Introduction
Although randomized controlled experiments remain the gold standard for medical research, observational studies—studies in which the researcher does not assign or alter any factor of interest—still play an important role.
Some research questions lend themselves exclusively to observational designs. In many instances, the factor of interest cannot be controlled by the investigator. Consider a study on the impact of race on the effectiveness of pegylated interferon and ribavirin in treating chronic hepatitis C virus infection (1). The researcher cannot randomly assign being Caucasian to one group of patients and being African American to another group; such a study must be observational. Similarly, genome-wide association studies must be observational because researchers cannot alter people’s genetic makeup. Other research questions might not involve relating a factor of interest to an outcome. Studies that seek only to characterize an outcome in a single population of interest will, by definition, be observational. For example, a recent study sought to determine the proportion of patients who lost independence or physical function 1 year after suffering a myocardial infarction (MI) (2).
Even for research questions that could be answered by a randomized trial, an observational study might be advantageous. Randomized trials are costly and time-consuming to run and entail navigating and complying with complex regulations (see references 3 and 4 for examples). Using data from a registry or a previously completed trial investigating a different research question would allow analysis of data far more quickly. In fact, more data might be available from registries than could be obtained in a new trial. For example, consider early studies on the efficacy of coronary artery bypass grafting (CABG) compared with percutaneous coronary intervention (PCI). A pooled analysis of eight randomized controlled trials involved a total of only 3,371 patients (an average 421 patients per trial) (5), whereas an analysis of a single registry included 6,814 patients (6). Observational data also can allow researchers to consider a wider group of patients than would be possible in a controlled trial; many randomized experiments exclude large subpopulations, particularly those that have other comorbidities or significant risk factors. Finally, in many instances, it can be unethical to consider randomizing some factors that are known to compromise patient health, such as smoking status or physical activity level.
Although using observational data for biomedical research can have advantages over implementing and running a randomized controlled trial, there are many pitfalls in using observational data that can undermine the validity of conclusions drawn from such analysis. Investigators must be aware of these potential problems so that they can be mitigated when possible and acknowledged as limitations otherwise.
This chapter focuses on scenarios in which the data have not been collected to specifically answer the question of interest—that is, when general registry data or data from a previous trial are used. (Observational studies in which the investigator designs the data collection to answer a question that lends itself exclusively to an observational design are less prone to the pitfalls we will discuss, although certainly not immune.) The chapter refers to the factor being studied relative to an outcome as “treatment,” even if the factor has nothing to do with medical therapy and cannot be assigned by the investigator.
Many types of named biases and issues can arise from using observational data (Table 13–1). We discuss those most commonly encountered in biomedical research, but the underlying cause of most biases is either confounding or obtaining a sample that is not representative of the population of interest. In fact, the underlying problem with confounding, as we will discuss shortly, is that we do not obtain a representative sample of the population of interest in all treatment groups. Therefore, if we can recognize situations where the sample is not representative of the population of interest, we can recognize almost all problems associated with observational data and are free from having to memorize a long list of pitfalls. This highlights the importance of clearly defining the population.
Key Concepts
As a motivating example, we consider the research question in the report by Pocock et al. (5). The investigators were interested in determining the difference in the average survival time after CABG compared with PCI among American adults with coronary artery disease (CAD) who have just undergone coronary catheterization. In this example, the population, or group of patients the investigator is interested in studying, is all US adults with CAD who had an indication for and underwent coronary catheterization. The parameter of interest, or summary measure of the population, is the difference between the mean survival time if the entire population had undergone CABG and the mean survival time if the entire population had undergone PCI. Because it is not logistically feasible to collect survival times on all US patients with CAD, nor is it possible to have patients undergo both CABG and PCI simultaneously, we must collect data on smaller numbers of patients who undergo only one of the procedures. In other words, we obtain data from a sample of all patients included in the population. Assuming for now that all patients were followed until death, we can calculate the difference in the average survival time for CABG versus PCI among the patients included in our sample. A summary measure of the sample, such as the difference in average survival time, is called a statistic. We hope to use the computed statistics to infer likely values for the parameter.
