Key Points
Overview:
The past 5 years have been a time of tremendous progress in our understanding of the genetic basis of polygenic traits, with over 1100 genomic loci having been associated with over 165 complex (ie, polygenic) traits. While this has led to huge advances in our understanding of the mechanisms of disease, the clinical application of this information to risk prediction has been more limited. The reasons for this are outlined here. Since a detailed discussion of genetic risk for every disease is beyond the scope of this chapter, we focus on a few illustrative examples.
Genome-wide association studies (GWAS) have dramatically increased our understanding of the mechanisms of common human diseases.
Applying these results to clinical medicine is difficult due to both incomplete heritability and an incomplete understanding of the genetic basis of human disease.
Further studies are needed to better understand how genetically based risk prediction can be effectively applied to the management of human diseases. Studies are also needed that address the ethics of genetic disclosure and its psychologic effects on patients.
Basic Definitions and Principles
Monogenic traits are those which are determined by a single gene. Examples include diseases such as cystic fibrosis and Duchenne muscular dystrophy, as well as benign traits such as having attached earlobes. Polygenic traits, in contrast, are the result of many genes. Examples again include both diseases such as type 2 diabetes mellitus, as well as nondisease traits such as stature. Note that polygenic traits, although frequently quantitative (eg, blood pressure or low-density lipoprotein [LDL] cholesterol), can also be binary (eg, coronary artery disease or schizophrenia). For nonquantitative polygenic traits, a threshold model is frequently used to explain modes of inheritance.
In its most simplistic formulation, the variation of any trait can be partitioned into a genetic component (G) and an environmental component (E). Heritability refers to the proportion of trait variation that can be attributed to genetic variation (ie, G/[G + E]), and its value ranges from 0 (ie, no genetic contribution) to 1 (completely genetic). Although the variation of a trait can be directly measured, the heritability must be indirectly inferred. There are many methods for this, but one of the most common is through the comparison of monozygotic and dizygotic twins; if the correlation for the trait among monozygotic twins is higher than that among dizygotic twins, then the trait is more heritable (the interested reader is encouraged to refer to Genetics and Analysis of Quantitative Traits for a further discussion of this method, which is known as Falconer’s formula).
A new method developed in recent years to identify genetic variants that increase or decrease the likelihood of a polygenic trait. In a typical GWAS, one begins with a collection of individuals with a given trait (such as coronary artery disease), as well as a collection of controls. In each individual from the case and control populations, a large number of genetic variants (typically on the order of 1 million) throughout the genome are assayed, and those variants that are most over- or under-represented among cases are determined. Thus, a GWAS is a type of case-control study that seeks to discover genetic variants associated with disease.
The fundamental unit for reporting the effect size of a genetic variant on a trait. It expresses the proportion of individuals in the case group who carry a given allele relative to the proportion in the control group. When the odds ratio (OR) is greater than 1, a given allele is more frequent in the case group; conversely, when the OR is less than 1, it is more common in the control group.
Prior to 2005, fewer than a dozen genetic loci outside of the human leukocyte antigen (HLA) had been reproducibly associated with polygenic traits; since then, over 1100 genetic loci have been associated with over 165 traits. The key to this progress was the advent of the GWAS, which allowed polygenic traits to be mapped at a rate that was previously unimaginable. However, while GWASs have led to breathtaking progress in our understanding of the mechanisms of disease, their clinical applicability has been disappointing to many. The central issue is that the ORs of the vast majority of discovered loci are small, generally less than 1.5. Moreover, even in aggregate, the loci that have been associated with most diseases seem to explain only a small fraction of the observed heritability. This does not by any means imply that the discovered loci are biologically unimportant; for example, the HMGCR locus whose gene product is the target of statins has a common variant that only affects LDL by a modest 2.8 mg/dL, yet it is clearly an important locus in the biology of lipid metabolism. Instead, it suggests that many of the variants discovered through GWASs are weakly penetrant, and that it is their biologic relevance, rather than their clinical utility, that is of greatest importance.
Thus, even though a large number of loci have been discovered, and even though the studies have been performed in very large sample sizes (often in over 100,000 individuals), only a small amount of genetic risk is currently explained. For example, in type 2 diabetes mellitus (T2DM), 39 loci have been associated with the disease as of early 2011. Together, these loci explain at most approximately 25% of the heritability of the disease. Given that the disease is itself only about 60% heritable (although this can vary depending on the characteristics of the sampled population), this implies that at most approximately 15% of variance in disease incidence can be explained by these 39 loci, a fraction that is too small to be clinically useful in predicting who is most at risk for T2DM.
It is currently unclear where the “missing heritability” lies for polygenic traits. GWASs performed to date have focused on common variants, and it is possible that there are other, rarer variants of larger effect that explain the missing heritability. For example, an intriguing recent study by Alkuraya and colleagues discovered that rare but highly penetrant homozygous mutations in the gene DNASE1L3 cause a disease that phenocopies systemic lupus erythematosus. Similarly, it is possible that interactions among genes or between genes and the environment will explain the bulk of the missing heritability. Finally, many postulate that modifications to DNA (“epigenetics”), rather than DNA variation itself, will be more important than previously realized.
