Introduction
This chapter will look at the present state and challenges of integrating genomic test results and genomic decision support (GDS) into an electronic health record (EHR). The fundamental premise of this chapter is that EHRs are becoming increasingly prevalent and that any intervention that seeks to change clinical outcomes will have to do so through the EHR. Where possible in this chapter, the present state and challenges will be compared and contrasted in commercial and internally developed EHRs. Commercial EHRs while significantly more prevalent may have initially less flexibility to accept genomic test results and to provide GDS especially in the age of regulated meaningful use. The meaningful use of EHRs is defined by the CMS EHR incentive program funded through the HITECH acts as part of the American Recovery and Reinvestment Act of 2009. For purposes of this chapter, we define GDS as a form of computerized clinical decision support or clinical decision support system (CDSS). This chapter will also examine the present state of and challenges with usability in regards to genomic test results and genomic CDS.
Opportunities and Need for Genomic Decision Support
Until recently, the use of genetic and genomic information was largely restricted to diagnostic guidance or esoteric treatment guidance, in which the only consumer of the information is an expert in that field (eg, oncology, genetic medicine for rare diseases). One of the earliest examples of implementation of genetic medicine is with azathioprine and thiopurine methyltransferase (TPMT) activity, which can be tested through genetics as well as enzyme activity. However, a recent growing body of evidence points to common medications as targets for genomic guidance, including clopidogrel, warfarin, and simvastatin. Each of these has been a top grossing medication worldwide within the last decade, and can be commonly prescribed by a diverse group of practitioners, including specialists and primary care physicians. Indeed, the body of genetic evidence is growing, and, as of first-quarter 2012, the US Food and Drug Administration lists pharmacogenetic information in the structured product labels of 99 medications. The future of personalized medicine envisions a time in which many medications have potential pharmacogenetic influences. Given a growing list of medications with pharmacogenetic influences and more individuals exposed to potential prescribing conditions, there is a need to develop and maintain genomic CDS that will enable easy access to the current evidence-based practices for safe and effective prescribing. Indeed, one can argue that the diversity and complexity of genomic testing and results is an ideal candidate for CDS for several reasons: (1) the complexity is difficult for a broad community to maintain, (2) proper interpretation of genetic test results requires knowledge of specific allele changes and combinations that currently are not expressed in self-evident nomenclature (eg, CYP2C19*2 represents a poor metabolizing variants while CYP2C19*17 is a hypermetabolizing variant), (3) the ability to incorporate, within CDS, drug-genome, drug-drug, and drug-drug-genome interactions to a level of complexity not easily synthesized by a human, and (4) the field changes rapidly.
Genomics and Clinical Decision Support
Portions of the section on genomic and CDS were adapted from Hoffman and Williams, 2011.
CDS refers broadly to providing clinicians and/or patients with clinical knowledge and patient-related information, intelligently filtered, or presented at appropriate times, to enhance patient care. CDS does not require a computer or EHR and is simply anything that supports a clinical decision. A CDSS refers to computerization of CDS, where the CDS can be viewed as the content paired with a computerized delivery system. A CDSS incorporates patient- specific data, a rules engine, and a medical knowledge base to produce a patient-specific assessment or recommendation for clinicians. CDS can be grouped in three general categories: passive, asynchronous, and active.
Passive decision support consists of nonmandatory resources available at the time of care in the EHR. Two examples of passive decision support are electronic resources (E-resources) and context specific.
E-resources are electronic content collections within the patient record. These E-resources can contain external collections, links to the system’s medical libraries, and patient education resources, internally developed care guidelines, protocols, laboratory, and formulary information. Links to these resources take the user to the resource home page where a search query can begin. Resources are queried until an answer is found adding to the time needed to find an answer. This is a significant issue as Levy and colleagues (2008) found that busy clinicians want a resource that provides an answer within 2 to 3 minutes of search initiation. These authors also found issues with accuracy and completeness of genomics content in the commonly used online resources.
To decrease the time needed to find answers to clinical questions, informaticists realized that where the clinician is navigating in the patient EHR could provide context for the question being asked allowing “presearch” of content libraries. This presents the clinician with a filtered set of resources that minimize search time. This approach can be called context-specific passive decision support (eg, InfoButtons). InfoButtons or equivalent capabilities appear in locations relevant to the most commonly asked questions–the problem list, laboratory results and medication list (including medication ordering). The preferred content resource can be changed based on a determination by the knowledge managers about which resource is most likely to provide the best answer for a given question. In the case of an InfoButton in the problem list associated with the problem “Diabetes” the preferred content could be internally developed best practice guidelines of the specific healthcare system, or a professional society. For genetic conditions such as Marfan syndrome, the preferred content could be Genetics Home Reference (a concise, simplified resource) or GeneReviews (more complete and detailed information but tailored more for genetics professionals). Genomic content experts could create content specific to best care practices for the system.
