Assessment of Drug Efficacy and Relevant Clinical Issues
A series of related issues is pertinent to the decision-making process a clinician uses in the application of drug therapy
The first section of this chapter considers the quality of the research data on drug efficacy by classifying studies based on predetermined criteria for methodological rigor. The companion section on meta-analysis reviews the rationale for and the potential complications inherent in statistically summarizing data across several studies that assess treatment efficacy. Although mindful of the inherent shortcomings in this statistical approach, we believe such summaries provide the clinician with a meaningful quantitative statement about the clinical value of a specific therapy
The next section addresses issues relevant to the clinician-patient relationship during the assessment, initial treatment, and maintenance/prophylactic phases of psychopharmacotherapy
The final two sections discuss the U.S. Food and Drug Administration (FDA) regulatory process and the cost of treatment. Expenses associated with assessment and treatment and, more importantly, the total economic impact on patients, their families, and society are considered.
TABLE 3-1 CLASSIFICATION OF STUDY DESIGNS | ||||||||||||||||||||||||||
---|---|---|---|---|---|---|---|---|---|---|---|---|---|---|---|---|---|---|---|---|---|---|---|---|---|---|
|
Evaluation of Drug Study Designs
To accurately understand the literature, we have provided two perspectives so that practitioners can make the best decision about specific drug choices for their patients:
We classify studies (i.e., class I, II, III) on the basis of their methodological rigor so that the reader can judge the quality of their data (Table 3-1).
We provide statistical summaries of drug outcome studies. These produce a “bottom line” quantitative assessment of the difference between an experimental drug and placebo or other standard comparator agent (see “Drug Management” later in this chapter).
We first consider the classification of studies by rigor (i.e., the extent to which the design allows the investigator to adequately test the hypothesis in question). There are several important elements in a well-controlled study (see Table 3-1). Without adequate controls and appropriate methodology the ability to generalize is compromised, bringing into question the validity of a study, the interpretation of its results, or both.
The recent concerns raised about potential bias related to the conduct and publication of industry-sponsored trials make these issues even more critical to provide the most objective guidance to clinicians in caring for their patients (1,2).
DOUBLE-BLIND TECHNIQUES
In a double-blind study, neither the patient nor the evaluator knows who is receiving active experimental medication or placebo. If enough patients are available for three or more groups, one or more active control medication groups can also be included. A standard active drug control serves two important purposes. First, it validates the experiment by demonstrating that the standard drug is clearly superior to placebo in this population. Second, it serves as a benchmark (because it has a known efficacy) by which to compare a new treatment. For example, a new drug could be equal to or better than a standard drug, and both should be better than placebo. Alternatively, the new drug could be less effective than the standard drug but more efficacious than placebo.
RANDOM ASSIGNMENT
Random assignment is the most important element of a controlled trial.Without it, patients most likely to respond could be preferentially assigned to one treatment arm, and any difference in efficacy would be secondary to this bias. When random assignment is used, a variety of confounding variables are equalized across the groups, including those of which the investigators are unaware (3). Randomized follow-up methods are particularly helpful in resolving important questions not addressed in short-term trials (4).
STUDY DESIGN
Parallel groups involve the assignment of patients to two or more treatments (e.g., new agent vs. placebo, a standard agent, or both) that proceed concurrently. Unlike crossover designs, any carryover effects of the first treatment are avoided.
In crossover studies, patients are randomly assigned to one of the two arms so that a placebo is given first and then the active drug, or vice versa. The usual design is a placebo lead-in period, then active drug A or B, succeeded by a placebo period again, and then the “crossover” from A to B or B to A. This design can also take a simpler form if B is a placebo. If patients are maintained on placebo and the crossover to active treatment is not randomized (or in some other way controlled), they may be (and often are) switched in response to a spontaneous change in clinical state. Any concurrent improvement or deterioration is then in part due to this clinical change.
In addition, change can be due to the cyclic nature of the disorder and not the effect of the drug. Other, nonpharmacological interventions may also be introduced. For example, if staff members become concerned about a patient, they may intervene with more intensive milieu, family or individual therapy, or the clinician may feel compelled to switch from placebo to drug.