The difference in the mean survival time calculated in our sample is not likely to equal the true mean difference in the population, although we hope that the two would be close. Some of the difference may result from sampling error, perhaps more accurately described as sampling variability or sampling deviation. If we redid the study using the same methods to identify and select subjects, we would obtain a different sample and, thus, a different statistic. The difference between the two statistics calculated from the two samples results from sampling variability. This deviation occurs because we observe data only on a portion of the total population. We might consider taking samples from the population and calculating the statistic of interest many times, with the hope that the average of the statistics calculated from each sample would equal the parameter. If this is the case, then the statistic is considered a valid estimate of the parameter. We can ensure that the sample statistic is valid by guaranteeing that the sample is representative of the population. Any discrepancy between the average of the statistic and the parameter results from systemic error
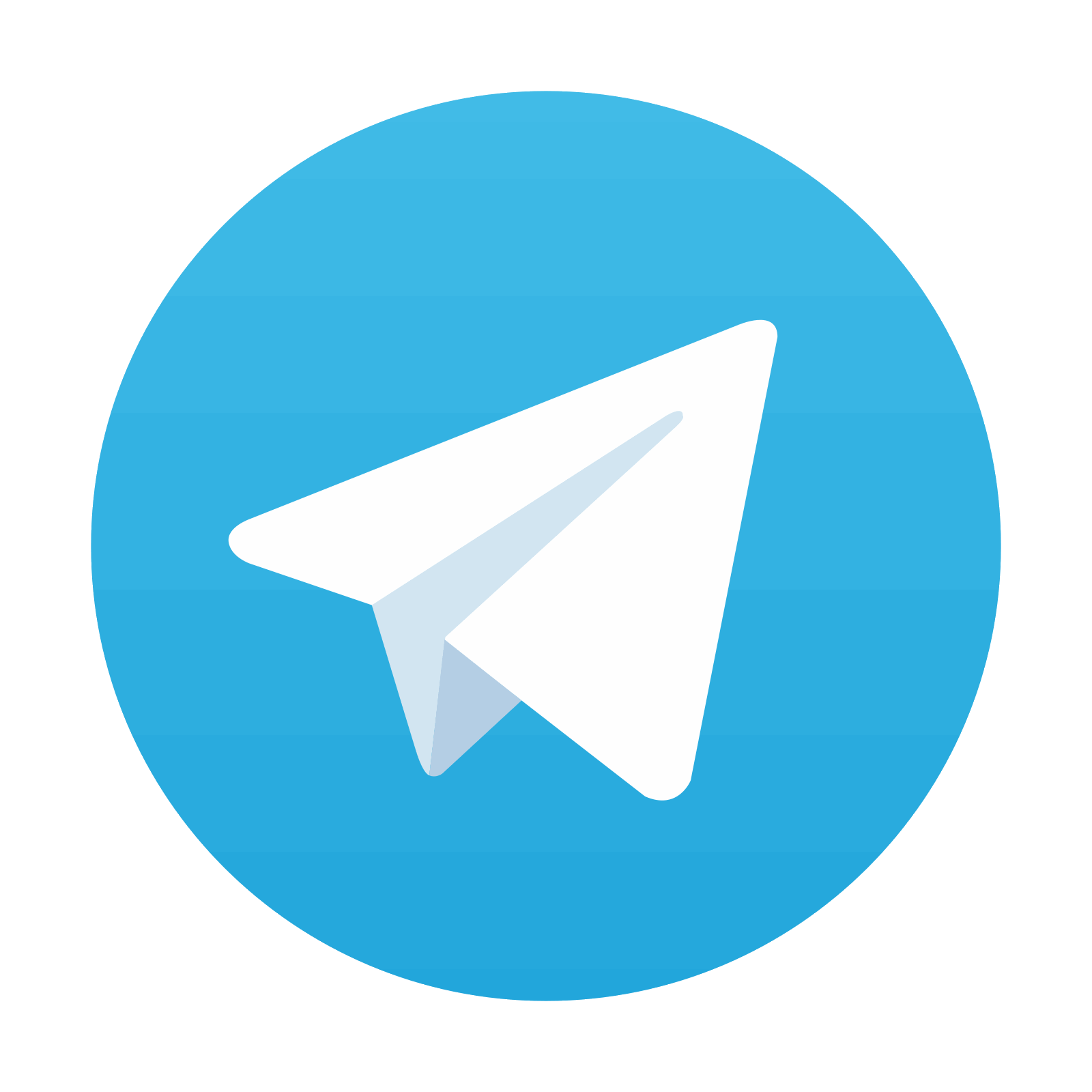
Stay updated, free articles. Join our Telegram channel

Full access? Get Clinical Tree
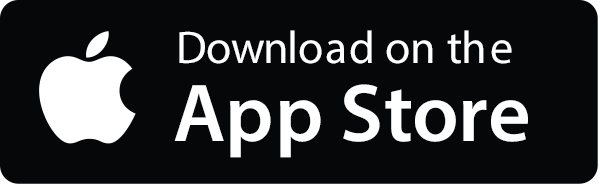

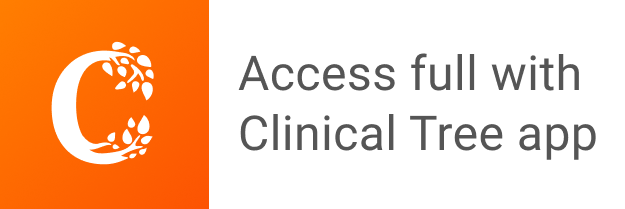