Passive decision support can facilitate the clinician’s access to information needed to answer a clinical question, but requires the clinician to recognize that a clinical question exists. The remaining types of CDS are the most amenable to computerization. For the purposes of this chapter CDSS and asynchronous and active decision support will be used interchangeably. Active and asynchronous CDS are designed to provide the clinician with information he/she needs to know but was never requested.
Active (or synchronous) CDS describes a workflow in which a clinician process, such as prescribing a medication, is monitored in real time by rules of logic and clinician behavior is influenced based on the logic embedded in a rule. The most widely recognized approach is pop-up alert windows warning the user of a potentially risky decision, such as an allergy or drug-drug interaction (DDI). Other modalities are gaining favor (to avoid “alert fatigue”), including workflow logic in which warnings and alerts are embedded into the user navigation through the EHR application. For example the contents of an application window may vary based on genomic risks or additional screening procedures may be added to order lists.
Pharmacogenomic testing for the drug carbamazepine (Tegretol) demonstrates how active CDS can work. It is known that patients who carry a specific human leukocyte antigen (HLA) type (B*1502) are at increased risk for an adverse drug reaction. If a clinician uses a computerized medication order system and chooses carbamazepine an algorithm is run represented by the logic model in Fig. 183-1. The information in the left hand box is automatically collected by the program from various parts of the electronic data warehouse (EDW) (Table 183-1).
Information Needed | Reason Needed |
---|---|
Age | Dosing algorithm |
Weight | Dosing algorithm |
Sex | Risk for pregnant women |
Pregnant? (if female) | Risk for pregnant women |
Clinical indication | Generate list of appropriate alternative medications |
Ethnicity | Population risk |
Drug allergies | Prior history of reaction |
Interacting medications | Dosing and safety |
In this case the indication is psychomotor epilepsy. If the patient is female over a predetermined age and the drug presents a risk to a fetus the clinician would be prompted to order a pregnancy test. Ethnicity is requested because HLA-B*1502 has a significantly higher prevalence in many Asian populations. If testing has been done previously and the patient carries the HLA-B*1502 allele, the clinician sees the alert represented in the right hand box of Fig. 183-1. If the InfoButton is clicked (this is the blue circle with the white “i”) the clinician is taken to the FDA alert’s abstract. There is also a link to alternative medications. If this is clicked, the system will look at the indication for treatment and other factors and would propose clonazepam as the recommended alternative, given its equivalence to carbamazepine in the treatment of psychomotor seizures. Alternatively, if the HLA-B*1502 test had not been done previously the clinician would be alerted to order this test before prescribing carbamazepine. If the patient was not of Asian ethnicity, the query about the HLA-B*1502 would not have triggered and the prescribing would have proceeded with consideration of the other factors.
A unique aspect of germline genetic or genomic testing is that the test only needs to be done once in a patient’s lifetime. An article by Riegert-Johnson et al. identified a significant issue with genetic tests being repeated, resulting in wasted resources. An examination at Intermountain Healthcare looking at two thrombophilia tests showed that roughly 5% of these tests represented duplicates (Williams et al., unpublished data). When additional data were examined a small number of clinicians were identified that were responsible for a disproportionate number of these duplicate tests. CDSS could be used to alert and educate the clinicians that such testing is needlessly duplicative. Changes in behavior can be monitored prospectively to ensure that test ordering behavior has changed.
Asynchronous CDS involves the system monitoring behaviors, perhaps assembling data that was not completely available at the time of clinician or patient interaction and comparing this to rules or algorithms crafted to identify opportunities to improve clinical care, recognize patient safety issues, or close targeted care gaps. The output of asynchronous CDS can be messages to the clinician generally within the EHR at a time the clinician is not expecting the message nor seeing the patient. Several pregenomics and pre-HIPAA studies have successfully sent results via alphanumeric paging. When celecoxib (Celebrex) was removed from the market it was important to identify and contact patients taking the medication, a nearly impossible task for a clinician using paper records. With EHRs, CDSS could message each physician with target population patients and potentially contact patients directly through a personal health record (PHR). Asynchronous CDS can deliver monthly dashboards about a clinician’s patient population with a given disease, such as diabetes presenting information such as hemoglobin A1c, eye examinations, medications, etc. This allows clinicians to take actions such as recalling the patient, placing orders etc. Care gaps can be tracked over time guide for further action.
Genomic data may purport to multiple opportunities for asynchronous CDS. Current and future programs in genomic testing may test multiple variants simultaneously. Examples will be described below.
Challenges to the Implementation of Genomic Decision Support
Previously we defined GDS as a form of computerized clinical decision support or CDSS. In order to move toward GDS, EHR systems will require a number of enhancements. The legacy of commercial EHRs traces back to early needs to better support administrative transactions. These transaction-oriented systems were not designed to enhance the care of individual patients. Some internally developed EHR suppliers began to take a person-centric approach to their system architecture in the 1980s to 1990s. This shift enabled users to more readily assemble an accurate picture of diagnostic and therapeutic events associated with a specific patient allowing contributions from EHRs to the individualization of care.