CONCOMITANT MEDICATIONS
Avoidance of active concomitant medication is the next important requirement. Such medication constitutes a major artifact because it can markedly weaken the drug-placebo difference. Thus, compared treatments may appear equally efficacious due to the concomitant medication and not any inherent efficacy of the experimental agent. Some studies have used multiple agents, in different doses, with some known to be specifically effective for the disorder under investigation. For example, in some studies, comparing carbamazepine or valproate with placebo or lithium, patients have also received adjunctive antipsychotics, making firm conclusions difficult (see “Alternative Treatment Strategies” in Chapter 10).
Concomitant medications should not be confused with rescue medications. The latter are nonspecific agents (or potentially effective drugs given in subtherapeutic doses) provided so
patients can remain in the study for an adequate time, allowing for a valid comparison between the experimental agent and placebo (or standard drug). Often, rescue medications are used in the early phases and are decreased or eliminated before the critical evaluation at the end of the study. This enables more patients to complete the study (i.e., fewer dropouts), with the early impact of the rescue medication having at best only minimal effects on the final evaluations.
patients can remain in the study for an adequate time, allowing for a valid comparison between the experimental agent and placebo (or standard drug). Often, rescue medications are used in the early phases and are decreased or eliminated before the critical evaluation at the end of the study. This enables more patients to complete the study (i.e., fewer dropouts), with the early impact of the rescue medication having at best only minimal effects on the final evaluations.
When it is likely that a second drug used in a copharmacy strategy can produce a better effect than a single drug, all patients can receive the standard drug and also be randomized to a second drug, placebo or other comparator. Alternatively, designs can use placebo plus placebo, drug A plus placebo, drug B plus placebo, or A and B combined.
SUBJECT DROPOUTS
Usually a substantial proportion of patients drop out before studies are completed. Endpoint analysis uses the last observation made on a subject. Patients often gradually improve or worsen. Some drop from the study when they get so much better that they are discharged or it seems pointless to continue. Some deteriorate to a dangerous state and must be dropped for clinical reasons. In either case, reaching this point indicates that the drug is beneficial or not and is at least a qualitative endpoint. Last observation carried forward (LOCF), a standard method of data analysis, carries the last data point forward week by week. Random regression models estimate what would happen at a later time point, assuming that patients change in a linear fashion. Improvement, however, often levels off and “creating” data points based on questionable assumptions can also potentially introduce a bias.
ADEQUATE SAMPLE AND APPROPRIATE POPULATION
Equally critical to a properly designed study is sample adequacy (i.e., size and appropriateness). It is hard to make definitive conclusions with very small sample sizes (e.g., five per group) because variation is too great. The minimal sample size needed to make inferences also depends on how large the experimental drug-placebo effect size is (i.e., the larger the effect size, the smaller the sample needed).
The population studied should also be appropriate to the disorder. For example, in a study of antibiotics for pneumococcal pneumonia, the subject population should have this disease and not a viral pneumonitis.
Overly complicated entrance criteria may be counterproductive, in that patients who have a classic presentation may be excluded because they fail to meet one or more less-important criteria. This may result in too small a sample size and can lead to the inclusion of patients who technically fit the criteria but are clearly inappropriate. This problem is particularly true with an uncommon disorder and with patients who are difficult to enroll in clinical trials (e.g., acutely manic).
Another issue is subjects who volunteer for an advertised study. Undoubtedly, some will have the true disorder, but others, although responding to an advertisement, may only minimally meet symptom criteria and may not have spontaneously sought help otherwise. This situation is particularly apparent when the disorder approximates normal emotions or problems. Some symptomatic volunteers may include newly recognized classic cases, whereas patients referred to a tertiary referral center may include atypical, treatment-resistant individuals.
STANDARDIZED ASSESSMENTS
Reliable and valid rating instruments are important. Although a global assessment of clinical improvement is important, a valid rating scale can also qualitatively rate symptom change. In an open study, patients are often evaluated by the investigator’s global impression, an approach obviously subject to bias. The use of adequately normed and standardized quantitative scales to assess patients at baseline and during treatment provides an element of objectivity. A reliably trained rater using valid instruments anchored by clear operational definitions makes it much harder for bias to enter, even if the study is not double blind.
