, inadequate experience, personal and professional bias, fuzzy thinking brought on by overwork and fatigue, or even the physician’s own tolerance for risk.
Cognitive Errors: Iterative Hypothesis Testing, Bayesian Reasoning, and Heuristics
As cognitive psychologists began to study how physicians think, they found that even well-trained doctors can engage in faulty thinking because they take cognitive shortcuts, reinforced by a professional culture that rewards the appearance of certainty.13,14 This means that preventing diagnostic errors is likely to depend on understanding how physicians approach diagnostic decisions, and providing them with tools (either cognitive or adjunctive, such as information technology) to help them make correct decisions more often.
Beginning in the 1970s, several researchers began to try to understand how great diagnosticians think. Led by Dr. Jerome Kassirer (later the editor of the New England Journal of Medicine), they observed the diagnostic reasoning of dozens of clinicians, and found that the good ones naturally engaged in a process called iterative hypothesis testing.15 This means that, after hearing the initial portion of a case, they immediately began thinking about possible scenarios to explain the facts, modifying their opinions as more information became available. For example, a skilled physician presented with the case of a 57-year-old man with 3 days of chest pain, shortness of breath, and light-headedness responds by thinking, “The worst thing this could be is a heart attack or blood clot to the lungs. I need to ask a few more questions to see if the chest pain bores through to the back, which would make me worry about an aortic dissection. I’ll also ask about typical cardiac symptoms, such as sweating and nausea, and see if the pain is squeezing or radiates to the left arm or jaw. But even if it doesn’t, I’ll certainly get an ECG to be sure no cardiac event has occurred. If he also reports a fever or cough, I might begin to suspect pneumonia or pleurisy. The chest x-ray should help sort that out.”
Every answer the patient gives and each positive or negative finding on the physical examination (yes, there is a fever; no, the spleen is not enlarged) triggers an automatic, almost intuitive recalibration of the probability of the various alternatives. The skilled diagnostician does this so effortlessly that novices often struggle as they try to understand the science that underlies the expert’s decision to embrace certain facts (the clear lung fields in the patient with dyspnea markedly elevate the probability of pulmonary embolism since this would be highly unusual in severe heart failure) while discarding others (the lack of an S3 gallop—a subtle and often absent finding—does little to dissuade the expert from the possibility of heart failure).
We now recognize that much of this art consists of applying an unconscious, intuitive version of Bayes’ theorem, developed by the eighteenth-century British theologian-turned-mathematician Thomas Bayes.16 In essence, Bayes’ theorem says that any medical test must be interpreted from two perspectives. The first is: How accurate is the test? That is, how often does it give right or wrong answers? The second is: How likely is it that this patient has the disease the test is looking for? Bayesian reasoning is why it is foolish to screen apparently healthy 35-year-old executives with a cardiac treadmill test (or, for that matter, a “heart scan”), because positive results will mostly be false positives. Conversely, a 65-year-old smoker with high cholesterol who develops squeezing chest pain when shoveling snow has about a 95% chance of having significant coronary artery disease. In this case, a negative treadmill test lowers this probability to only about 80%, so the clinician who reassures the patient that the normal treadmill means his heart is fine is making a terrible, and potentially fatal, mistake.
In addition to misapplications of iterative hypothesis testing and failure to appreciate the implications of Bayesian reasoning, we now understand that many diagnostic errors are caused by cognitive shortcuts (“heuristics”) that clinicians take, often in the name of efficiency.17 For example, many errors occur when clinicians are too quick to pronounce judgment, and then defend that turf too vigorously despite the emergence of contradictory evidence.13,14 This is human nature, of course; we tend to see what we expect to see rather than than what’s actually in front of our eyes. By the way, did you notice the word “than” used twice in a row in the previous sentence? Even when we don’t intend to do it, our brains can take cognitive shortcuts to get us to our goal—whether it’s finishing a sentence or discharging a patient from the ER.
This particular cognitive bias, known as “anchoring” (or “premature closure”), is only one of the many pitfalls that underlie many diagnostic errors. Other common biases include the availability heuristic, framing effects, and blind obedience (Table 6-1).22 Practicing physicians were first introduced to these concepts in the past decade, although the concepts have long been applied to other domains in which decisions need to be made under conditions of uncertainty, ranging from battlefields to Wall Street.
Circumstance and Pitfall | Classic Definition | Corrective Strategies | Example |
---|---|---|---|
Availability heuristic | Judging by ease of recalling past cases | Verify with legitimate statistics (“If you hear hoof beats, think about horses, not zebras”) | A patient with crushing chest pain was incorrectly treated for myocardial infarction, despite indications that an aortic dissection was present18 |
Anchoring heuristic (premature closure) | Relying on initial impressions | Reconsider in light of new data or second opinion, consider extremes (“If the patient dies unexpectedly, what would it be from?”) | Repeated positive blood cultures with Corynebacterium were dismissed as contaminants; the patient was eventually diagnosed with Corynebacterium endocarditis19 |
Framing effects | Being swayed by subtle wording | Examine case from alternative perspectives (“Let’s play devil’s advocate …”) | A heroin-addicted patient with abdominal pain was treated for opiate withdrawal, but proved to have bowel perforation20 |
Blind obedience | Showing undue deference to authority or technology | Reconsider when authority is more remote (in case of human authority); assess test accuracy (in case of technology) | A false-negative rapid test for Streptococcus pharyngitis resulted in a delay in diagnosis21 |
Over the past decade, researchers began to study these biases in healthcare. In one interesting study, internal medicine residents were presented with a series of cases in which the diagnosis was known.23 The researchers then presented additional cases with similar symptoms but different correct diagnoses. The residents were more likely to offer (mistaken) diagnoses drawn from the first cases in the subsequent cases, clear evidence of availability bias. The researchers repeated the exercise after asking residents to engage in reflective practice, a process that lies at the core of a skill known as metacognition (thinking about one’s own thinking).24 The impact of availability bias was lessened considerably, offering hope that some errors related to faulty application of heuristics can be prevented.
