“Lies, damned lies, and statistics with biases.”
24.1 Incidence and Prevalence
Number of “new” cases/number of people at risk of acquiring that disease | Total number of cases (new + old)/whole population | |
Acute cases, e.g., incidence of Zika virus infection in August | Chronic cases only,a e.g., prevalence of diabetes in United States | |
aIt does not make sense to calculate prevalence of acute disease. |
Attack rate is a special type of incidence rate helpful in outbreaks.
Attack rate = Number of new cases in a population at risk/number of population at risk.
For example, 100 people were eating at a Chinese buffet, and 25 people got diarrhea = the attack rate is 25%.
24.2 Defining Characteristics of a New Diagnostic Test
24.2.1 Sensitivity, Specificity, Positive Predictive Value (PPV), and Negative Predictive Value (NPV)
Patients with true diseasea | Patients without diseasea | |||
TP/(TP + FP) = TP/(total number of patients with positive result) = PPVb | ||||
TN/(TN + FN) = TN/ (total number of patients with negative result) = NPVc | ||||
TP/(TP + FN) = TP/(total number of patients with disease) = sensitivityd | TN/(TN + FP) = TN/(total number of patients without disease) = specificitye | |||
aTypically, new diagnostic tests are compared with gold standard tests, which have the highest sensitivity and specificity. | ||||
bTest question commonly asks PPV in this manner: “If the test is positive, how likely does the patient truly has the disease?” 2 PPV and NPV: statistical measures of a test’s ability to identify truly positive (PPV) and truly negative (NPV) states. | ||||
cIf the test is negative, how likely is that the patient does not have the disease?2 | ||||
dMRS: Truly sick people are sensitive. So, sensitivity is a ratio that involves only the patients with the disease (true positive and false negative). | ||||
eMRS: Truly healthy minds are very specific in what they want to do. |
For all the following calculations, the “TRUE” value is always the numerator, and the denominator is the sum of “TRUE” and “FALSE” values.
To answer most of the following questions, we recommend drawing the two by two table given on previous page. Practice creating the table again and again until you are very good at it.
A test developed for diagnosing lung cancer has a sensitivity of 80%. There are 100 patients who actually have lung cancer in the study and 300 who do not. Calculate the total number of false negatives?
A 60-year-old man requests fecal occult blood (FOB) testing for colon cancer screening as he does not want to do colonoscopy. He reports that he has been eating barbecued meat, processed food, and bacon at least two times per day all his life. A detailed artificial intelligence software analysis reveals that patients with similar baseline characteristics have a 10% prevalence of colon cancer. FOB comes back positive. Studies have reported that FOB has a sensitivity of 80% and a specificity of 70%. What is the likelihood that this patient really has colon cancer?
If FOB had come back negative, what is the likelihood that the patient does not have colon cancer?
What is the accuracy of FOB in patients with these baseline characteristics?
What happens to NPV of a test as prevalence increases?
When a test with 12% NPV comes back negative, what are the chances that the patient truly has the disease?
24.2.2 Effects of Using Different Cut-Off Values
Let’s say that we have created a test for diagnosis and that the test result has a numerical value. When we plot the frequency distribution of results obtained from general population, this will generally produce a bell-shaped (symmetric) distribution curve. Examples of such tests include fasting blood glucose, HbA1C, serum cholesterol, etc.
In the following graphs, fasting blood sugar cut-off is set at 126: > 126 mg/dL is diabetes and < 126 is not diabetes.
We can use different cut-offs for the test to serve different purposes, but we need to know what happens to test characteristics when we change the cut-off:
24.2.3 The ROC Curve (Receiver Operating Characteristic Curve)
In this graph the true positive fraction/rate (TP/TP+FN= sensitivity) is plotted against the false positive rate (100-Specificity).
The best test is plotted in the upper left corner (100% sensitivity and 100% specificity). Note, the horizontal axis is not specificity but 100-specificity.
Accuracy of the test is measured by the area under the curve. The closer the ROC curve is to the upper left corner, the higher is the overall accuracy of the test.
In the above example the red-line test has better accuracy than the dotted-line test.
SEN = Sensitivity moves with the Negative predictive value. When you are lowering the cut-off point, you are including more patients with disease, thus false negative rate decreases. So, when the test is negative, it is more likely to be truly negative. But as we try to increase the sensitivity of the test, we lose the specificity.
SPE = Specificity moves with the Positive predictive value. When you increase the cutoff point, you are making sure that you only get patients with the disease, thus the false positive rate decreases. So, when the test is positive, it is more likely to be truly positive.