DATA ANALYSIS
The presentation of data and the statistical methods are two critical factors. The inclusion of baseline and final ratings on each patient from a standardized (or even a simple, global, semiquantitative) scale allows for useful comparisons
between those on active treatment or placebo. Even if formal analyses are not done, raw numbers can provide the clinician with a “feel” for what actually happened, whereas the mean change scores on some abstract scale may have little intuitive meaning to the clinician. It is best to have the data speak directly to the reader in an uncomplicated fashion, and such information should always be included.
between those on active treatment or placebo. Even if formal analyses are not done, raw numbers can provide the clinician with a “feel” for what actually happened, whereas the mean change scores on some abstract scale may have little intuitive meaning to the clinician. It is best to have the data speak directly to the reader in an uncomplicated fashion, and such information should always be included.
Equally important is the use of suitable, quantitative, statistical analyses, including more complicated models, because they can hold certain variables constant, control for artifacts, and provide supplementary information. Whatever statistics are used, they should be explicitly described in sufficient detail so the reader knows exactly what was done and can make a judgment about their appropriateness. For example, there are many different types of analyses of variance (ANOVA), analyses of covariance (ANCOVA), or multivariate analyses of variance (MANOVA), and some may not be appropriate to the task at hand. If only the results of an ANOVA with a p < 0.001 (p is an estimate of the probability that the results occurred by chance) are provided, the reader should be justifiably dubious because this model may not be proper. In addition, overly complicated statistics can introduce considerable bias into a study. When such models are used, they should be supplemented with raw data or simple, easily understood statistics.
ADEQUATE MEDICATION DOSE
It is important that subjects receive adequate doses of the experimental and (when present) comparator medications to maximize the potential differences from placebo. Further, this can also help determine the relative benefit of either agent in comparison with placebo. Often, however, such trials use the recommended doses even though clinical experience may dictate otherwise.
ACTIVE CONTROL GROUPS
In addition to a placebo group, an important method in experimental science is the use of an active control group. The importance of having a standard drug is to show that the measurement system is working and to assess how well it is working. If the new drug is equal to the standard drug and both are clearly better than placebo, then the experiment is valid. If the new drug is equal to the standard drug and both are equal to placebo, then the experiment did not work (given that the standard is known to be effective). This is called a failed clinical trial. However, it is sometimes incorrectly interpreted to support the assertion that the new drug is ineffective. For example, a large study found St. John’s wort and a standard antidepressant were no more effective than placebo (5). If it was a valid trial, the standard antidepressant should have been more effective than placebo.
Classifying Study Designs
Several features should be considered when classifying study designs by their quality. Our classification is intended as a device to focus on the important criteria. As noted in Table 3-1, a class Istudy satisfies at least the first 10 of the 11 criteria listed.
The use of an active control drug is an important factor that enhances the value of a study. A class II study satisfies at least 6 of the 11 criteria. For example, a single-blind study introduces more bias, but if the other criteria (e.g., random assignment, parallel groups) are met, then the data may still be valid. An AB design with no randomization or statistical analyses may still have many excellent features. A mirror-image design, such as Baastrup and Schou’s study (6) of the prophylactic effects of lithium, would be a good example (see “Maintenance/Prophylaxis” in Chapter 10). Such studies may have many elements of a better-controlled design, including
Patients have a classic presentation
Objective, quantifiable, and meaningful measures are used to evaluate important clinical factors
An adequate sample is used
There is a sufficiently long period of observation
A class III study is one that meets at least 5 of the
II criteria. Although these studies have some important elements of a controlled trial, many aspects are uncontrolled. Because a bias can exist, however, does not mean it invalidates the result, only that it may. Since every question cannot be answered by a class I design for reasons of practicality or cost, class II and class III studies are very useful to at least partially resolve questions that would not otherwise be addressed.
An example of a class III study is the ABA design. A variable-length placebo lead-in period, drug period, and postdrug placebo period are suspect, however, because many nonrandom variables can influence their length. The choice of when to start an active drug may correspond to a worsening of the patient’s condition, while the choice to stop treatment may foreshadow discharge with its own stresses. Such nonrandom events constitute major artifacts. With such a design, the staff can guess early and late in the hospitalization that patients are on placebo and that they are on active treatment in the middle of the study, making the blind more illusionary than real.