EHR developers are actively assessing innovations needed to ensure that EHR systems can fully support the integration of genetic information into patient care. There are distinct categories of EHR developers. (1) The academic or large private institution model with an EHR highly tailored to the unique needs of the institution, sometimes through a ground-up design of a unique system. EHRs developed in this model have the benefit of agility and build as needed, although resources needed for support are significant. Successful strategies developed in these contexts often prove difficult to deploy more widely because of the site-specific customization. (2) EHRs developed through a commercial model for installation in a wide variety of settings. These systems must be flexible enough to meet the needs of a wide variety of organizations, must ensure that they meet the legal and regulatory requirements of a system that is distributed commercially and must enable information exchange of a subset of their data elements across platforms at the sacrifice of customization for institutional workflows. Regardless of the development model, EHR developers have realized that improvements in foundational capabilities will enhance the ability to better support personalized medicine.
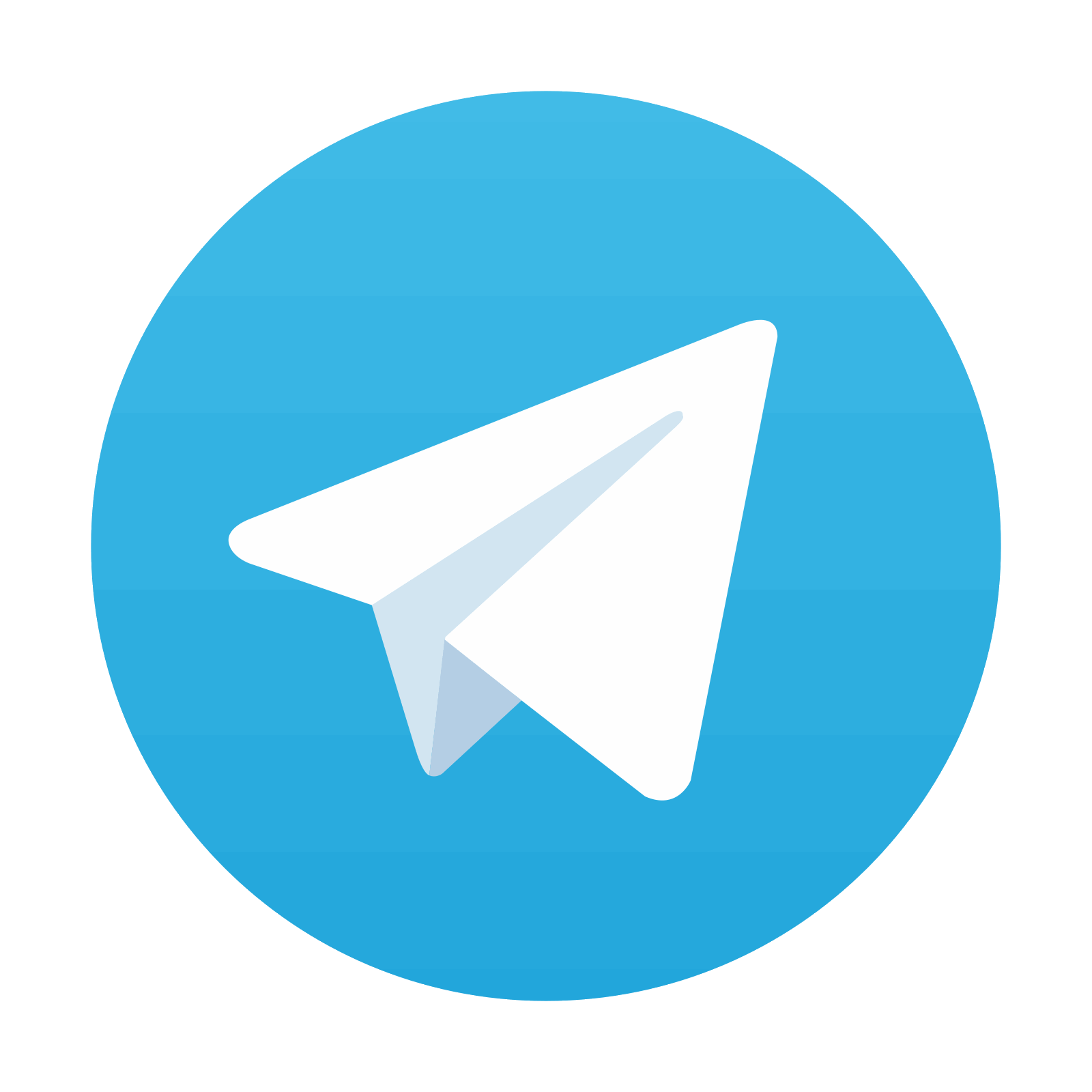
Stay updated, free articles. Join our Telegram channel

Full access? Get Clinical Tree
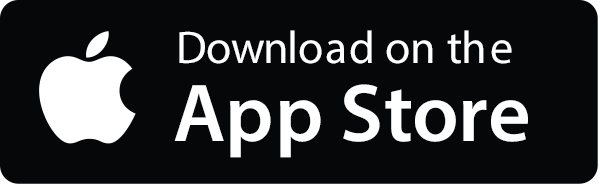