But as we try to increase the specificity of the test, we lose the sensitivity.
24.3 Different Types of Statistical Studies
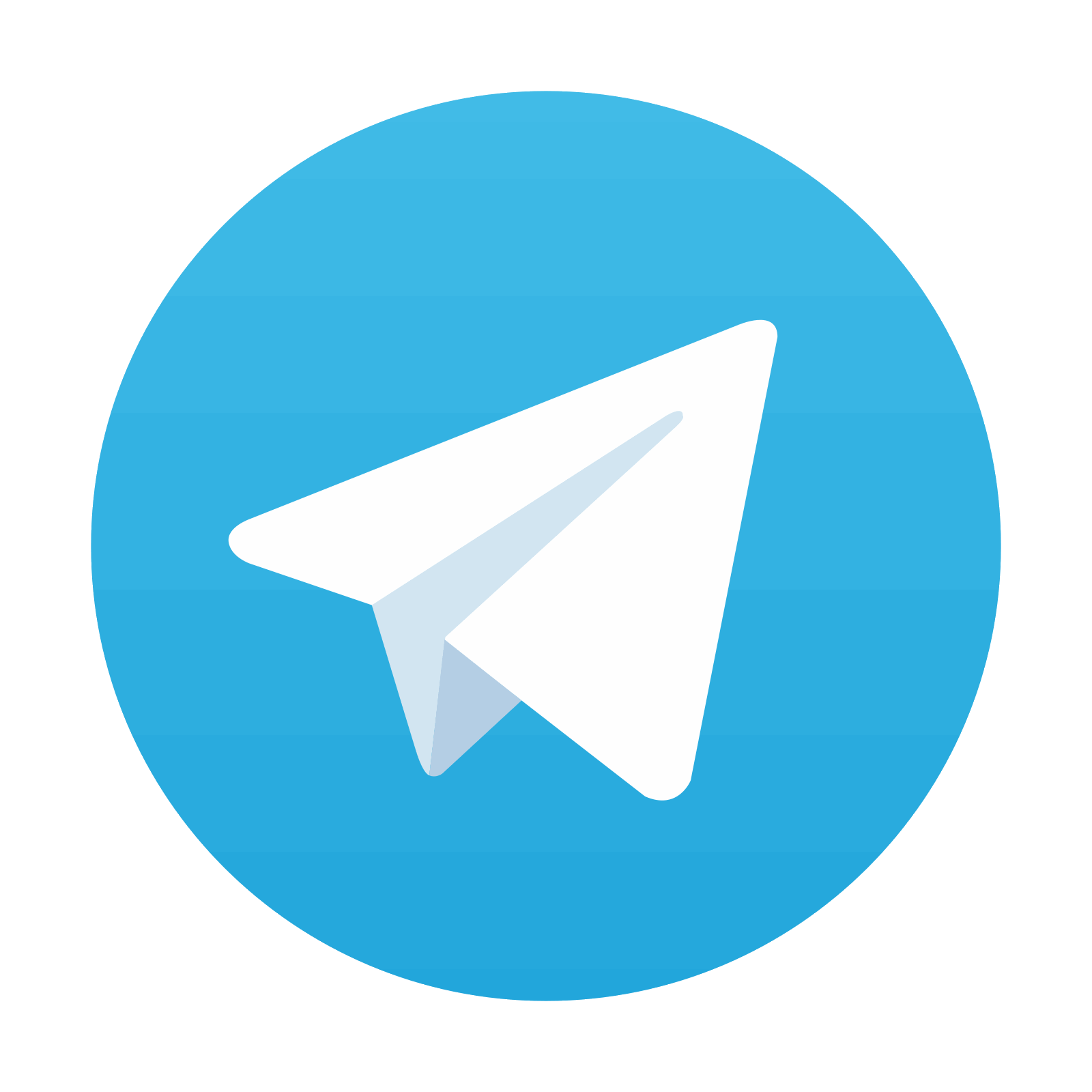
Stay updated, free articles. Join our Telegram channel

Full access? Get Clinical Tree
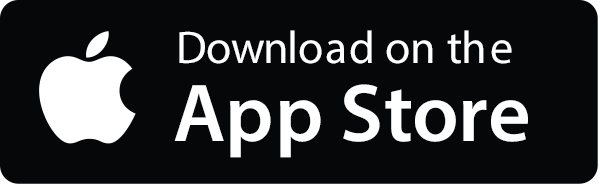