Although there are many confounds with such a design, it does provide important information about whether a patient relapses when switched to placebo after active treatment. It is not possible to do a meaningful statistical analysis on an ABA design because there is no control group for comparison. The fact that some patients improve more on a drug in period B than in the placebo period A may be a factor of time or rater bias. Because there is no control group, one cannot say that this improvement is better than what would occur in the natural course of the illness. Relapse in the second placebo period, however, can provide some information.
Although ABA designs are only marginally better than open trials, they may be relevant to another scientific question (i.e., once the disease process is “turned off,” will patients relapse when placebo is substituted?). For most psychotropics, we do not know whether relapse will occur if a drug is stopped shortly after achieving remission. Since the active disease has only been suppressed, relapse is more likely after discontinuation.
A mirror-image study (i.e., a design in which the time period on a new treatment is compared retrospectively with a similar time period without the new therapy) is often more like the “real world” of clinical practice, and hence results may be easier to generalize. The bias in mirror-image studies, however, comes from nonrandom assignment and the absence of a blind. Because the control period occurred in a prior time segment, other variables could have changed in the interim. Without blinding, there is also no way to avoid the possible bias of an evaluator’s enthusiasm for a given treatment. Careful assessment by objective measurements can attenuate this bias.
More recently, large sample effectiveness studies were conducted to assess the usefulness of various treatments in more typical clinical settings. These designs are hybrids using components of both controlled and naturalistic trials. (See Chapters 5, 7, and 10).
Less Definitive Designs
Uncontrolled open studies are the most biased, with concomitant medication the source of greatest error. For example, a patient started on drug A who fails to immediately respond then has drug B added, but drug A could have a delayed effect on the patient, which is falsely attributed to the addition of drug B. Some case reports may attribute coincidental events to a specific drug. Thus, the critical reader should always clarify the role of concomitant medication as an artifact. Sometimes, clinical myths can develop from several case reports on the efficacy of a specific drug when all patients were also on concomitant medication. Rare side effects can also be identified in case reports, but the writer should always be wary of coincidence.
Open designs can differ dramatically in their quality. Reports are often published on a variety of patients given different concomitant medications, diagnosed without the use of inclusion or exclusion criteria, and with outcome determined by the clinical investigator’s opinion, based only on memory. By contrast, other studies include specified diagnostic criteria, patients who are excellent examples of the disorder under study, only one treatment, and quantitative and concurrent evaluations. Often the most important ingredient in an open study is the investigator’s clinical judgment, which is, in fact, the measuring instrument. Although a more clinically experienced investigator may remain unbiased, those with less experience may unknowingly err in this regard. Open designs that incorporate quantitative evaluation of the medical record are superior to those that rely on clinician recall. We feel that a good open study can be better than a small-sample ABA study.
Systematic case-control studies (e.g., a nonrandomized control group) can also provide useful information, but unfortunately they are rarely used in psychopharmacology research. In situations when uncommon conditions or those that pose an imminent danger to the patient (e.g., neuroleptic malignant syndrome) make it impossible to conduct prospective, controlled trials, case-control methodology can provide some degree of rigor. Because these studies do not
include random assignment, however, the reported outcome can be substantially biased.
include random assignment, however, the reported outcome can be substantially biased.
Good observational case-controlled or cohort studies can make reasonably accurate estimates. For example, comparisons of observational and randomized studies by Benson and Hartz (7) and Concato et al. (8) found that similar results were obtained by both methods. It is unusual, however, to have the same treatment investigated by both methodologies.
Finally, early in the investigation of the effects of a drug, it is important to clarify which conditions benefit and which do not. For example, the efficacy of imipramine for depression was discovered after it was developed for the treatment of schizophrenia. Other examples include the use of imipramine for panic attacks and the efficacy of clomipramine for obsessive-compulsive disorder (OCD). Because we cannot conduct class I, II, or III studies addressing all possible variables, good open studies can provide valuable information.
CONCLUSION
We apply our classification of study designs throughout the text to help the clinician interpret the quality of results from clinical trials. Further, we give the critical reader a perspective on the depth and validity of the available data. Most studies in the analyses provided are class I or II designs.