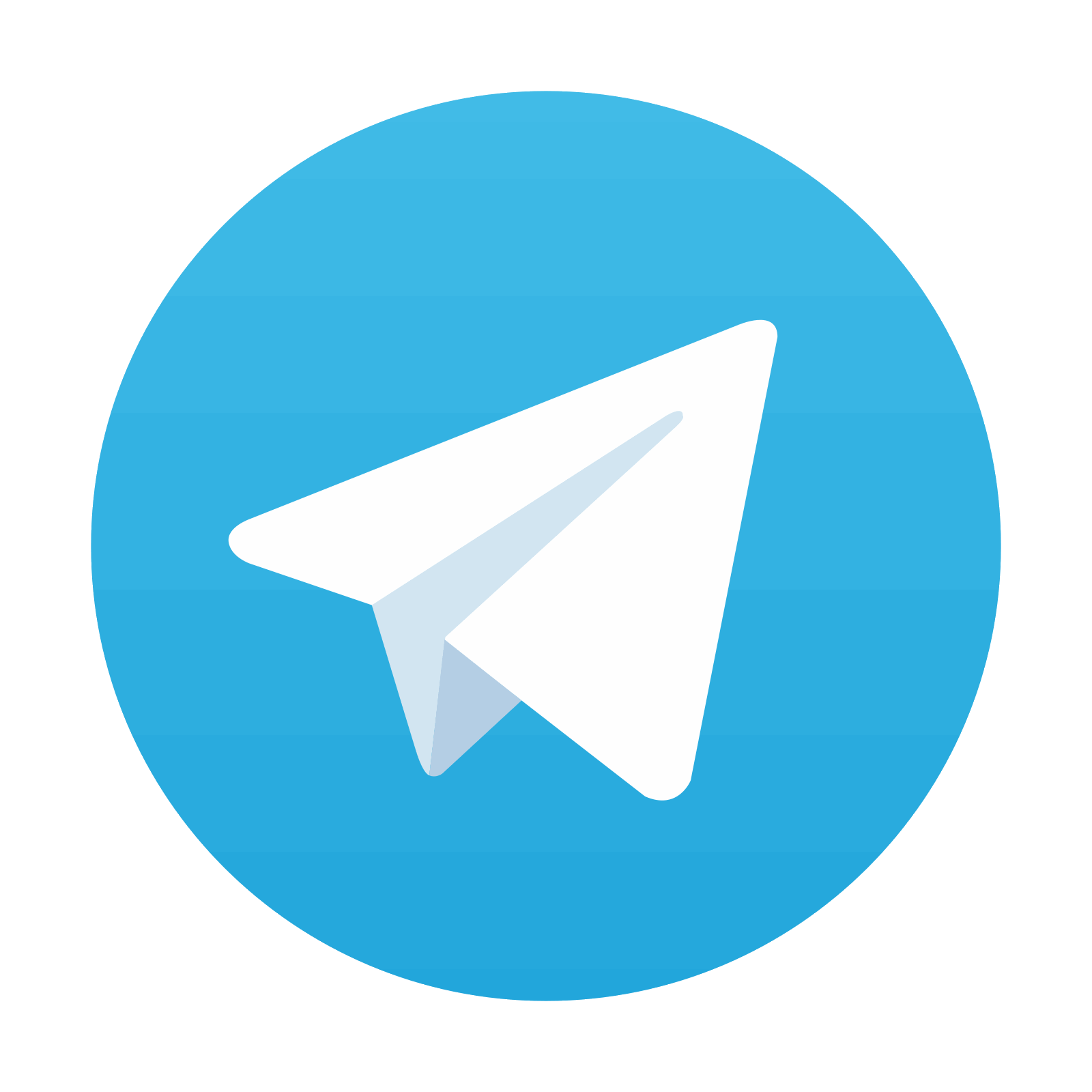
Stay updated, free articles. Join our Telegram channel

Full access? Get Clinical Tree
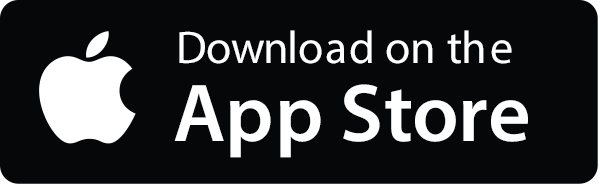