Thus, despite the success of GWASs as a tool for discovering the mechanisms of disease, translating these discoveries into risk profiling has proven more difficult. The following examples demonstrate cases where people have attempted risk profiling for polygenic traits, highlighting both successful and unsuccessful attempts.
Example 1: Type 2 diabetes and limitations to the use of genetics in risk prediction
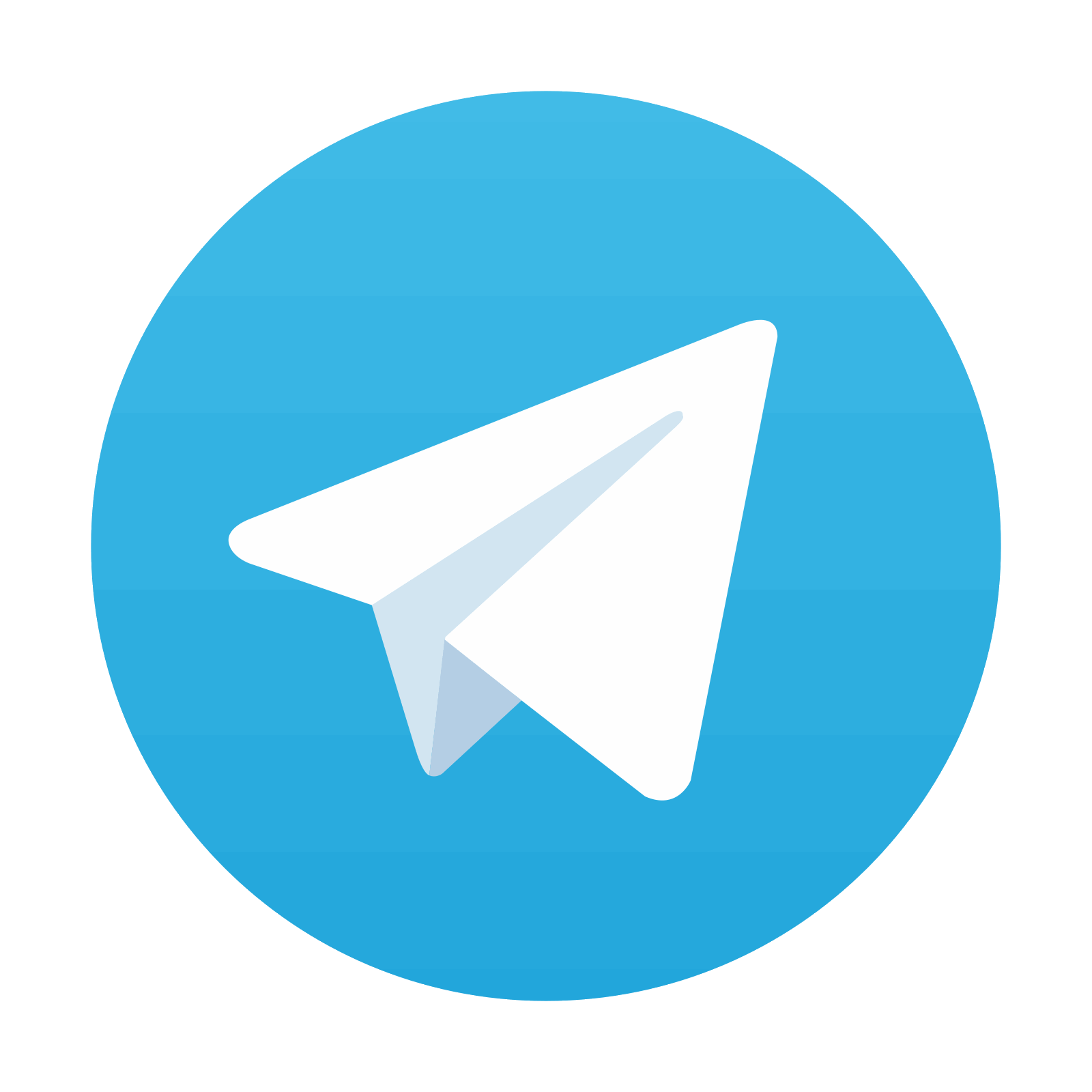
Stay updated, free articles. Join our Telegram channel

Full access? Get Clinical Tree
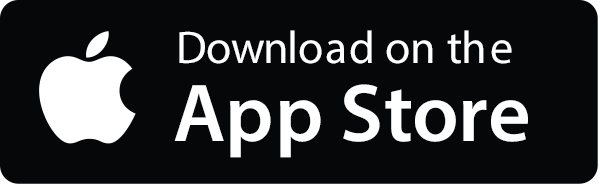