Statistical Summarization of Drug Studies
Meta-analysis is a statistical method that combines data from individual drug studies to obtain a quantitative summary of their results (9). This statistical approach includes
The overall effect (i.e., how effective is a drug)
The probability that this overall effect is statistically significant
The statistical confidence limits on the overall effect
The extent of variability among all studies as well as the degree to which it is accounted for by discrepant results from a small fraction of the total number of studies
The possible effects of methodological or substantive variables that could alter the outcome
When helpful, meta-analyses are computed or provided to summarize the overall effects from controlled clinical trials. These analyses use this data to compute an effect size and calculate the probability that a given drug is different from placebo and equivalent to or more effective than standard drug treatments. The goal is to estimate the extent of clinical improvement with a specific treatment as an aid to therapeutic decision making. Meta-analysis can complement a narrative review and often accompanies a literature summary (10).In one sense, a metaanalysis can be seen as a quantitative literature review using a more explicit and structured approach.
Unfortunately, efficacy is often assumed on the basis of clinical lore or by uncritically accepting the results of a few studies. An article may review several highly publicized references to support a certain position, but the careful reader may find that many of the studies quoted are poorly controlled or report duplicate data. A good example was the literature on clonazepam as a treatment for acute mania. Many review articles quote numerous references to support its efficacy, but a careful scrutiny of the literature revealed only one small, controlled study, the interpretation of which was limited by the use of active concomitant medication (see “Lithium Plus Benzodiazepines” in Chapter 10). Ideally, to make an informed judgment about a new drug, one should critically consider each individual study before drawing any conclusions. Although the number of uncontrolled trials vastly exceeds their better-designed counterparts, an increasing number of controlled reports are now being published. Meta-analysis can provide a systematic estimate of the merit of these data.
OMNIBUS METHODS VERSUS META-ANALYSIS
Meta-analysis is not simply counting the number of studies that find a significant difference or taking an average of their mean improvement. Hedges and Olkin (11) refer to such statistical models as omnibus or “vote-counting methods,” noting that they have a number of methodological problems. For example, they do not weigh studies according to the sample size. Furthermore, such methods only calculate one statistical parameter, indicating the probability that the studies considered together show a
statistically significant difference. As a result, they can be overly influenced by one or a few disparate studies.
statistically significant difference. As a result, they can be overly influenced by one or a few disparate studies.
An important difference between such methods and meta-analysis is the ability to clarify whether all studies included show a consistent effect size (i.e., estimate homogeneity). For example, if a few studies find a large difference and the majority none, an omnibus method might still conclude a statistically significant difference. The appropriate conclusion, however, is that the results across studies are highly inconsistent. Thus, with omnibus methods, errors in a few small studies can disproportionately contribute to the final results, a phenomenon that Gibbons et al. (12) have illustrated with simulations.
One of the major purposes of meta-analysis is to demonstrate whether findings are consistently and clearly statistically significant when studies are combined. When there is a consistent finding with some studies significant and others having strong trends, a box score method may misleadingly show some positive and some negative outcomes. Often, large studies are clearly positive, but some of the smaller, ostensibly negative studies may actually show a strong trend that does not reach statistical significance due to their limited sample size. Typically, when multiple studies have the same outcome, the results of a metaanalysis will be highly statistically significant. By contrast, p values of 0.05 or 0.01 are very difficult to interpret because an artifact from a single study could produce such “nonsignificant” significant levels.
META-ANALYTIC STATISTICAL METHOD
In preparing such analyses, investigators usually conduct a computer-assisted literature search for all studies on a given psychotropic, review the bibliography of each report to identify other pertinent articles, and obtain translations of the relevant non-English language articles whenever possible. All double-blind, random-assignment studies in the world literature that tested a given drug against placebo or other standard agents should be systematically identified. Next, standard techniques such as recommended by Hedges and Olkin (11) for continuous data or the Mantel-Haenszel model for discontinuous data are used (13). Because continuous data are more statistically powerful than discrete data, the former are preferentially used to derive the effect size.
Frequently, the sample size (N), mean (x), and standard deviations (SDs) are extracted as well as how many patients had a good or poor response by deriving a standard cutoff point to separate responders from nonresponders. When a semiquantitative scale is provided, patients with moderate improvement or more can be classified as “responders” and those with minimal improvement, no change, or worse as “nonresponders.” For most medication studies, the majority of patients on placebo are usually rated only minimally improved. Thus, this level of change is usually an appropriate choice for a cutoff point to distinguish drug versus placebo differences. We note here the importance of having an a priori working definition of response threshold because choosing the best cutoff point in each individual study would bias the outcome (13).
Graphic Inspection of Results
The essence of meta-analysis is inspection of the data. Thus, this approach produces a visual or numerical representation of each study in the context of all the others. A review of the actual data gives the critical reader a feel for the data as well as an index of suspicion if there is undue variability, which is far more important than any statistical parameter.
Studies in the literature often present a wide variety of data obtained with different rating scales, measuring instruments, and statistical techniques, which makes it difficult to compare results expressed in a wide variety of units. In statistics, actual scores are often converted to standardized scores by subtracting a given value for each subject from the mean and dividing the result by the SD. This creates a new value in Z score units, with a mean of 0 and a SD of 1 (i.e., standard scores). In meta-analysis, the mean of the control group is subtracted from the mean of the experimental group and divided by the pooled group SD, a process similar to the concept of percent change score. Thus, data are expressed in uniform units rather than in actual raw score means and SDs, which often vary substantially between studies.
With meta-analysis, if a given study is discrepant (e.g., has a high placebo response rate or an unusually high drug efficacy rate), it will stand out. This information can be expressed graphically,
using Z units derived from effect sizes, or percent response versus percent nonresponse, or the odds ratio (a statistical term used as an alternate to chi square). The reader can then note whether the finding is similar in all studies, or conversely, whether there is a big effect in some but not others.
using Z units derived from effect sizes, or percent response versus percent nonresponse, or the odds ratio (a statistical term used as an alternate to chi square). The reader can then note whether the finding is similar in all studies, or conversely, whether there is a big effect in some but not others.
In essence, meta-analysis abstracts results from each study and expresses them in a common unit so that one can easily compare.This allows one to focus on the hypothesis under examination rather than be distracted by the myriad differences among studies.
When the results from several studies are converted into similar units, a simple inspection of a graph or table readily reveals which studies have different outcomes from the majority. Such discrepancies can also be examined by a variety of statistical indices. For example, one can calculate a statistical index of homogeneity, remove the most discrepant study, and recalculate, revealing that all but one study is homogenous. If two studies are discrepant, one could remove both and again reexamine the indices of homogeneity, and so on. For an example, we summarize the relative efficacy of unilateral nondominant versus bilateral electrode placement for the administration of electroconvulsive therapy (ECT). Here, 10 studies had one result and two others a different outcome (see Tables 8-5 and 8-6).
Effect Size
Effect size defines the magnitude of the difference between the experimental and the control groups regardless of sample size.This is quite different from the statistical significance, which is the probability that such a finding may occur by chance, leading to rejection of the null hypothesis. Statistical significance is determined in part by the sample size, so studies with a large number of subjects may find a highly significant result. In contrast, effect size is independent of sample size. Thus, in a six-person study, if two of three patients benefit from an antipsychotic and one of three improves on placebo, this result would not be statistically significant. But, if 200 of 300 patients benefit from an antipsychotic, while only 100 of 300 benefit from placebo, this would be highly statistically significant. Although the effect size (i.e., 67% on drug and 33% on placebo improving) is the same in both studies, only the results of the second study are clearly statistically significant because of its larger sample size.
The effect size of a continuous variable is commonly expressed as the difference between the mean of the experimental minus the mean of the control group divided by the pooled SD. For example, data from the National Institute of Mental Health Collaborative Study demonstrated that antipsychotic-treated patients averaged a 4.2-point increase on a 6-point improvement scale, whereas the placebo patients averaged only a 2.2-point increase (i.e., an average difference of 2 points). The SD of these data was approximately 1.7, so in effect size units, the improvement was approximately 1.2 (i.e., 2.0/1.7) SD units. For discontinuous data, the effect size for a drug-placebo comparison is usually expressed as the difference between the percent improvement with the experimental drug and the percent improvement with placebo.
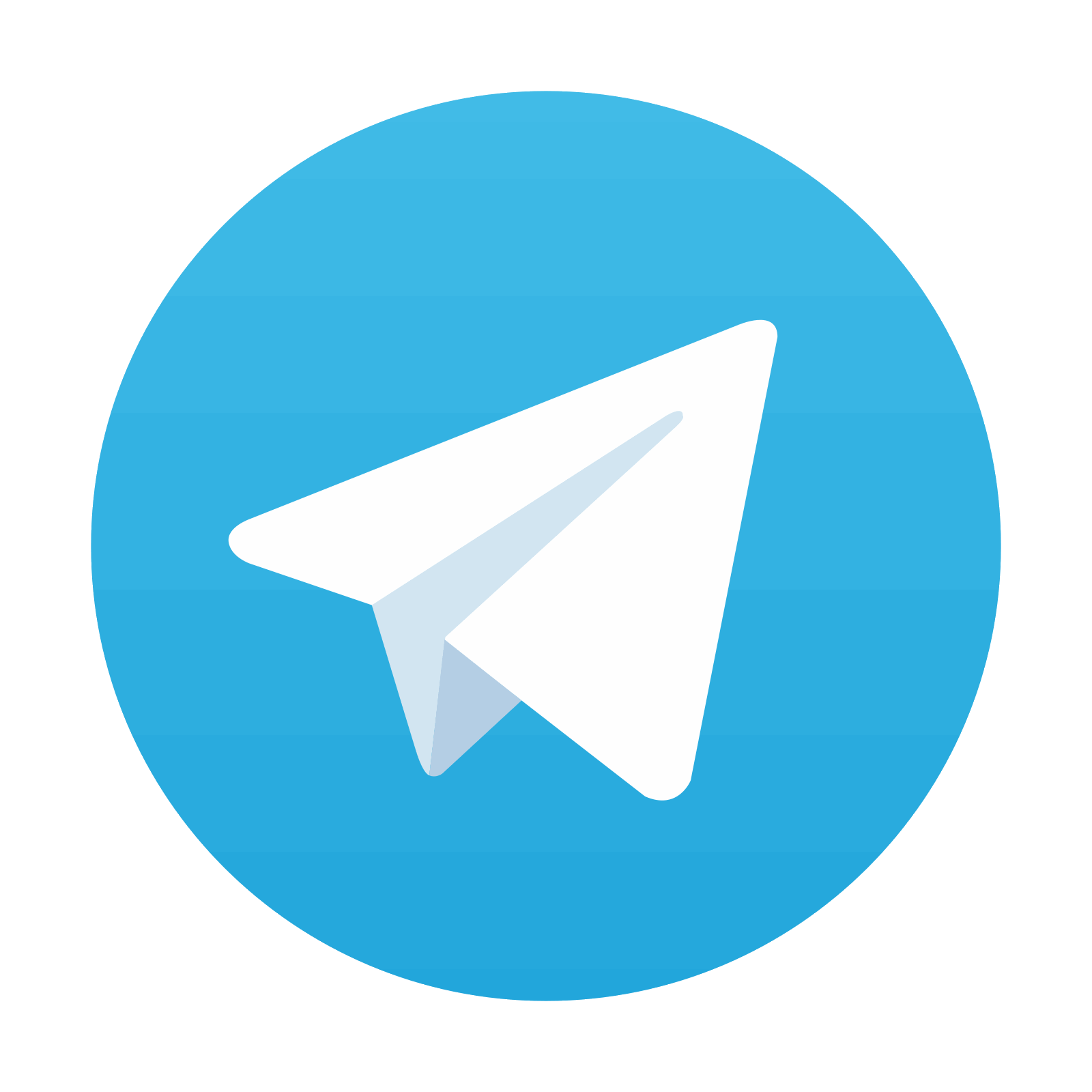
Stay updated, free articles. Join our Telegram channel

Full access? Get Clinical Tree
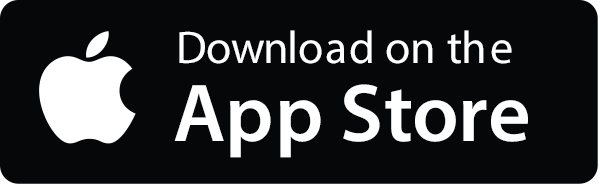

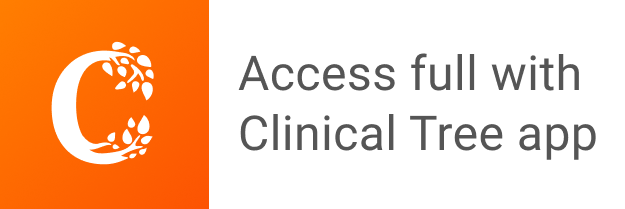